- 1Hunan Key Laboratory of Joint Degeneration and Injury, Changsha, China
- 2Department of Orthopedics, Xiangya Hospital, Central South University, Changsha, China
Objectives: Previous observational studies have suggested associations between concentrations of several circulating micronutrients and sarcopenia. However, the causality inferred from those studies was subjected to residual confounding and reverse causation. Therefore, we aimed to examine the causal effects of the levels of genetically predicted serum micronutrients on sarcopenia.
Methods: Single nucleotide polymorphisms (SNPs) were chosen from large-scale genome-wide association studies of participants only with European descent and were used as genetic instruments for the levels of 10 serum micronutrients (calcium, magnesium, selenium, copper, iron, zinc, Vitamin A, Vitamin B12, Vitamin D, and Vitamin E). Sarcopenia was defined by referencing to the 2019 definition given by the European Working Group on Sarcopenia in Older People (EWGSOP). A two-sample Mendelian randomization (MR) analysis was carried out to examine the associations between the levels of genetically predicted serum micronutrients and the risk of sarcopenia. Then, sensitivity analyses (including weighted median, MR-Egger and leave-one-out sensitivity analyses) were performed to evaluate the robustness of study findings. The estimates were presented as odds ratio (OR) with their 95% confidence intervals (CIs) per one standard deviation (SD) increase in the exposures.
Results: A total of 378,635 UK Biobank participants, including 572 participants who were identified with sarcopenia, were included in this study. The iron status was shown to have a clear effect on the risk of sarcopenia based on MR analyses. The per one SD increment in the genetically-determined serum iron level corresponded to a 53% increase in the risk of sarcopenia (OR = 1.53, 95% CI: 1.31–1.78, P = 0.001). The exclusion of SNPs of the circulating iron level (i.e., rs1799945 SNP, rs1800562 SNP or rs855791 SNP) did not attenuate the magnitude of the signal in MR analysis. There was little evidence supporting the associations between other remaining micronutrients and sarcopenia.
Conclusions: An increased risk of sarcopenia was observed with a genetically higher concentration of iron, suggesting that iron may play a role in the occurrence or development of sarcopenia.
Introduction
Sarcopenia is a progressive and generalized skeletal muscle disorder accompanied by accelerated loss of muscle mass and degradation of function, which is found to have high morbidity and mortality (1, 2). The prevalence of sarcopenia in the elderly population (age >60 years) was reported to be around 5–10% (3, 4). With the aging of the world's population, sarcopenia is likely to impose an increasing burden on public health resources in the future (5). However, the risk factors of sarcopenia remain insufficiently delineated, especially for those nutritional factors drawing considerable attention as modifiable risk factors and potentially therapeutic targets (6).
Micronutrients are critical for physiological functions of human being, and recent studies have found potential associations between micronutrients and muscle performance in elderly individuals (7, 8). The associations between disturbances of several serum micronutrients (calcium, magnesium, selenium, copper, iron, zinc, Vitamin A, Vitamin B12, Vitamin D, and Vitamin E) and sarcopenia have been identified in previous observational studies, but the findings are controversial (6, 9–16). Besides, given the observational design of these studies, the causal relationships between the levels of aforementioned serum micronutrients and the risk of sarcopenia remained unknown to a large extent. The existence of a potential causal association between a risk factor of interest and a target disease can be assessed by the Mendelian randomization (MR) approach using genetic variants as instrumental variables (17–19). As the genotype is allocated randomly at conception, genetic variants are not influenced by potential confounding factors such as environmental exposures, and cannot be altered by the occurrence of a disease (20). Nevertheless, to our best knowledge, the effects of micronutrients in sarcopenia have not been clearly elucidated on a large scale with the use of MR.
In this study, a two-sample MR analysis was carried out to explore the potential causal associations between the levels of serum micronutrients (calcium, magnesium, selenium, copper, iron, zinc, Vitamin A, Vitamin B12, Vitamin D, and Vitamin E) and the risk of sarcopenia. Our findings will help identify the mechanisms how micronutrients act as risk factors for sarcopenia and provide potential implications in the prognosis and treatment of sarcopenia.
Methods
Population
The UK Biobank is a prospective cohort study containing data of about 500,000 participants aged from 40 to 69 years who are recruited from the UK National Health Services between 2006 and 2010. Its study protocol was available online and more details can be found from the literature (21). To minimize potential confounding caused by ancestry, our study was restricted to the participants of white European descent only, and accordingly, the UK Biobank dataset was quality-controlled and filtered by removing individuals with non-white European ancestry. Other exclusion criteria were sex mismatch, excess heterozygosity, data deficiency (i.e., with missing information on grip strength, standing height, and fat-free mass), and second-degree relatives (22, 23). This study has been conducted using the UK Biobank Resource under Application Number 77,646.
