- 1Precision Nutrition, Research Department, Sidra Medicine, Doha, Qatar
- 2Bariatric and Metabolic Surgery Department, Hamad Medical Corporation, Doha, Qatar
Background: Obesity is a complex disease with underlying genetic, environmental, psychological, physiological, medical, and epigenetic factors. Obesity can cause various disorders, including cardiovascular diseases (CVDs), that are among the most prevalent chronic conditions in Qatar. Recent studies have highlighted the significant roles of the gut microbiome in improving the pathology of various diseases, including obesity. Thus, in this study, we aimed to investigate the effects of dietary intake and gut microbial composition in modulating the risk of CVD development in obese Qatari adults.
Methods: We enrolled 46 adult subjects (18–65 years of age) who were classified based on their CVD risk scores, calculated using the Framingham formula, into a CVD no-risk group (score of <10%, n = 36) and CVD risk group (score of ≥10%, n = 10). For each study subject, we measured the gut microbial composition with a 16s rDNA sequencing method that targeted the v3-v4 region using Illumina Miseq, and their nutritional status was recorded based on 24-h dietary recall. Dietary intake, bacterial taxa summary, diversity index, microbial markers, pathway analysis, and network correlation were determined for the study subjects.
Results: The CVD risk group showed a lower intake of vitamin D, reduced relative abundance of genera Ruminococcus and Bifidobacterium, no change in bacterial diversity, and higher levels of taurine, hypotaurine, and lipoic acid metabolism than the CVD no-risk group. Besides, the relative abundance of genus Ruminococcus was positively correlated with the intake of protein, monounsaturated fat, vitamin A, and vitamin D.
Conclusion: Taken together, our results suggest that the genus Ruminococcus could be used as a microbial marker, and its reduced relative abundance could mediate the risk of CVDs in the Obese Qatari population.
Introduction
According to a recent WHO report, the prevalence of obesity has reached pandemic proportions, and the obesity-related mortality rate is at least 2.8 million people each year worldwide. A recent cross-sectional study conducted on Qatari adults suggested that the prevalence rate for obesity is 33% (1), and the rate is higher in women compared to men. Obesity is a complex network of pathologies, as it is a multifactorial disorder that includes genetic, environmental, psychological, physiological, medical, and epigenetic factors (2–5), and its underlying mechanisms mainly include (1) an imbalance in the ratio between the accumulation of excess fat and energy expenditure by the body and (2) resetting of the set bodyweight point to a higher value (2, 6). In addition to these factors, the gut microbiota has been shown to play an unprecedented role in the pathogenesis of obesity; crosstalk between the gut microbiota and obesity is like a double-edged sword because both entities are regulated by each other (7–9).
Obesity is also closely associated with the pathogenesis of cardiovascular diseases (CVDs), including cardiac arrhythmias, congenital heart disease, coronary heart disease, heart failure, cardiomyopathy, and pulmonary embolism (10, 11). A prominent pathway linked to obesity-associated CVDs is the activation of inflammatory mediated pathways, for example, the secretion of lipopolysaccharide synthesis, which mainly acts through toll-like receptors, causes the aggravation of atherosclerotic plaque formation (12). Furthermore, a handful of evidence from clinical and pre-clinical studies has shown that the gut microbiota also plays a crucial role in obesity-associated CVDs, primarily through the secretion of gut microbiota-derived metabolites such as L-carnitine, betaine, choline, TMAO, and short-chain fatty acids (SCFAs) (13–15), and the fat content of the diet influences how the gut microbiota effects CVDs (8, 16, 17). Marzullo et al. (18) demonstrated that obesity is associated with CVDs through its modulation of the gut microbiota at both lower taxonomic and higher taxonomy levels of change. Gut microbial diversity is an important indicator of the health status of the gut: the higher the diversity, the healthier the gut. Pre-clinical and clinical studies have demonstrated that microbial diversity decreases with the severity of obesity (8, 17, 19, 20). On the other hand, Lin et al. (21) studied the microbial diversity in Chinese subjects and found no relationship between microbial diversity and body mass index (BMI). Obesity has been demonstrated to increase CVD risk, mainly through gut microbial dysbiosis, which can cause a leaky gut and lead to CVDs through a variety of mechanisms, such as secretion of SCFAs and TMAO, potentiation of systemic inflammation, and regulation of G-protein receptors (GPRs) (18). In addition, Fu et al. demonstrated that the gut microbiota contributes to variations in lipid profiles, especially triglycerides and HDLs, independently of BMI, and these changes are small but contribute significantly to outcomes (22).
Numerous studies have demonstrated that diet is a predominant factor in the modulation of gut microbiota (23, 24), and it can even abruptly change the gut microbiota on a daily timescale in humans (25), suggesting its potential role in maintaining the health of the gut microbiota. Multiple studies have demonstrated that the diet is one of the most prominent epigenetic factors affecting the gut microbial composition in terms of both richness and abundance (23, 24). It is believed that acute dietary adjustments can change the microbial composition substantially, but because of the stability of the enterotype, they do not cause a switch from one enterotype to another; whereas chronic dietary adjustments can lead to the formation of a different enterotype (26).
Thus, in this study, we aimed to characterize the gut microbial composition and search for links between dietary patterns, gut microbial dysbiosis, and the development of CVDs in Qatari obese adults. We also aimed to explore the molecular pathway mechanisms that may contribute to the development of obesity-associated CVDs and that involve the gut microbiota in this population.
Patients and Methods/Materials
Recruitment of Study Participants
The study participants were approached and recruited after having consented to participation at Hamad Medical Corporation, Doha, Qatar. This study was approved by the Institutional Review Boards of both Sidra Medicine (IRB, #1604002867) and Hamad Medical Corporation (IRB, #16419/16). We applied two inclusion criteria: (1) Qatari citizens with BMI > 30 kg/m2 and (2) male or female in the age range of 18–65 years. The exclusion criteria were those who had had (1) bariatric surgery, (2) chronic diseases other than obesity, and (3) antibiotics in the last 3 months. A total of 46 study participants were included. From each study participant, we collected stool and blood samples, and dietary data were collected by a trained dietician, who interviewed the patients on their 24-h dietary recall. Anthropometric measurements, such as body weight, height, and waist circumference, as well as blood pressure (BP) and heart rate (HR) were measured.
Nutrient Intake
The 24-h food recall data were collected by the dietician and computed using Nutritionist Pro software (Axxya Systems LLC) to obtain the micro- and macro-nutrient intake for each study participant. The obtained data were used to compare the CVD risk and CVD no-risk groups.
Biochemical Parameters
Blood samples were used to measure various biochemical parameters, such as fasting blood glucose (FBG), glycosylated hemoglobin (HbA1c), insulin, lipid profiles (HDL, LDL, and TG), alkaline phosphatase (ALP), alanine transaminase (ALT), aspartate transaminase (AST), thyroid stimulated hormone (TSH), triiodothyronine (T3), thyroxine (T4), vitamin D, vitamin B12, folate, uric acid, and iron saturation levels.
Calculation of CVD Risk Score
The CVD risk score was calculated using the Framingham risk score method (27), mainly considering age, gender, smoking habits, systolic blood pressure, total cholesterol and HDL levels, HbA1C, and treatment for hypertension.
16s rDNA Sequencing
Stool samples were collected in an OMNI-gene Gut tube, and microbial genomic DNA (gDNA) was extracted using QIAamp Fast DNA Stool Mini Kit (Qiagen, catalog number #51604). The extracted gDNA was amplified using primers that targeted the v3-v4 region of 16s rDNA, indexed using Nextera XT kit v2 (catalog number #FC-131-1002, Illumina), and sequenced according to the Illumina protocol described previously (28) using MiSeq Reagent Kit v3 for 600 cycles (catalog number #MS-102-3003, Illumina).
Gut Microbial Computational Analysis
Classification of Taxa
The raw fastq files were demultiplexed using MiSeq Control Software. To merge the forward and reverse sequences, we used a paired-End read mergeR v0.9.8 tool (29), and the merged reads were trimmed using the Trimmomatic v0.36 tool (30) to obtain reads with a quality score of >30. The Quantitative Insights Into Microbial Ecology (QIIME) v1.9.0 pipeline (31) was used to convert the FASTQ files into FASTA files, which were all combined into a single FASTA file. The Greengenes database (gg_13_08) was used to obtain the operational taxonomic units (OTUs) by aligning the sequences with a confidence threshold of 97% (32).
Gut Microbial Diversity Indices
Alpha diversity is a measure of microbial richness and relative abundance, and it was estimated by R packages (such as Phyloseq and ggplot2), using the Observed, Chao1, Shannon, and Simpson methods, as previously described (28). Beta diversity is a measure of the similarity or dissimilarity of the microbial composition between groups, and it was determined by principal coordinate analysis as proposed in QIIME v1.9.0 (Bray-Curtis method) and as previously described (28).
Identification of Gut Microbial Markers
Gut microbial markers were estimated using the linear discriminant analysis effect size (LEfSe) tool as described previously (33). The LEfSe tool uses a non-parametric factorial Kruskal–Wallis rank sum test to identify features with significantly differential relative abundance, followed by Linear Discriminant Analysis (LDA) to calculate the effect size of each differentially abundant microbial feature. Features were considered significant if the LDA value was >2.0.
