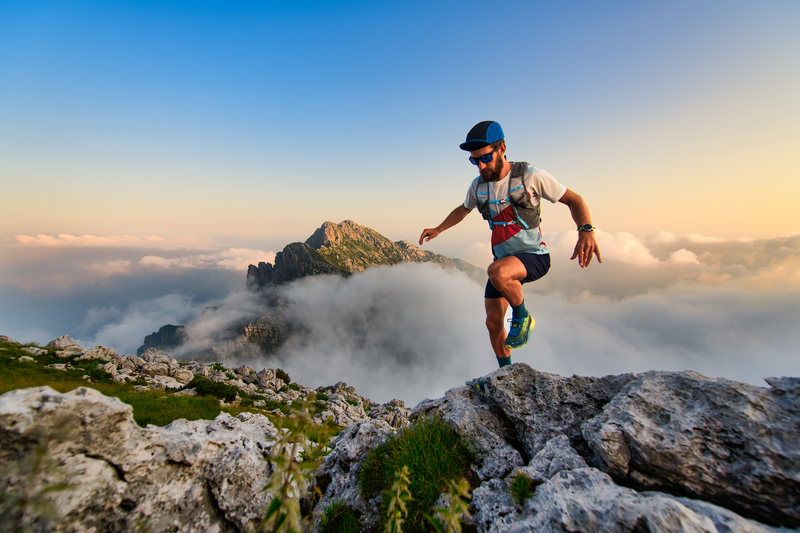
94% of researchers rate our articles as excellent or good
Learn more about the work of our research integrity team to safeguard the quality of each article we publish.
Find out more
ORIGINAL RESEARCH article
Front. Nutr. , 28 June 2022
Sec. Nutrition and Food Science Technology
Volume 9 - 2022 | https://doi.org/10.3389/fnut.2022.845317
This article is part of the Research Topic Advances in Tomato and Tomato Compounds Research and Technology View all 13 articles
Tomato-based products are significant components of vegetable consumption. The processing tomato industry is unquestionably in need of a rapid definition method for measuring soluble solids content (SSC) and lycopene content. The objective was to find the best chemometric method for the estimation of SSC and lycopene content from visible and near-infrared (Vis-NIR) absorbance and reflectance data so that they could be determined without the use of chemicals in the process. A total of 326 Vis-NIR absorbance and reflectance spectra and reference measurements were available to calibrate and validate prediction models. The obtained spectra can be manipulated using different preprocessing methods and multivariate data analysis techniques to develop prediction models for these two main quality attributes of tomato fruits. Eight different method combinations were compared in homogenized and intact fruit samples. For SSC prediction, the results showed that the best root mean squared error of cross-validation (RMSECV) originated from raw absorbance (0.58) data and with multiplicative scatter correction (MSC) (0.59) of intact fruit in Vis-NIR, and first derivatives of reflectance (R2 = 0.41) for homogenate in the short-wave infrared (SWIR) region. The best predictive ability for lycopene content of homogenate in the SWIR range (R2 = 0.47; RMSECV = 17.95 mg kg–1) was slightly lower than that of Vis-NIR (R2 = 0.68; 15.07 mg kg–1). This study reports the suitability of two Vis-NIR spectrometers, absorbance/reflectance spectra, preprocessing methods, and partial least square (PLS) regression to predict SSC and lycopene content of intact tomato fruit and its homogenate.
Tomato (Lycopersicon esculentum Mill.) and tomato-based products are significant components of vegetable consumption. The volume of processed tomatoes in 2020 exceeded 38 million tons in the world (1). From a processing point of view, the two most important quality attributes of tomato fruits are soluble solids content (SSC) and lycopene content (2, 3).
The highest cost of compaction is the energy used to evaporate water from the raw material to concentrate it to 28–38°Brix, which results in a product that can be transported more easily in this form and is eligible for further processing. Thus, when it comes to the SSC level of raw tomato, the higher SSC, the less water has to be evaporated from it, reducing the cost and energy consumption of this operation (4). This means that the processing industry pays extra price for raw tomatoes above a certain level of SSC (5).
In general, the total dry matter (DM) and SSC of the fruit increase as it ripens, in parallel with their pigmentation. The color of red-fruit varieties makes it easy to distinguish whether they are ripe or not (6–8). While SSC can be easily measured by refractometer in°Brix (9), its estimation based only on maturity depends on the cultivar (10). Therefore, it is of great importance to develop a non-destructive method for the accurate estimation of SSC of intact tomato fruits (11–14) or rapid monitoring of their homogenates in analytical laboratory or during the quality check of incoming raw material at the receiving area of processing plant (15, 16).
Lycopene, the main carotenoid component of red ripe tomato fruit, is often considered the main preference of consumers’ acceptance and a major factor for cardiovascular protection in addition to its importance in the reduction of oxidative stress active substances (17, 18).
There are examples for the estimation of lycopene content of red-ripe fruits based on their color (19, 20), but accurate values can only be obtained by expensive and time-consuming laboratory analytics (21–24). There is demand for making this procedure quicker, cheaper, and easier. Measuring spectral reflectance can be a good option for this approach (25–29).
The method is based on the near-infrared (NIR) absorption of the overtones and combination bands of water and organic molecules, mainly O–H, C–H, N–H, and C = O groups. NIR spectra are complex and more difficult to interpret as in other spectral regions like visible (Vis) spectra (30, 31).
Recently, visible-near infrared (Vis-NIR) spectroscopy has been increasingly used in studies for non-destructive determination of ingredients of fruits (32–36) and especially of tomato (22, 23, 37, 38). The rapid determination of SSC in an intact fruit or in a sample homogenized from it is a difficult task due to its high water content (39). A number of usable calibrations have already been made for the rapid determination of SSC and lycopene content in paste from processing tomatoes as the material concentrated to a°Brix value of 28–38 already contains less water (40, 41).