Defining Genetic Instruments
Single nucleotide polymorphisms (SNPs) were derived from the recently-conducted large-scale, European ancestry genome-wide association studies (GWAS) and were used as variables of genetic instruments for serum micronutrients (calcium, magnesium, selenium, copper, iron, zinc, Vitamin A, Vitamin B12, Vitamin D and Vitamin E). All SNPs associated with a corresponding exposure at the genome-wide significance level (P <5 ×10−8) were extracted. From all genetic instruments, three independent ones were selected for systemic iron status, which could explain ~3.8% of the variation in blood iron (24). Six calcium- (25) or magnesium-SNPs (26) were identified by published GWAS studies, which could explain 0.37 and 1.62% of the variance in blood calcium or magnesium levels, respectively. Two significant SNPs associated with the blood copper level were selected, accounting for 5% of the phenotypic variance of the copper level (27). Similarly, two SNPs were selected as instrumental variables for zinc, explaining 4.59% of the variance of its concentration (27). Two vitamin A- (28) or vitamin E-SNPs (29) were identified according to published GWAS studies, which could explain 2.3 and 1.7% of the variance in blood vitamin A or vitamin E levels, respectively. Eight independent SNPs were selected as instrumental variables for circulating vitamin B12 that were previously identified in European populations, explained 5.1% of circulating vitamin B12 variance (30). Six SNPs were selected as instrumental variables for vitamin D, explaining 7.5% of the variance of its concentration (31). The detailed information for genetic instrumental variables used in this study was presented in Table 1.
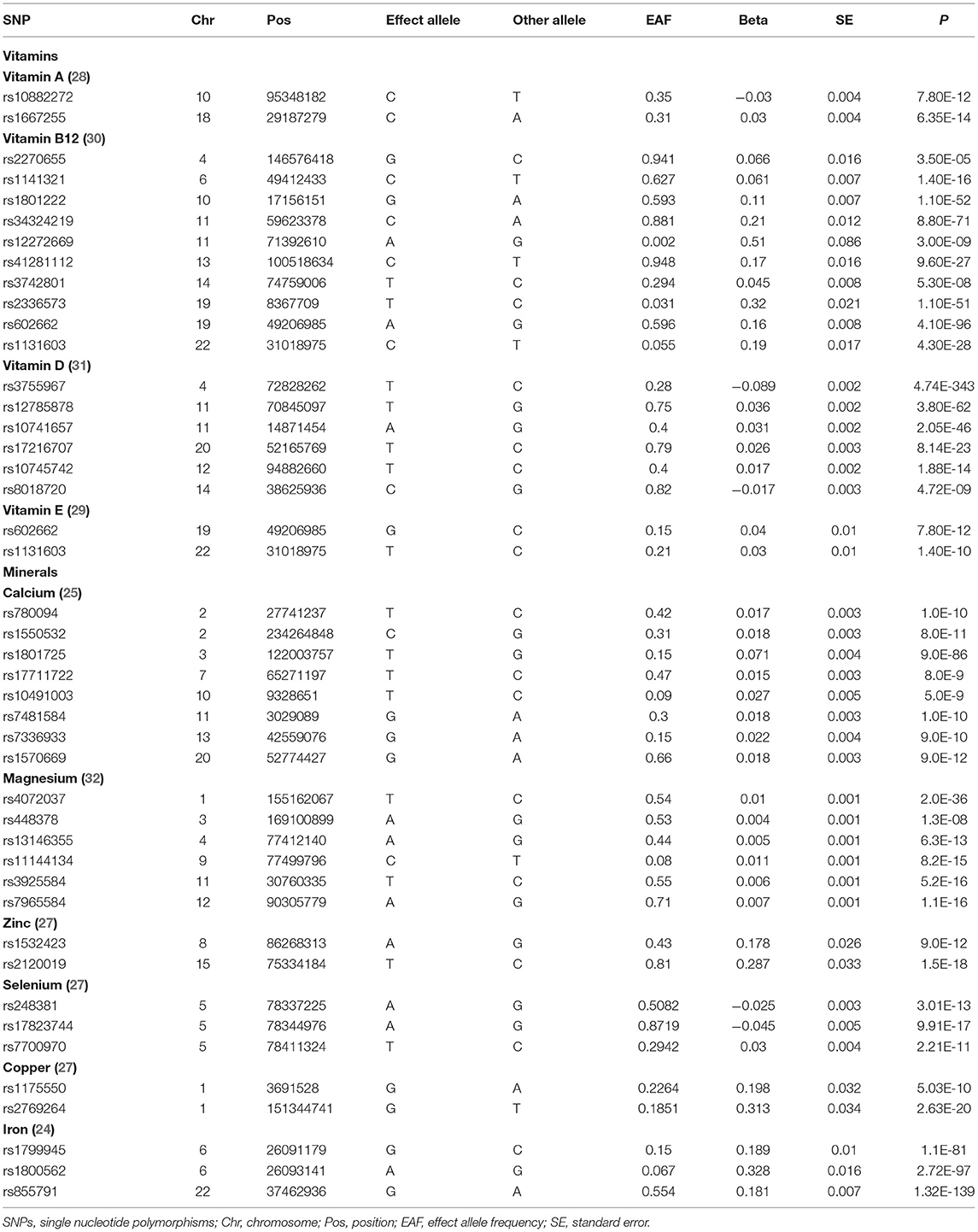
Table 1. Vitamins- and minerals-associated SNPs used as genetic instruments in the Mendelian randomization analyses.