Functional Pathway Profiling
We performed Phylogenetic Investigation of Communities by Reconstruction of Unobserved States (PICRUSt) analysis according to the literature review by Langille et al. (34). PICRUSt is a bioinformatics software package designed to predict metagenome functional content from marker gene surveys.
Association of Marker Microbes and Host Phenotype
We used a Statistical Inference of Associations between Microbial Communities and host phenoTypes (SIAMCAT) machine learning tool to identify the associations between marker microbes and host phenotype, and SIAMCAT analysis was performed according to Wirbel et al. and following ridge logistic regression analysis as described previously (35).
Correlation Between Diet and Microbial Composition
Correlation network analysis between diet and microbial composition was performed using GraphPad Prism software.
Statistical Analysis
A normality test was used to check the distribution of the patients' phenotypic data. Unless otherwise specified, data are presented as the median and interquartile range (IQR). Comparisons of the two groups were performed with Student's t-test and Mann–Whitney test, wherever applicable on Prism Software version 8, (GraphPad). A value of p < 0.05 was considered statistically significant.
Results
Demographic, Anthropometric, Clinical, and Biochemical Parameters of Study Participants
First, we estimated the demographic, anthropometric, clinical, and biochemical parameters for each study participant. Interestingly, we did find statistically significant difference in age, but not HR parameter between the CVD no-risk and CVD risk groups (Table 1). Additionally, we measured diabetes mellitus-related parameters, lipid profiles, liver function profiles, vitamins, thyroid function profiles, uric acid, and iron saturation levels for each patient. The CVD risk group was found to have higher levels of FBG, HbA1c, ALT, AST, and vitamin B12 than the CVD no-risk group (Table 1). The actual number of subjects used to derive the above data is mentioned in Supplementary Table 1.
Nutrient Intake of CVD No-Risk and CVD Risk Study Participants
We calculated the macro-and micro-nutritional intake of each study participant. The consumption of MUFA, polyunsaturated fat (PUFA), vitamin D, the sum of trans-fat and saturated fat, and phosphorus was significantly lower, but interestingly, folate consumption was higher in the CVD risk group than in the CVD no-risk group (Figure 1A). In addition, we attempted to find a correlation between nutritional intake and the risk of CVD and found that dietary consumption of sodium, salt, and beta-carotene positively correlated with CVD risk score, whereas vitamin D and lactose were negatively correlated with CVD risk (Figures 1B–F). The significant results in both the comparison and correlation analyses of vitamin D intake suggest vitamin D has a crucial role in the development of obesity-associated CVDs (Figures 1A,B).
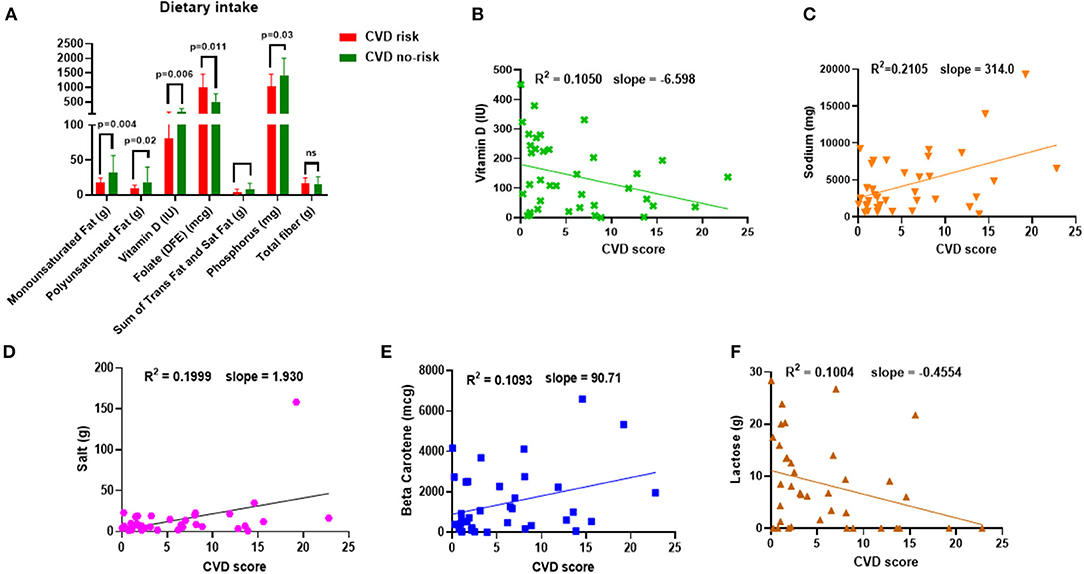
Figure 1. Dietary intake and correlation to CVD risk. (A) Dietary intake of MUFA, PUFA, vitamin D, folate, the sum of trans-fat and saturated fat, phosphorus, and total fiber in the CVD no-risk and CVD risk groups. (B–F) Correlation analysis of nutrient intake (sodium, salt, beta-carotene, lactose, and vitamin D) and CVD risk in the obese population. CVD no-risk N = 30; CVD risk N = 8. P < 0.05 was considered statistically significant using Student's t-test or Mann-Whitney test was performed, wherever applicable.
Gut Microbial Composition in Obese Subjects With Increased CVD Risk
We measured the gut microbial compositions of both the CVD no-risk and CVD risk groups. At the phyla level, the CVD risk group had a higher relative abundance of Proteobacteria (11.184 vs. 4.833%, p = 0.021) and lower relative abundances of Firmicutes (29.00 vs. 39.76%, p = 0.034) and Actinobacteria (0.91 vs. 2.81%, p < 0.014) than the CVD no-risk group (Figure 2A; Supplementary Figure 1). Although the CVD risk group had a higher proportion of Bacteroidetes (57.49%) than the CVD no-risk group (51.84%), statistically, the difference was not significant (Figure 2A; Supplementary Figure 1). We also estimated the Firmicutes and Bacteroidetes (F/B) and Firmicutes/Proteobacteria (F/P) ratios for the two groups. The CVD risk group F/B ratio showed no difference from that of the CVD no-risk group (p = 0.945) (Figure 2B), whereas the CVD risk group F/P ratio was significantly higher (49.4 vs. 10.13, p = 0.035) in the CVD risk group than that of the CVD no-risk group (49.4 vs. 10.13, p = 0.035) (Figure 2C).
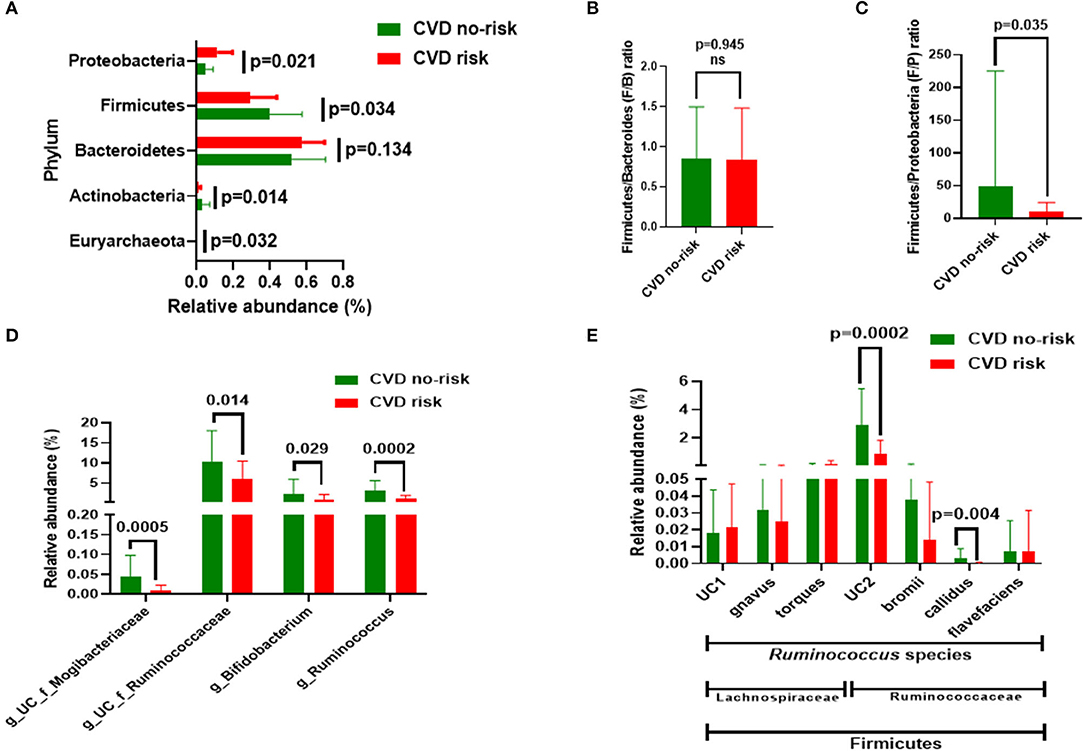
Figure 2. Gut microbial composition in the CVD no-risk and CVD risk groups. (A) The four major bacterial phyla, except Euryarchaeota, were found in both groups. Data are shown as relative abundance (percentage of total gut microbiota composed of each phylum). (B,C) Ratio of Firmicutes to Bacteroidetes and Firmicutes and Proteobacteria, respectively, in the CVD no-risk and CVD risk groups. (D) Relative abundance of four major genera in the CVD no-risk and CVD risk groups. (E) Relative abundance of species of the Ruminococcus genus from the families of Ruminococcaceae and Lachnospiraceae in the CVD risk and CVD no-risk groups. The results are expressed as mean ± SD. CVD no-risk, N = 36; CVD risk, N = 10. P < 0.05 was considered statistically significant using Student's t-test. “g_UC” and “f_UC” represent unclassified bacteria at the genus and family level, respectively.
