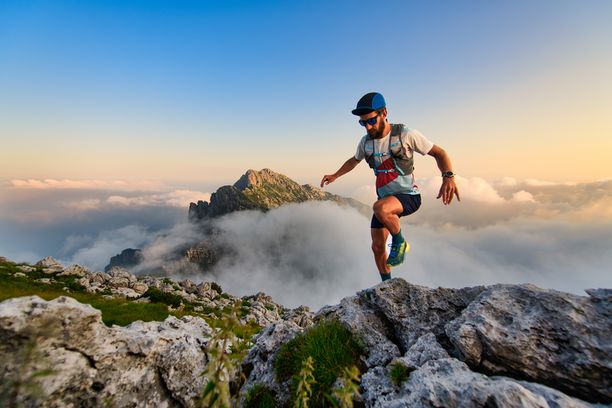
94% of researchers rate our articles as excellent or good
Learn more about the work of our research integrity team to safeguard the quality of each article we publish.
Find out more
ORIGINAL RESEARCH article
Front. Nutr., 16 June 2022
Sec. Food Chemistry
Volume 9 - 2022 | https://doi.org/10.3389/fnut.2022.940404
This article is part of the Research TopicNutrition and Chemistry of Cereal Macromolecules in Cereal-based ProductsView all 10 articles
Diabetic kidney disease (DKD) is an important complication of diabetes. The prevention of DKD can effectively reduce the mortality rate of diabetic patients and improve their quality of life. The present study examined the effects of fermented and germinated foxtail millet whole grain (FG-FM) on kidney lesions in a diabetic mouse model (Db/Db mice). The results proved that the FG-FM consumption significantly alleviated the kidney tissue damage in the diabetic mouse model. The transcriptome analysis of kidney tissues demonstrated that the overactivation of signaling pathways related to inflammation and immunity in the diabetic mouse model was significantly inhibited with the FG-FM intake. Moreover, the consumption of the FG-FM diet effectively elevated the bacterial diversity, increased the relative abundance of probiotics and decreased the relative abundance of previously reported DKD-related bacteria in the gut microbiota of diabetic mice. Our study confirmed foxtail millet as a potential source of functional food for the non-pharmacological intervention of DKD.
Diabetes mellitus (DM) is a chronic metabolic disease with a dramatically increased incidence globally in the past decades. Over one million deaths are directly attributed to DM each year (1), and the main factors responsible for DM-related mortality are diabetes complications (2). Diabetic kidney disease (DKD) is one of the major complications of diabetes and the leading cause of chronic kidney disease and 20%-40% of diabetic patients have combined diabetic kidney disease (3, 4). The population survey showed that kidney disease is an important risk factor accounting for the increased mortality in patients with type 2 diabetes (5). Although the progress in pathology research has facilitated the study of target drug discovery, there is still no ideal drug for DKD because the success rate of these compounds in clinical trials has been disappointingly low (6). Therefore, the development of non-pharmacological DKD intervention is of great significance for improving both length and quality of life in the diabetic population.
The growing evidence support that gut microbiota plays a pivotal role in the pathogenesis of DKD. As a complex ecosystem with 100 trillion microbes, the gut microbiota executes a wide range of important biological functions, including nutrient absorption and metabolism, vitamin production, regulation of development, resistance to pathogens, and maintenance of immune homeostasis (7–11). Significant changes in the gut microbiota have been confirmed in a variety of diseases, including obesity, DM, DKD, inflammatory bowel disease, cardiovascular disease, and cancer (12–14). The composition of gut microbiota in the DKD patients was significantly different from that of non-DKD diabetes patients (15). A marked expansion of bacterial communities at different classification levels, including Proteobacteria, Selenomonadales, Neosynechococcus, Shigella, Escherichia coli, etc., were found in DKD patients when compared to diabetic patients without kidney disease (15). Consequently, the gut microbiota dysbiosis induces intestinal barrier damage, prompts the translocation of endotoxin and other toxins, aggravates the system inflammatory levels, and finally contributes to kidney injury (16–18). Moreover, Cai et al. also reported that the proportion of short chain fatty acids (SCFAs)-producing bacteria in gut microbiota was decreased in DKD patients, and the oral supplement of SCFAs could improve kidney injury in the diabetic mouse model (19). These studies suggested that targeting the gut microbiota could be a novel strategy for the prevention of DKD.
Diet is a direct and effective factor affecting the gut microbiota (20). The foxtail millet (Setaria italica) is one of the major food crops in northern China, which is rich in dietary fiber, minerals, vitamins, and proteins (21–23). Previously studies have proved that foxtail millet has anti-inflammatory property and may prevent chronic diseases such as atherosclerosis and diabetes (24, 25). Moreover, animal studies proved that the intake of cereal bran effectively promotes beneficial bacteria and increases the production of SCFAs in gut microbiota (26). Thus, foxtail millet can serve as an important source of prebiotics. In our previous study, the effects of different pretreated foxtail millet cereal flour on an acute colitis mouse model with severe gut microbial dysbiosis were studied (20). The results showed that the fermented and germinated foxtail millet whole grain (FG-FM) cereal flour had the strongest prebiotic function and could almost completely restore the gut microbiota disorder in the colitis mouse model (20). Our findings coincide with studies that have shown that germination or fermentation can improve the prebiotic performance of whole grain (27–29).
Based on the fact that the gut microbiota plays an important role in the pathogenesis of DKD, as well as the powerful prebiotic properties of the FG-FM, we hypothesize that FG-FM has an impact on the prevention or treatment of DKD. In the current study, we tested the effects of fermented and germinated foxtail millet whole grain (FG-FM) intake on diabetes-related kidney injuries in genetically diabetic C57BL/KsJ-db/db (Db/Db) mice and explored its impact on gut microbiota. Our study provides a cost-effective non-pharmacological strategy for the prevention or treatment of DKD.
The foxtail millet seeds were provided by the Crop Institute of Shandong Academy of Agricultural Sciences. The FG-FM cereal flour used for the animal study was produced from foxtail millet seeds as described previously (20). In brief, foxtail millet seeds were soaked in tap water for 12 h at room temperature. After the water was drained, the seeds were left to sprout at room temperature for 24 h. The germinated seeds were dried, ground, and then passed through an 80-mesh sieve to produce germinated whole-grain foxtail millet flour. The germinated whole grain foxtail millet flour was mixed with water (1:2), cooked for 10 min in a 75 ± 5°C water bath, and then fermented with Lactobacillus Plantarum NBRC 15,891 (obtained from NITE Biological Resource Center) at 37°C. The resulting slurry was dried, ground, and then passed through an 80-mesh sieve to produce germinated and fermented foxtail millet whole grain (FG-FM) cereal flour used in this study. The prepared FG-FM cereal flour contained 58.54% starch, 10.87% protein, 2.85% fat, 19.41% dietary fiber,5.47% moisture, and 2.87% ash.
