- 1Shenzhen Key Laboratory of Marine Bioresources and Ecology, Brain Disease and Big Data Research Institute, College of Life Sciences and Oceanography, Shenzhen University, Shenzhen, China
- 2Shenzhen-Hong Kong Institute of Brain Science-Shenzhen Fundamental Research Institutions, Shenzhen, China
- 3Affiliated Hangzhou Xixi Hospital, Zhejiang University School of Medicine, Hangzhou, China
Trace elements and minerals play a significant role in human health and diseases. In recent years, ionomics has been rapidly and widely applied to explore the distribution, regulation, and crosstalk of different elements in various physiological and pathological processes. On the basis of multi-elemental analytical techniques and bioinformatics methods, it is possible to elucidate the relationship between the metabolism and homeostasis of diverse elements and common diseases. The current review aims to provide an overview of recent advances in the application of ionomics in metabolic disease research. We mainly focuses on the studies about ionomic or multi-elemental profiling of different biological samples for several major types of metabolic diseases, such as diabetes mellitus, obesity, and metabolic syndrome, which reveal distinct and dynamic patterns of ion contents and their potential benefits in the detection and prognosis of these illnesses. Accumulation of copper, selenium, and environmental toxic metals as well as deficiency of zinc and magnesium appear to be the most significant risk factors for the majority of metabolic diseases, suggesting that imbalance of these elements may be involved in the pathogenesis of these diseases. Moreover, each type of metabolic diseases has shown a relatively unique distribution of ions in biofluids and hair/nails from patients, which might serve as potential indicators for the respective disease. Overall, ionomics not only improves our understanding of the association between elemental dyshomeostasis and the development of metabolic disease but also assists in the identification of new potential diagnostic and prognostic markers in translational medicine.
1. Introduction
Metabolic diseases are well-known as a major threat to global public health, which include diabetes mellitus (DM), obesity, metabolic syndrome (MetS), non-alcoholic fatty liver disease (NAFLD), hyperlipidemia, hyperuricemia (or gout), bone metabolic disease (such as osteoporosis and osteomalacia), and some other diseases that are caused by endocrine dysfunction (e.g., thyroid diseases) or nutrient imbalance (such as Wilson's disease) (1, 2). In the past several decades, much effort has been made to understand the underlying mechanisms and to develop rational treatments for these diseases and disorders. However, due to the complex pathogenesis and characteristics of most metabolic diseases, the progress still remains quite limited. Thus, effective strategies of early diagnosis and treatment of metabolic diseases are urgently needed.
Among known risk factors and possible molecular mechanisms associated with metabolic diseases, dyshomeostasis of various elements has been implicated in the onset and progression of many types of them (3–6). Except for a small number of elements that are required in large amounts (called macroelements or macronutrients), such as potassium (K), sodium (Na), calcium (Ca), and magnesium (Mg), the majority of them are trace elements. Biological trace elements (or micronutrients, mostly metals) are needed in small quantities but are essential for growth, development, and various physiological processes of all living organisms, which include iron (Fe), zinc (Zn), copper (Cu), manganese (Mn), molybdenum (Mo), nickel (Ni), cobalt (Co), chromium (Cr), vanadium (V), selenium (Se), iodine (I), and several other elements (7, 8). They serve as critical cofactors for the assembly of metalloproteins, which participate in a very wide range of biological processes such as enzymatic reaction, cellular signaling transduction, redox regulation, mitochondrial function, immunological response, and hormone and vitamin biosynthesis (9, 10). In addition, several environmental toxic elements, such as cadmium (Cd), mercury (Hg), arsenic (As), lead (Pb), and aluminum (Al), have detrimental effects on human health (11, 12).
It has been known that the cell has developed complex regulatory mechanisms (such as uptake, excretion, and storage) to tightly control the metabolism and homeostasis of various trace elements in order to ensure appropriate supply while preventing accumulation to toxic levels (13). Previously, a large number of studies have reported that deficiency or overload of certain trace elements are related to a variety of metabolic diseases and disorders (14–18). For example, Fe deficiency is significantly associated with obesity, whereas excessive Fe accumulation in adipose tissue and liver is often seen in type 2 diabetes mellitus (T2DM) and NAFLD (14, 15). Furthermore, some elements may interact and interfere with the absorption, bioavailability, and even normal functions of others (19–21). On the other hand, trace element levels may be altered in various clinical conditions, which may be associated with the prognosis and efficacy of the treatment (22). Although numerous studies have been performed to evaluate the status of single or several elements in biological samples of different types of metabolic diseases, it is still unclear how disruption of the balance of various elements are involved in the development and progression of these diseases. A more systematic understanding of the metabolism and homeostasis of trace elements and their relationships with metabolic diseases is urgently needed.
In the recent 20 years, with the explosive growth of multi-omics data (such as genome, transcriptome, and proteome) and a corresponding increase in data analysis methods, the complex relationship between trace elements and human health or diseases has been examined comprehensively. The concept of metallome (the ensemble of all metal ions in an organism) and its extension ionome (representing the composition of all metals, metalloids, and non-metals found in a living system) have also been introduced (23, 24). Ionomics, the study of the ionome, involves simultaneous and quantitative analyses of elemental composition in living systems (such as cells, tissues, and organs) and changes in this composition under different physiological and pathological conditions by using high-throughput elemental profiling techniques (24–26). This approach has been extensively applied in plants, yeast, and most recently mammals (including humans), which provides a very useful platform for not only identifying new characteristics and components of trace element metabolism and homeostasis but also gaining information about how the complex ionomic network is affected by different factors and states (26–28). In addition, isotopic and speciation analysis of elements is becoming an inherent or complementary part of ionomics, which is important for a more accurate assessment of the status of elements present in biological samples (29, 30). In recent years, ionomics approach has been applied to study the global effect of a large amount of elements for various human diseases, which offers a great opportunity to discover new ion-based mechanisms of these diseases (especially complex disease) and to enable the possibility to develop new diagnostic tools and therapeutic strategies (31, 32).
In this review, we present a comprehensive survey on the complex relationship between ionomic profiles in diverse human biological samples and several major types of metabolic diseases mainly based on recent large-scale ionomic/metallomic studies, such as case-control studies, cohort- and population-based studies, and meta-analyses. Such information may provide a holistic and integrated understanding of the critical roles trace elements play in metabolic diseases.
2. Ionomics technology and its application in human health and diseases
The major purpose of ionomics is to measure the ion contents in a biological system and to elucidate their correlations to phenotypes of interest. Nowadays ionomics has been widely used for studies on environmental monitoring, nutrient utilization, biofortification, food safety, and forward and reverse genetics, particularly in plant developmental and stress biology which has been extensively reviewed in the literature (23–25, 33–35). In addition, the rapid expansion of ionomics in human health and diseases has provided new evidence for the relationship between trace elements and a variety of common diseases, which may be helpful for the early detection of disease and the innovation of new drugs against imbalanced elements.