Ascertainment of Sarcopenia
The individual-level phenotypic data on sarcopenia along with its related traits (i.e., grip strength and appendicular lean mass), the relevant confounding factors (i.e., age, sex and relatedness), and the genotypic data are all available in the UK Biobank website (http://www.nealelab.is/uk-biobank). Sarcopenia was defined by referencing to the 2019 definition given by the European Working Group on Sarcopenia in Older People (EWGSOP) (33) using the phenotypic data from the UK Biobank. Participants were diagnosed with confirmed sarcopenia if they had both low grip strength and low muscle mass. Low grip strength was defined as a maximum handgrip strength <27 kg in male and <16 kg in female patients. Skeletal muscle mass index (SMI) was derived from appendicular lean mass (kg) divided by height (m) squared. Low muscle mass was defined as an SMI <7.0 kg/m2 in men and <5.5 kg/m2 in women. Participants who had non-white European ancestry (to minimize confounding by ancestry), or sex mismatch, or excess heterozygosity, or data deficiency, or second-degree relatives (22, 23) were excluded.
Statistical Analysis
Outcome Data Analysis
The relations between genetically determined levels of circulating micronutrients (calcium, magnesium, selenium, copper, iron, zinc, Vitamin A, Vitamin B12, Vitamin D and Vitamin E) and sarcopenia were examined by logistic regression with adjustments for age, sex, genotype measurement batch, and 20 genetic principal components. In order to explicitly account for the possible population stratification, the genetic principal components that were pre-calculated based on selected genome-wide genotype markers were included.
Two-Sample MR Analysis
The two-sample MR analysis was carried out to estimate the potential causal associations between the concentrations of serum micronutrients and the risk of sarcopenia. The principal analyses were conducted by the inverse-variance weighted (IVW) approach, which is to estimate the effect of each SNP on the outcome by computing the Wald ratio and to perform a meta-analysis for the combined causal effect using the inverse variance of SNPs as weights (34). In IVW analysis, the random-effects model, which provides the most precise and unbiased estimates, was employed. The random-effects model assumes that all SNPs are valid instrumental variables (35) or the horizontal pleiotropy is balanced (36).
Sensitivity MR Analyses for Pleiotropy
In addition to the two-sample MR analysis, other MR methods were also employed as sensitivity analysis to strengthen the causal inference, each relying on different assumptions to provide confidence in result robustness. In sensitivity analyses, the weighted median method was used first as a proof for IVW analysis, which calculates the combined causal effect of exposure on the outcome based on the weighted median value of SNP-specific estimates, as long as >50% of the selected SNPs are valid variants (37). Furthermore, the MR Egger analysis was carried out to identify both directional and horizontal pleiotropy. Since the MR analysis is based on the hypothesis that the associations between genetic variants and the outcome are due to the pathway through the exposure, any other pathway in the relationship may result in a bias for the estimates in which the pleiotropy occurs. The MR-Egger method is to test the pleiotropic effects by investigating whether the intercept of the association between exposure and outcome is different from zero (38). The leave-one-out sensitivity analysis was carried out by eliminating a single variant from the analysis one by one, and the fluctuation of estimates in response to the elimination of each variant implies the possibility of outlier variant in the causal estimation. It is noteworthy that five exposures (vitamin A, vitamin E, zinc, selenium and copper) with <3 SNPs in their instrumental variables were excluded from the sensitivity analysis.
Reported Results and Software
The odds ratio (OR) with 95% confidence interval (CI) per standard deviation (SD) increase in the exposures is presented as the outcome. P-value <0.05 was considered statistically significant. All statistical tests were two-tailed. The two-sample MR analyses were carried out using the Mendelian Randomization package in R (version 3.6.3) (39). The associations between genetic variants and sarcopenia were established via the PLINK (version 2.0) software.
Results
Participants
Among 487,413 UK Biobank participants, 11,379 participants (2.3%) failed to provide information on grip strength, standing height or fat-free mass. After exclusion criteria were applied, a total of 378,635 individuals of European descent were included (mean age: 56.89 years; male: 46.2%). Of them 577 (0.2%) had a diagnosis of sarcopenia. The flowchart of participants selection is shown in Supplementary Figure 1. The characteristics of the included individuals are presented in Table 2.
Causal Effects of the Levels of Serum Micronutrients on Sarcopenia
Among the micronutrients examined, a genetically predicted higher iron level was found to be positively associated with sarcopenia in IVW analysis (Figure 1; Supplementary Table 1). The ORs of sarcopenia was 1.53 (95% CI, 1.31–1.78; P = 0.001) per SD increase in the serum iron level. The serum calcium level showed a potential inverse relationship with sarcopenia (OR = 0.21 per one SD increase of serum calcium level, 95% CI: 0.04–1.14, P = 0.071), although not reaching the significance level yet. The genetic predisposition to high circulating levels of other remaining serum micronutrients (magnesium, selenium, copper, zinc, Vitamin A, Vitamin B12, Vitamin D, and Vitamin E) showed no significant associations with sarcopenia (Figure 1).