The FG-FM cereal flour diet was designed based on the AIN-93M standard rodent formula. The cereal flour diet contained 50% FG-FM cereal flour, and the remaining 50% was supplemented with standard nutrients according to the AIN-93M formula. The control diet in the study was a standard AIN-93M rodent diet. The animal diets were prepared by Nantong Troffe feed Technology Co., Ltd (Jiangsu, China), and the detailed compositions of experimental diets were listed in the Supplementary Table S1.
The 8–10 weeks old male genetically diabetic C57BL/KSJ-db/db (Db/Db) mice and their non-diabetic littermates C57BL/KSJ-m+/+db (Db/m) were obtained from GemPharmatech Co. Ltd. (Nanjing, China). The mice were housed in a specific pathogen-free facility (12 h daylight cycle) with ad libitum access to food and water, and the body weights were recorded every week. After 2 weeks of acclimation, mice were assigned into three groups (10 mice/group) with two different genotypes and two dietary treatments: 1) Db/m mice fed a standard AIN-93M rodent diet (CTRL group); 2) Db/Db mice fed a standard AIN-93M rodent diet (Db-93M group); 3) Db/Db mice fed an FG-FM cereal flour-based diet (Db-FM). The animal protocol was approved by the Institutional Animal Care and Use Committees of the Qilu Hospital of Shandong University.
After 8 weeks on a diet, the mice were anesthetized with isoflurane inhalation and then terminated by cervical dislocation. By opening the abdomen cavity, the large intestine was removed and placed on an ice plate. Then cecal content was collected, snap-frozen in liquid nitrogen, and stored at −80°C until further analysis. The kidneys were removed and weighed, one of the kidneys was snap-frozen in liquid nitrogen and stored at −80°C for further total RNA extraction and transcriptome analysis, the other was processed for paraffin embedding, cut into 5 μm sections, and finally stained with hematoxylin and eosin (H&E) for histopathological analysis. The pathological features of liver tissue were also analyzed by H&E staining. Other tissues were removed and weighed, including the spleen and epididymal fat.
Total bacterial DNA was extracted from frozen cecal contents using a QIAamp DNA Stool Mini Kit (Qiagen, Valencia, CA). The region V3–V4 of the 16S rRNA gene was amplified, purified, and quantified sequentially. Then the DNA libraries were constructed following the manufacturer's instructions. After quality inspection, the constructed DNA library was sequenced with Illumina HiSeq 2,500 platform (Illumina, Inc, San Diego, California). The resulting pair-end reads were joined by fastq-join (Version 1.3.1, https://code.google.com/p/ea-utils/) and pear (30), and then cut and quality filtered by Cutadapt (version 1.18) (31) to obtain clean tags. After that, the resulting clean tags were assigned to OTUs using USEARCH (Version 11.0.667, http://www.drive5.com/usearch/) with a 97% threshold of pairwise identity. The OTUs were then aligned against the Silva database (Release132, http://www.arb-silva.de) (32). QIIME (33) software was used to generate an information table of the relative abundance of bacterial communities at different classification levels, and then the R software was used to plot the community structure at each taxonomic level of the sample. The linear discriminant analysis (LDA) effect size (LEfSE, https://huttenhower.sph.harvard.edu/galaxy/) was applied to identify bacterial communities responsible for the differences in cecal microbiota compositions between different groups, using an LDA score threshold of >4.0. The raw Illumina read data were uploaded into SRA at NCBI under the BioProject ID PRJNA835687.
Total RNA was extracted from frozen kidney tissue, and the concentration and quality of extracted total RNA were determined by Nanodrop 2000, agarose gel electrophoresis, and Agilent 2100 bioanalyzer (Agilent, Santa Clara, CA, USA) in order. After the mRNA was purified from total RNA using Oligo (dT) beads (NEB, San Diego, CA, USA). The Illumina TruseqTM RNA sample prep Kit was used for sequencing library preparation based on purified mRNA samples. The resulting library fragments were quantified using an Agilent High Sensitivity DNA assay on a Bioanalyzer 2100 system (Agilent, Santa Clara, CA, USA) for concentration and size distribution, and the molar concentration of DNA libraries was analyzed by q-PCR using KAPA SYBR FAST Universal 2X qPCR Master Mix and DNA Quantification Standards and Primer Premix Kit (KAPA Biosystems, Woburn, MA, USA). The prepared libraries were mixed proportionally, then sequenced using an Illumina Novaseq 6000 platform (read length 2 × 150 bp).
After adaptor removal, quality and size trimming, low complexity filtering, and ribosomal RNA (rRNA) removal of raw data, the resulting clean reads were mapped to the Mus musculus reference genome using the program HISAT2 (https://ccb.jhu.edu/software/hisat2/index.shtml) (34). After assessment of mapping results, the transcripts were assembled and annotated using Cufflinks (https://cole-trapnelllab.github.io/cufflinks/) (35) or StringTie (https://ccb.jhu.edu/software/stringtie/) (36). Then, read counts of annotated transcripts (genes) were calculated using RSEM (37), and then transformed into FPMK (fragments per kilobases per million fragments) values for further analysis. Differentially expressed genes (DEGs) were analyzed using DESeq2 or edgeR software (38, 39). GO (Gene Ontology) and KEGG (Kyoto Encyclopedia of Genes and Genomes) enrichment analysis of DEGs was performed with Goatools (https://github.com/tanghaibao/GOatools) (40) and R software.
One-way analysis of variance (ANOVA) followed by Fisher's LSD test (GraphPad Software, Inc., La Jolla, California) was used for multiple comparisons. The results were considered statistically significant when p < 0.05. Data were expressed as means ± SEM.