2.1. Techniques for elemental quantification and related data resources
In early times, elemental profiling analysis in various samples was mainly used in geoscience, environmental science, and ecological studies. In the last decade, ionomics has grown in popularity in different areas of life science as a powerful tool for studying the metabolism and homeostasis of diverse trace elements and minerals in different organisms, including plants and humans. The major techniques for the quantification of chemical elements include inductively coupled plasma mass spectrometry (ICP-MS), inductively coupled plasma atomic/optical emission spectroscopy (ICP-AES/ICP-OES), X-ray fluorescence (XRF), atomic absorption spectrometry (AAS), neutron activation analysis (NAA), and several other methods (23, 33). Currently, ICP-MS and ICP-AES are the two most widely used high-throughput analytical tools. ICP-MS has been the dominant technique for the analysis of multi-elemental concentrations due to its high accuracy, high sensitivity, wide measurement capability, and the ability for isotopic analysis (36). Although ICP-AES/ICP-OES is less sensitive than ICP-MS, it offers the advantages of lower cost and simplicity of operation (37). Both methods have been used for ionomics studies in yeast, plants, and animals, which demonstrate the potential benefits of ionomics in the exploration of new aspects of trace elements in biology and medicine (26, 27, 38–40). Other techniques, although used less frequently, may provide alternative approaches for determining various elements from different samples (41, 42).
With the increase in volume of data generated by ionomics studies, it becomes more and more important to develop appropriate information management systems for ionomics data acquisition, storage, retrieval, and analysis. However, publicly accessible platforms and databases that allow researchers to obtain such resources are very rare so far. The iHUB system is the first integrated workflow system, which contains ionomics data of yeast and important plants such as Arabidopsis thaliana, maize, rice, and soybean, and provides open access for raw data management, data mining and analysis, and knowledge discovery (43). The OPTIMAS Data Warehouse (OPTIMAS-DW) is a comprehensive and integrated data resource for maize, which contains ionomics and other omics data such as transcriptome, proteome, metabolome, and phenome, and allows users to extract information of particular interest (44). Unfortunately, an ionomics database associated with human disease is still absent.
On the other hand, with the rapid progress in the experimental and computational identification of novel trace element-related genes and their functions, relevant information should be well-maintained for further utilization. A known ionome gene (KIG) database was recently developed, which is a curated list of genes known to affect uptake, accumulation, and distribution of elements in several plant species (45). New systems and annotation tools are clearly required to obtain a better understanding of the function and regulation of genes affecting the ionome on a genome-wide scale.
2.2. Bioinformatics for ionomics data analysis
The recent explosion of ionomics data has prompted the development of computational strategies and methods for investigation of the associations between ionomic profiles and complex traits. Bioinformatics approaches for ionomics data analysis include both basic statistical methods (such as statistical significance testing and analysis of variance) and advanced multivariate statistical methods (e.g., principal component analysis and logistic regression analysis), which could also be applied for other types of omics data (31). Moreover, network-based systems biology approaches and machine learning algorithms have been used to clarify the dynamic interplay between elements under different physiological or metabolic conditions and to build ionome-based prediction models or markers for a variety of diseases (46–49). Very recently, an integrated R-based tool called IonFlow was developed for the analysis of ionomics data sets, which may help researchers to process, explore, and interpret their data via multiple approaches (50).
2.3. Application of ionomics in human disease
Before the concept of ionomics was introduced, ICP-MS had been used to evaluate the concentrations of certain trace elements in biofluids, hair, nails, and some other human tissues for different diseases such as cancer, neurodegenerative diseases, and coronary heart disease, demonstrating that imbalance of trace elements may be one of the risk factors of many complex or common diseases (51–53). Most of these preliminary studies only focused on single or a very limited number of trace elements and lacked deep analysis of the interactions between elements. In recent years, ionome-based studies have been performed for a variety of complex diseases, which may assist in diagnosis and prognosis evaluation of disease. Considering that most ionomics results have been derived from cross-sectional or case-control studies, it is uncertain whether disrupted ion homeostasis is a potential cause of these diseases. Large-scale prospective, longitudinal, and interventional studies are necessary to solve these questions. In addition, the intrinsic limitation of current elemental analytical techniques and the validity and reliability of using ionomic information in the prediction of diseases imply that there is still a long way ahead of us before ionomics can be widely used in medical clinics. However, the application of ionomics in several types of diseases has provided evidence for its potential benefits, which suggests significant correlations between trace elements and the development of different diseases and may help early detection and improve therapeutic regimens for patients in the future.
3. Recent progress in ionomics of metabolic diseases
It has been known for a long time that trace elements and minerals play a critical role in the onset and progression of metabolic diseases (32, 54, 55). Previously, lots of studies have been conducted to explore the linkages between single or multiple elements and various metabolic diseases, such as the association between intake of certain elements and the incidence of DM and osteoporosis and the implication of heavy metal exposure in the epidemiology of metabolic diseases (56–58). In recent years, ionomics approaches have been used to examine the variations of elemental profiles in different body fluids and tissues from patients with metabolic diseases, providing new clues for not only better understanding of the relationship between trace element dyshomeostasis and these diseases but also the development of novel biomarkers for detection, diagnosis, and prognosis of them. In the following text, we will discuss recent progress on ionomics studies of several major types of metabolic diseases. A summary of the main ionomics results obtained from the literature is given in Table 1.
3.1. Diabetes mellitus
DM comprises a group of metabolic diseases characterized by chronic hyperglycemia resulting from insufficient insulin secretion and/or insulin resistance in target tissues, which may lead to multi-organ dysfunction and failure (59). There are three main types of DM: type 1 DM (T1DM), T2DM, and gestational DM (GDM). T2DM accounts for more than 90% of all diabetic cases, which has become the most prevalent metabolic disease worldwide. It has been suggested that ion homeostasis may play an important role in T2DM and some other types of DM (3).