We identified many genera that were significantly decreased in the CVD risk group compared with the CVD no-risk group, mainly the genera Ruminococcus (0.95 vs. 2.97%, p = 0.0002), unclassified Ruminocococcaceae family genera (5.82 vs. 10.36%, p = 0.014), and Bifidobacterium (0.75 vs. 2.20%, p = 0.03). Additionally, other genera, such as unclassified genera from the Mogibacteriaceae and Coriobacteriaceae families, and Clostridium, Christensenella, and Lactobacillus, were significantly lower in the CVD risk group than the CVD no-risk group (Figure 2D; Supplementary Figure 2).
A further exploration of the genus Ruminococcus to the species level identified R. callidus and an unclassified species from the Ruminococcaceae family as being significantly decreased in the CVD risk compared with the CVD no-risk group, whereas other species such as R. bromii and R. flavefaciens were not significantly changed. In addition, the relative abundances of other Ruminococcus species from the Lachnospiraceae family, such as R. gnavus, R. torques, and unclassified, were the same in the two groups (Figure 2E).
Gut Microbial Diversity in Obese Subjects With Increased CVD Risk
We estimated the microbial richness and abundance in the CVD risk and CVD no-risk groups using Observed, Chao1, Shannon, and Simpson methods. There was no significant change or shift in the microbial richness or the relative abundance in the CVD risk compared with the CVD no-risk group (Figures 3A–D). We estimated the similarities or dissimilarities between the two groups, and it did not uncover any similarities between the two groups (Figure 3E).
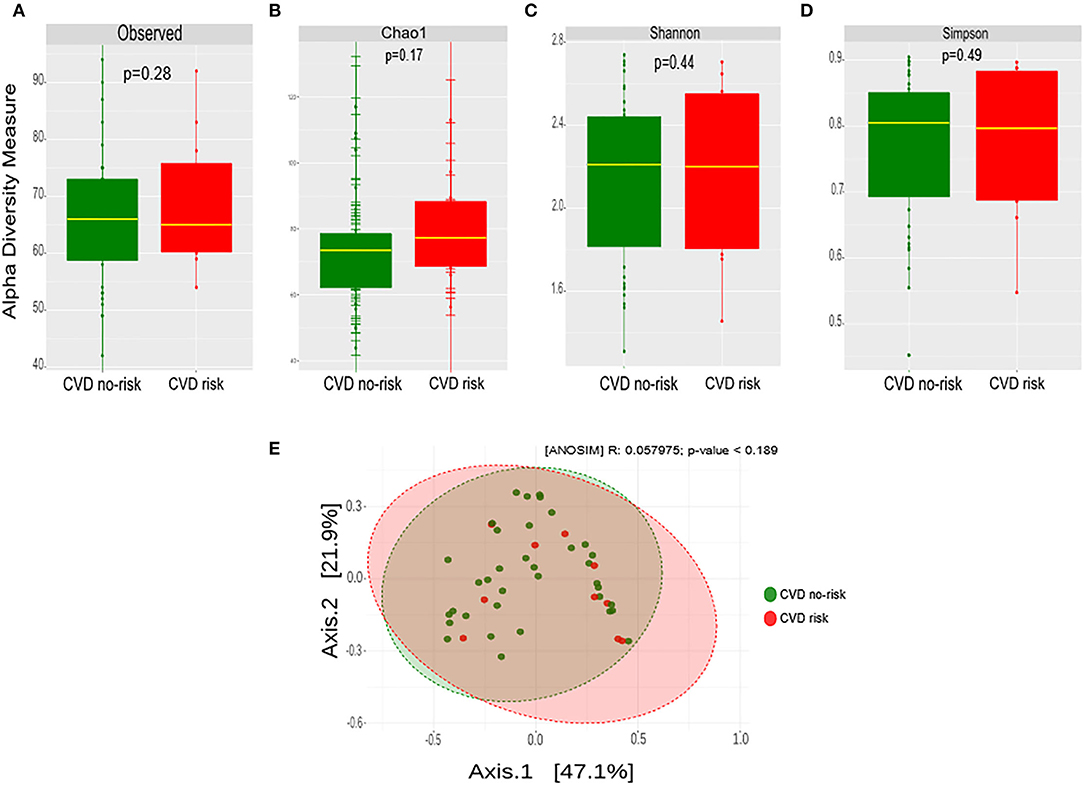
Figure 3. Gut microbial diversity in the CVD no-risk and CVD risk groups. (A–D) Alpha diversity was measured by the four commonly used methods, Observed, Chao1, Shannon, and Simpson. The boxplots show median and interquartile (IQR) range and whiskers extending to the most extreme points within 1.5-fold IQR. (E) Beta diversity index was measured by the Bray-Curtis method using principle coordinate analysis of the relative abundance of OTUs. The two-variances explained by Axis.1 and Axis.2 are 47.1 and 21.9%, respectively. ANOSIM, analysis of similarity; CVD no-risk, N = 36; CVD risk, N = 10. P < 0.05 was considered statistically significant using Student's t-test.
Microbial Markers in Obese Subjects With Increased CVD Risk
Next, we performed LEfSe analysis to identify differential bacterial genera between the two groups. The genera Ruminococcus, Shewanella, Treponema, and unclassified genera from the Mogibacteriaceae and Rs_045 families were found to be microbial markers, of which, only the genus Ruminococcus and an unclassified genus from the Mogibacteriaceae family were present across all obese subjects, and these two genera were significantly enriched in the CVD no-risk group compared with the CVD risk group. The other bacterial genera, such as Shewanella, Treponema, and an unclassified genus from the Rs_045 family, were enriched in the CVD risk group, and interestingly, these were not present in all obese subjects (Figure 4A; Supplementary Figure 3).
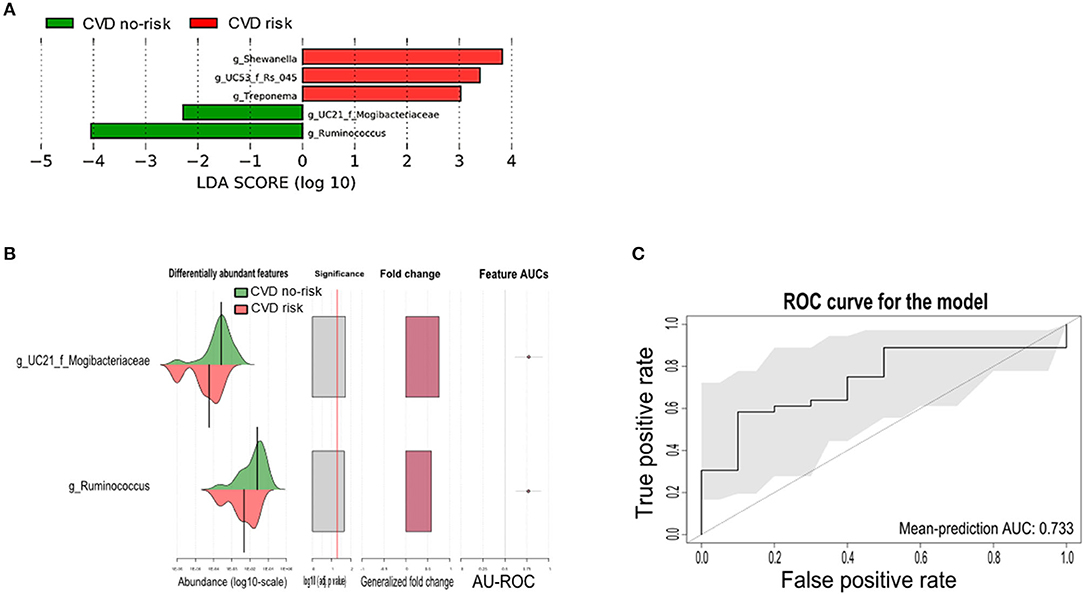
Figure 4. Gut microbial markers and their validation in the CVD risk group. (A) Gut microbial markers were measured by LEfSe analytical tool with an LDA cut-off value of >2.0 in both the CVD no-risk and CVD risk groups. (B,C) Gut microbial markers from LEfSe analysis were validated using the SIAMCAT tool, which displayed the cross-validation error as a receiver operating characteristic (ROC) curve with the 95% confidence interval shaded in gray. The area under the ROC (AUROC = 0.733) is given below the curve. The x-axis and y-axis represent false-positive and true-positive rates, respectively, for the tested markers. An AUROC value of more than 0.7 is considered fairly good in terms of the test's discriminative ability. CVD no-risk, N = 36; CVD risk, N = 10. “g_UC”: unclassified bacteria at the genus level.
We then evaluated the potential associations between the gut microbial markers and host phenotype using the SIAMCAT tool. We found that the gut microbial markers, such as the genus Ruminococcus and the unclassified genus from the Mogibacteriaceae family, were significantly enriched (mean AUC of the ROC curve was 0.733) and associated with the CVD no-risk group, suggesting their vital role in obesity-associated CVD (Figures 4B,C).