As demonstrated in Figure 1A, the body weights of Db/Db mice (Db-93M group and Db-FM group) were significantly higher than that of Db/m mice (CTRL group) due to the genotypic difference. In the first 4 weeks on diets, the body weights of both groups of Db/Db mice (Db-93M and Db-FM) increased with no significant difference observed between groups. However, from the 5th week, the body weights of the mice in the Db-93M group began to decline, resulting in significantly lower body weights of the mice in the Db-93M group than those of mice in the Db-FM group. Consistent with body-weight loss, the epididymal fat weights of mice from the Db-93M group were significantly lower than that of the Db-FM group (Figure 1B, p = 0.0127). On the contrary, the kidney weights of mice from the Db-93M group were significantly higher than those from the Db-FM group (p < 0.0001, Figure 1C). Liver and kidney weights did not differ significantly between Db-93M and Db-FM groups.
Figure 1. Physiological parameters. (A) Body weight changes during 8 weeks on diets, asterisks indicate significant differences between the Db-93M group and the Db-FM group (*p < 0.05, **p < 0.01). (B) Epididymal fat weights comparison. (C) Kidney weights comparison. (D) Liver weights comparison. (E) Spleen weights comparison. Data are expressed as the mean ± SEM.
The experimental mice were executed after 8 weeks on diets. It was found that the kidneys in the CTRL group (Figure 2A) were normal in appearance and morphology while in both groups of Db mice, the kidney was surrounded by a large amount of fat. Six of the total nine mice in the Db-93M group had severe lesions in at least one of the kidneys (Figure 2B, right; Figure 2C), while the kidney of mice in the Db-FM group showed no morphological abnormalities (Figure 2B, left). The result of H&E staining (Figure 2D) clearly showed that the normal micro-structure of the kidney tissues was almost destroyed in the Db-93M group. In contrast, in the CTRL and Db-FM groups, the micro-structure of kidney tissues remained intact. These results together suggested that the FG-FM diet significantly alleviated kidney injury in the diabetic mouse model.
Figure 2. Histopathological analysis. (A) The appearance and morphology of the kidneys from CTRL group; (B) the appearance and morphology of the kidneys, the left one is the kidney from the Db-FM group, the right one is the kidney from the Db-93M group; (C) the kidney of mice from the Db-93M group; (D) histopathological examination of kidney tissues (top: 40 ×; bottom: 200 ×); (E) histopathological examination of liver tissues (top: 40 ×; bottom: 200 ×).
The kidney lesions of the mice in the Db-93M group were also associated with weight loss in the later period of feeding (from the 5th week onwards), due to the weight loss is an important feature of a mouse model with kidney disease. The Db/Db mice (Db-93M and Db-FM) had obvious fat droplets formation in the liver tissue compared to the CTRL group (Figure 2E).
An average of 50.38 ± 0.31, 52.23 ± 0.40, and 50.72 ± 0.34 million clean reads were obtained for the kidney tissues in mice from CTRL, Db-93M, and Db-FM groups, respectively. The clean reads were mapped to the mouse reference genome (http://asia.ensembl.org/Mus_musculus/Info/Index) with high proportions: CTRL group, 96.41%; Db-93M group, 96.94%; and Db-FM group, 96.93%.
The significantly differentially expressed genes (DEGs) were identified based on the quantification and comparison of gene expression levels of kidney tissues in mice from CTRL, Db-93M, and Db-FM groups. As shown in Figure 3, when comparing the groups with different genotypes fed with the same AIN-93M diet (CTRL vs. Db-93M), 4,112 DEGs, including 3,187 upregulated and 925 downregulated genes, were identified. When comparing the Db/Db groups fed with different diets (Db-93M vs. Db-FM), 2,800 DEGs, including 226 upregulated and 2,574 downregulated genes, were identified. Surprisingly, two groups of mice with different genotypes and different feeding (CTRL vs. Db-FM) showed minimal differences. Only 984 DEGs were identified, including 486 upregulated and 498 downregulated genes.
Figure 3. The gene expression analysis performed by RNA-sequencing (RNA-Seq, n = 6). (A) Numbers of differential expressed genes (DEGs) in kidney tissue of different groups; the volcanic maps for DEGs in (B) CTRL vs. Db-93M group; the red dots were up-regulated, and the green dots were down-regulated in Db-93M group when compared to CTRL group. (C) CTRL vs. Db-FM groups; the red dots were up-regulated, and the green dots were down-regulated in the Db-FM group when compared to the CTRL group. (D) Db-93M vs. Db-FM group; the red dots were up-regulated, and the green dots were down-regulated in the Db-FM group when compared to the Db-93M group.
The KEGG enrichment analyses were performed to identify biological pathways significantly affected by different treatments. As shown in Figure 4A, when comparing the CTRL group and the Db-93M group, several signaling pathways related to inflammation, infection, and immunity were affected, including cytokine-cytokine receptor interaction pathway, natural killer mediated cytotoxicity pathway, B cell receptor signaling pathway, NF-κB signaling pathway, etc. The comparison between the Db-93M group and the Db-FM group also showed similar characteristics (Figure 4C). However, the affected signaling pathways in the comparison between the CTRL group and the Db-FM group did not show obvious associations with inflammation and immunity biological process (Figure 4B). Furthermore, among the top 20 most significant pathways resulting from KEGG enrichment analysis, 15 signaling pathways contained more than 100 DEGs in the comparison between CTRL and Db-93M, and also 15 in the comparison between Db-93M and Db-FM. Notably, none of the signaling pathways had more than 100 DEGs in the comparison between CTRL and Db-FM, and even the most affected signaling pathway contained only 36 DEGs. This also proved that, from the perspective of the transcriptome, the difference between the CTRL group and the Db-FM group was relatively inconspicuous, while the Db-93M group was significantly different from the other two groups.
Figure 4. The KEGG enrichment results (kidney tissues) based on the identified DEGs from. (A) Comparison of CTRL group and Db-93M group. (B) Comparison of CTRL group and Db-FM group. (C) Comparison of Db-93M group and Db-FM group.