To date, the ionomes in blood (including whole blood, plasma, and serum), urine, hair, and even teardrops have been examined in different cohorts of patients with T2DM, implying that changes in elemental profiles represent a potential source of biomarkers of this complex disease (60–70). The majority of significantly changed elements in different biofluids (especially in the blood samples) are positively associated with T2DM, including several heavy metals (such as Cu and Cd) and Se whose accumulation in the body may be a high risk for T2DM. In contrast, concentrations of Zn and Mg in the blood and hair/nail samples were observed to generally have a negative association with the prevalence of T2DM. These trends are consistent with previous observations that environmental heavy metals and Se might affect insulin sensitivity and normal insulin regulatory function and cause pathoglycemia or even more serious illness such as T2DM, whereas deficiency of Zn, an essential trace metal for synthesis and secretion of insulin, may have a major impact on the pathogenesis of T2DM (3, 64, 67). Thus, such variations could be used for differentiating patients with T2DM from healthy controls. Moreover, significant correlations (|correlation coefficient| > 0.3 and p < 0.05) between elements and increased Cu/Zn ratio were also reported, suggesting possible benefits of the use of such information in detection and prognosis evaluation of T2DM (61, 63). By using machine learning algorithms [such as discriminate analysis, support vector machine (SVM), and random forest], several new approaches and models for the early detection of T2DM based on multi-elemental contents have been developed, which exhibit good prediction performance and may serve as a valuable tool of its diagnosis (66, 70–72). Very recently, the blood levels of several essential trace elements (such as V, Cr, Mn, and Se) were found to be negatively correlated with hemoglobin A1c (HbA1c) in diabetic subjects, especially in those with HbA1c ≥ 7.0%, suggesting mutual effects between them and metabolic abnormalities of blood glucose during the onset and progression of T2DM (69). On the other hand, although a significant number of studies only examined the concentrations of single or a small set of trace elements in biofluids of T2DM patients, similar or more complex patterns of changes were obtained for some of them in different regions of the world (73–80). For example, serum Cu, Zn, and Se levels were found to vary with the effect of glycemic control in T2DM patients, indicating a potential relationship between their homeostasis and the prognosis and treatment of T2DM (73). Long-term Ni and As exposures were also observed to be associated with elevated prevalence of T2DM in Chinese elderly and Mexican women, respectively, implying the harmfulness of more heavy metal contamination in the pathogenesis of T2DM (74, 75). Although González de Vega et al. reported lower plasma concentrations of total Se found in Spanish patients with T2DM which might be due to decreased levels of selenoprotein P (76), the majority of other studies on Se and DM suggest that Se may increase the risk of T2DM across a wide range of exposure levels (77). In addition, several recent ionomics studies have found that trace element imbalance is involved in the development of diabetic complications. Marín-Martínez et al. reported that decreased Co level in saliva and increased strontium (Sr) level in plasma were associated with the presence of chronic complications (both microvascular and macrovascular) in patients with T2DM, which provides some useful enlightenment for predicting diabetic complications (81). Other ionomics studies revealed that increased Sr and decreased Zn and Se levels in plasma have a significant association with incident cardiovascular disease (CVD) risk in patients with T2DM, whereas decreased Mg and Cr levels in blood might be involved in the progression of microvascular complications (such as diabetic retinopathy and diabetic nephropathy) (82, 83).
Ionomics studies have also been conducted for several other types of DM (84–87). One Italian group measured the blood levels of multiple trace elements in patients with T1DM and T2DM from Sardinia, and observed similar alternations of significantly changed elements between them (Cr, Mn, and Ni deficiency) in that area (84). Similar to T2DM, elevated serum level of Cu and lower levels of Zn and/or Mg were also found in subjects with T1DM from different regions, suggesting that perturbation in the balance of these essential metals may be related to the pathology of both types of DM (80, 85). Peruzzu et al. reported that whole blood levels of Cr and Cu were strongly correlated with glycemic control whereas Zn, Fe, and Se were associated with lipid metabolism in patients with T1DM, suggesting different effects of these elements on metabolic control in this disease (86). With regard to GDM, Roverso et al. carried out the first ionomics study of the placentas from women affected by GDM, and showed that a decreased level of Cd and increased level of Se might be linked to the molecular pathways of GDM (87). They further measured the ionomes of placenta, maternal whole blood, and umbilical cord blood samples from a larger cohort of GDM and normal pregnant women, which revealed that a number of elements detected in the umbilical cord blood of fetuses were significantly associated with the occurrence of GDM (such as increased Cu and decreased Fe levels) (88). This may indicate that elemental profiles in the cord blood provide more evidence for understanding the biochemical processes occurring during GDM. Moreover, several recent case-control and cohort studies as well as meta-analyses reported that exposure to toxic heavy metals [such as Ni, antimony (Sb), Cd, and Cu] and insufficient Zn content in the body in early pregnancy are associated with an increased risk of GDM, either individually or as a metal mixture (89–96). Overall, these studies demonstrate high complexity and tissue specificity of the ionomes in patients with different types of DM. However, elevated Cu and Se concentrations, decreased Zn concentrations, and increased Cu/Zn ratio in various biofluid samples might be one of the common risk factors for DM. Further effort is needed to investigate the molecular mechanisms underlying dyshomeostasis of trace elements in patients with different types of DM.
3.2. Obesity
Obesity is a chronic and complex disease characterized by excessive amounts of fat within adipocytes, which may result from insulin resistance, dysregulation in lipid and other metabolic balances, and inflammatory and/or hormonal processes (97). It may increase the risk of other diseases and health problems, such as T2DM, hypertension, CVD, and certain cancers. Both genetic susceptibility and environmental factors contribute significantly to the development of obesity (98). Among them, trace element and mineral dyshomeostasis has already been known to be associated with the pathogenesis of this disease (99). On the other hand, obese patients may be at a greater risk of developing imbalance of trace elements.
Before the use of ionomics in obesity research, many studies have shown a possible relationship between obesity and disturbance of homeostasis of certain trace elements, such as Zn or Fe deficiency and Cu overload, although the exact metabolic roles of these elements remain unclear (100–102). In the recent decade, a variety of studies have been carried out to examine the concentrations of more elements or ionomes in different biological samples of patients with obesity and healthy controls, which demonstrate more complicated changes linked to the prevalence of this disease (60, 62, 103–117). Consistent with previous observations, significantly increased levels of Cu and decreased levels of Zn and Fe in multiple samples (especially in serum) appear to be the most common features for obesity. However, the relationship between obesity and the circulating levels of Se is still controversial. A recent meta-analysis revealed that overweight/obese individuals might have lower levels of Se in the urine and nails but higher levels in the hair (18). Compared to DM, deficiency of essential trace elements may play a more important role in the pathogenesis of obesity and obesity-related metabolic dysfunction, especially in women and children. The Cu/Zn ratio was also mainly increased in several types of samples of the obese groups (in particular in diabetic obese individuals), and was negatively associated with increased risk of metabolic abnormalities in the urine (108, 113). In addition, exposure to environmental toxic metals in the body may significantly influence the ionome and other metabolic parameters of obesity. For instance, Skalny et al. reported that Hg-exposed overweight/obese subjects had quite different hair elemental profiles (such as elevated Se and Zn and decreased Mn and Mg levels) and a more adverse effect on metabolic parameters when compared to unexposed obese adults, suggesting that metabolic alterations observed in obesity may be partially related to Hg-associated disturbances in trace element metabolism (118).
A significant number of studies with larger cohorts have been performed recently to improve our understanding of the relationship between obesity and single or few essential/non-essential elements in various biofluids and tissues, which showed generally similar changes in the concentrations of some of them in different populations, such as increased serum and hepatic tissue Cu levels (119, 120), decreased serum, hair, and salivary Zn levels (121–123), decreased fingernail Se levels (124), and increased toxic heavy metal (such as Al, Hg, Pb, and As) contents (125–128). Many of these significantly changed elements are positively or negatively correlated with body mass index (BMI) and waist circumference, which are commonly used to estimate the risk of overweight, obesity, and some other metabolic diseases such as MetS (128, 129). Moreover, levels of some elements are associated with insulin resistance in obese adults. Kim and Song examined the concentrations of several trace elements in the hair of viscerally obese adults, and found that Cr and Se levels were inversely associated with insulin resistance, whereas Cu level has a positive correlation with insulin resistance (130). Thus, imbalance of these micronutrients might be involved in the development of obesity, which could be used as potential indicators for its incidence. Interestingly, although serum levels of Cu and Zn are thought to be significantly related to obesity in both children and adults, a recent study showed that they did not differ significantly between pre-obese and obese children, implying that alternations of the two metals are earlier than the occurrence of obesity (131). Nevertheless, further research is needed to confirm these findings prospectively in larger study populations and to extend the understanding of the roles of different elements in the prevalence of obesity.