The development of a NIR calibration is a complex task that involves spectral collection using a NIR device, chemometrics, spectra pretreatment, calibration model development, and model validation.
The objective of this study was to evaluate the use of Vis-NIR range of spectra for measuring the SSC and lycopene content of tomato fruit and its homogenate. Choosing the best preprocessing and calibration method for the validation of these important parameters can contribute for developing a non-destructive way, which is applicable to measure these parameters quickly and accurately.
Fruits were produced in open-field experiments of processing tomato in 2019 and 2020. These experiments were carried out at the Experimental Farms of the Hungarian University of Agriculture and Life Sciences (S1), Gödöllő (47°34′N. 19°22′E; elevation 231 m) and Szarvas (S2) (46°53′N. 20°31′E; elevation 81 m) in 2019, and Experimental Farms of the Univer Agro Kft in Szentkirály (S3) (46°54′N. 19°59′E; elevation 91 m) in 2020. Production technology was the same as our previous processing tomato experiments (42–44). There were different processing tomato hybrids from three different seed companies: HeinzSeed (Pomodoro Agro Kft., Mezőberény, Hungary): H1015, H1281, H1307, H1765, H1776, H1879, H1884; BASF Nunhems (Nunhems Hungary Kft., Budapest, Hungary): N6438, NUN283, NUN287, NUN507, NUN812, NUN912, Ussar; and United Genetics (Orosco Kft., Orosháza, Hungary): UG812J, UG1410, UG5202, UG8114, UG13577, UG13579, UG14014, Prestomech. H1015 F1 and UG812J were used in both years only. Samples were formulated from 10 healthy fruits with similar visual appearance in four repetitions of each treatment combinations. The fruits were harvested by hand in red ripe stage in August of both years.
Spectral and analytical measurements were performed with tomato samples right after harvesting. For the intact tomato samples, data are output as reflectance only, by ASD, because Perten is inappropriate for measuring intact fruits as its sample container does not fit intact fruit size. The reflectance and absorbance data were obtained from the laboratory of Regional Knowledge Centre of Hungarian University of Agriculture and Life Sciences (Gödöllő). For the spectral acquisition, the tomato samples were used in two forms, namely, intact tomato fruits (n = 132) and homogenates (n = 192). In the first step, the intact tomato fruits were cleaned before the collection of spectra. Spectral measurements were taken with two instruments, namely, ASD FieldSpec HandHeld 2™ (Analytical Spectral Devices. Inc., Co., United States) Portable Spectroradiometer (spectral range: 325–1,075 nm) and Perten DA7200 (Perten Instruments, Forr-Lab Kft., Budapest, Hungary) NIR analyzer (spectral range: 950–1,650 nm).
A total of 132 spectral samples were directly acquired in the range of 325–1,075 nm from S1 site in 2019 using the ASD spectroradiometer. Fruit samples were derived from irrigation and microbiological treatment combinations of H1015 and UG812J processing tomato hybrids (45).
After measurement of intact fruits, a total of 1,920 tomato fruits were washed, cut, and homogenized for the 192 samples from S2 and S3 sites in 2020. A black Teflon plate (diameter 75 mm) was filled with 26 ± 1 mm of samples, ASD spectroradiometer positioned 2 mm above samples, with Hi-Brite Contact Probe (Analytical Spectral Devices. Inc., Co., United States). Light source of the device was halogen bulb with color temperature 2,900 K, using Zenith Polymer® reference panel made of sintered polytetrafluoroethylene (SphereOptics GmbH, Uhldingen, Germany) for calibration. The spectral scanning was made in five replicates. The instrument has a spectral resolution of < 3.0 nm at 700 nm and wavelength accuracy of ± 1 nm. The black plate perfectly fit into the Perten DA7200 rotation cup, the instrument was worked in the 950–1,650 nm spectral range, and the spectral resolution was 5 nm. For further spectral analysis, an average of five reflectance and absorbance recordings from each sample was used.
Mettler-Toledo Easy R40 refractometer (Mettler Toledo Kft., Budapest, Hungary) was used to measure the SSC of homogenized tomato samples in each replicate (10 fruits) with temperature control on 20°C (46). Its integrated Peltier temperature control quickly heats up or cools down the measurement cell, maintaining the sample reliably at the desired temperature. The tomato homogenate was filtered with gauze and dripped on the measuring cell. An average of two measurements from each repetition of samples were used for the models.
The sample was made from homogenization of 10 fruits. The sample preparation was conducted according to Daood et al. (47). Hitachi Chromaster HPLC (VWR International Kft., Debrecen, Hungary) using a Model 5110 Pump, a Model 5430 Diode Array Detector, and a Model 5210 auto-sampler. The separation and data processing were operated using the EZChrom Elite software (Agilent Technologies, Inc., Santa Clara, CA, United States). Carotenoids were detected between 190 and 700 nm. Separation of carotenoids was performed on a core C-30, 150 × 4.6 mm, 2.6 μm (Thermo Scientific, Waltham, MA, United States) column with a gradient elution of (A) tert-butyl-methyl ether in (B) 2% water in methanol (48). The gradient started with 3% A in B, changed to 35% A in B in 20 min, steady isocratic for 5 min, and finally turned to 3% A in B in 5 min. The flow rate was 0.6 ml min–1. For quantification, the area of each compound was recorded at the maximum absorbance wavelength. Concentration of carotenoids was calculated as 8-apo-carotenal equivalent. The internal standard was set at a known concentration to the samples. Standard material for lycopene (Sigma-Aldrich, Budapest, Hungary) was also used, as an external standard, for its identification and quantitation.