When further analyzing the details of DEGs, a clear trend was observed. The changes that occurred in the Db-93M group (compared to the CTRL group) could be corrected in the Db-FM group. Take the cytokine-cytokine receptor interaction pathway as an example (Supplementary Figure S1), the abundance of chemokines and their receptors, including CCLs and CXCLs, as well as inflammatory cytokines and corresponding receptors, were significantly elevated in the Db-93M group when compared to CTRL group (Supplementary Figure S1A). Simultaneously, most of these upregulated genes were found to be significantly downregulated in the Db-FM group, when compared to the Db-93M group (Supplementary Figure S1B). Subsequently, the overactivation of the downstream signaling pathway, such as the NF-κB signaling pathway caused by the overexpression of chemokines and inflammatory cytokines in the Db-93M group was also normalized in the Db-FM group (Supplementary Figure S2). In addition, other signaling pathways related to inflammation and immunity also showed a similar trend, which was overactivated in Db-93M, and this overactivation was re-inhibited in the Db-FM group (Supplementary Figures S3, S4, and S5). Taken together, these results showed that intake of FG-FM could significantly alleviate kidney damage in diabetic mice by inhibiting the overactivation of signaling pathways in inflammation, infection, or immunity in the kidney.
The microbial species richness (Chao, Supplementary Figure 5A) and diversity (Shannon, Supplementary Figure 5B) index were calculated to analyze the effect of FG-FM on the gut microbiota of Db/Db mice. There was no significant difference in the Chao index among the three groups, indicating that neither the genotypic difference nor FG-FM had a significant effect on the bacterial species richness of the gut microbiota. Moreover, no significant variations in the species diversity between the CTRL group and Db/Db groups were evidenced by the Shannon diversity index (p = 0.17). However, the Shannon index of Db-FM mice was significantly higher than that of Db-93M mice (p = 0.0061), indicating that FG-FM significantly enhanced the bacterial species diversity of gut microbiota in Db/Db mice.
The shared and specific OTUs analysis was demonstrated by the Venn diagram in Figure 5C. The gut microbiota of mice from the CTRL group had the largest number of unique OTUs (174), followed by that of the Db-FM group (144). Moreover, except for the OTUs shared by three groups (1093), the CTRL group and Db-FM had 432 shared OTUs, while the Db-93M group only shared 68 OTUs with the CTRL group and 55 OTUs with the Db-FM group. From these results, the intake of FG-FM could effectively change the gut microbiota of Db/Db mice, making them have more common microbiota characteristics with the CTRL group.
Figure 5. The gut microbiota composition analysis. (A) The microbial richness index of Chao. (B) The microbial diversity index of Shannon. (C) Venn diagrams that illustrated observed overlap of OTUs from different groups. (D) The composition of gut microbiota in mice at phylum level. (E) The composition of gut microbiota in mice at the genus level. “Others” represent the sum of all the phyla/genera, which abundances were under 1%. Data are expressed as the mean ± SEM.
As illustrated in Figures 5D,E, gut microbiota composition varied in different groups. At the phylum level, the most abundant bacterial taxa was Firmicutes (CTRL, 43.90%; Db-93M, 41.93%; Db-FM, 43.60%) in all the three groups. The second dominant phylum was Bacteroidetes in gut microbiota (CTRL, 23.61%; Db-93M, 23.78%; Db-FM, 27.66%). Whereas in the Db-93M group, the relative abundance of phylum Proteobacteria (21.52%) increased dramatically, far more than the proportion of Proteobacteria in the gut microbiota from the other two groups (CTRL, 16.83%; Db-FM, 10.70%). At the genus level, the most abundant bacterial taxa in the CTRL group was Akkermansia (CTRL, 10.59%), followed by the Lachnospiraceae NK4A136 group (5.42%); in the Db-93M group, the most abundant bacterial taxa was Lachnospiraceae NK4A136 group (7.78%), followed by genus Blautia (6.58%); in Db-FM group, the most abundant bacterial taxa was Mucispirillum (11.32%), followed by genus Lachnospiraceae NK4A136 group (9.90%).
Taken together, these results showed that the consumption of FG-FM could effectively increase the bacterial diversity and reduce the proportion of phylum Proteobacteria in the gut microbiota of Db/Db mice. These prebiotic properties may contribute to preventing kidney disease in the diabetic mouse model.
The effects of FG-FM on the gut microbiota of Db/Db mice were further analyzed by LFfSe (LDA Effect Size), and the results (LEfSe Cladogram and histogram of LDA scores) were demonstrated in Figures 6A,B. By comparing the gut microbiota of the Db-93M and Db-FM groups, a total of 57 different bacterial taxa were found, including 22 dominant bacterial taxa in the gut microbiota of the Db-FM group and 35 dominant bacterial taxa in the Db-93M group. The relative abundances of several commensal bacteria that have been reported contributing to inflammation control and disease prevention were significantly higher in the Db-FM group than in the Db-93M group. For example, Ruminococcaceae (41), Odoribacter (42), Ileibacterium (43), Lachnospiraceae NK4A136 (44), Rikenellaceae RC9 gut group (45), and Mucispirillum schaedleri (46). The details of representative taxa are shown in Figure 6C. Furthermore, the important “signature” bacteria of dysbiosis in gut microbiota, including Proteobacteria and Escherichia-Shigella (47–49) were found to be significantly enriched in the Db-93M group. The results of the LEfSe analysis further validated the prebiotic characteristics of FG-FM cereal flour, including promoting the proliferation of probiotics and limiting the expansion of previously reported DKD-related bacteria in the gut microbiota of diabetic mouse model (15).
Figure 6. LEfSe analysis of gut microbiota. (A) Effect size of significantly enriched taxa in each group when performing a comparison between Db-93M group and Db-FM group. (B) Taxonomic cladogram obtained using LEfSe analysis result from the comparison between Db-93Mgroup and Db-FM group. (C) Relative abundance of representative bacterial taxa in the gut microbiota of Db-93M group and Db-FM group identified by LEfSe analysis.