3.3. Metabolic syndrome
MetS is a group of metabolic disorders, including abdominal obesity, hypertension, high blood sugar levels (insulin resistance or glucose intolerance), and dyslipidemia [high triglyceride and low high-density lipoprotein (HDL)-cholesterol levels]. It may raise the risk of CVD, T2DM, stroke, and other serious health problems (4). The cause of MetS has been intensively studied, and many factors (such as genetic and metabolic susceptibility) may contribute to the development of MetS (132). Among these, an imbalance in trace element status is an important risk factor and potential biomarkers for MetS (133). The important roles of several micronutrients (such as Fe, Zn, and Cr) in carbohydrate and lipid metabolism and their alterations in MetS have been discussed in multiple reviews, which provide evidence for the involvement of trace element dyshomeostasis in the pathology of MetS (4, 134–136).
In early years, a limited number of studies have been conducted to explore the distribution and relationships of selected trace elements in different samples of patients with MetS and normal controls, which revealed that some of these elements might be related to the incidence and course of MetS (137–140). For instance, elevated circulating Cu levels and body Fe stores as well as reduced serum levels of Zn were reported in patients with MetS in different areas. On the other hand, controversy exists with regard to the relationship between MetS and Se levels in body, probably due to differences in study design, methodology, and population characteristics (141).
Several ionomic profiling studies with large cohorts of patients were carried out in the recent decade (62, 142–150), which basically support previous observations that accumulations of Cu and Fe in the body are strongly associated with the increased prevalence of MetS. Moreover, imbalance of additional elements were detected in different biological samples of MetS cases, implying a high degree of variability of the ionomic patterns in patients with MetS. Generally, the majority of significantly changed elements have positive associations with MetS, especially environmental toxic metals (such as Pb, Cd, and As), implying that exposure to these elements affects the body composition and metabolic profiles and further exacerbates the risk of MetS (142, 144–149). In contrast, decreased Mg levels were often observed in the blood (including plasma and serum) and hair samples from patients from different regions (62, 142, 143, 150), which could influence glucose metabolism and insulin sensitivity and action as well as promote chronic inflammation, linking Mg deficiency with developing MetS and other metabolic disorders such as T2DM and obesity (151). So far the ionomic information available for assessing the potential relationship between Zn status and the incidence of MetS is inconsistent and may vary by gender. It seems that increased Zn levels in the serum/plasma samples may lead to the occurrence of MetS in men but have a protective effect in women (143, 148, 152). In addition, some of the elements were found to be significantly associated with different MetS components. For example, Wen et al. have shown that urine Cu and blood Pb levels were positively associated with obesity-related indices such as body roundness index and body/visceral adiposity index, and urine Ni was positively correlated with lipid accumulation product in a large cohort of MetS cases (145). Increased Ca/Mg ratio in the hair was also found to have a positive correlation with insulin resistance in Korean adult males (153). Very recently, Zhang et al. investigated the relationship between the blood concentrations of several essential metals and MetS components in Chinese children, which revealed that different metals correlate with MetS components individually, such as Cu (positively associated with elevated waist and triglyceride levels) and Mg (positively associated with reduced HDL-cholesterol levels) (154). Such information may suggest a potential use of ionomic data in monitoring the progression of MetS components and helping determine the proper treatment strategies.
Besides the ionome-level studies mentioned above, more case-control studies and meta-analyses recently focused on single or several trace elements and explored their differences between MetS patients and healthy controls in different biological samples (17, 155–164). Although some of these results appear to be contradictory and ambiguous, it appears that elevations of Se, Cu, Zn (in males), and toxic metals (such as Cd and Hg) and reduction of Mg in blood samples are associated with increased risk of MetS and its components. Besides Zn, gender difference may affect the correlation between MetS and some other elements such as Se (165). Moreover, interactions between different elements may modify their own roles in MetS, e.g., the harmful effects of Hg exposure on MetS could be significantly attenuated by high levels of Se in toenails (166). Future studies should aim to unravel the mechanisms underlying the associations between these elements and the development of MetS to assist in the prevention and early intervention of this disease and its components.
3.4. Non-alcoholic fatty liver disease
NAFLD is the most common chronic liver disease worldwide, which is characterized by excessive fat accumulation (≥5%) in hepatocytes in the absence of excessive alcohol consumption (167). It encompasses a broad histological spectrum ranging from simple hepatic steatosis to non-alcoholic steatohepatitis (NASH), cirrhosis, and hepatocellular carcinoma (HCC) that may develop further (168). Because of the lack of efficient diagnostic methods and therapeutic targets, early detection and treatment of NAFLD still face many challenges. The underlying mechanism for the pathogenesis and progression of NAFLD is complex, and disturbance in trace element and mineral metabolism and interactions have been identified as one of the key risk factors of this disease (169, 170). As NAFLD is closely associated with the components of MetS, obesity, and T2DM, a change in nomenclature from NAFLD to metabolic-associated fatty liver disease (MAFLD) has been recently proposed, which may better reflect the underlying pathophysiology of this disease (171). Here, we still use NAFLD in the following sections.
The association between certain trace elements and NAFLD risk has been reported since 1990s, such as hepatic Fe overload and lower liver and serum Cu concentrations in different types of NAFLD patients (including pronounced hepatic steatosis, NASH, and even NASH-related cirrhosis with HCC) (172–177). The serum ferritin, a marker of Fe storage, has been considered as an independent predictor of histologic severity and fibrosis in patients with NAFLD, which may also indicate that changes in Fe speciation [say, the conversion between Fe(II) and Fe(III) species] are related to the pathogenesis of this disease (178). However, children with NASH exhibit decreased serum Fe levels with no significant Fe accumulation in the liver, implying a new mechanism for Fe deficiency in pediatric NASH (179). To date, very few ionomics studies have been carried out for NAFLD, which mainly used blood samples from patients. For the first time, Asprouli et al. measured plasma ionomes in different stages (mild, moderate, and severe) of NAFLD subjects in Greece, which demonstrated that Zn had a negative association with the severity of the disease whereas cesium (Cs) showed a positive correlation (180). Li et al. examined the relationship between NAFLD and serum contents of a variety of metals based on cross-sectional and longitudinal analyses in Chinese men, and found that depressed Mo and elevated Zn levels were associated with an increased risk of NAFLD (181). It should be admitted that ionomics in NAFLD is still at the very beginning of its development. So far ionomic profiling of other biological samples in NAFLD patients is not yet available.