Gut Microbiota-Modulated Metabolic Pathways That Are Associated With Increased CVD Risk in Obese Subjects
We performed PICRUSt analysis to find potential pathways that are modulated based on microbial abundance in the CVD risk and CVD no-risk groups. The results revealed that metabolism of lipoic acid, taurine, and hypotaurine pathways were elevated, whereas the polycyclic aromatic hydrocarbon degradation pathway was decreased in the CVD risk group compared with the CVD no-risk group (Figure 5).
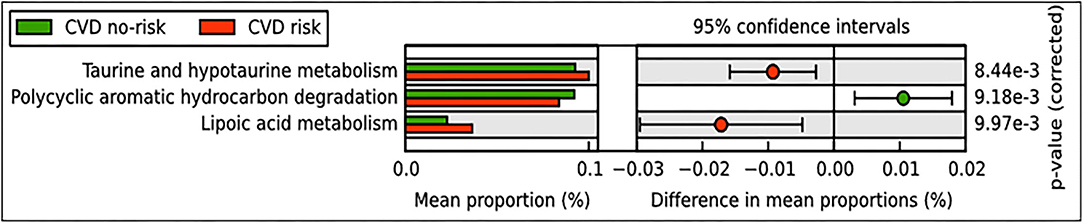
Figure 5. Predicted functional pathways in CVD no-risk and CVD risk groups. Predicted functional pathways were obtained using the PICRUSt method based on bacterial relative abundance shown as mean proportions in the CVD no-risk and CVD risk groups. P < 0.01 was considered statistically significant. CVD no-risk, N = 36; CVD risk, N = 10.
Nutrient Components Responsible for the Relative Abundance of Genus Ruminococcus in Obese Subjects
Finally, we performed correlation matrix analysis of data on diet and microbial composition. We found that different species of the genus Ruminococcus were positively correlated with the dietary intake of monounsaturated fat, vitamin D, vitamin A, and protein (Figure 6).
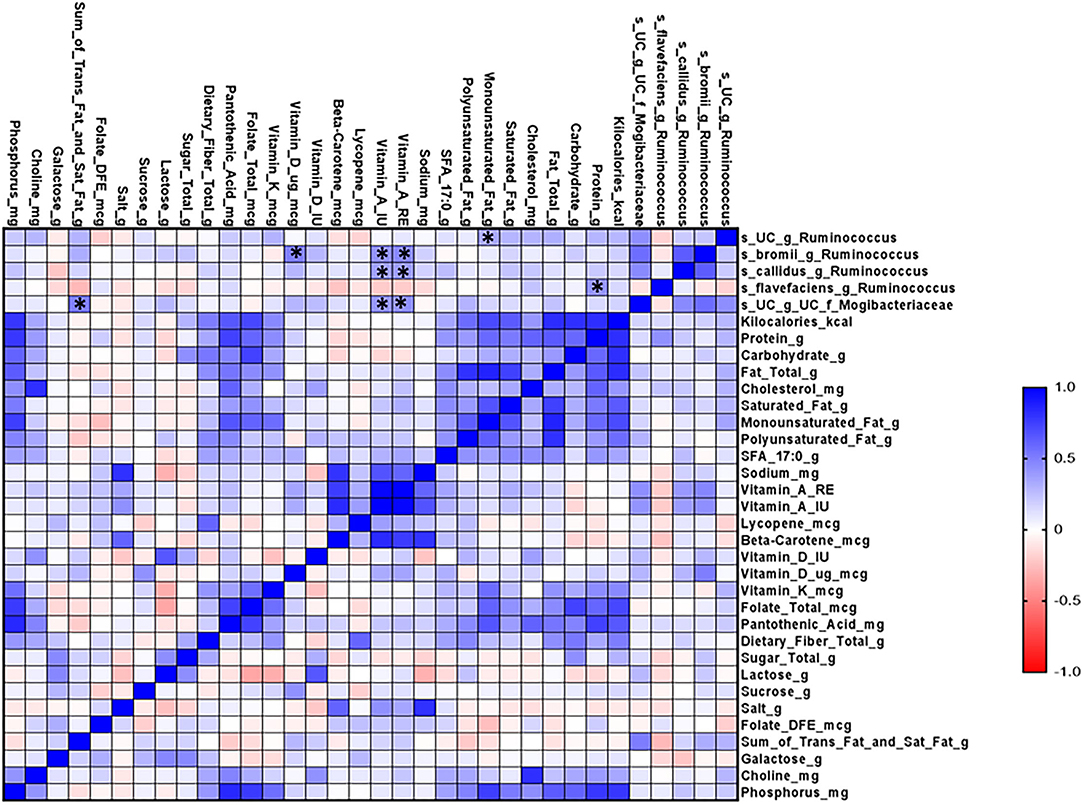
Figure 6. Microbial correlation matrix analysis of diet and gut microbiome in the CVD no-risk and CVD risk groups. Correlation matrix analysis was performed using GraphPad Prism software. The red line indicates a negative correlation and the blue line indicates a positive correlation. CVD no-risk, N = 30; CVD risk N = 8. *p < 0.05 was considered statistically significant using Student's t-test.
Discussion
At the phyla level, three major bacteria were modulated in the CVD risk group in this study, namely Firmicutes (decreased), Proteobacteria (increased), and Actinobacteria (decreased), compared with those in the CVD no-risk group. Moreover, though the relative abundance of Bacteroidetes was higher in the CVD risk group than the CVD no-risk group, the difference was not statistically significant (p > 0.05) (Figure 2A). Interestingly, when we analyzed the F/B ratio, it was not significantly altered in the CVD risk group (Figure 2B). Also, Of note, Duncan et al. demonstrated that, in the clinical setting, Firmicutes and Bacteroidetes had no influence on obesity development (36). However, the reduction in the Firmicutes relative abundance was compensated by the increased relative abundance of Proteobacteria, rather than Bacteroidetes, in the CVD risk group, which is evident from the decreased Firmicutes to Proteobacteria (F/P) ratio (Figure 3C). Shirey et al. also reported no change in the F/B ratio, but they found a significant decrease in the F/P ratio in infant gut microbiota (37). Thus, changes in the abundance of Firmicutes compared with Proteobacteria could be more influential for obesity-associated CVD risk than the F/B ratio.
In our study, we found no change in microbial richness, relative abundance, or beta diversity between the CVD risk and CVD no-risk groups (Figures 3A–E), suggesting there was no major shift in microbial composition, but there were alterations in the relative abundances of a few bacteria that could play roles in the development of obesity-associated CVDs. The relative abundances of many genera were significantly reduced in the CVD risk group, especially Ruminococcus, Bifidobacterium, Lactobacillus, Christensenella, Clostridium, and unclassified genera from the Ruminococcaceae, Mogibacteriaceae, and Coriobacteriaceae families (Figure 2D; Supplementary Figure 2). Identification of microbial markers by LEfSe analysis revealed the CVD risk group was enriched in a few bacteria genera, such as Shewanella, Treponema, and an unclassified genus from the family Rs-045, and depleted in Ruminococcus and an unclassified genus from the Mogibacteriaceae family (Figure 4A). We also employed the SIAMCAT pipeline to understand the relationships between microbial communities and host phenotype and discovered that the genus Ruminococcus and an unclassified genus from the Mogibacteriaceae family were significantly associated with obesity-associated CVD risk (ROC curve AUC = 0.733) (Figures 4B,C).
Multiple studies have demonstrated that diet is one of the most prominent epigenetic factors affecting the gut microbial composition in terms of both bacterial richness and abundance (23, 24). It is believed that acute dietary adjustment can change the microbial composition dramatically, but it does not cause a switch from one enterotype to another due to its stability, whereas chronic dietary adjustment does facilitate enterotype switching (26). In addition to its stability, a classic feature of the enterotype is its reversibility. Enterotypes are affected by various factors, such as diet, lifestyle, age, and environmental stress (38, 39). In our study, 24-h dietary analysis revealed that the CVD risk group consumed lower amounts of MUFA, PUFA, vitamin D, total trans-fat and saturated fat, and phosphorus, and a higher amount of folate (Figure 1A).
The most common MUFAs in the diet are oleic acid, palmitoleic acid, vaccenic acid, and eicosenoic acid (40). Long-term high-fat consumption may significantly affect the host phenotype through the modulation of gut microbiota (Firmicutes, Bacteroidetes, Actinobacteria, and Proteobacteria) and gut microbiota-derived SCFAs, and monounsaturated fat (MUFA) reduces obesity and obesity-associated CVDs by activating the synthetic and catabolic pathways of triglyceride-rich lipoprotein metabolism (41, 42). Hidalgo et al. (43) demonstrated that supplementation with the MUFA (oleic acid) to spontaneously hypertensive rats for 12 weeks significantly improved their hypertension through the up-regulation of Ruminococcaceae. In the latest report, Goiri et al. described a positive correlation between the consumption of MUFA and the relative abundance of Ruminococcus in dairy cows (44). In this study, we found that intake of dietary MUFA was positively correlated with the relative abundance of Ruminococcus. A study conducted by Clarke and Mozaffarian suggested that the intake of MUFA (1% of total energy content) significantly altered various CVD risk factors, mainly complete lipid profiles and apolipoproteins (45). In addition, Mente et al. reported that MUFA intake negatively correlated with coronary heart disease (46). In contrast to this, several meta-analyses have suggested that MUFA intake does not affect CHD risk and related deaths (47, 48). Thus, it is unclear if MUFA intake has protective effects against CVDs, and more comprehensive intervention studies are needed to clarify these discrepancies. Overall, however, the intake of MUFA might prove have beneficial effects on CVD risk through the up-regulation of Ruminococcus. Furthermore, numerous studies have explained in detail how the intake of PUFAs, such as linolenic acid and linoleic acid, can protect against obesity-associated CVDs (49, 50). Noriega et al. (51) demonstrated that supplementation with omega-3 PUFAs for 2 weeks increased the relative abundance of the genus Ruminococcus. Interestingly, although the CVD risk group in our study consumed a lower amount of PUFAs, we did not find any correlation between PUFA and the relative abundance of the genus Ruminococcus, suggesting that the beneficial effects of PUFAs are mediated by alternative mechanisms other than modulation of the genus Ruminococcus.