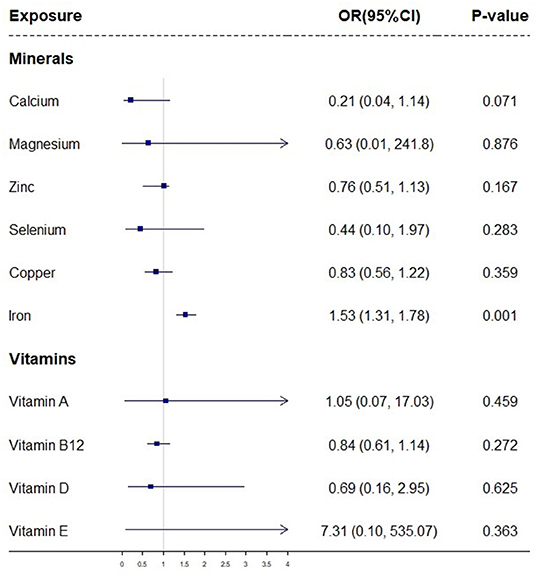
Figure 1. A forest plot showing associations between genetically determined levels of micronutrients and sarcopenia based on IVW MR analysis. The odds ratios (ORs) with their 95% confidence intervals (CIs) are scaled to 1-standard deviation (SD) increase in blood micronutrient level.
Sensitivity Analysis
As shown in Supplementary Table 1, the MR Egger analysis reached similar results on the causal associations between serum iron levels and sarcopenia (OR = 1.57; 95% CI, 1.01–2.44; P = 0.046). The weighted median (OR = 2.21) estimates on the association between serum iron and sarcopenia were consistent with IVW and MR Egger analyses, albeit not statistically significant.
The leave-one-out analysis was carried out by eliminating a single genetic variant at a time, in order to identify the SNP that is primarily affected by pleiotropy. The results of IVW in the leave-one-out analysis showed that the P-values of intercepts in the associations between serum iron levels and sarcopenia were markedly significant when rs1799945, rs1800562 and rS855791 were removed separately (Supplementary Figure 2).
Discussion
Our MR analysis on the ten serum micronutrients suggested a positive association between the genetically elevated concentration of circulating iron and the risk of sarcopenia, which was robust under different sensitivity analyses.
Comparison With Previous Studies
The literature on the association between the circulating iron level and sarcopenia is scarce and inconclusive. A longitudinal study covering 698 elderly participants from the InCHIANTI study rejected any association between the baseline serum iron level and degradation of physical function based on a follow-up of 3 months (40). On the contrary, recent clinical studies indicated a significant relationship between iron accumulation and grip strength and sarcopenia. A study in 2014 covering 1,380 middle-aged and elderly Korean female participants reported that sarcopenia was significantly associated with an increased serum ferritin level, and the risk of individuals with elevated serum ferritin (serum ferritin is a commonly-used clinical indicator for evaluating the iron load of human being) was 2.02-fold of those with a normal serum ferritin level (41). In 2016, a study covering 300 individuals who received hemodialysis found that the forearm grip strength had a significantly negative association with the serum ferritin level (42). In 2017, a study including 639 Italian elderly participants (>65 years) reported that sarcopenia patients had a significantly increased serum ferritin level (43). The inconsistency among different studies may be explained by the variations in study design and outcome measures. In our study, MR analysis was employed to avoid biases that often occur in observational studies and the utilization of data from large-scale genetic consortia. However, our results did not imply any causal effect of other remaining serum micronutrients on the risk of sarcopenia.
Possible Explanations
Several explanations may account for the association between the genetically predicted serum iron level and the risk of sarcopenia. The skeletal muscle atrophy or injury caused by iron accumulation is likely to be correlated with mitochondrial function, oxidative damage, and the ubiquitin proteasome pathway (44–47). The mitochondria in skeletal muscle cells can not only supply energy for muscle contraction but also regulate catabolic pathways and balance the intracellular oxidative reaction. Under physiological conditions, the non-heme iron that supports the synthesis of mitochondrial coenzymes is mainly provided by mitochondria. However, iron accumulation increases with age, and mitochondria as the main organelles for iron uptake are more likely to develop iron accumulation (48). It is believed that iron excess can generate massive reactive oxygen species (ROS) by Fenton reaction, which can further lead to RNA and DNA damage, and changes of protein conformation and lipid peroxidation. As a consequence, it will result in cell damage and even apoptosis. As suggested by several earlier studies, oxygen-free radicals produced by iron accumulation can cause mitochondrial RNA peroxidation and further induce the opening of mitochondrial permeability transition pores (mPTP), which may lead to the release of cytochrome C into the cytoplasm, the activation of caspase-3, and eventually the apoptosis of skeletal muscle cells (49–52). In 2016, Ikeda et al. reported a decrease of skeletal muscle mass in mouse model resulted from iron accumulation, which could be attributed to the molecular mechanism of the induction of oxidative stress and inhibition of the Akt-FOXO3a pathway (i.e., up-regulation of atrogin-1 and MuRF1). Silencing of FOXO3a expression in C2C12 myotube cells or application of ROS scavenger can inhibit the iron-induced expression of 200 atrogin-1 and MuRF1, as well as prevent cell atrophy (53). It has been demonstrated that the consumption of iron-enriched food and the impaired tissue iron metabolism can both promote high iron stores (54). To sum up, the effect of iron accumulation on sarcopenia is multifactorial, and in our study, the effect of an elevated serum iron level in increasing the risk of sarcopenia was observed.