In the last few years, a large number of studies have explored the correlation between single or several elements and the development of NAFLD. First, consistent with previous findings, Fe accumulation appears to be the most significant and common marker for NAFLD, and indicators of Fe status (e.g., serum Fe and ferritin levels) could be used for the prediction of disease severity and adverse outcomes (such as the risk of advanced hepatic fibrosis and HCC) in different populations (182–186). Second, decreased Cu and Mn concentrations in hepatic tissues, serum, and/or hair were reported in different cohorts of NAFLD patients, and higher Cu or Mn levels achieved significant protective effect against NAFLD, especially in men (187–192). In addition, recent Cu isotopic composition analysis showed that serum 65Cu/63Cu ratio was significantly lower in NAFLD patients and remained stable during disease progression, suggesting its potential for early diagnosis of NAFLD (193). Third, although the relationship between Zn deficiency and the incidence of NAFLD is still uncertain, low serum levels of Zn have been proposed to serve as an independent risk factor for poor prognosis in patients with NAFLD (194–197). In contrast, the roles of Se in different types of NAFLD are complex and conflicting (198). It seems that increased plasma/serum Se levels are positively associated with elevated prevalence of liver steatosis but negatively associated with that of hepatic fibrosis or HCC (198–201). Finally, exposure to toxic heavy metals (such as Pb, Cd, Hg, and Ni) was significantly associated with the onset and progression of NAFLD in different regions of the world, which is probably a causative factor of hepatic steatosis and fibrosis in some patients (202–208). Moreover, increased serum Ca and phosphorus (P) levels were also reported as independent risk factors for fatty liver in Korean population (209). Although not at the ionomic level, these single/few element-based studies might still provide useful information regarding the changes of trace elements in biological samples of NAFLD patients. Further investigation is necessary to evaluate the value of ionomic changes in the early detection of NAFLD individuals.
3.5. Hyperlipidemia and hyperuricemia
Hyperlipidemia (also referred to dyslipidemia) represents the condition of abnormally elevated levels of one or more lipids and/or lipoproteins in the blood, mainly including high levels of total cholesterol (hypercholesterolemia), triglycerides (hypertriglyceridemia), and low-density lipoprotein (LDL)-cholesterol or low levels of HDL-cholesterol. It is a significant risk factor for a variety of diseases such as fatty liver, CVD, DM, neurodegenerative diseases, and acute pancreatitis (210). Hyperuricemia (serum uric acid level ≥6.8 mg/dL), a key risk factor for the development of gout, is caused by increasing production and/or decreasing clearance of uric acid (the end product of purine metabolism) (211). In addition, hyperuricemia may also increase the risk of chronic kidney disease, CVD, metabolic disorders such as hyperlipidemia, obesity, and other insulin resistance-related syndromes (212). In recent years, the incidence of hyperlipidemia and hyperuricemia has increased significantly, which have become two of the most common health threatening disorders worldwide. Therefore, the prevention and treatment of the two pathological conditions are important for a reduced risk of more severe outcomes such as atherosclerosis, coronary heart disease, and renal disease (213, 214). It has been proposed that trace elements and minerals play an important role in the regulation of lipid and purine metabolism. Thus, investigation of the association between trace element imbalance and the occurrence of hyperlipidemia and hyperuricemia might be helpful to study the etiology and pathogenesis of the two metabolic diseases and to develop new lipid- and uric acid-lowering drugs.
The relationship between hyperlipidemia and trace element homeostasis has been analyzed in early times. Deficiency of several essential elements (Zn, Fe, Cr, and Se), Cu excess, and significant urinary excretion of Fe, Zn, and Cu were observed to be associated with the prevalence of hyperlipidemia (215–219). However, Cu deficiency and hepatic Fe overload were reported to contribute to hypertriglyceridemia and hypercholesterolemia in rats, and the genetic mechanisms driven to hypertriglyceridemia might favor Fe deposits (220–222). Moreover, some lipid-lowering agents (e.g., statins) could greatly influence the serum levels of multiple trace elements such as Zn and Cu (223). In the last decade, very few ionomics studies have been conducted for hyperlipidemia patients. Yerlikaya et al. found that lower levels of essential elements (Cr, Fe, Mn, Mo, and Se) as well as higher levels of Co, Ni, and Cd in serum are associated with an increased risk of primary hyperlipidemia, suggesting an important role of these elements in the regulation of lipid homeostasis (224). On the other hand, several studies have either confirmed previous observations or revealed additional elements that are associated with hyperlipidemia. For example, high Mn exposure could decrease the risk of high triglycerides (225), while elevated blood/serum levels of toxic heavy metals (such as Hg, As, and Pb) may increase the risk of different types of hyperlipidemia (226–228). Very recently, Barragán et al. examined the associations of plasma essential trace elements with hyperlipidemia in a Mediterranean population, which demonstrated that plasma Mg concentrations and combined effect of plasma Zn, Cu, and Se levels had a positive association with hypercholesterolemia whereas plasma Mn concentrations would have negative effects on the amount of plasma lipids (229, 230). A U-shaped link was suggested to exist between plasma Se levels and hypercholesterolemia, indicating that both low and high Se concentrations might have negative effects on plasma lipids (230).
Dyshomeostasis of trace elements has also been implicated in the incidence and progression of hyperuricemia for a long time. In general, circulating levels of several essential metals (such as Ca, Fe, and Cu) and toxic metals (Pb and As) showed positive correlations with the prevalence of hyperuricemia in different populations (231–238). In contrast, concentrations of Mg in serum and I in urine were inversely associated with the prevalence of hyperuricemia (239, 240). Although the relationship between Zn levels and hyperuricemia has not been explored, dietary Zn intake was reported to be inversely correlated with hyperuricemia in different regions, suggesting that Zn deficiency might increase the susceptibility of hyperuricemia (241, 242). Interestingly, there was a discrepancy of the association between blood Cd, serum uric acid levels, and hyperuricemia in different genders. Several studies have shown that blood Cd levels had a positive correlation with increasing risk of hyperuricemia in men (243, 244) or a negative correlation in women in Chinese and Korean populations (237). However, an opposite pattern was observed among populations in the U.S., in which serum Cd levels (in the acceptable range) were positively associated with increased prevalence of hyperuricemia in women, but inversely associated in men (245). This may suggest that different compositions of blood, race, and different ranges of blood Cd can affect the effect of Cd exposure on uric acid levels (246). So far the ionomics study on hyperuricemia is almost a blank. Wang et al. measured the plasma levels of 13 metals in hyperuricemia patients and controls in Chinese Han adults and found that higher plasma levels of several metal ions (particularly Zn and As) and lower levels of Co might increase hyperuricemia risk (247). Ma et al. reported that increased urinary levels of toxic metals (As and Cd) and decreased levels of essential metals (Co, Mn, and I) might have a positive combined effect on hyperuricemia (248). A systematic meta-analysis has been recently conducted to clarify the relationship between exposure to certain metals and the risk of hyperuricemia, which revealed that exposure to As, Ca, Cd, and Pb is associated with an increased risk of hyperuricemia whereas Mo exposure appears to be associated with a decreased prevalence of this disease (249). All these findings reinforce the importance of heavy metal (especially environmental toxic metal) accumulations in the risk of hyperlipidemia and hyperuricemia in general population. Moreover, alternations specific for each of the two metabolic diseases (e.g., elevated serum Co levels in hyperlipidemia and decreased plasma Co and urinary I levels in hyperuricemia) might help to provide potential markers for distinguishing them before clinical symptoms appear. Further ionome-level studies are required to validate the above results and to explore new patterns of elemental profiles in more biological samples and larger cohorts.