Recent evidence suggests that the beneficial effects of vitamin D are mainly modulated via the gut microbiota, along with other demonstrated mechanisms (52–54). In this study, we found that vitamin D consumption was significantly lower in the CVD risk group than the CVD no-risk group. An interesting study recently conducted by Singh et al. (55) showed that vitamin D supplementation modulated the relative abundance of Ruminococcus in healthy subjects from Qatar. A point to be considered is that, when they categorized the subjects as responders (more than 20 ng/μl) or non-responders (<20 ng/μl) based on the dose of supplemented vitamin D, they found that higher vitamin D was related Ruminococcus bacteria species (R. bromii) enrichment, whereas when they compared data from subjects pre- or post-vitamin D supplementation, vitamin D supplementation inversely correlated with the relative abundance of the genus Ruminococcus. Furthermore, Thomas et al. (56) demonstrated that a higher level of 1,25(OH)2D (the active form of vitamin D) was positively associated with the genus Ruminococcus. Researchers should be careful in drawing conclusions on the roles of the genus Ruminococcus because its members are assigned to both Ruminococcaceae and Lachnospiraceae families and could have distinct biological roles related to vitamin D levels. In support of this, Ghaly et al. (57) demonstrated that vitamin D supplementation led to enrichment in both Ruminococcaceae and Lachnospiraceae families in a dose-dependent manner, i.e., moderate amounts of vitamin D increased Ruminococcaceae, whereas high amounts of vitamin D increased Lachnospiraceae, which can worsen colitis conditions. In our study, we found no change in the relative abundances of bacterial species from the Lachnospiraceae family, whereas species from Ruminococcaceae family (especially, R. callidus and an unclassified Ruminococcus species) decreased in the CVD risk group in comparison to the CVD no-risk group (Figure 2E). Furthermore, correlation analysis revealed that vitamin D intake was negatively correlated with obesity-associated CVD risk (Figure 1B); thus, vitamin D intake seems to be associated with the relative abundance of the genus Ruminococcus, and its levels are critical in defining the relative abundance of the genus Ruminococcus from either Ruminococcaceae or Lachnospiraceae families.
Ruminococcus is a member of one of the three enterotypes (Enterotype 3) that are thought to be involved in shaping core microbiota; enterotypes are not often perturbated by acute stimuli, rather are believed to be stable (58). Contrary to this, Liang et al. (59) found another enterotype dominated by Enterobacteriaceae in a population in Taiwan, suggesting that enterotypes are quite robust among populations. Ruminococcus has a predominant role in the fermentation of dietary fiber to produce SCFAs, butyrates that are important energy sources for intestinal epithelial cells (60). Butyrates can have beneficial actions, such as maintaining intestinal barrier function and regulating the expression of various genes related to lipids, inflammatory and immune responses, and cell cycle processes (61–63) through complex mechanisms, such as inhibiting histone deacetylation, secreting glucagon-like peptide 1, regulating inflammatory mediators, enhancing antioxidant capacity, and activating the PPAR-γ pathway (64–66). Many studies have suggested that a reduction in butyrate production can harm the homeostasis of gut flora and cause leaky gut, which has been linked to the development of obesity-associated CVDs (67). Interestingly, when we analyzed the dietary data, we did not find any difference in dietary fiber intake between the CVD risk and CVD no-risk groups (Figure 1A), suggesting that the genus Ruminoccoccus has a beneficial role other than butyrate production. In addition, contrary reports suggest that age can influence the relative abundance of Ruminococcus, which decreases with age (68), whereas Arumugam et al. reported that Ruminococcus are not modulated by age, BMI, gender, or nationality (58), rather it was demonstrated to be modulated according to dietary constituents (69). In this study, we also found that subjects in the CVD risk group had a significantly higher median age than the CVD no-risk group (Table 1). Therefore, because of its heterogenicity, there may be both positive and negative effects from Ruminococcus; some species (for example R. bromii) has shown potential beneficial effects through degradation of resistant starch in shaping the gut microbial composition (70), whereas R. gnavus has been implicated in the pro-inflammatory process in Crohn's disease (71). In this study, we identified many species of the genus Ruminococcus from the Ruminococcaceae family and found R. callidus and unclassified Ruminococcus species to be significantly decreased in the CVD risk group. In addition, other Ruminococcus species of the Lachnospiraceae family were unaltered in the CVD risk group, which potentially explains the heterogeneous role of the genus Ruminococcus.
Obesity is an important risk factor for CVDs and promotes disease development through complex mechanisms; predominantly through the activation of inflammatory and oxidative stress pathways (10, 72). Obesity is also a key factor in the development of liver injury, insulin resistance, and diabetes mellitus. Li et al. (73) reported that the relative abundance of the genus Ruminococcus was lower in non-alcoholic fatty liver disease (NAFLD) patients compared with healthy subjects, but Boursier et al. (74) and others found that Ruminococcus sp. were decreased in patients with fibrosis. Lee et al. (75) also found that Ruminococcus species, especially R. faecis, reduced the increased levels of ALT seen in an experimental NAFLD mouse model. In our study, the CVD risk subjects also had higher levels of ALT and diabetic parameters such as HbA1c and FBG than the CVD no-risk subjects (Table 1). Pathway analysis by the PICRUSt tool revealed many pathways that were modulated, especially taurine and hypotaurine metabolism, lipoic acid metabolism, and the polycyclic aromatic hydrocarbon degradation pathway (Figure 5). Taurine and hypotaurine are non-essential amino acids that can be obtained from the diet. The heart, skeletal muscle, and retina were found to have high concentrations of taurine (76, 77), and taurine has been involved in many diverse biological functions, primarily bile salt formation and fat digestion. The primary roles of taurine are its antioxidant and anti-inflammatory properties. A handful of clinical trials on taurine supplementation have suggested that taurine significantly improves many pathological conditions, including dilated cardiomyopathy, ischemic heart disease, hypertension, congestive heart failure, atherosclerosis, and obesity (78, 79). In addition, Fang et al. demonstrated that taurine supplementation significantly ameliorated the reduction in the relative abundance of Ruminococcus in immunosuppressed mice, suggesting a positive correlation between these two entities (80). Moreover, lipoic acid, a potent natural antioxidant, has been reported to alleviate various CVDs associated with elevated levels of oxidative stress (81). Polyaromatic hydrocarbons are known carcinogens and have been implicated in the pathogenesis of various CVDs through the activation of inflammatory responses (82, 83). In our study, we found that the CVD risk group had higher levels of taurine, hypotaurine, and lipoic acid metabolism and lower levels of polycyclic aromatic hydrocarbon degradation than the CVD no-risk group (Figure 5). Thus, it seems that the genus Ruminococcus and an unclassified genus of the Mogibacteriaceae family might prevent obesity-associated CVDs through the suppression of oxidative stress and inflammatory responses.
Out pilot study had some limitations, such as (1) the CVD risk group included fewer subjects than the CVD no-risk group and (2) recording of food intake was based on the 24-h recall method. However, the findings from this pilot study are promising and will be further evaluated in a comprehensive study with a larger cohort.
Conclusions
Although there were fewer samples in the CVD risk group, the findings clearly showed this group had gut microbial dysbiosis that did not cause a drastic change in the gut microbial diversity but rather a moderate shift from Firmicutes to Proteobacteria. This minor shift had a potentially negative effect on obesity severity. The reduced relative abundance of Ruminococcus from the Ruminococcaceae family and an unclassified genus from the Mogibacteriaceae family significantly increased the risk of CVDs, and this unfavorable effect might be mediated by increased oxidative stress and the activation of inflammatory pathways. However, future mechanistic and intervention studies are warranted to confirm the findings of this study, which could be useful for the management of CVDs in obese conditions. We summarized this hypothesis in the graphical abstract (Figure 7).