A substantial proportion of diabetic patients will develop DKD, which is the leading cause of chronic kidney disease and even end-stage kidney disease globally (50). Dietary and nutritional factors play key roles in the progression of DKD (51, 52). Foxtail millet is a typical underutilized crop which has received less attention than main crops. In the current study, we have identified a novel function of FG-FM in preventing kidney damage associated with diabetes mellitus. The FG-FM diet significantly alleviated the morphological lesions of the kidney as well as the overactivation of inflammatory and cytokine signaling pathways in the kidney of diabetic mice. Moreover, the gut microbiota analysis revealed the FG-FM diet as an excellent prebiotic in the prevention of kidney disease. Our study provided a novel non-pharmacological intervention strategy for Diabetic kidney disease (DKD)
Transcriptomics analysis revealed that a large number of genes involved in inflammatory and immune signaling pathways were overactivated in the kidney tissues of Db-93M mice, indicating a high level of inflammation in the kidney of diabetic mice. In addition, we also observed severe kidney lesions in these mice when no special diet intervention was applied. These results clearly demonstrated the development of DKD in diabetic mice. However, the FG-FM intervention protected the kidney from developing both the high levels of inflammation and the morphological damage in the diabetic mice. Inflammation is a well-established feature of DKD, manifested as the increased level of inflammatory cytokines in the serum of DKD patients, as well as anti-inflammatory treatments are effective in the prevention of DKD (53, 54). Notably, we found that the transcription of chemokines and their receptors, including CCLs and CXCLs, as well as several inflammatory cytokines, such as IL1β and TNFα, were significantly upregulated in the kidney of diabetic mice, while such elevation was not observed in mice fed with the FG-FM diet. In support of our results, the overexpression of CCLs, CXCLs, and inflammatory cytokines has been proved to be associated with DKD or other kidney diseases (55–57) and has been widely used to induce kidney injury in animal models (58–60). Besides chemokines, the over expression of several inflammatory cytokines, such as IL1β and TNFα, have been identified as biomarkers in diabetic kidney disease (61). Their overexpression is not only an indicator of kidney inflammation but also a trigger for further deterioration of renal lesions (62). Therefore, the suppression of these hyperactivated inflammatory or immune-related cytokines and signaling pathways in the Db-FM group could strongly explain the rescue effects of FG-FM on kidney lesions in diabetic mice. Our findings not only confirmed the function of the FG-FM diet at the transcriptional level, but also partially explained its molecular mechanism for relieving renal lesions.
Consistent with the alleviated DKD symptoms in Db-FM groups, improvement in the gut microbiota was also identified. In line with what we found, the reduction of bacterial diversity has been reported previously exist in patients (63, 64) and animal models (65) with kidney disease. We, therefore, speculate that the promotion of microbial diversity index may be attributed to the prebiotic properties of FG-FM and beneficial for the alleviation of nephropathy symptoms in diabetic mice (66). The proliferation of probiotics in the gut microbiota of the Db-FM group is also important evidence of the prebiotic function of FG-FM. For example, the relative abundance of several SCFAs producing bacteria, such as Odoribacter, Lachnospiraceae NK4A136, and Rikenellaceae RC9 gut group (67–69), was obviously increased by FG-FM ingestion. SCFAs have the ability to ameliorate diabetic nephropathy via inhibition of the NF-κB signaling pathway (70). Coincidentally, the transcriptome analysis displayed that the overactivation of the NF-κB signaling pathway in the Db-93M group was normalized in the Db-FM group. Furthermore, the unusual expansion of Proteobacteria and Escherichia-Shigella has been considered as common features of the imbalanced gut microbiota in different inflammatory-related diseases (49, 71, 72), and the decrease in the proportion of Proteobacteria and Escherichia-Shigella in the gut microbiota of mice in the Db-FM group could be regarded as a sign of the improved gut microbiota. Taken together, our study proved that FG-FM could inhibit the overactivation of inflammatory signaling pathways via restoring the pro-inflammatory characteristics of gut microbiota, thereby preventing the occurrence of renal lesions in diabetic mice.
In conclusion, our study demonstrated that diet intervention with fermented and germinated foxtail millet whole grain (FG-FM) could effectively prevent and protect DKD in the diabetic mouse model. At the same time, we revealed the excellent prebiotic function of FG-FM in preventing kidney disease. The promising results from our animal study provide a non-pharmacological strategy for preventing DKD through functional foods developed based on foxtail millet whole grain.
The datasets presented in this study can be found in online repositories. The names of the repository/repositories and accession number(s) can be found below: NCBI SRA; PRJNA835687.
The animal study was reviewed and approved by Institutional Animal Care and Use Committees of the Qilu Hospital of Shandong University.
Conceptualization, data curation, and validation: DZ and LL. Methodology: XLiu, WL, and BQ. Investigation: XLiu, WL, BQ, YZ, and XW. Writing-original draft preparation: XLiu and WL. Writing-review and editing: DZ and XLi. Project administration: DZ. Funding acquisition: DZ and BQ. All authors have read and agreed to the published version of the manuscript.
This research was funded by National Science Foundation of China grants (82071512), Shandong Provincial Natural Science Foundation grant (ZR2019ZD33), Shandong Provincial Key Research and Development program (2019GHZ031), and Research Project of Jinan Microecological Biomedicine Shandong Laboratory (JNL-2022003A to XLi).
The authors declare that the research was conducted in the absence of any commercial or financial relationships that could be construed as a potential conflict of interest.
All claims expressed in this article are solely those of the authors and do not necessarily represent those of their affiliated organizations, or those of the publisher, the editors and the reviewers. Any product that may be evaluated in this article, or claim that may be made by its manufacturer, is not guaranteed or endorsed by the publisher.
The Supplementary Material for this article can be found online at: https://www.frontiersin.org/articles/10.3389/fnut.2022.940404/full#supplementary-material
1. Organization. WH. Diabetes (10 November 2021). Available online at: https://www.who.int/health-topics/diabetes#tab=tab_1
2. Papatheodorou K, Banach M, Bekiari E, Rizzo M, Edmonds M. Complications of Diabetes 2017. J Diabetes Res. (2018) 2018:3086167. doi: 10.1155/2018/3086167
3. Afkarian M, Zelnick LR, Hall YN, Heagerty PJ, Tuttle K, Weiss NS, et al. Clinical manifestations of kidney disease among us adults with diabetes, 1988–2014. JAMA. (2016) 316:602–10. doi: 10.1001/jama.2016.10924
4. Srivastava SP, Zhou H, Setia O, Liu B, Kanasaki K, Koya D, et al. Loss of endothelial glucocorticoid receptor accelerates diabetic nephropathy. Nat Commun. (2021) 12:2368. doi: 10.1038/s41467-021-22617-y
5. Afkarian M, Sachs MC, Kestenbaum B, Hirsch IB, Tuttle KR, Himmelfarb J, et al. Kidney disease and increased mortality risk in type 2 diabetes. J Am Soc Nephrol. (2013) 24:302–8. doi: 10.1681/ASN.2012070718
6. Koya D, Araki S, Haneda M. Therapeutic management of diabetic kidney disease. J Diabetes Investig. (2011) 2:248–54. doi: 10.1111/j.2040-1124.2011.00112.x
7. Backhed F, Ley RE, Sonnenburg JL, Peterson DA, Gordon JI. Host-bacterial mutualism in the human intestine. Science. (2005) 307:1915–20. doi: 10.1126/science.1104816
8. Upadhyaya S, Banerjee G. Type 2 Diabetes and gut microbiome: at the intersection of known and unknown. Gut Microbes. (2015) 6:85–92. doi: 10.1080/19490976.2015.1024918
9. Saxena R, Sharma VK. Chapter 9 - a Metagenomic Insight into the Human Microbiome: Its Implications in Health and Disease. In: Kumar D, Antonarakis S, editors. Medical and Health Genomics. Oxford: Academic Press (2016). p. 107–19.