3.6. Osteoporosis and osteomalacia
Osteoporosis is a systemic bone metabolic disease characterized by bone mass reduction and bone microstructure destruction, which increases the risk of fracture, particularly in older postmenopausal women. The pathogenesis of osteoporosis is complex and involves the interaction of genetic, hormonal, environmental, and nutritional factors (250). Osteomalacia (or rickets in children) is a metabolic bone disorder of decreased mineralization of newly formed bone due to the lack of available Ca, P, or vitamin D. The major causative factors of osteomalacia include nutritional deficiency, impaired absorptive capabilities, and renal insufficiency or dialysis (251). Trace elements have been known to be essential for maintaining bone health, whose imbalance may be significantly associated with the disorders of bone metabolism and further lead to osteoporosis and/or osteomalacia (252, 253). Analysis of the relationship between trace element contents and osteoporosis/osteomalacia may assist in the prevention, diagnosis, and treatment of bone metabolic diseases.
The correlation between certain trace element and mineral levels within the body and osteoporosis has been examined in a large number of population-based studies, case-control cohort studies, and meta-analyses since the early 1970s. In general, reduced circulating levels and/or elevated urinary excretion of a variety of essential metals [such as Ca, Cu, Zn, Mg, Se, and boron (B)] as well as accumulation of Fe, Mn, and toxic heavy metals (Cd, Pb, and As) are strongly related to the prevalence of osteoporosis (254–266). In the recent decade, with the widespread use of ionomics approaches in various complex diseases, several studies have been carried out to provide evidence of the relationship between trace elements in blood and bone tissues and osteoporosis. For the first time, Noor et al. analyzed the difference of the bone concentrations of 27 elements from Indonesian postmenopausal women with and without osteoporosis, which showed that increased B and As and decreased Se levels in bone tissues might directly contribute to microarchitectural abnormalities in osteoporotic bones (267). Another ionomics study suggested that low serum levels of Cu, Zn, Fe, and Mg are associated with the incidence of osteoporosis in Turkish postmenopausal women, implying that Fe deficiency is also one of the risk factors for the development of osteoporosis (268). Lin et al. measured multi-elemental contents in human bone tissues of older Chinese adults and found a significant correlation between decreased Cu, Zn, and Mn levels and increased risk of osteoporosis, indicating that both Mn exposure and deficiency (although very rare in human body) might lead to abnormal bone metabolism, osteoporosis, and even fractures (269). A SVM-based prediction model for bone mineral density loss and osteoporosis was recently proposed by using urinary concentrations of several toxic metals (such as As and Cd) and some other features, suggesting the possibility to develop new methods for the early detection of osteoporosis based on elemental distribution in biofluids (270). Additionally, lowering excessive toxic metals and/or supplementation of deficient essential elements might be valuable for the therapy of osteoporosis.
In the past several decades, besides low serum levels of Ca and P that have been proposed as a screening test in patients who have clinical symptoms suggestive of osteomalacia (271), elevated concentrations of several other elements have been observed in different biological samples of osteomalacia patients, such as Al, Fe, Cd, and Sr in bone or serum and Pb in blood or hair (childhood rickets) (272–275). In contrast, Fe deficiency was reported in autosomal dominant hypophosphatemic rickets, a rare form of rickets caused by mutations in the fibroblast growth factor 23 (FGF23) gene, implying that monitoring the Fe status is helpful for diagnosis, prognosis, and treatment of this genetic disease (276). Moreover, Ca/Zn ratio in bone was found to be related to the degree of osteomalacia in Cd-exposed subjects, indicating that interactions among these metals might be involved in the pathogenesis of osteomalacia (277). To date, ionomics studies on osteomalacia/rickets are extremely rare. Previously, Miekeley et al. reported that elevated concentrations of Ca, Cd, Al, Fe, and several other elements in hair were associated with the incidence of osteomalacia, which could be used as a complementary tool for its early detection (278). Dogan et al. examined multiple trace element levels in serum and found significantly decreased Zn concentrations in children with nutritional rickets associated with vitamin D deficiency, suggesting an important role of Zn in the early steps of bone maturation (279). However, the correlations between many other trace elements and the prevalence of osteomalacia/rickets are still unclear. Future investigations are necessary to identify more ionome-level variations for bone metabolic diseases.
4. Development of integrated ionomic fingerprints for metabolic disease
Comparison of the integrated ionomic profiles in different biological samples for major types of metabolic diseases has demonstrated common/specific trends in the changes of elemental contents (Figure 1). Considering that the number of ionomics studies for each subtype of blood samples (i.e., whole blood, serum, and plasma) is very limited for most metabolic diseases, in this review, we combined different subtypes of blood samples to try to give a general trend of the changes of elemental concentrations in the blood as previously adopted for cancer blood metallomics (280).