The spectral data were analyzed using the Unscrambler 11.0 software (CAMO Analytics AS., Oslo, Norway). Preprocessing of spectral data is often of vital importance if reasonable results are to be obtained whether the analysis is used for exploratory data mining, classification, or building a good robust prediction model (49). The obtained spectra can be manipulated using different preprocessing methods and multivariate data analysis techniques to develop prediction models for these two main quality attributes of tomato fruits. Three preprocessing methods were used to improve the quality of original spectra, multiplicative scatter correction (MSC), standard normal variate (SNV), Savitzky-Golay based on first derivative (1DER) as in previous studies (32, 50, 51), and their combinations with reflectance and absorbance spectra. Partial least-square regression (PLSR) was used to develop calibration models between spectral data and SSC or lycopene content of tomatoes. Eight different method combinations were compared in fruit and homogenized samples (Table 1). The calibration set was 75%, and the validation set was 25% of the total samples. The correct number of regression factors for the PLSR model was judged by root mean square error of cross-validation (RMSECV) obtained by 10-fold cross-validation.
Table 1. Predictive capability of calibration models for SSC and lycopene content of tomato samples by ASD and Perten spectrometers.
Reflectance data were obtained from intact tomato fruits (n = 132) by ASD only, while from homogenized samples (n = 192) reflectance and absorbance were measured by ASD and Perten, respectively. One of the four replicates of each individual tomato groups was selected to represent the entire population for validation.
Figure 1 shows the visual representation of reflectance spectra of all intact tomato fruit samples obtained by ASD HH2 device. The profiles present broad but identifiable bands, ascribable to the contributions of the main constituents of the food matrix such as water and sugar. Reflectance value is below 0.1 from 400 to 575 nm as previously detected by ElMasry and Sun (52), including an intense absorption peak between 450 and 475 nm as found by Ciaccheri et al. (53). Above 560 nm, reflectance values rose sharply because of the red coloration of ripened fruits (54). The variability of spectra was the highest in the Vis-NIR region between 650 and 930 nm. The reflectance maximum was measured between 700 and 705 nm, as found by Clément et al. (52). In the NIR, there was a local absorption maximum at 976 nm.
Figure 1. Average reflectance spectra of intact tomato fruit samples for calibration and validation in Vis-NIR by ASD; vertical bars represent the standard deviation (calibration n = 99; validation n = 33).
Figure 2 shows average reflectance spectra of all tomato homogenate samples for calibration and validation dataset in the range 375–1,075 nm. The results showed some absorbance peaks due to the vibration of O–H, C–H, and N–H bonds, which are related to inner fruit compositions such as sugars and acids. The absorption in the visible spectra is due to the fruit pigments such as chlorophyll, β-carotene, and lycopene. The highest bands in the VIS region (peaks at 550 and 607 nm) are because of the absorption of the chlorophyll, β-carotene, and lycopene similar to the results described previously (55). Yellow (570–590 nm), orange (590–620 nm), and red (620–750 nm) regions of reflectance spectra correlated well with tomato fruit color (56).
Figure 2. Average reflectance spectra of homogenized tomato fruit samples for calibration and validation in Vis-NIR by ASD; vertical bars represent the standard deviation (calibration n = 144; validation n = 48).
The average absorbance spectra of the homogenized tomato fruit samples in the SWIR region measured by Perten can be seen in Figure 3. The highest bands (peaks at 1,095 nm) were in the SWIR region, which are due to the C–H, O–H, and N–H bonds (30, 57). The typical absorption bands related to the high water content of tomato samples can be seen around 950 and 1,450 nm, and a sugar-related peak appears also in the spectrum around 1,100 nm. Similar absorption bands were reported in the studies of tomato fruits and nectarines (58).
Figure 3. Average absorbance spectra of homogenized tomato fruit samples for calibration and validation in SWIR by Perten; vertical bars represent the standard deviation (calibration n = 144; validation n = 48).
To produce good quality paste of tomatoes, they need to be harvested at red ripe stage of fruits, with the highest possible DM content. Immediately after the spectra were measured, SSC measurements were performed using homogenized fruit samples. The samples were then frozen as the high-performance liquid chromatography (HPLC) capacity for lycopene determination did not allow simultaneous measurement of all samples. To make our models as general as possible, 25 varieties harvested over 2 years from three regions of Hungary were included in the samples.
Tomato fruit SSC is the first and lycopene content is the second most important quality attribute for the processing industry. For both properties, the distributions of the reference values in the calibration and validation set were comparable. The sequences of validation samples were designed to represent the characteristics of the calibration sequences. Samples were selected by genotype, by treatment combination, with three from the four replicates for calibration and one for validation (Figures 4, 5). In the figures, the transparent bars indicate the number of validation samples that contained fewer categories than the calibration samples. Values below 4°Brix have been found in the calibration samples, which would limit the opportunities of profitable processing and imply price reduction for the grower when measured at delivery. Processors expect a high SSC because the lower the water content of the raw material, the lower the cost of concentration.
Figure 4. Distribution of SSC and lycopene content of intact tomato fruit for the calibration (n = 99) and validation (n = 33) sets.
Figure 5. Distribution of SSC and lycopene content of homogenized samples for the calibration (n = 144) and validation (n = 48) sets.
Table 2 represents the average SSC and lycopene content of intact tomato fruits and homogenates used for calibration and validation. The parameters of the sample population selected for calibration and validation only slightly differed. Since the samples of intact and homogenized fruits were from two consecutive years, the higher SSC can be explained by the effect of seasonal variation according to our previous studies (59, 60). The effect of higher temperature on lycopene content is larger and opposite to that of SSC, which may have been caused by extreme high-temperature events in 2020 (59, 61).
Table 2. SSC and lycopene content of intact tomato fruits and homogenized samples in the calibration and validation sets.
Both instruments were used to perform reflectance and absorbance measurements on homogenized samples, but the intact fruits could only be measured with the ASD instrument as they do not fit in the Perten sample tray due to their size.