10. Yu LC. Microbiota Dysbiosis and barrier dysfunction in inflammatory bowel disease and colorectal cancers: exploring a common ground hypothesis. J Biomed Sci. (2018) 25:79. doi: 10.1186/s12929-018-0483-8
11. Wu HJ, Wu E. The role of gut microbiota in immune homeostasis and autoimmunity. Gut Microbes. (2012) 3:4–14. doi: 10.4161/gmic.19320
12. Ni J, Wu GD, Albenberg L, Tomov VT. Gut microbiota and ibd: causation or correlation? Nat Rev Gastroenterol Hepatol. (2017) 14:573–84. doi: 10.1038/nrgastro.2017.88
13. Tremaroli V, Backhed F. Functional interactions between the gut microbiota and host metabolism. Nature. (2012) 489:242–9. doi: 10.1038/nature11552
14. Cheng WY, Wu CY Yu J. The role of gut microbiota in cancer treatment: friend or foe? Gut. (2020) 69:1867–76. doi: 10.1136/gutjnl-2020-321153
15. He X, Sun J, Liu C, Yu X, Li H, Zhang W, et al. Compositional alterations of gut microbiota in patients with diabetic kidney disease and type 2 diabetes mellitus. Diabetes Metab Syndr Obes. (2022) 15:755–65. doi: 10.2147/DMSO.S347805
16. Ramezani A, Raj DS. the gut microbiome, kidney disease, and targeted interventions. J Am Soc Nephrol. (2014) 25:657–70. doi: 10.1681/ASN.2013080905
17. Koppe L, Fouque D, Soulage CO. Metabolic abnormalities in diabetes and kidney disease: role of uremic toxins. Curr Diab Rep. (2018) 18:97. doi: 10.1007/s11892-018-1064-7
18. Salguero MV, Al-Obaide MAI, Singh R, Siepmann T, Vasylyeva TL. Dysbiosis of gram-negative gut microbiota and the associated serum lipopolysaccharide exacerbates inflammation in type 2 diabetic patients with chronic kidney disease. Exp Ther Med. (2019) 18:3461–9. doi: 10.3892/etm.2019.7943
19. Cai K, Ma Y, Cai F, Huang X, Xiao L, Zhong C, et al. Changes of gut microbiota in diabetic nephropathy and its effect on the progression of kidney injury. Endocrine. (2022) 76:294–303. doi: 10.1007/s12020-022-03002-1
20. Kolodziejczyk AA, Zheng D, Elinav E. Diet-microbiota interactions and personalized nutrition. Nat Rev Microbiol. (2019) 17:742–53. doi: 10.1038/s41579-019-0256-8
21. Devi PB, Vijayabharathi R, Sathyabama S, Malleshi NG. Priyadarisini VB. Health benefits of finger millet (Eleusine Coracana L) polyphenols and dietary fiber: a review. J Food Sci Technol. (2014) 51:1021–40. doi: 10.1007/s13197-011-0584-9
22. Verma S, Srivastava S, Tiwari N. Comparative study on nutritional and sensory quality of barnyard and foxtail millet food products with traditional rice products. J Food Sci Technol. (2015) 52:5147–55. doi: 10.1007/s13197-014-1617-y
23. Dong JL, Wang L, Lu J, Zhu YY, Shen RL. Structural, Antioxidant and adsorption properties of dietary fiber from foxtail millet (Setaria Italica) bran. J Sci Food Agric. (2019) 99:3886–94. doi: 10.1002/jsfa.9611
24. Kam J, Puranik S, Yadav R, Manwaring HR, Pierre S, Srivastava RK, et al. Dietary interventions for type 2 diabetes: how millet comes to help. Front Plant Sci. (2016) 7:1454. doi: 10.3389/fpls.2016.01454
25. Liu F, Shan S, Li H, Li Z. Treatment of peroxidase derived from foxtail millet bran attenuates atherosclerosis by inhibition of Cd36 and Stat3 in vitro and in vivo. J Agric Food Chem. (2020) 68:1276–85. doi: 10.1021/acs.jafc.9b06963
26. Kumar A, Henderson A, Forster GM, Goodyear AW, Weir TL, Leach JE, et al. Dietary rice bran promotes resistance to salmonella enterica serovar typhimurium colonization in mice. BMC Microbiol. (2012) 12:71. doi: 10.1186/1471-2180-12-71
27. Chu J, Zhao H, Lu Z, Lu F, Bie X, Zhang C. Improved physicochemical and functional properties of dietary fiber from millet bran fermented by bacillus natto. Food Chem. (2019) 294:79–86. doi: 10.1016/j.foodchem.2019.05.035
28. Sharma S, Saxena DC, Riar CS. Changes in the gaba and polyphenols contents of foxtail millet on germination and their relationship with in vitro antioxidant activity. Food Chem. (2018) 245:863–70. doi: 10.1016/j.foodchem.2017.11.093
29. Sharma N, Alam T, Goyal SK, Fatma S, Pathania S, Niranajan K. Effect of different storage conditions on analytical and sensory quality of thermally processed, milk-based germinated foxtail millet porridge. J Food Sci. (2018) 83:3076–84. doi: 10.1111/1750-3841.14371
30. Zhang J, Kobert K, Flouri T, Stamatakis A. Pear: a fast and accurate illumina paired-end read merger. Bioinformatics. (2014) 30:614–20. doi: 10.1093/bioinformatics/btt593
31. Kechin A, Boyarskikh U, Kel A, Filipenko M. Cutprimers: a new tool for accurate cutting of primers from reads of targeted next generation sequencing. J Comput Biol. (2017) 24:1138–43. doi: 10.1089/cmb.2017.0096
32. Quast C, Pruesse E, Yilmaz P, Gerken J, Schweer T, Yarza P, et al. The silva ribosomal rna gene database project: improved data processing and web-based tools. Nucleic Acids Res. (2013) 41:D590–6. doi: 10.1093/nar/gks1219
33. Caporaso JG, Kuczynski J, Stombaugh J, Bittinger K, Bushman FD, Costello EK, et al. Qiime allows analysis of high-throughput community sequencing data. Nat Methods. (2010) 7:335–6. doi: 10.1038/nmeth.f.303