Strengths and Limitations
Firstly, the primary strength of this study is attributed to the application of the MR method. From our two-sample MR analysis, we derived data from multiple large-scale GWAS datasets and thus improved the precision of SNPs selection and the power of statistical analysis. In comparison with conventional observational studies, the MR method is more resistant to biases caused by confounding factors and reverse causation. Secondly, we examined the linkage disequilibrium of the included genetic variants. Thirdly, the weighted median analysis was carried out as proof for the observed causalities in IVW analysis and the MR-Egger and leave-one-out approaches were employed to control the bias caused by pleiotropic effects in sensitivity analyses. Finally, the bias of population stratification was minimized in our study as our sample was restricted to participants of European descent in the UK Biobank.
Nevertheless, limitations of this study should also be highlighted. First of all, despite the use of multiple MR approaches to prevent confounding from pleiotropy, the residual bias cannot be fully eliminated, as it is an established limitation of the MR method (55). For example, the micronutrients-related SNPs affect sarcopenia-related outcomes through other causal pathways than through micronutrients exposure cannot be entirely ruled out. Secondly, the association between the serum iron level and sarcopenia observed in IVW analysis was not statistically significant in weighted median analysis. This might be attributed to the assumption of IVW that all the SNPs satisfy the genetic instrumental assumptions in MR analysis, while the weighted median method adopts the hypothesis that over half of the SNPs are valid. Finally, due to the low initial response rate and a degree of healthy responder bias in the UK biobank study, the prevalence of sarcopenia was relatively low in our analysis (56), so was the proportion of variance in the micronutrients explained by genetic variants (ranging from 8% for zinc to 0.37% for calcium). Although no causal association between the levels of nine serum micronutrients (calcium, magnesium, selenium, copper, zinc, Vitamin A, Vitamin B12, Vitamin D, and Vitamin E) and sarcopenia was observed, we could not completely rule out the possibility that our study may not have adequate power to detect a weak association.
Clinical and Research Implications
Our findings implied a positive association between the genetically predicted iron level and the risk of sarcopenia, which supports a logical speculation that down-regulating serum iron may be beneficial in reducing the risk of sarcopenia. The decrease in iron overload by caloric restriction points out an important direction for sarcopenia intervention (47, 57). However, a recent animal study found that the oral administration of an iron chelator (deferiprone) failed to modulate iron in muscles of old mice model, which indicated the complexity of restricting iron under the conditions of muscle aging and regeneration. Therefore, more comprehensive researches are expected in the future to confirm our findings and elucidate the underlying biological mechanisms, so that appropriate monitoring, treatment and prevention strategies can be developed to effectively manage and control the burden of sarcopenia patients.
Conclusion
This is the first comprehensive two-sample MR study that investigated the potential associations between genetically predicted concentrations of ten micronutrients and the risk of sarcopenia. From our comprehensive analyses, an increased risk of sarcopenia was observed in relation to a genetically higher concentration of iron, which was robust under different sensitivity analyses. However, further research is warranted to confirm our findings and elucidate the underlying mechanisms.
Data Availability Statement
The original contributions presented in the study are included in the article/Supplementary Materials, further inquiries can be directed to the corresponding author/s. All relevant GWAS summary statistic data are included in Table 1. The data underlying the results for the UK Biobank presented in the study are available from the UK Biobank: https://www.ukbiobank.ac.uk/, for researchers who meet the criteria for access to the data.
Ethics Statement
The studies involving human participants were reviewed and approved by North West Center for Research Ethics Committee (11/NW/0382). The patients/participants provided their written informed consent to participate in this study.
Author Contributions
HL: concept and design. TS, WL, HH, JW, YW, and HL: acquisition, analysis, or interpretation of data, and critical revision of the manuscript for important intellectual content. WL, TS, and HL: drafting of the manuscript. TS: statistical analysis. All authors have revised the article critically for important intellectual content and approved the final version to be published.
Funding
This work was supported by the National Natural Science Foundation of China (81930071, 81902265, 82072502, and U21A20352), the Key Research and Development Program of Hunan Province (2021SK2017), and the Youth Science Foundation of Xiangya Hospital (2021Q14). The funding source had no role in the design and conduct of the study; collection, management, analysis, and interpretation of the data; praparation, review, or approval of the manuscript; and the decision to submit the manuscript for publication.
Author Disclaimer
The interpretation of these data is the sole responsibility of the authors.
Conflict of Interest
The authors declare that the research was conducted in the absence of any commercial or financial relationships that could be construed as a potential conflict of interest.
Publisher's Note
All claims expressed in this article are solely those of the authors and do not necessarily represent those of their affiliated organizations, or those of the publisher, the editors and the reviewers. Any product that may be evaluated in this article, or claim that may be made by its manufacturer, is not guaranteed or endorsed by the publisher.