The results of SSC showed reliable correlation coefficient of cross-validation (R2 = 0.68) originated from raw reflectance of intact fruits and absorbance preprocessed by MSC (R2 = 0.72) with RMSECV 0.58 and 0.59, respectively in Vis-NIR spectra. In this spectral range, the absorbance data gave the smallest error (RMSECV 0.43) for the homogenized samples, which could not be improved by SNV (R2 = 0.58; RMSECV 0.43). The predictive capability of the SWIR spectrum for SSC gave the best results when using the first derivative of reflectance spectra, better than in the Vis-NIR (R2 = 0.61), and with lower error (RMSECV = 0.41).
Figure 6 shows the scatter plot of measured and predicted SSC using Vis-NIR PLSR models in the calibration and validation set of intact fruits by ASD. The best correlation was performed using the absorbance data + MSC spectral transformation of the samples for the determination of SSC agreed with others (32). The statistical parameters of prediction were R2 = 0.7216 and RMSECV = 0.59°Brix.
Figure 6. Calibration (Cal) and validation (Val) set of reference (n = 99) vs. predicted (n = 33) SSC of intact tomato fruits derived from the best PLSR model from absorbance of Vis-NIR spectra with MSC preprocessing.
The correlation coefficient (R2 = 0.6821) and error (RMSECV = 0.58) of the first derivative of absorbance in homogenized fruit samples are only slightly different from the reflectance results (Figure 7), as has been described by others (51). Based on the graphical representations of the SSC calibrations and validations, the reflectance-based SWIR spectrum seems to be a better prediction method for homogenized samples.
Figure 7. Calibration (Cal) and validation (Val) set of reference (n = 99) vs. predicted (n = 33) SSC of tomato homogenates derived from the best PLSR model from reflectance of SWIR spectra with first derivative preprocessing.
Although DM is of major importance in tomato physiological research (62), the DM content of the fruit is closely related to its SSC (63). Therefore, SSC has been widely used in practice for the grading of raw tomatoes as it requires simpler sample preparation, cheaper devices, and less labor (4).
There are several difficulties to estimate SSC of tomato fruits non-destructively by spectral characteristic. Tomato fruits have a low SSC, which makes it more difficult to make a reliable prediction compared to fruits with a higher DM content (64). Especially, NIR-SSC predictions were heavily influenced by the correlation of inner and outer mesocarp SSC, which varied during fruit development (35).
Usually, the range of SSC in tomato fruit from the same cultivar and the same growing condition were relatively limited, so fruits using from different cultivars and production sites to achieve a wide range of fruit SSC are recommended for model development (65, 66). Therefore, we aimed to include more varieties and growing sites in the experiment to analyze a more representative sample population.
The scientific evidence generally agrees that Vis-NIR spectroscopy can be used to assess SSC in intact, thin-skinned fruit and is already being used in commercial practice. It is also expected that models based on transmittance will be more reliable in predicting SSC than models based on reflectance or absorbance as these methods are sensitive to surface reflectance variation (35, 67).
According to PLSR, based on Vis-NIR absorbance spectra, the best lycopene prediction of fruits resulted with MSC. The correlation coefficient of cross-validation was R2 = 0.46 and RMSECV = 39.6 mg kg–1 (Table 1). The best calibration of homogenate absorbance spectra by ASD had a more reliable predictive capability, RMSECV = 15.07 mg kg–1, where the correlation coefficient was R2 = 0.68 (Figure 8).
Figure 8. Calibration (Cal) and validation (Val) set of reference (n = 144) vs. predicted (n = 48) lycopene content of homogenized tomato fruit samples derived from the best PLSR model from absorbance of Vis-NIR spectra.
The predictive ability in the SWIR range (R2 = 0.47; RMSECV = 17.95 mg kg–1) was lower for lycopene than in the Vis-NIR range, as represented in Figure 9.
Figure 9. Calibration (Cal) and validation (Val) set of reference (n = 144) vs. predicted (n = 48) lycopene content of homogenized tomato fruit samples derived from the best PLSR model from absorbance of SWIR spectra with first derivative preprocessing.
The concentration of lycopene is not homogeneous in the fruit of tomatoes, being highest under the skin and much lower in the rest of the fruit (68), and recently bred tomato varieties with high lycopene content require more accurate methods to quantify lycopene content (69).
Lycopene content is well defined by non-destructively measured color values of tomato fruits, which in turn is highly dependent on variety and ripeness (56, 70, 71). VIS reflectance spectra can therefore be readily used to assess the main carotenoid in intact tomato fruit (72). Further investigation of NIR spectra on additional varieties to evaluate carotenoids in intact tomato fruit may help to develop more robust models (72, 73).
Vis-NIR spectroscopy is a rapid tool to assist the industry or the laboratory for the estimation of the quality of raw or homogenized tomato fruits.
The use of multiplicative scattering correction and the first derivative were efficient preprocessing techniques for the validation and resulted in the most accurate estimation models of ingredients in tomato. Calibration models of raw absorbance from Vis-NIR spectra resulted in reliable prediction of intact fruits’ SSC (R2VAL = 0.72), but SWIR spectral instrument produced lower RMSECV (0.41°Brix). Raw absorbance by Vis-NIR spectral range resulted slightly lower RMSECV of homogenate lycopene content (15.07 mg kg–1) comparing model of absorbance with first derivative in SWIR range (17.95 mg kg–1).
Combination of the two techniques (spectral range) could result in a more accurate calibration model for intact berries, which could be used to select raw material before the processing and for monitoring its homogenate in analytical laboratory or during the quality check of incoming raw material at the receiving area of processing plant. More homogeneous samples would result in a more accurate calibration, but this would not be conducive to the wide applicability of these models in practice. Vis-NIR spectroscopy appears to be a rapid and cost-effective technique compared to laboratory analytics, but using raw spectra requires a high level of skill because preprocessing is necessary. Traditional chemometric methods are time-consuming with higher cost and environmental impact, especially for lycopene analytics. The accuracy of the prediction obtained in this study indicates that Vis-NIR spectroscopy offers a useful method for quick and convenient evaluation of quality traits of tomato fruit. Further studies may give perspective to obtain better calibration models involving middle infrared range with more diverse sample population in accurate predictive capability.