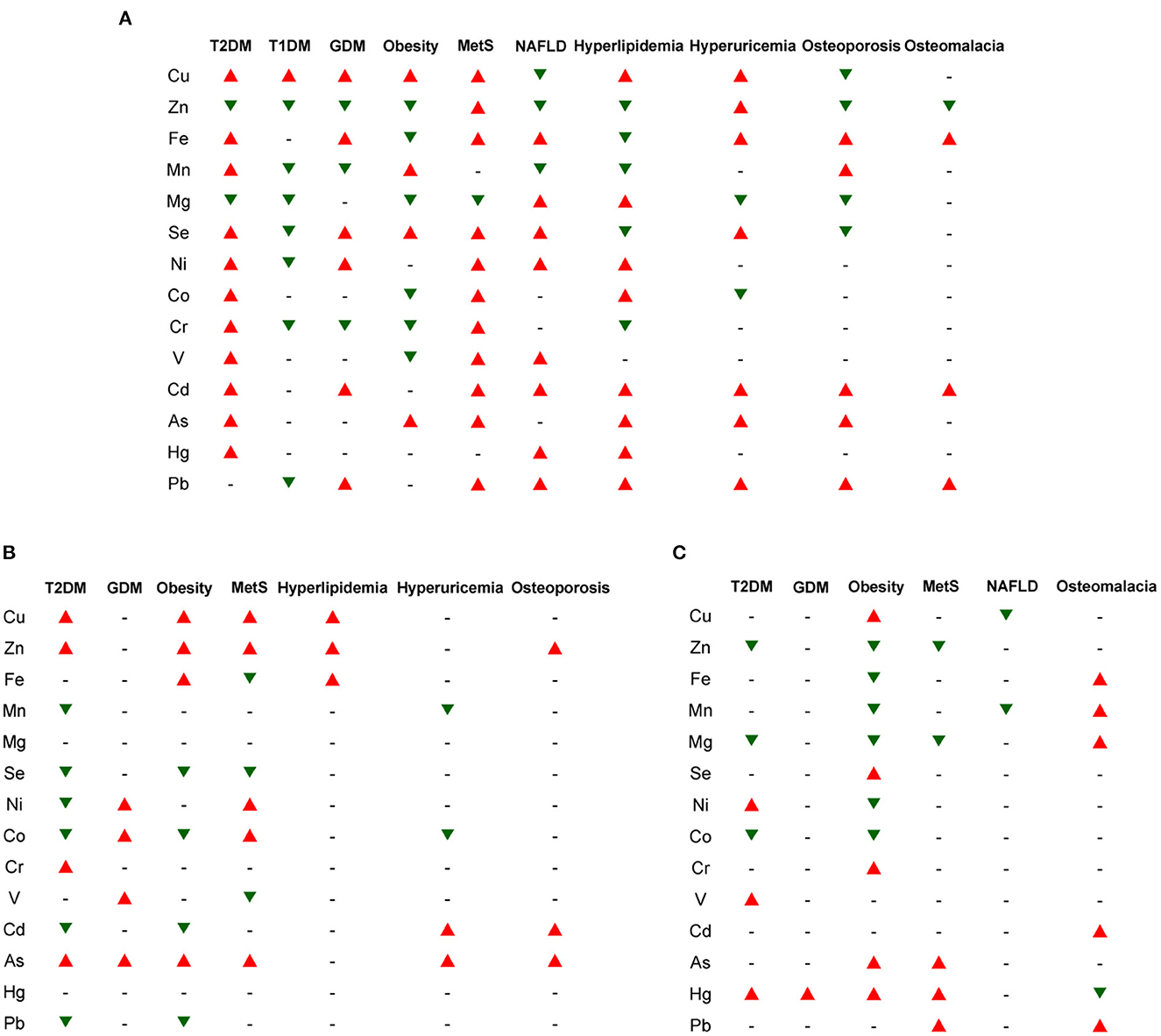
Figure 1. The general trends in the changes of major elemental concentrations in different biological samples for several types of metabolic diseases. (A) blood (including whole blood, serum, and plasma); (B) urine; (C) hair/nails. The red and green triangles indicate increased and decreased trends of elemental levels, respectively, which have been reported in the majority of ionomic and other related studies using the corresponding samples from patients. The short line (–) represents unclear or no significant change. T1DM, type 1 diabetes mellitus; T2DM, type 2 diabetes mellitus; GDM, gestational diabetes mellitus; MetS, metabolic syndrome; NAFLD, non-alcoholic fatty liver disease.
Among all examined ions, Cu levels are significantly increased in almost all examined samples (except hair/nails) from patients with various metabolic diseases (except NAFLD and bone metabolic diseases), indicating that Cu accumulation is one of the common risk factors for a wide variety of metabolic diseases and might act as a general marker for diagnosis and poor prognosis of them. Elevated concentrations of Se and environmental heavy metals (such as Ni, Cd, As, and Pb) and reduced levels of Zn and Mg are often observed in the blood/serum/plasma and hair/nails in patients with metabolic diseases, suggesting that alternations in the homeostasis of these elements may also serve as indicators of these diseases. In contrast, higher excretion of Zn and lower excretion of Se, Cd, and Pb in the urine were observed in several major types of metabolic diseases (especially T2DM, obesity, and MetS), which may partially contribute to the imbalance of these elements within the patients. In addition, the Cu/Zn ratio is also increased in the serum/hair from patients with T2DM and obesity, indicating its potential as a general circulating marker for these diseases.
Although the elemental contents are distinct and highly dynamic in different samples from patients with same/different metabolic diseases, each sample type appears to have specific ionomic pattern, which could be further used to develop novel biomarkers or prediction models for the respective disease. Clustering analysis revealed that same sample types from patients with different metabolic diseases may have quite similar or correlated ionomic patterns (Figure 2), such as blood (including whole blood, serum, and/or plasma) and hair/nails from T2DM and MetS as well as urine samples from T2DM and obesity, which might be associated with common pathogenetic mechanisms (say, insulin resistance) of these diseases (281). On the other hand, although most sample types from same/different diseases showed diverse elemental profiles, certain samples from patients with the same disease were clustered together, suggesting a potential relationship between them (Figure 2). For example, blood and hair/nails from obesity patients have same trends in the changes of major elemental concentrations (say, decreased levels of Zn, Fe, and Mg and increased levels of Se), whereas the urine samples of obesity have somewhat different elemental patterns (such as increased Zn and Fe, decreased Se and Cd, and unchanged Mg levels). Previous human biomonitoring programs have shown significantly different elemental contents between hair and urine samples in physiological conditions (282). It has been suggested that urine can be used for the monitoring of very low levels of elements and the assessment of recent exposure of heavy metals due to its rapid clearing and metabolism, whereas hair and nail samples may serve as indicators for long term exposure of environmental chemicals and toxic metal accumulation in various populations (282–284). Moreover, the elemental ratio or correlation analysis (shown in Table 1) may offer additional clues with respect to the complex crosstalk between elements in different types of metabolic diseases.
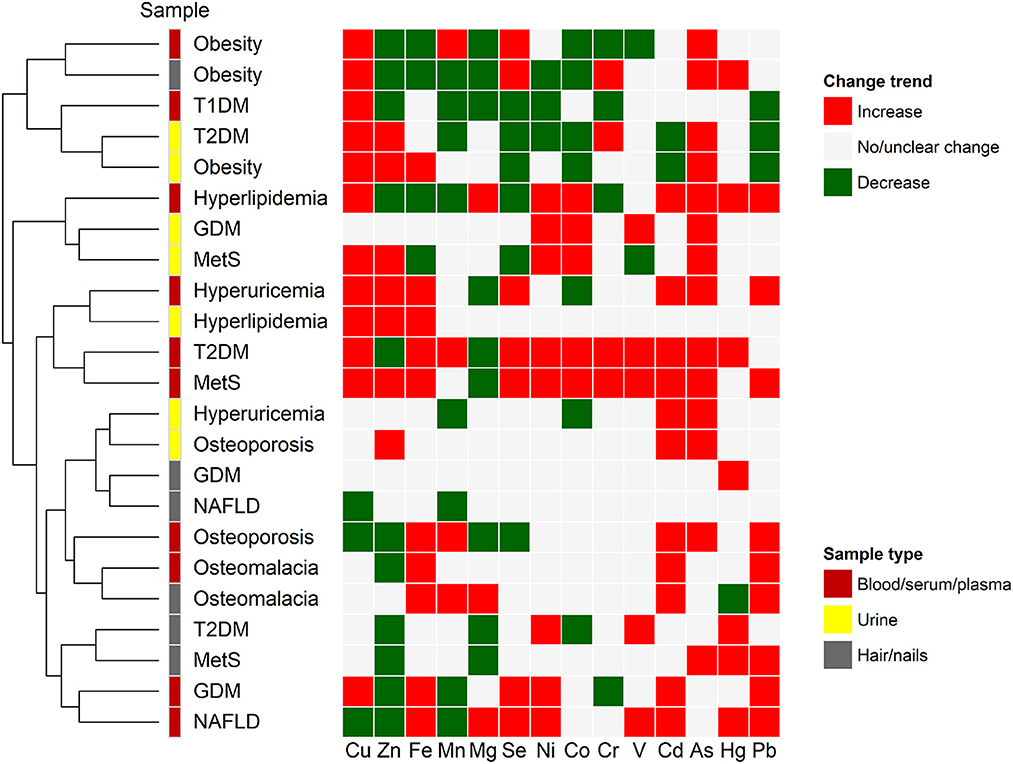
Figure 2. Clustering of ionomic profiles for metabolic disease. Alternations in the concentrations of major elements in several types of biological samples of patients with different metabolic diseases were analyzed using hierarchical clustering approach. Red and green colors represent increased and decreased trends of elemental concentrations, respectively, when compared with healthy controls. The gray color represents no significant or unclear change. Abbreviations of different diseases are the same as those in Figure 1.