34. Kim D, Langmead B, Salzberg SL. Hisat: a fast spliced aligner with low memory requirements. Nat Methods. (2015) 12:357–60. doi: 10.1038/nmeth.3317
35. Trapnell C, Williams BA, Pertea G, Mortazavi A, Kwan G, van Baren MJ, et al. Transcript assembly and quantification by rna-seq reveals unannotated transcripts and isoform switching during cell differentiation. Nat Biotechnol. (2010) 28:511–5. doi: 10.1038/nbt.1621
36. Pertea M, Pertea GM, Antonescu CM, Chang TC, Mendell JT, Salzberg SL. Stringtie enables improved reconstruction of a transcriptome from Rna-Seq reads. Nat Biotechnol. (2015) 33:290–5. doi: 10.1038/nbt.3122
37. Li B, Dewey CN. Rsem: Accurate transcript quantification from Rna-Seq data with or without a reference genome. BMC Bioinformatics. (2011) 12:323. doi: 10.1186/1471-2105-12-323
38. Love MI, Huber W, Anders S. Moderated estimation of fold change and dispersion for Rna-Seq data with Deseq2. Genome Biol. (2014) 15:550. doi: 10.1186/s13059-014-0550-8
39. Robinson MD, McCarthy DJ, Smyth GK. Edger: a bioconductor package for differential expression analysis of digital gene expression data. Bioinformatics. (2010) 26:139–40. doi: 10.1093/bioinformatics/btp616
40. Klopfenstein DV, Zhang L, Pedersen BS, Ramirez F, Warwick Vesztrocy A, Naldi A, et al. Goatools: a python library for gene ontology analyses. Sci Rep. (2018) 8:10872. doi: 10.1038/s41598-018-28948-z
41. Schirmer M, Garner A, Vlamakis H, Xavier RJ. Microbial genes and pathways in inflammatory bowel disease. Nat Rev Microbiol. (2019) 17:497–511. doi: 10.1038/s41579-019-0213-6
42. Hiippala K, Barreto G, Burrello C, Diaz-Basabe A, Suutarinen M, Kainulainen V, et al. Novel odoribacter splanchnicus strain and its outer membrane vesicles exert immunoregulatory effects in vitro. Front Microbiol. (2020) 11:575455. doi: 10.3389/fmicb.2020.575455
43. Wang Y, Ablimit N, Zhang Y, Li J, Wang X, Liu J, et al. Novel Beta-Mannanase/Glp-1 fusion peptide high effectively ameliorates obesity in a mouse model by modifying balance of gut microbiota. Int J Biol Macromol. (2021) 191:753–63. doi: 10.1016/j.ijbiomac.2021.09.150
44. Li L, Bao J, Chang Y, Wang M, Chen B, Yan F. Gut microbiota may mediate the influence of periodontitis on prediabetes. J Dent Res. (2021) 100:1387–96. doi: 10.1177/00220345211009449
45. Hu C, Ding L, Jiang C, Ma C, Liu B, Li D, et al. Effects of management, dietary intake, and genotype on rumen morphology, fermentation, and microbiota, and on meat quality in yaks and cattle. Front Nutr. (2021) 8:755255. doi: 10.3389/fnut.2021.755255
46. Herp S, Brugiroux S, Garzetti D, Ring D, Jochum LM, Beutler M, et al. Mucispirillum schaedleri antagonizes salmonella virulence to protect mice against colitis. Cell Host Microbe. (2019) 25:681–94. doi: 10.1016/j.chom.2019.03.004
47. Thingholm LB, Ruhlemann MC, Koch M, Fuqua B, Laucke G, Boehm R, et al. Obese individuals with and without type 2 diabetes show different gut microbial functional capacity and composition. Cell Host Microbe. (2019) 26:252–64. doi: 10.1016/j.chom.2019.07.004
48. Baothman OA, Zamzami MA, Taher I, Abubaker J, Abu-Farha M. The role of gut microbiota in the development of obesity and diabetes. Lipids Health Dis. (2016) 15:108. doi: 10.1186/s12944-016-0278-4
49. Shin NR, Whon TW, Bae JW. Proteobacteria: microbial signature of dysbiosis in gut microbiota. Trends Biotechnol. (2015) 33:496–503. doi: 10.1016/j.tibtech.2015.06.011
50. Alicic RZ, Rooney MT, Tuttle KR. Diabetic kidney disease: challenges, progress, and possibilities. Clin J Am Soc Nephrol. (2017) 12:2032–45. doi: 10.2215/CJN.11491116
51. Li Q, Wen F, Wang Y, Li S, Lin S, Qi C, et al. Diabetic kidney disease benefits from intensive low-protein diet: updated systematic review and meta-analysis. Diabetes Ther. (2021) 12:21–36. doi: 10.1007/s13300-020-00952-5
52. Pereira RA, Ramos CI, Teixeira RR, Muniz GAS, Claudino G, Cuppari L. Diet in Chronic Kidney Disease: An Integrated Approach to Nutritional Therapy. Rev Assoc Med Bras. (1992) (2020):s59–s67. doi: 10.1590/1806-9282.66.S1.59
53. Garcia-Garcia PM, Getino-Melian MA, Dominguez-Pimentel V, Navarro-Gonzalez JF. Inflammation in diabetic kidney disease. World J Diabetes. (2014) 5:431–43. doi: 10.4239/wjd.v5.i4.431
54. Perez-Morales RE, Del Pino MD, Valdivielso JM, Ortiz A, Mora-Fernandez C, Navarro-Gonzalez JF. Inflammation in diabetic kidney disease. Nephron. (2019) 143:12–6. doi: 10.1159/000493278
55. Pawlak K, Kowalewska A, Mysliwiec M, Pawlak D. 3-Hydroxyanthranilic acid is independently associated with monocyte chemoattractant protein-1 (Ccl2) and macrophage inflammatory protein-1beta (Ccl4) in patients with chronic kidney disease. Clin Biochem. (2010) 43:1101–6. doi: 10.1016/j.clinbiochem.2010.06.008
56. Guo L, Lv J, Zhang J, Deng H, Feng S, Liu S, et al. Elevated serum Il-21 levels are associated with stable immune status in kidney transplant recipients and a mouse model of kidney transplantation. Aging. (2020) 12:18396–414. doi: 10.18632/aging.103713