The original contributions presented in this study are included in the article/supplementary material, further inquiries can be directed to the corresponding author.
ZP and MÉ: conceptualization and writing—original draft preparation. ST, HD, PS, and GaP: methodology and investigation. MÉ: software, data curation, and visualization. MÉ, ZP, and ST: validation. LH: resources and funding acquisition. ZP and GáP: writing—review and editing. GáP and LH: supervision. All authors have read and agreed to the published version of the manuscript.
This research was supported by the EFOP-3.6.3-VEKOP-16-2017-00008 project. This study was supported by the ÚNKP-21-4 New National Excellence Program of the Ministry for Innovation and Technology from the source of the National Research, Development and Innovation Fund.
GáP, GaP, and PS were employed by Univer Product PLC.
The remaining authors declare that the research was conducted in the absence of any commercial or financial relationships that could be construed as a potential conflict of interest.
All claims expressed in this article are solely those of the authors and do not necessarily represent those of their affiliated organizations, or those of the publisher, the editors and the reviewers. Any product that may be evaluated in this article, or claim that may be made by its manufacturer, is not guaranteed or endorsed by the publisher.
1. World Processing Tomato Council [WPTC]. World Production Estimate of Tomatoes for Processing. (2021). Available online at: https://www.wptc.to/pdf/releases/WPTC%20World%20Production%20estimate%20as%20of%2004-04-2022.pdf (accessed June 17, 2022).
2. Rocco CD, Morabito R. Robust optimisation approach applied to the analysis of production/logistics and crop planning in the tomato processing industry. Int J Prod Res. (2016) 54:5842–61. doi: 10.1080/00207543.2016.1181284
3. Martínez-Hernández GB, Boluda-Aguilar M, Taboada-Rodríguez A, Soto-Jover S, Marín-Iniesta F, López-Gómez A. Processing, packaging, and storage of tomato products: influence on the lycopene content. Food Eng Rev. (2016) 8:52–75. doi: 10.1007/s12393-015-9113-3
4. Rocco CD, Morabito R. Production and logistics planning in the tomato processing industry: a conceptual scheme and mathematical model. Comput Electron Agric. (2016) 127:763–74. doi: 10.1016/j.compag.2016.08.002
5. Branthôme F-X. Hungary: Univer Doubles its Processing Capacity. (2017). Available online at: http://www.tomatonews.com/en/hungary-univer-doubles-itsprocessing-capacity_2_128.html (accessed June 17, 2022).
6. Opara UL, Al-Ani MR, Al-Rahbi NM. Effect of fruit ripening stage on physico-chemical properties, nutritional composition and antioxidant components of tomato (Lycopersicum esculentum) cultivars. Food Bioprocess Technol. (2012) 5:3236–43. doi: 10.1007/s11947-011-0693-5
7. Raffo A, Leonardi C, Fogliano V, Ambrosino P, Salucci M, Gennaro L, et al. Nutritional value of cherry tomatoes (Lycopersicon esculentum cv. Naomi F1) harvested at different ripening stages. J Agric Food Chem. (2002) 50:6550–6. doi: 10.1021/jf020315t
8. Nour V, Ionica ME, Trandafir I. Bioactive compounds, antioxidant activity and color of hydroponic tomato fruits at different stages of ripening. Not Bot Horti Agrobot Cluj Napoca. (2015) 43:404–12. doi: 10.15835/nbha43210081
9. Grandillo S, Zamir D, Tanksley SD. Genetic improvement of processing tomatoes: a 20 years perspective. Euphytica. (1999) 110:85–97. doi: 10.1023/A:1003760015485
10. Baltazar A, Aranda JI, González-Aguilar G. Bayesian classification of ripening stages of tomato fruit using acoustic impact and colorimeter sensor data. Comput Electron Agric. (2008) 60:113–21. doi: 10.1016/j.compag.2007.07.005
11. Wati RK, Pahlawan MFR, Masithoh RE. Development of calibration model for pH content of intact tomatoes using a low-cost Vis/NIR spectroscopy. IOP Conf Ser Earth Environ Sci. (2021) 686:012049. doi: 10.1088/1755-1315/686/1/012049
12. Saad AG, Jaiswal P, Jha SN. Non-destructive quality evaluation of intact tomato using VIS-NIR spectroscopy. Int J Adv Res. (2014) 2:632–9.
13. Rahman A, Kandpal LM, Lohumi S, Kim MS, Lee H, Mo C, et al. Nondestructive estimation of moisture content, pH and soluble solid contents in intact tomatoes using hyperspectral imaging. Appl Sci. (2017) 7:109. doi: 10.3390/app7010109
14. Borba KR, Aykas DP, Milani MI, Colnago LA, Ferreira MD, Rodriguez-Saona LE. Portable near infrared spectroscopy as a tool for fresh tomato quality control analysis in the field. Appl Sci. (2021) 11:3209. doi: 10.3390/app11073209
15. Bureau S, Arbex de Castro Vilas Boas A, Giovinazzo R, Jaillais B, Page D. Toward the implementation of mid-infrared spectroscopy along the processing chain to improve quality of the tomato based products. LWT Food Sci Technol. (2020) 130:109518. doi: 10.1016/j.lwt.2020.109518
16. Kubo MTK, Rojas MI, Miano AC, Augusto PED. Rheological properties of tomato products. In: Porretta S editor. Tomato Chemistry, Industrial Processing and Product Development. London: The Royal Society of Chemistry (2019). p. 3–25. doi: 10.1039/9781788016247-00001
17. Rao VA, Young GL, Rao LG. Lycopene and Tomatoes in Human Nutrition and Health. Boca Raton, FL: CRC Press (2018). p. 204.