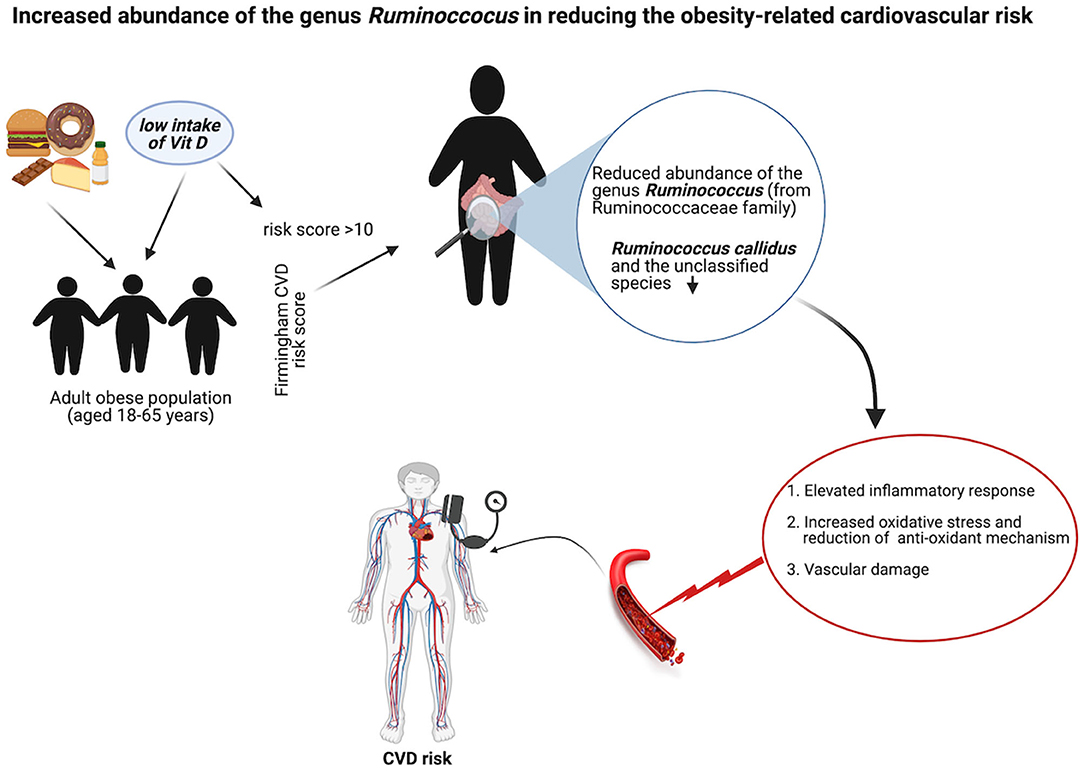
Figure 7. Schematic representation of the role of Ruminococcus in obese-related CVD. Created with BioRender.com.
Data Availability Statement
The datasets presented in this study can be found in online repositories. The names of the repository/repositories and accession number(s) can be found below: https://www.ncbi.nlm.nih.gov/, PRJNA785127.
Ethics Statement
The study participants were approached and recruited after having consented at Hamad Medical Corporation, Doha, Qatar. This study was approved by the Institutional Review Board from both the Sidra Medicine (IRB, #1604002867) and Hamad Medical Corporation (IRB, #16419/16).
Author Contributions
AT and WE designed the study and reviewed the manuscript. WE was involved in the subject recruitment process and sample collection process. AL and DB processed the samples. AL performed gut microbiome data analysis and wrote the manuscript. SA-S performed diet assessment. SA analyzed dietary intake. All authors contributed to the article and approved the submitted version.
Funding
This study was supported by Sidra Medicine, Doha, Qatar (SDR400005).
Conflict of Interest
The authors declare that the research was conducted in the absence of any commercial or financial relationships that could be construed as a potential conflict of interest.
Publisher's Note
All claims expressed in this article are solely those of the authors and do not necessarily represent those of their affiliated organizations, or those of the publisher, the editors and the reviewers. Any product that may be evaluated in this article, or claim that may be made by its manufacturer, is not guaranteed or endorsed by the publisher.
Acknowledgments
We acknowledge Sidra Medicine, Doha, Qatar, for funding the project. We also would like to thank Ms. Hala Shabana, a dietician at HMC, for her contribution to the dietary data collection, Mrs. Maha Ibrahim and Ammar Al Najem, phlebotomists at HMC, for helping with patient blood draws, and Ms. Neveen Azmy Emam for sample handling and storage. Finally, we acknowledge Insight Editing London for the language editing service.
Supplementary Material
The Supplementary Material for this article can be found online at: https://www.frontiersin.org/articles/10.3389/fnut.2022.849005/full#supplementary-material
Abbreviations
ALP, alkaline phosphatase; ALT, alanine transaminase; AST, aspartate transaminase; BMI, body mass index; BMR, basal metabolic rate; BMR_C, basal metabolic rate calorie; BP, blood pressure; CVDs, cardiovascular diseases; F/B, Firmicutes/Bacteroidetes; F/P, Firmicutes/Proteobacteria; FBG, fasting blood glucose; FFM, free fat mass; FM, fat mass; HbA1c, glycosylated hemoglobin; HDL, high-density lipoprotein; HR, heart rate; HT, height; LDL, low-density lipoprotein; MM, muscle mass; MUFA, monounsaturated fat; PUFA, polyunsaturated fat; SCFAs, short-chain fatty acids; T3, triiodothyronine; T4, thyroxine; TC, total cholesterol; TG, triglycerides; TMAO, trimethylamine-N-oxide; TSH, thyroid-stimulating hormone.
References
1. Mohideen FS, Rajkumar Honest PC, Syed MA, David KV, Abdulmajeed J, Ramireddy N. Prevalence of multimorbidity among adults attending primary health care centres in Qatar: a retrospective cross-sectional study. J Family Med Prim Care. (2021) 10:1823–8. doi: 10.4103/jfmpc.jfmpc_2446_20
2. Schwartz MW, Seeley RJ, Zeltser LM, Drewnowski A, Ravussin E, Redman LM, et al. Obesity pathogenesis: an endocrine society scientific statement. Endocr Rev. (2017) 38:267–96. doi: 10.1210/er.2017-00111
3. Loos RJ. Genetic determinants of common obesity and their value in prediction. Best Pract Res Clin Endocrinol Metab. (2012) 26:211–26. doi: 10.1016/j.beem.2011.11.003
4. Gebreab SZ, Vandeleur CL, Rudaz D, Strippoli MF, Gholam-Rezaee M, Castelao E, et al. Psychosocial stress over the lifespan, psychological factors, and cardiometabolic risk in the community. Psychosom Med. (2018) 80:628–39. doi: 10.1097/PSY.0000000000000621
5. Singh P, Rai SN. Factors affecting obesity and its treatment. Obesity Med. (2019) 16:1–9. doi: 10.1016/j.obmed.2019.100140
6. Zhang Y, Liu J, Yao J, Ji G, Qian L, Wang J, et al. Obesity: pathophysiology and intervention. Nutrients. (2014) 6:5153–83. doi: 10.3390/nu6115153
7. Harakeh SM, Khan I, Kumosani T, Barbour E, Almasaudi SB, Bahijri SM, et al. Gut microbiota: a contributing factor to obesity. Front Cell Infect Microbiol. (2016) 6:95. doi: 10.3389/fcimb.2016.00095
8. Ley RE, Backhed F, Turnbaugh P, Lozupone CA, Knight RD, Gordon JI. Obesity alters gut microbial ecology. Proc Natl Acad Sci USA. (2005) 102:11070–5. doi: 10.1073/pnas.0504978102
9. Vamanu E, Rai SN. The link between obesity, microbiota dysbiosis, and neurodegenerative pathogenesis. Diseases. (2021) 9:45. doi: 10.3390/diseases9030045
10. Carbone S, Canada JM, Billingsley HE, Siddiqui MS, Elagizi A, Lavie CJ. Obesity paradox in cardiovascular disease: where do we stand? Vasc Health Risk Manag. (2019) 15:89–100. doi: 10.2147/VHRM.S168946
11. Powell-Wiley TM, Poirier P, Burke LE, Despres JP, Gordon-Larsen P, Lavie CJ, et al. Obesity and cardiovascular disease: a scientific statement from the American Heart Association. Circulation. (2021) 143:e984–1010. doi: 10.1161/CIR.0000000000000973
12. Tedgui A, Owens AP III, Mackman N. 2011 Nobel Prize in physiology or medicine: toll-like receptors, dendritic cells, and their roles in atherosclerosis. Arterioscler Thromb Vasc Biol. (2011) 31:2767–8. doi: 10.1161/ATVBAHA.111.240432
13. Koeth RA, Wang Z, Levison BS, Buffa JA, Org E, Sheehy BT, et al. Intestinal microbiota metabolism of L-carnitine, a nutrient in red meat, promotes atherosclerosis. Nat Med. (2013) 19:576–85. doi: 10.1038/nm.3145
14. Wang Z, Klipfell E, Bennett BJ, Koeth R, Levison BS, Dugar B, et al. Gut flora metabolism of phosphatidylcholine promotes cardiovascular disease. Nature. (2011) 472:57–63. doi: 10.1038/nature09922
15. Zhu W, Gregory JC, Org E, Buffa JA, Gupta N, Wang Z, et al. Gut microbial metabolite TMAO enhances platelet hyperreactivity and thrombosis risk. Cell. (2016) 165:111–24. doi: 10.1016/j.cell.2016.02.011
16. Drouin-Chartier JP, Cote JA, Labonte ME, Brassard D, Tessier-Grenier M, Desroches S, et al. Comprehensive review of the impact of dairy foods and dairy fat on cardiometabolic risk. Adv Nutr. (2016) 7:1041–51. doi: 10.3945/an.115.011619
17. Isolauri E. Microbiota and obesity. Nestle Nutr Inst Workshop Ser. (2017) 88:95–105. doi: 10.1159/000455217
18. Marzullo P, Di Renzo L, Pugliese G, De Siena M, Barrea L, Muscogiuri G, et al. From obesity through gut microbiota to cardiovascular diseases: a dangerous journey. Int J Obes Suppl. (2020) 10:35–49. doi: 10.1038/s41367-020-0017-1
19. Ley RE, Turnbaugh PJ, Klein S, Gordon JI. Microbial ecology: human gut microbes associated with obesity. Nature. (2006) 444:1022–3. doi: 10.1038/4441022a
20. Duan M, Wang Y, Zhang Q, Zou R, Guo M, Zheng H. Characteristics of gut microbiota in people with obesity. PLoS ONE. (2021) 16:e0255446. doi: 10.1371/journal.pone.0255446
21. Lin SW, Freedman ND, Shi J, Gail MH, Vogtmann E, Yu G, et al. Beta-diversity metrics of the upper digestive tract microbiome are associated with body mass index. Obesity. (2015) 23:862–9. doi: 10.1002/oby.21020
22. Fu J, Bonder MJ, Cenit MC, Tigchelaar EF, Maatman A, Dekens JA, et al. The gut microbiome contributes to a substantial proportion of the variation in blood lipids. Circ Res. (2015) 117:817–24. doi: 10.1161/CIRCRESAHA.115.306807
23. Turnbaugh PJ, Ridaura VK, Faith JJ, Rey FE, Knight R, Gordon JI. The effect of diet on the human gut microbiome: a metagenomic analysis in humanized gnotobiotic mice. Sci Transl Med. (2009) 1:6ra14. doi: 10.1126/scitranslmed.3000322
24. Faith JJ, McNulty NP, Rey FE, Gordon JI. Predicting a human gut microbiota's response to diet in gnotobiotic mice. Science. (2011) 333:101–4. doi: 10.1126/science.1206025
25. David LA, Materna AC, Friedman J, Campos-Baptista MI, Blackburn MC, Perrotta A, et al. Host lifestyle affects human microbiota on daily timescales. Genome Biol. (2014) 15:R89. doi: 10.1186/gb-2014-15-7-r89