Supplementary Material
The Supplementary Material for this article can be found online at: https://www.frontiersin.org/articles/10.3389/fnut.2022.913155/full#supplementary-material
References
1. Cruz-Jentoft AJ, Bahat G, Bauer J, Boirie Y, Bruyere O, Cederholm T, et al. Sarcopenia: revised European consensus on definition and diagnosis. Age Ageing. (2019) 48:16–31. doi: 10.1093/ageing/afy169
2. Beaudart C, Zaaria M, Pasleau F, Reginster JY, Bruyere O. Health outcomes of sarcopenia: a systematic review and meta-analysis. PLoS ONE. (2017) 12:e0169548. doi: 10.1371/journal.pone.0169548
3. Morley JE, Anker SD, von Haehling. S. Prevalence, incidence, and clinical impact of sarcopenia: facts, numbers, epidemiology-update 2014. J Cachexia Sarcopenia Muscle. (2014) 5:253–9. doi: 10.1007/s13539-014-0161-y
4. Shafiee G, Keshtkar A, Soltani A, Ahadi Z, Larijani B, Heshmat R. Prevalence of sarcopenia in the world: a systematic review and meta- analysis of general population studies. J Diabetes Metab Disord. (2017) 16:21. doi: 10.1186/s40200-017-0302-x
5. World Health Organization. Ageing and Health. (2018). Available online at. https://www.who.int/news-room/fact-sheets/detail/ageing-and-health (accessed July 23, 2021).
6. Cruz-Jentoft AJ, Sayer AA. Sarcopenia. Lancet. (2019) 393:2636–46. doi: 10.1016/S0140-6736(19)31138-9
7. Ganapathy A, Nieves JW. Nutrition and sarcopenia-what do we know? Nutrients. (2020) 12:1755. doi: 10.3390/nu12061755
8. Shenkin A. The key role of micronutrients. Clin Nutr. (2006) 25:1–13. doi: 10.1016/j.clnu.2005.11.006
9. Can B, Kara O, Kizilarslanoglu MC, Arik G, Aycicek GS, Sumer F, et al. Serum markers of inflammation and oxidative stress in sarcopenia. Aging Clin Exp Res. (2017) 29:745–52. doi: 10.1007/s40520-016-0626-2
10. Hayhoe RPG, Lentjes MAH, Mulligan AA, Luben RN, Khaw KT, Welch AA. Cross-sectional associations of dietary and circulating magnesium with skeletal muscle mass in the EPIC-Norfolk cohort. Clin Nutr. (2019) 38:317–23. doi: 10.1016/j.clnu.2018.01.014
11. Gariballa S, Alessa A. Association between nutritional blood-based biomarkers and clinical outcome in sarcopenia patients. Clin Nutr ESPEN. (2018) 25:145–8. doi: 10.1016/j.clnesp.2018.03.002
12. Chen YL, Yang KC, Chang HH, Lee LT, Lu CW, Huang KC. Low serum selenium level is associated with low muscle mass in the community-dwelling elderly. J Am Med Dir Assoc. (2014) 15:807–11. doi: 10.1016/j.jamda.2014.06.014
13. Zhao G. Is iron accumulation a possible risk factor for sarcopenia? Biol Trace Elem Res. (2018) 186:379–83. doi: 10.1007/s12011-018-1332-z
14. Park S, Ham JO, Lee BK. A positive association of vitamin D deficiency and sarcopenia in 50 year old women, but not men. Clin Nutr. (2014) 33:900–5. doi: 10.1016/j.clnu.2013.09.016
15. Verlaan S, Aspray TJ, Bauer JM, Cederholm T, Hemsworth J, Hill TR, et al. Nutritional status, body composition, and quality of life in community-dwelling sarcopenic and non-sarcopenic older adults: a case-control study. Clin Nutr. (2017) 36:267–74. doi: 10.1016/j.clnu.2015.11.013
16. Kim Y, Shin S, Hong N, Rhee Y. Low serum vitamin e level associated with low hand grip strength in community-dwelling adults: korean national health and nutrition examination survey (KNHANES VII) 2016–2018. Nutrients. (2021) 13:1598. doi: 10.3390/nu13051598
17. Sekula P, Del Greco MF, Pattaro C, Kottgen A. Mendelian randomization as an approach to assess causality using observational data. J Am Soc Nephrol. (2016) 27:3253–65. doi: 10.1681/ASN.2016010098
18. Smith GD, Ebrahim S. Mendelian randomization: can genetic epidemiology contribute to understanding environmental determinants of disease? Int J Epidemiol. (2003) 32:1–22. doi: 10.1093/ije/dyg070
19. Lawlor DA, Harbord RM, Sterne JA, Timpson N, Davey Smith. G. Mendelian randomization: using genes as instruments for making causal inferences in epidemiology. Stat Med. (2008) 27:1133–63. doi: 10.1002/sim.3034
20. Smith GD, Ebrahim S. Mendelian randomization: prospects, potentials, and limitations. Int J Epidemiol. (2004) 33:30–42. doi: 10.1093/ije/dyh132
21. Sudlow C, Gallacher J, Allen N, Beral V, Burton P, Danesh J, et al. UK biobank: an open access resource for identifying the causes of a wide range of complex diseases of middle and old age. PLoS Med. (2015) 12:e1001779. doi: 10.1371/journal.pmed.1001779
22. Hindy G, Akesson KE, Melander O, Aragam KG, Haas ME, Nilsson PM, et al. Cardiometabolic polygenic risk scores and osteoarthritis outcomes: a mendelian randomization study using data from the malmo diet and cancer study and the UK Biobank. Arthr Rheumatol. (2019) 71:925–34. doi: 10.1002/art.40812