18. Jürkenbeck K, Spiller A, Meyerding SGH. Tomato attributes and consumer preferences – a consumer segmentation approach. Br Food J. (2020) 122:328–44. doi: 10.1108/BFJ-09-2018-0628
19. Kim DS, Lee DU, Lim JH, Kim S, Choi JH. Agreement between visual and model-based classification of tomato fruit ripening. Trans ASABE. (2020) 63:667–74. doi: 10.13031/TRANS.13812
20. Petropoulos SA, Fernandes Â, Xyrafis E, Polyzos N, Antoniadis V, Barros L, et al. The optimization of nitrogen fertilization regulates crop performance and quality of processing tomato (Solanum lycopersicum l. cv. heinz 3402). Agronomy. (2020) 10:10050715. doi: 10.3390/agronomy10050715
21. Goisser S, Wittmann S, Fernandes M, Mempel H, Ulrichs C. Comparison of colorimeter and different portable food-scanners for non-destructive prediction of lycopene content in tomato fruit. Postharvest Biol Technol. (2020) 167:111232. doi: 10.1016/j.postharvbio.2020.111232
22. Deák KJ, Szigedi T, Pék Z, Baranowski P, Helyes L. Carotenoid determination in tomato juice using near infrared spectroscopy. Int Agrophys. (2015) 29:275–82. doi: 10.1515/intag-2015-0032
23. Szuvandzsiev P, Helyes L, Lugasi A, Szántó C, Baranowski P, Pék Z. Estimation of antioxidant components of tomato using VIS-NIR reflectance data by handheld portable spectrometer. Int Agrophys. (2014) 28:521–7.
24. Goisser S, Krause J, Fernandes M, Mempel H. Determination of tomato quality attributes using portable NIR-sensors. In: Beyerer J, Puente León F, Längle T editors. Optical Characterization of Materials: Conference Proceedings. Karlsruhe: KIT Scientific Publishing (2019). p. 1–12.
25. Choudhary R, Bowser TJ, Weckler P, Maness NO, McGlynn W. Rapid estimation of lycopene concentration in watermelon and tomato puree by fiber optic visible reflectance spectroscopy. Postharvest Biol Technol. (2009) 52:103–9. doi: 10.1016/j.postharvbio.2008.10.002
26. Ciaccheri L, Tuccio L, Mencaglia AA, Mignani AG, Hallmann E, Sikorska-Zimny K, et al. Directional versus total reflectance spectroscopy for the in situ determination of lycopene in tomato fruits. J Food Compos Anal. (2018) 71:65–71. doi: 10.1016/j.jfca.2018.01.023
27. Deák KJ, Szigedi T, Palotás G, Daood HG, Helyes L. Determination of °Brix, lycopene, β-carotene and total carotenoid content of processing tomatoes using near infrared spectroscopy. Acta Hortic. (2015) 1081:253–8.
28. Ibrahim A, Daood HG, Bori Z, Helyes L. Using infrared spectroscopy for tracking and estimating antioxidant in tomato fruit fractions. Eur J Eng Res Sci. (2018) 3:21–30. doi: 10.24018/ejers.2018.3.5.736
29. Szuvandzsiev P, Daood HG, Posta K, Helyes L, Pék Z. Application of VIS-NIR reflectance spectra for estimating soluble solid and lycopene content of open-field processing tomato fruit juice from irrigation and mycorrhiza treatments. Acta Hortic. (2017) 1159:73–7. doi: 10.17660/ActaHortic.2017.1159.11
30. Eldin AB. Near infrared spectroscopy. In: Akyar I editor. Wide Spectra of Quality Control. Rijeka: InTech Europe (2011). p. 237–48.
31. Osborne BG. Near-infrared spectroscopy in food analysis. In: Meyers RA editor. Encyclopedia of Analytical Chemistry. Hoboken, NJ: John Wiley & Sons Ltd (2006). p. 1–14. doi: 10.1002/9780470027318.a1018
32. De Oliveira GA, Bureau S, Renard CMGC, Pereira-Netto AB, De Castilhos F. Comparison of NIRS approach for prediction of internal quality traits in three fruit species. Food Chem. (2014) 143:223–30. doi: 10.1016/j.foodchem.2013.07.122
33. Anderson NT, Walsh KB, Flynn JR, Walsh JP. Achieving robustness across season, location and cultivar for a NIRS model for intact mango fruit dry matter content. II. Local PLS and nonlinear models. Postharvest Biol Technol. (2021) 171:111358. doi: 10.1016/j.postharvbio.2020.111358
34. Pissard A, Marques EJN, Dardenne P, Lateur M, Pasquini C, Pimentel MF, et al. Evaluation of a handheld ultra-compact NIR spectrometer for rapid and non-destructive determination of apple fruit quality. Postharvest Biol Technol. (2021) 172:1111375. doi: 10.1016/j.postharvbio.2020.111375
35. Walsh KB, Blasco J, Zude-Sasse M, Sun X. Visible-NIR ‘point’ spectroscopy in postharvest fruit and vegetable assessment: the science behind three decades of commercial use. Postharvest Biol Technol. (2020) 168:111246. doi: 10.1016/j.postharvbio.2020.111246
36. Mishra P, Roger JM, Rutledge DN, Woltering E. SPORT pre-processing can improve near-infrared quality prediction models for fresh fruits and agro-materials. Postharvest Biol Technol. (2020) 168:111271. doi: 10.1016/j.postharvbio.2020.111271
37. Torres I, Pérez-Marín D, De la Haba MJ, Sánchez MT. Fast and accurate quality assessment of Raf tomatoes using NIRS technology. Postharvest Biol Technol. (2015) 107:9–15. doi: 10.1016/j.postharvbio.2015.04.004