26. Wu GD, Chen J, Hoffmann C, Bittinger K, Chen YY, Keilbaugh SA, et al. Linking long-term dietary patterns with gut microbial enterotypes. Science. (2011) 334:105–8. doi: 10.1126/science.1208344
27. Wilson PW, D'Agostino RB, Levy D, Belanger AM, Silbershatz H, Kannel WB. Prediction of coronary heart disease using risk factor categories. Circulation. (1998) 97:1837–47. doi: 10.1161/01.CIR.97.18.1837
28. Lakshmanan AP, Shatat IF, Zaidan S, Jacob S, Bangarusamy DK, Al-Abduljabbar S, et al. Bifidobacterium reduction is associated with high blood pressure in children with type 1 diabetes mellitus. Biomed Pharmacother. (2021) 140:111736. doi: 10.1016/j.biopha.2021.111736
29. Zhang J, Kobert K, Flouri T, Stamatakis A. PEAR: a fast and accurate illumina paired-end reAd mergeR. Bioinformatics. (2014) 30:614–20. doi: 10.1093/bioinformatics/btt593
30. Bolger AM, Lohse M, Usadel B. Trimmomatic: a flexible trimmer for Illumina sequence data. Bioinformatics. (2014) 30:2114–20. doi: 10.1093/bioinformatics/btu170
31. Caporaso JG, Kuczynski J, Stombaugh J, Bittinger K, Bushman FD, Costello EK, et al. QIIME allows analysis of high-throughput community sequencing data. Nat Methods. (2010) 7:335–6. doi: 10.1038/nmeth.f.303
32. DeSantis TZ, Hugenholtz P, Larsen N, Rojas M, Brodie EL, Keller K, et al. Greengenes, a chimera-checked 16S rRNA gene database and workbench compatible with ARB. Appl Environ Microbiol. (2006) 72:5069–72. doi: 10.1128/AEM.03006-05
33. Lakshmanan AP, Al Za'abi M, Ali BH, Terranegra A. The influence of the prebiotic gum acacia on the intestinal microbiome composition in rats with experimental chronic kidney disease. Biomed Pharmacother. (2021) 133:110992. doi: 10.1016/j.biopha.2020.110992
34. Langille MG, Zaneveld J, Caporaso JG, McDonald D, Knights D, Reyes JA, et al. Predictive functional profiling of microbial communities using 16S rRNA marker gene sequences. Nat Biotechnol. (2013) 31:814–21. doi: 10.1038/nbt.2676
35. Wirbel J, Zych K, Essex M, Karcher N, Kartal E, Salazar G, et al. Microbiome meta-analysis and cross-disease comparison enabled by the SIAMCAT machine learning toolbox. Genome Biol. (2021) 22:93–119. doi: 10.1186/s13059-021-02306-1
36. Duncan SH, Lobley GE, Holtrop G, Ince J, Johnstone AM, Louis P, et al. Human colonic microbiota associated with diet, obesity and weight loss. Int J Obes. (2008) 32:1720–4. doi: 10.1038/ijo.2008.155
37. Shirey TB, Dykes JK, Luquez C, Maslanka SE, Raphael BH. Characterizing the fecal microbiota of infants with botulism. Microbiome. (2015) 3:54. doi: 10.1186/s40168-015-0119-0
38. Claesson MJ, Cusack S, O'Sullivan O, Greene-Diniz R, de Weerd H, Flannery E, et al. Composition, variability, and temporal stability of the intestinal microbiota of the elderly. Proc Natl Acad Sci USA. (2011) 108(Suppl. 1):4586–91. doi: 10.1073/pnas.1000097107
39. Palmer C, Bik EM, DiGiulio DB, Relman DA, Brown PO. Development of the human infant intestinal microbiota. PLoS Biol. (2007) 5:e177. doi: 10.1371/journal.pbio.0050177
40. Orsavova J, Misurcova L, Ambrozova JV, Vicha R, Mlcek J. Fatty acids composition of vegetable oils and its contribution to dietary energy intake and dependence of cardiovascular mortality on dietary intake of fatty acids. Int J Mol Sci. (2015) 16:12871–90. doi: 10.3390/ijms160612871
41. Zheng C, Khoo C, Furtado J, Ikewaki K, Sacks FM. Dietary monounsaturated fat activates metabolic pathways for triglyceride-rich lipoproteins that involve apolipoproteins E and C-III. Am J Clin Nutr. (2008) 88:272–81. doi: 10.1093/ajcn/88.2.272
42. Machate DJ, Figueiredo PS, Marcelino G, Guimaraes RCA, Hiane PA, Bogo D, et al. Fatty acid diets: regulation of gut microbiota composition and obesity and its related metabolic dysbiosis. Int J Mol Sci. (2020) 21:4093. doi: 10.3390/ijms21114093
43. Hidalgo M, Prieto I, Abriouel H, Villarejo AB, Ramirez-Sanchez M, Cobo A, et al. Changes in gut microbiota linked to a reduction in systolic blood pressure in spontaneously hypertensive rats fed an extra virgin olive oil-enriched diet. Plant Foods Hum Nutr. (2018) 73:1–6. doi: 10.1007/s11130-017-0650-1
44. Goiri I, Zubiria I, Lavin JL, Benhissi H, Atxaerandio R, Ruiz R, et al. Evaluating the inclusion of cold-pressed rapeseed cake in the concentrate for dairy cows upon ruminal biohydrogenation process, ruminal microbial community and milk production and acceptability. Animals. (2021) 11:2553. doi: 10.3390/ani11092553
45. Mozaffarian D, Clarke R. Quantitative effects on cardiovascular risk factors and coronary heart disease risk of replacing partially hydrogenated vegetable oils with other fats and oils. Eur J Clin Nutr. (2009) 63(Suppl. 2):S22–33. doi: 10.1038/sj.ejcn.1602976
46. Mente A, de Koning L, Shannon HS, Anand SS. A systematic review of the evidence supporting a causal link between dietary factors and coronary heart disease. Arch Intern Med. (2009) 169:659–69. doi: 10.1001/archinternmed.2009.38
47. Hooper L, Summerbell CD, Thompson R, Sills D, Roberts FG, Moore HJ, et al. Reduced or modified dietary fat for preventing cardiovascular disease. Cochr Database Syst Rev. (2012) CD002137. doi: 10.1002/14651858.CD002137.pub3
48. Skeaff CM, Miller J. Dietary fat and coronary heart disease: summary of evidence from prospective cohort and randomised controlled trials. Ann Nutr Metab. (2009) 55:173–201. doi: 10.1159/000229002
49. Huang CW, Chien YS, Chen YJ, Ajuwon KM, Mersmann HM, Ding ST. Role of n-3 polyunsaturated fatty acids in ameliorating the obesity-induced metabolic syndrome in animal models and humans. Int J Mol Sci. (2016) 17:1689. doi: 10.3390/ijms17101689
50. Mozaffarian D, Ascherio A, Hu FB, Stampfer MJ, Willett WC, Siscovick DS, et al. Interplay between different polyunsaturated fatty acids and risk of coronary heart disease in men. Circulation. (2005) 111:157–64. doi: 10.1161/01.CIR.0000152099.87287.83
51. Noriega BS, Sanchez-Gonzalez MA, Salyakina D, Coffman J. Understanding the impact of omega-3 rich diet on the gut microbiota. Case Rep Med. (2016) 2016:3089303. doi: 10.1155/2016/3089303
52. Cantorna MT, Lin YD, Arora J, Bora S, Tian Y, Nichols RG, et al. Vitamin D regulates the microbiota to control the numbers of RORgammat/FoxP3+ regulatory T cells in the colon. Front Immunol. (2019) 10:1772. doi: 10.3389/fimmu.2019.01772
53. Yamamoto EA, Jorgensen TN. Relationships between vitamin D, gut microbiome, and systemic autoimmunity. Front Immunol. (2019) 10:3141. doi: 10.3389/fimmu.2019.03141
54. Akimbekov NS, Digel I, Sherelkhan DK, Lutfor AB, Razzaque MS. Vitamin D and the host-gut microbiome: a brief overview. Acta Histochem Cytochem. (2020) 53:33–42. doi: 10.1267/ahc.20011
55. Singh P, Rawat A, Alwakeel M, Sharif E, Al Khodor S. The potential role of vitamin D supplementation as a gut microbiota modifier in healthy individuals. Sci Rep. (2020) 10:21641. doi: 10.1038/s41598-020-77806-4
56. Thomas RL, Jiang L, Adams JS, Xu ZZ, Shen J, Janssen S, et al. Vitamin D metabolites and the gut microbiome in older men. Nat Commun. (2020) 11:5997. doi: 10.1038/s41467-020-19793-8
57. Ghaly S, Kaakoush NO, Lloyd F, McGonigle T, Mok D, Baird A, et al. High dose vitamin D supplementation alters faecal microbiome and predisposes mice to more severe colitis. Sci Rep. (2018) 8:11511. doi: 10.1038/s41598-018-29759-y
58. Arumugam M, Raes J, Pelletier E, Le Paslier D, Yamada T, Mende DR, et al. Enterotypes of the human gut microbiome. Nature. (2011) 473:174–80. doi: 10.1038/nature09944
59. Liang C, Tseng HC, Chen HM, Wang WC, Chiu CM, Chang JY, et al. Diversity and enterotype in gut bacterial community of adults in Taiwan. BMC Genomics. (2017) 18(Suppl. 1):932. doi: 10.1186/s12864-016-3261-6
60. Maniar K, Singh V, Kumar D, Moideen A, Bhattacharyya R, Banerjee D. Metformin: A candidate drug to control the epidemic of diabetes and obesity by way of gut microbiome modification. In: Faintuch J, Faintuch S, editors. Microbiome and Metabolome in Diagnosis, Therapy, and other Strategic Applications. Cambridge, MA: Academic Press (2019). p. 401–8.