23. Bycroft C, Freeman C, Petkova D, Band G, Elliott LT, Sharp K, et al. Genome-wide genetic data on 500,000 UK Biobank participants. BioRxiv. (2017) 166298. doi: 10.1101/166298
24. Gill D, Del Greco MF, Walker AP, Srai SKS, Laffan MA, Minelli C. The effect of iron status on risk of coronary artery disease: a mendelian randomization study-brief report. Arterioscler Thromb Vasc Biol. (2017) 37:1788–92. doi: 10.1161/ATVBAHA.117.309757
25. O'Seaghdha CM, Wu H, Yang Q, Kapur K, Guessous I, Zuber AM, et al. Meta-analysis of genome-wide association studies identifies six new loci for serum calcium concentrations. PLoS Genet. (2013) 9:e1003796. doi: 10.1371/journal.pgen.1003796
26. Meyer TE, Verwoert GC, Hwang SJ, Glazer NL, Smith AV, van Rooij FJ, et al. Genome-wide association studies of serum magnesium, potassium, and sodium concentrations identify six loci influencing serum magnesium levels. PLoS Genet. (2010) 6:e1001045. doi: 10.1371/journal.pgen.1001045
27. Evans DM, Zhu G, Dy V, Heath AC, Madden PA, Kemp JP, et al. Genome-wide association study identifies loci affecting blood copper, selenium and zinc. Hum Mol Genet. (2013) 22:3998–4006. doi: 10.1093/hmg/ddt239
28. Mondul AM, Yu K, Wheeler W, Zhang H, Weinstein SJ, Major JM, et al. Genome-wide association study of circulating retinol levels. Hum Mol Genet. (2011) 20:4724–31. doi: 10.1093/hmg/ddr387
29. Wang T, Xu L. Circulating vitamin e levels and risk of coronary artery disease and myocardial infarction: a mendelian randomization study. Nutrients. (2019) 11:2153. doi: 10.3390/nu11092153
30. Fanidi A, Carreras-Torres R, Larose TL, Yuan JM, Stevens VL, Weinstein SJ, et al. Is high vitamin B12 status a cause of lung cancer? Int J Cancer. (2019) 145:1499–503. doi: 10.1002/ijc.32033
31. Jiang X, O'Reilly PF, Aschard H, Hsu YH, Richards JB, Dupuis J, et al. Genome-wide association study in 79,366 European-ancestry individuals informs the genetic architecture of 25-hydroxyvitamin D levels. Nat Commun. (2018) 9:260. doi: 10.1038/s41467-017-02662-2
32. Larsson SC, Burgess S, Michaelsson K. Serum magnesium levels and risk of coronary artery disease: mendelian randomisation study. BMC Med. (2018) 16:68. doi: 10.1186/s12916-018-1065-z
33. Cruz-Jentoft AJ, Bahat G, Bauer J, Boirie Y, Bruyere O, Cederholm T, et al. Sarcopenia: revised European consensus on definition and diagnosis. Age Ageing. (2019) 48:601. doi: 10.1093/ageing/afz046
34. Lee CH, Cook S, Lee JS, Han B. Comparison of two meta-analysis methods: inverse-variance-weighted average and weighted sum of z-scores. Genomics Inform. (2016) 14:173–80. doi: 10.5808/GI.2016.14.4.173
35. Burgess S, Bowden J, Fall T, Ingelsson E, Thompson SG. Sensitivity analyses for robust causal inference from mendelian randomization analyses with multiple genetic variants. Epidemiology. (2017) 28:30–42. doi: 10.1097/EDE.0000000000000559
36. Hemani G, Bowden J, Davey Smith. G. Evaluating the potential role of pleiotropy in Mendelian randomization studies. Hum Mol Genet. (2018) 27:R195–208. doi: 10.1093/hmg/ddy163
37. Kodali HP, Pavilonis BT, Schooling CM. Effects of copper and zinc on ischemic heart disease and myocardial infarction: a Mendelian randomization study. Am J Clin Nutr. (2018) 108:237–42. doi: 10.1093/ajcn/nqy129
38. Burgess S, Thompson SG. Interpreting findings from Mendelian randomization using the MR-Egger method. Eur J Epidemiol. (2017) 32:377–89. doi: 10.1007/s10654-017-0255-x
39. Yavorska OO, Burgess S. MendelianRandomization: an R package for performing Mendelian randomization analyses using summarized data. Int J Epidemiol. (2017) 46:1734–9. doi: 10.1093/ije/dyx034
40. Bartali B, Frongillo EA, Guralnik JM, Stipanuk MH, Allore HG, Cherubini A, et al. Serum micronutrient concentrations and decline in physical function among older persons. JAMA. (2008) 299:308–15. doi: 10.1001/jama.299.3.308
41. Kim TH, Hwang HJ, Kim SH. Relationship between serum ferritin levels and sarcopenia in Korean females aged 60 years and older using the fourth Korea national health and nutrition examination survey (KNHANES IV-2, 3), 2008-2009. PLoS One. (2014) 9:e90105. doi: 10.1371/journal.pone.0090105
42. Nakagawa C, Inaba M, Ishimura E, Yamakawa T, Shoji S, Okuno S. Association of increased serum ferritin with impaired muscle strength/quality in hemodialysis patients. J Ren Nutr. (2016) 26:253–7. doi: 10.1053/j.jrn.2016.01.011