38. Ibrahim A, Daood H, Friedrich L, Hitka G, Helyes L. Monitoring, by high-performance liquid chromatography, near-infrared spectroscopy, and color measurement, of phytonutrients in tomato juice subjected to thermal processing and high hydrostatic pressure. J Food Process Preserv. (2021) 45:e15370. doi: 10.1111/jfpp.15370
39. Golic M, Walsh K, Lawson P. Short-wavelength near-infrared spectra of sucrose, glucose, and fructose with respect to sugar concentration and temperature. Appl Spectrosc. (2003) 57:139–45. doi: 10.1366/000370203321535033
40. Vitális F, Zaukuu JLZ, Bodor Z, Aouadi B, Hitka G, Kaszab T, et al. Detection and quantification of tomato paste adulteration using conventional and rapid analytical methods. Sensors. (2020) 20:6059. doi: 10.3390/s20216059
41. Zhang L, Schultz MA, Cash R, Barrett DM, McCarthy MJ. Determination of quality parameters of tomato paste using guided microwave spectroscopy. Food Control. (2014) 40:214–23. doi: 10.1016/j.foodcont.2013.12.008
42. Horváth KZ, Andryei B, Helyes L, Pék Z, Neményi A, Nemeskéri E. Effect of mycorrhizal inoculations on physiological traits and bioactive compounds of tomato under water scarcity in field conditions. Not Bot Horti Agrobot Cluj Napoca. (2020) 48:1233–47. doi: 10.15835/nbha48311963
43. Nemeskéri E, Horváth K, Pék Z, Helyes L. Effect of mycorrhizal and bacterial products on the traits related to photosynthesis and fruit quality of tomato under water deficiency conditions. Acta Hortic. (2019) 1233:61–5. doi: 10.17660/ActaHortic.2019.1233.10
44. Nemeskéri E, Neményi A, Bőcs A, Pék Z, Helyes L. Physiological factors and their relationship with the productivity of processing tomato under different water supplies. Water. (2019) 11:586. doi: 10.3390/w11030586
45. Andryei B, Horváth KZ, Duah SA, Takács S, Égei M, Szuvandzsiev P, et al. Use of plant growth promoting rhizobacteria (PGPRs) in the mitigation of water deficiency of tomato plants (Solanum lycopersicum L.) Növekedést serkentõ rhizobaktériumok használata paradicsom növények vízhiányának mérséklésére. J Cent Eur Agric. (2021) 22:167–77.
46. Ayusto-Yuste MC, González-Cebrino F, Lozano-ruiz M, Fernández-León AM, Bernalte-García MJ. Influence of ripening stage on quality parameters of five traditional tomato varieties grown under organic conditions. Horticulturae. (2022) 8:313. doi: 10.3390/horticulturae8040313
47. Daood HG, Bencze G, Palotás G, Pék Z, Sidikov A, Helyes L. HPLC analysis of carotenoids from tomatoes using cross-linked C18 column and MS detection. J Chromatogr Sci. (2014) 52:985–91. doi: 10.1093/chromsci/bmt139
48. Daood HG, Ráth S, Palotás G, Halász G, Hamow K, Helyes L. Efficient HPLC separation on a Core-C30 column with MS2 characterization of isomers, derivatives and unusual carotenoids from tomato products. J Chromatogr Sci. (2021) 60:336–47. doi: 10.1093/chromsci/bmab085
49. Yao H, Lewis D. Spectral preprocessing and calibration techniques. In: Sun DW editor. Hyperspectral Imaging for Food Quality Analysis and Control. Cambridge, MA: Academic Press (2010). p. 45–78. doi: 10.1016/B978-0-12-374753-2.10002-4
50. Pedro AMK, Ferreira MMC. Nondestructive determination of solids and carotenoids in tomato products by near-infrared spectroscopy and multivariate calibration. Anal Chem. (2005) 77:2505–11. doi: 10.1021/ac048651r
51. Ścibisz I, Reich M, Bureau S, Gouble B, Causse M, Bertrand D, et al. Mid-infrared spectroscopy as a tool for rapid determination of internal quality parameters in tomato. Food Chem. (2011) 125:1390–7. doi: 10.1016/j.foodchem.2010.10.012
52. Clément A, Bacon R, Sirois S, Dorais M. Mature-ripe tomato spectral classification according to lycopene content and fruit type by visible, NIR reflectance and intrinsic fluorescence. Qual Assur Saf Crop Foods. (2015) 7:747–56. doi: 10.3920/QAS2014.0521
53. Ciaccheri L, Tuccio L, Mencaglia AA, Sikorska-Zimny K, Hallmann E, Kowalski A, et al. Prediction models for assessing lycopene in open-field cultivated tomatoes by means of a portable reflectance sensor: cultivar and growing-season effects. J Agric Food Chem. (2018) 66:4748–57. doi: 10.1021/acs.jafc.8b01570
54. Panjai L, Röhlen-Schmittgen S, Ellenberger J, Noga G, Hunsche M, Fiebig A. Effect of postharvest irradiation with red light on epidermal color and carotenoid concentration in different parts of tomatoes. J Food Meas Charact. (2021) 15:1737–46. doi: 10.1007/s11694-020-00770-0
55. Najjar K, Abu-Khalaf N. Non-destructive quality measurement for three varieties of tomato using VIS/NIR spectroscopy. Sustainability. (2021) 13:10747. doi: 10.3390/su131910747
56. Stinco CM, Rodríguez-Pulido FJ, Luisa E-GM, Gordillo B, Vicario IM, Meléndez-Martínez AJ. Lycopene isomers in fresh and processed tomato products: correlations with instrumental color measurements by digital image analysis and spectroradiometry. Food Res Int. (2013) 50:111–20.