57. Mehaffey E, Majid DSA. Tumor necrosis factor-alpha, kidney function, and hypertension. Am J Physiol Renal Physiol. (2017) 313:F1005–F8. doi: 10.1152/ajprenal.00535.2016
58. Unsal V, Cicek M, Sabancilar I. Toxicity of carbon tetrachloride, free radicals and role of antioxidants. Rev Environ Health. (2021) 36:279–95. doi: 10.1515/reveh-2020-0048
59. Sahreen S, Khan MR, Khan RA, Alkreathy HM. Protective effects of carissa opaca fruits against ccl4-induced oxidative kidney lipid peroxidation and trauma in rat. Food Nutr Res. (2015) 59:28438. doi: 10.3402/fnr.v59.28438
60. Pedigo CE, Ducasa GM, Leclercq F, Sloan A, Mitrofanova A, Hashmi T, et al. Local Tnf causes Nfatc1-dependent cholesterol-mediated podocyte injury. J Clin Invest. (2016) 126:3336–50. doi: 10.1172/JCI85939
61. Araujo LS, Torquato BGS, da Silva CA, Dos Reis Monteiro MLG, Dos Santos Martins ALM, da Silva MV, et al. Renal expression of cytokines and chemokines in diabetic nephropathy. BMC Nephrol. (2020) 21:308. doi: 10.1186/s12882-020-01960-0
62. Wen Y, Lu X, Ren J, Privratsky JR, Yang B, Rudemiller NP, et al. Klf4 in macrophages attenuates tnfalpha-mediated kidney injury and fibrosis. J Am Soc Nephrol. (2019) 30:1925–38. doi: 10.1681/ASN.2019020111
63. Wu PH, Liu PY, Chiu YW, Hung WC, Lin YT, Lin TY, et al. Comparative gut microbiome differences between ferric citrate and calcium carbonate phosphate binders in patients with end-stage kidney disease. Microorganisms. (2020) 8:2040. doi: 10.3390/microorganisms8122040
64. Jiang S, Xie S, Lv D, Wang P, He H, Zhang T, et al. Alteration of the gut microbiota in chinese population with chronic kidney disease. Sci Rep. (2017) 7:2870. doi: 10.1038/s41598-017-02989-2
65. Singh H, Miyamoto S, Darshi M, Torralba MG, Kwon K, Sharma K, et al. Gut microbial changes in diabetic db/db mice and recovery of microbial diversity upon pirfenidone treatment. Microorganisms. (2020) 8:1347. doi: 10.3390/microorganisms8091347
66. Chen C, You LJ, Huang Q, Fu X, Zhang B, Liu RH, et al. Modulation of gut microbiota by mulberry fruit polysaccharide treatment of obese diabetic Db/Db mice. Food Funct. (2018) 9:3732–42. doi: 10.1039/c7fo01346a
67. Brandsma E, Kloosterhuis NJ, Koster M, Dekker DC, Gijbels MJJ, van der Velden S, et al. A proinflammatory gut microbiota increases systemic inflammation and accelerates atherosclerosis. Circ Res. (2019) 124:94–100. doi: 10.1161/CIRCRESAHA.118.313234
68. Zhang J, Song L, Wang Y, Liu C, Zhang L, Zhu S, et al. Beneficial effect of butyrate-producing lachnospiraceae on stress-induced visceral hypersensitivity in rats. J Gastroenterol Hepatol. (2019) 34:1368–76. doi: 10.1111/jgh.14536
69. Jiang W, Wu N, Wang X, Chi Y, Zhang Y, Qiu X, et al. Dysbiosis gut microbiota associated with inflammation and impaired mucosal immune function in intestine of humans with non-alcoholic fatty liver disease. Sci Rep. (2015) 5:8096. doi: 10.1038/srep08096
70. Huang W, Man Y, Gao C, Zhou L, Gu J, Xu H, et al. Short-chain fatty acids ameliorate diabetic nephropathy Via Gpr43-mediated inhibition of oxidative stress and Nf-Kappab signaling. Oxid Med Cell Longev. (2020) 2020:4074832. doi: 10.1155/2020/4074832
71. Rizzatti G, Lopetuso LR, Gibiino G, Binda C, Gasbarrini A. Proteobacteria: a common factor in human diseases. Biomed Res Int. (2017) 2017:9351507. doi: 10.1155/2017/9351507
Keywords: foxtail millet, fermentation, germination, diabetic kidney disease, gut microbiota
Citation: Liu X, Qiu B, Liu W, Zhang Y, Wang X, Li X, Li L and Zhang D (2022) The Preventive Effects of Fermented and Germinated Foxtail Millet Whole Grain on Kidney Damage in a Diabetic Mouse Model. Front. Nutr. 9:940404. doi: 10.3389/fnut.2022.940404
Received: 10 May 2022; Accepted: 26 May 2022;
Published: 16 June 2022.
Edited by:
Sen Ma, Henan University of Technology, ChinaReviewed by:
Yanhui Han, University of Massachusetts Amherst, United StatesCopyright © 2022 Liu, Qiu, Liu, Zhang, Wang, Li, Li and Zhang. This is an open-access article distributed under the terms of the Creative Commons Attribution License (CC BY). The use, distribution or reproduction in other forums is permitted, provided the original author(s) and the copyright owner(s) are credited and that the original publication in this journal is cited, in accordance with accepted academic practice. No use, distribution or reproduction is permitted which does not comply with these terms.
*Correspondence: Lingfei Li, bGlsZkB5bmF1LmVkdS5jbg==; Di Zhang, ZGl6aGFuZ0BzZHUuZWR1LmNu
Disclaimer: All claims expressed in this article are solely those of the authors and do not necessarily represent those of their affiliated organizations, or those of the publisher, the editors and the reviewers. Any product that may be evaluated in this article or claim that may be made by its manufacturer is not guaranteed or endorsed by the publisher.
Research integrity at Frontiers
Learn more about the work of our research integrity team to safeguard the quality of each article we publish.