43. Perna S, Peroni G, Faliva MA, Bartolo A, Naso M, Miccono A, et al. Sarcopenia and sarcopenic obesity in comparison: prevalence, metabolic profile, key differences. A cross-sectional study in Italian hospitalized elderly. Aging Clin Exp Res. (2017) 29:1249–58. doi: 10.1007/s40520-016-0701-8
44. Altun M, Edstrom E, Spooner E, Flores-Moralez A, Bergman E, Tollet-Egnell P, et al. Iron load and redox stress in skeletal muscle of aged rats. Muscle Nerve. (2007) 36:223–33. doi: 10.1002/mus.20808
45. Arruda LF, Arruda SF, Campos NA, de Valencia FF, Siqueira EM. Dietary iron concentration may influence aging process by altering oxidative stress in tissues of adult rats. PLoS ONE. (2013) 8:e61058. doi: 10.1371/journal.pone.0061058
46. Hofer T, Marzetti E, Xu J, Seo AY, Gulec S, Knutson MD, et al. Increased iron content and RNA oxidative damage in skeletal muscle with aging and disuse atrophy. Exp Gerontol. (2008) 43:563–570. doi: 10.1016/j.exger.2008.02.007
47. Xu J, Knutson MD, Carter CS, Leeuwenburgh C. Iron accumulation with age, oxidative stress and functional decline. PLoS ONE. (2008) 3:e2865. doi: 10.1371/journal.pone.0002865
48. Seo AY, Xu J, Servais S, Hofer T, Marzetti E, Wohlgemuth SE, et al. Mitochondrial iron accumulation with age and functional consequences. Aging Cell. (2008) 7:706–16. doi: 10.1111/j.1474-9726.2008.00418.x
49. Duvigneau JC, Piskernik C, Haindl S, Kloesch B, Hartl RT, Huttemann M, et al. A novel endotoxin-induced pathway: upregulation of heme oxygenase 1, accumulation of free iron, and free iron-mediated mitochondrial dysfunction. Lab Invest. (2008) 88:70–7. doi: 10.1038/labinvest.3700691
50. Liang LP, Jarrett SG, Patel M. Chelation of mitochondrial iron prevents seizure-induced mitochondrial dysfunction and neuronal injury. J Neurosci. (2008) 28:11550–6. doi: 10.1523/JNEUROSCI.3016-08.2008
51. Marzetti E, Calvani R, Cesari M, Buford TW, Lorenzi M, Behnke BJ, et al. Mitochondrial dysfunction and sarcopenia of aging: from signaling pathways to clinical trials. Int J Biochem Cell Biol. (2013) 45:2288–301. doi: 10.1016/j.biocel.2013.06.024
52. Veatch JR, McMurray MA, Nelson ZW, Gottschling DE. Mitochondrial dysfunction leads to nuclear genome instability via an iron-sulfur cluster defect. Cell. (2009) 137:1247–58. doi: 10.1016/j.cell.2009.04.014
53. Ikeda Y, Imao M, Satoh A, Watanabe H, Hamano H, Horinouchi Y, et al. Iron-induced skeletal muscle atrophy involves an Akt-forkhead box O3-E3 ubiquitin ligase-dependent pathway. J Trace Elem Med Biol. (2016) 35:66–76. doi: 10.1016/j.jtemb.2016.01.011
54. Tsuchiya H, Ebata Y, Sakabe T, Hama S, Kogure K, Shiota G. High-fat, high-fructose diet induces hepatic iron overload via a hepcidin-independent mechanism prior to the onset of liver steatosis and insulin resistance in mice. Metabolism. (2013) 62:62–9. doi: 10.1016/j.metabol.2012.06.008
55. Bennett DA, Holmes MV. Mendelian randomisation in cardiovascular research: an introduction for clinicians. Heart. (2017) 103:1400–7. doi: 10.1136/heartjnl-2016-310605
56. Fry A, Littlejohns TJ, Sudlow C, Doherty N, Adamska L, Sprosen T, et al. Comparison of sociodemographic and health-related characteristics of UK biobank participants with those of the general population. Am J Epidemiol. (2017) 186:1026–34. doi: 10.1093/aje/kwx246
Keywords: sarcopenia, Mendelian randomization, micronutrients, minerals, vitamins
Citation: Sha T, Li W, He H, Wu J, Wang Y and Li H (2022) Causal Relationship of Genetically Predicted Serum Micronutrients Levels With Sarcopenia: A Mendelian Randomization Study. Front. Nutr. 9:913155. doi: 10.3389/fnut.2022.913155
Received: 05 April 2022; Accepted: 31 May 2022;
Published: 22 June 2022.
Edited by:
Jie Zhao, The University of Hong Kong, Hong Kong SAR, ChinaReviewed by:
Kai Gao, Chinese Institute for Brain Research, ChinaXiaolu Nie, Capital Medical University, China
Copyright © 2022 Sha, Li, He, Wu, Wang and Li. This is an open-access article distributed under the terms of the Creative Commons Attribution License (CC BY). The use, distribution or reproduction in other forums is permitted, provided the original author(s) and the copyright owner(s) are credited and that the original publication in this journal is cited, in accordance with accepted academic practice. No use, distribution or reproduction is permitted which does not comply with these terms.
*Correspondence: Hui Li, lihui1988@csu.edu.cn
†These authors have contributed equally to this work and share first authorship