57. Huang Y, Lu R, Chen K. Assessment of tomato soluble solids content and pH by spatially-resolved and conventional Vis/NIR spectroscopy. J Food Eng. (2018) 236:19–28. doi: 10.1016/j.jfoodeng.2018.05.008
58. Flores K, Sánchez MT, Pérez-Marín D, Guerrero JE, Garrido-Varo A. Feasibility in NIRS instruments for predicting internal quality in intact tomato. J Food Eng. (2009) 91:311–8. doi: 10.1016/j.jfoodeng.2008.09.013
59. Takács S, Pék Z, Csányi D, Daood HG, Szuvandzsiev P, Palotás G, et al. Influence of water stress levels on the yield and lycopene content of tomato. Water. (2020) 12:2165. doi: 10.3390/W12082165
60. Pék Z, Szuvandzsiev P, Neményi A, Tuan LA, Bakr J, Nemeskéri E, et al. Comparison of a water supply model with six seasons of cherry type processing tomato. Acta Hortic. (2019) 1233:41–6. doi: 10.17660/ActaHortic.2019.1233.7
61. Helyes L, Lugasi A, Pék Z. Effect of natural light on surface temperature and lycopene content of vine ripened tomato fruit. Can J Plant Sci. (2007) 87:927–9.
62. Heuvelink E, Okello RCO, Giovannoni JJ, Dorais M. Tomato. In: Wien HC, Stützel H editors. The Physiology of Vegetable Crops. Wallingford: CAB International (2020). p. 138–78.
63. Khuriyati N, Matsuoka T. Monitoring NIR internal spectroscopy properties soilless of on-plant culture tomato fruits in using for control of nutrient solution among the quality parameters considered for tomato fruits, soluble solids content (SSC) is the most important component. Environ Control Biol. (2005) 43:39–46.
64. Sohaib ASS, Zeb A, Qureshi WS, Arslan M, Ullah MA, Alasmary W, et al. Towards fruit maturity estimation using NIR spectroscopy. Infrared Phys Technol. (2020) 111:103479. doi: 10.1016/j.infrared.2020.103479
65. Acharya UK, Subedi PP, Walsh KB. Robustness of tomato quality evaluation using a portable Vis-SWNIRS for dry matter and colour. Int J Anal Chem. (2017) 2017:2863454. doi: 10.1155/2017/2863454
66. Brito AA, Campos F, Nascimento A dos R, Corrêa G, de C, Silva FA, et al. Determination of soluble solid content in market tomatoes using near-infrared spectroscopy. Food Control. (2021) 126:108068. doi: 10.1016/j.foodcont.2021.108068
67. Wang H, Zhang R, Peng Z, Jiang Y, Ma B. Measurement of SSC in processing tomatoes (Lycopersicon esculentum Mill.) by applying Vis-NIR hyperspectral transmittance imaging and multi-parameter compensation models. J Food Process Eng. (2019) 42:e13100. doi: 10.1111/jfpe.13100
68. Toor RK, Savage GP. Antioxidant activity in different fractions of tomatoes. Food Res Int. (2005) 38:487–94.
69. Ilahy R, Siddiqui MW, Piro G, Lenucci MS, Hdider C, Helyes L. A focus on high-lycopene tomato cultivars: horticultural performance and functional quality. Acta Hortic. (2017) 1159:57–64. doi: 10.17660/ActaHortic.2017.1159.9
70. Brandt S, Pék Z, Barna É, Lugasi A, Helyes L. Lycopene content and colour of ripening tomatoes as affected by environmental conditions. J Sci Food Agric. (2006) 86:568–72. doi: 10.1002/jsfa.2390
71. Ilahy R, Tlili I, Siddiqui MW, Hdider C, Lenucci MS. Inside and beyond color: comparative overview of functional quality of tomato and watermelon fruits. Front Plant Sci. (2019) 10:769. doi: 10.3389/fpls.2019.00769
72. Tilahun S, Park DS, Seo MH, Hwang IG, Kim SH, Choi HR, et al. Prediction of lycopene and β-carotene in tomatoes by portable chroma-meter and VIS/NIR spectra. Postharvest Biol Technol. (2018) 136:50–6. doi: 10.1016/j.postharvbio.2017.10.007
Keywords: tomato, Vis-NIR, spectroscopy, SSC, lycopene, absorbance, reflectance, preprocessing
Citation: Égei M, Takács S, Palotás G, Palotás G, Szuvandzsiev P, Daood HG, Helyes L and Pék Z (2022) Prediction of Soluble Solids and Lycopene Content of Processing Tomato Cultivars by Vis-NIR Spectroscopy. Front. Nutr. 9:845317. doi: 10.3389/fnut.2022.845317
Received: 29 December 2021; Accepted: 02 June 2022;
Published: 28 June 2022.
Edited by:
Spyridon Alexandros Petropoulos, University of Thessaly, GreeceReviewed by:
Rajko Vidrih, University of Ljubljana, SloveniaCopyright © 2022 Égei, Takács, Palotás, Palotás, Szuvandzsiev, Daood, Helyes and Pék. This is an open-access article distributed under the terms of the Creative Commons Attribution License (CC BY). The use, distribution or reproduction in other forums is permitted, provided the original author(s) and the copyright owner(s) are credited and that the original publication in this journal is cited, in accordance with accepted academic practice. No use, distribution or reproduction is permitted which does not comply with these terms.
*Correspondence: Zoltán Pék, cGVrLnpvbHRhbkB1bmktbWF0ZS5odQ==
Disclaimer: All claims expressed in this article are solely those of the authors and do not necessarily represent those of their affiliated organizations, or those of the publisher, the editors and the reviewers. Any product that may be evaluated in this article or claim that may be made by its manufacturer is not guaranteed or endorsed by the publisher.
Research integrity at Frontiers
Learn more about the work of our research integrity team to safeguard the quality of each article we publish.