61. Kasubuchi M, Hasegawa S, Hiramatsu T, Ichimura A, Kimura I. Dietary gut microbial metabolites, short-chain fatty acids, and host metabolic regulation. Nutrients. (2015) 7:2839–49. doi: 10.3390/nu7042839
62. Chen W, Zhang S, Wu J, Ye T, Wang S, Wang P, et al. Butyrate-producing bacteria and the gut-heart axis in atherosclerosis. Clin Chim Acta. (2020) 507:236–41. doi: 10.1016/j.cca.2020.04.037
63. Gao F, Lv YW, Long J, Chen JM, He JM, Ruan XZ, et al. Butyrate improves the metabolic disorder and gut microbiome dysbiosis in mice induced by a high-fat diet. Front Pharmacol. (2019) 10:1040. doi: 10.3389/fphar.2019.01040
64. Yadav H, Lee JH, Lloyd J, Walter P, Rane SG. Beneficial metabolic effects of a probiotic via butyrate-induced GLP-1 hormone secretion. J Biol Chem. (2013) 288:25088–97. doi: 10.1074/jbc.M113.452516
65. Freire MO, Dalli J, Serhan CN, Van Dyke TE. Neutrophil resolvin E1 receptor expression and function in type 2 diabetes. J Immunol. (2017) 198:718–28. doi: 10.4049/jimmunol.1601543
66. Weng H, Endo K, Li J, Kito N, Iwai N. Induction of peroxisomes by butyrate-producing probiotics. PLoS ONE. (2015) 10:e0117851. doi: 10.1371/journal.pone.0117851
67. Murugesan S, Nirmalkar K, Hoyo-Vadillo C, Garcia-Espitia M, Ramirez-Sanchez D, Garcia-Mena J. Gut microbiome production of short-chain fatty acids and obesity in children. Eur J Clin Microbiol Infect Dis. (2018) 37:621–5. doi: 10.1007/s10096-017-3143-0
68. Biagi E, Nylund L, Candela M, Ostan R, Bucci L, Pini E, et al. Through ageing, and beyond: gut microbiota and inflammatory status in seniors and centenarians. PLoS ONE. (2010) 5:e10667. doi: 10.1371/annotation/df45912f-d15c-44ab-8312-e7ec0607604d
69. David LA, Maurice CF, Carmody RN, Gootenberg DB, Button JE, Wolfe BE, et al. Diet rapidly and reproducibly alters the human gut microbiome. Nature. (2014) 505:559–63. doi: 10.1038/nature12820
70. Scott KP, Antoine JM, Midtvedt T, van Hemert S. Manipulating the gut microbiota to maintain health and treat disease. Microb Ecol Health Dis. (2015) 26:25877. doi: 10.3402/mehd.v26.25877
71. Willing BP, Dicksved J, Halfvarson J, Andersson AF, Lucio M, Zheng Z, et al. A pyrosequencing study in twins shows that gastrointestinal microbial profiles vary with inflammatory bowel disease phenotypes. Gastroenterology. (2010) 139:1844–54 e1. doi: 10.1053/j.gastro.2010.08.049
72. Niemann B, Rohrbach S, Miller MR, Newby DE, Fuster V, Kovacic JC. Oxidative stress and cardiovascular risk: obesity, diabetes, smoking, and pollution: part 3 of a 3-part series. J Am Coll Cardiol. (2017) 70:230–51. doi: 10.1016/j.jacc.2017.05.043
73. Li F, Ye J, Shao C, Zhong B. Compositional alterations of gut microbiota in nonalcoholic fatty liver disease patients: a systematic review and meta-analysis. Lipids Health Dis. (2021) 20:22. doi: 10.1186/s12944-021-01440-w
74. Boursier J, Mueller O, Barret M, Machado M, Fizanne L, Araujo-Perez F, et al. The severity of nonalcoholic fatty liver disease is associated with gut dysbiosis and shift in the metabolic function of the gut microbiota. Hepatology. (2016) 63:764–75. doi: 10.1002/hep.28356
75. Lee G, You HJ, Bajaj JS, Joo SK, Yu J, Park S, et al. Distinct signatures of gut microbiome and metabolites associated with significant fibrosis in non-obese NAFLD. Nat Commun. (2020) 11:4982. doi: 10.1038/s41467-020-18754-5
76. Huxtable RJ. Physiological actions of taurine. Physiol Rev. (1992) 72:101–63. doi: 10.1152/physrev.1992.72.1.101
77. Lourenco R, Camilo ME. Taurine: a conditionally essential amino acid in humans? An overview in health and disease. Nutr Hosp. (2002) 17:262–70.
78. Xu YJ, Arneja AS, Tappia PS, Dhalla NS. The potential health benefits of taurine in cardiovascular disease. Exp Clin Cardiol. (2008) 13:57–65.
79. Qaradakhi T, Gadanec LK, McSweeney KR, Abraham JR, Apostolopoulos V, Zulli A. The anti-inflammatory effect of taurine on cardiovascular disease. Nutrients. (2020) 12:2847. doi: 10.3390/nu12092847
80. Fang H, Meng F, Piao F, Jin B, Li M, Li W. Effect of taurine on intestinal microbiota and immune cells in Peyer's patches of immunosuppressive mice. Adv Exp Med Biol. (2019) 1155:13–24. doi: 10.1007/978-981-13-8023-5_2
81. Skibska B, Goraca A. The protective effect of lipoic acid on selected cardiovascular diseases caused by age-related oxidative stress. Oxid Med Cell Longev. (2015) 2015:313021. doi: 10.1155/2015/313021
82. Jeng HA, Pan CH, Diawara N, Chang-Chien GP, Lin WY, Huang CT, et al. Polycyclic aromatic hydrocarbon-induced oxidative stress and lipid peroxidation in relation to immunological alteration. Occup Environ Med. (2011) 68:653–8. doi: 10.1136/oem.2010.055020
Keywords: Ruminococcus, CVD risk, obesity, vitamin D, diet
Citation: Lakshmanan AP, Al Zaidan S, Bangarusamy DK, Al-Shamari S, Elhag W and Terranegra A (2022) Increased Relative Abundance of Ruminoccocus Is Associated With Reduced Cardiovascular Risk in an Obese Population. Front. Nutr. 9:849005. doi: 10.3389/fnut.2022.849005
Received: 05 January 2022; Accepted: 22 March 2022;
Published: 28 April 2022.
Edited by:
Laura Bordoni, University of Camerino, ItalyReviewed by:
Joseph F. Pierre, University of Wisconsin-Madison, United StatesSachchida Nand Rai, University of Allahabad, India
Copyright © 2022 Lakshmanan, Al Zaidan, Bangarusamy, Al-Shamari, Elhag and Terranegra. This is an open-access article distributed under the terms of the Creative Commons Attribution License (CC BY). The use, distribution or reproduction in other forums is permitted, provided the original author(s) and the copyright owner(s) are credited and that the original publication in this journal is cited, in accordance with accepted academic practice. No use, distribution or reproduction is permitted which does not comply with these terms.
*Correspondence: Annalisa Terranegra, YXRlcnJhbmVncmFAc2lkcmEub3Jn