Although ionomics provides valuable insights into the mineral and trace element composition in a living organism and their relationship with different physiological and pathological conditions, there are still limitations for its potential role in diagnosis and prognosis of various diseases including metabolic diseases. One major limitation of current ionomics studies is the relatively small number and the varying characteristics (e.g., age, gender, and history of smoking and drinking) of patients and controls enrolled in different projects, which might restrict the power of ionomic evaluation when considering the high variability of trace element contents in biological samples. Another limitation is that ionomics study could not provide a definite cause and effect relationship between dyshomeostasis of trace elements and the development of metabolic diseases. Moreover, studies on the connection between alternations of elemental profiles and the levels of element-binding proteins (say, metalloproteins) and the dynamic interplay between elements are still lacking for most metabolic diseases. Nevertheless, ionomic analyses of biofluids, hair, nails, and other related tissues may be useful to give a comprehensive overview of the complex interactions between trace elements and minerals and metabolic diseases and help to generate new fingerprints for diagnosis, prognosis, and even for evaluation of therapeutic efficacy in these diseases, which cannot be solved only by the assessment of single or very few element levels.
5. Conclusions and perspectives
Disruption of ion metabolism and homeostasis plays a significant role in various diseases including cancer, metabolic diseases, neurological diseases, CVD, and many more. In the recent decade, ionomics has become a powerful tool for systematically investigating the composition and distribution of trace elements and minerals in different biological systems. However, our understanding of the relationship between ionome and the pathogenesis of metabolic diseases is limited so far.
This review focuses on several major types of metabolic diseases and introduces recent progress in the application of ionomics in the study of these diseases, which may raise the possibility of using such information for early detection and prognosis of patients with metabolic disease. Increased Cu concentrations in the majority of biological samples appear to be the most significant marker for various metabolic diseases. Elevated circulating levels of Se and environmental toxic metals and reduced Zn levels have also been demonstrated to be the common risk factors for the majority of metabolic diseases. Other elemental and inter-elemental biomarkers may also reflect the imbalance of ion contents that occurs in different samples of same/different types of metabolic diseases. In spite that the use of ionomics in the field of human disease remains at the initial stage, it may help to depict the crosstalk among different ions in disease pathogenesis and provide a potential source of diagnostic, prognostic, and predictive biomarkers, particularly in the context of metabolic disease. Future research with larger and more representative samples and/or more complex elemental composition (including chemical speciation and isotope analyses) is encouraged to improve our current understanding of the association between the ionomic network and the onset and progression of different types/subtypes of metabolic diseases.
Author contributions
YZ and HY conceived and wrote the manuscript. BH, JJ, and YX reviewed and edited the manuscript. All authors contributed to the article and approved the submitted version.
Funding
This work was funded by National Natural Science Foundation of China (32270680), Shenzhen-Hong Kong Institute of Brain Science-Shenzhen Fundamental Research Institutions (2022SHIBS0003), Medical Health Science and Technology Project of Zhejiang Provincial Health Commission (2020KY767), and Hangzhou Biomedicine and Health Industry Development Special Project (2021WJCY007).
Conflict of interest
The authors declare that the research was conducted in the absence of any commercial or financial relationships that could be construed as a potential conflict of interest.
Publisher's note
All claims expressed in this article are solely those of the authors and do not necessarily represent those of their affiliated organizations, or those of the publisher, the editors and the reviewers. Any product that may be evaluated in this article, or claim that may be made by its manufacturer, is not guaranteed or endorsed by the publisher.
References
1. Arner P, Bernard S, Salehpour M, Possnert G, Liebl J, Steier P, et al. Dynamics of human adipose lipid turnover in health and metabolic disease. Nature. (2011) 478:110–3. doi: 10.1038/nature10426
2. Otero YF, Stafford JM, McGuinness OP. Pathway-selective insulin resistance and metabolic disease: the importance of nutrient flux. J Biol Chem. (2014) 289:20462–9. doi: 10.1074/jbc.R114.576355
3. Dubey P, Thakur V, Chattopadhyay M. Role of minerals and trace elements in diabetes and insulin resistance. Nutrients. (2020) 12:1864. doi: 10.3390/nu12061864
4. Shi Y, Zou Y, Shen Z, Xiong Y, Zhang W, Liu C, et al. Trace elements, PPARs, and metabolic syndrome. Int J Mol Sci. (2020) 21:2612. doi: 10.3390/ijms21072612
5. Himoto T, Masaki T. Current trends of essential trace elements in patients with chronic liver diseases. Nutrients. (2020) 12:2084. doi: 10.3390/nu12072084
6. Siddiqui K, Bawazeer N, Joy SS. Variation in macro and trace elements in progression of type 2 diabetes. ScientificWorldJournal. (2014) 2014:461591. doi: 10.1155/2014/461591
8. Chellan P, Sadler PJ. The elements of life and medicines. Philos Trans A Math Phys Eng Sci. (2015) 373:20140182. doi: 10.1098/rsta.2014.0182
9. Mertz W. Review of the scientific basis for establishing the essentiality of trace elements. Biol Trace Elem Res. (1998) 66:185–91. doi: 10.1007/BF02783137
10. Jomova K, Makova M, Alomar SY, Alwasel SH, Nepovimova E, Kuca K, et al. Essential metals in health and disease. Chem Biol Interact. (2022) 367:110173. doi: 10.1016/j.cbi.2022.110173
11. Pratush A, Kumar A, Hu Z. Adverse effect of heavy metals (As, Pb, Hg, and Cr) on health and their bioremediation strategies: a review. Int Microbiol. (2018) 21:97–106. doi: 10.1007/s10123-018-0012-3
12. Javaid A, Akbar I, Javed H, Khan U, Iftikhar H, Zahra D, et al. Role of heavy metals in diabetes: mechanisms and treatment strategies. Crit Rev Eukaryot Gene Expr. (2021) 31:65–80. doi: 10.1615/CritRevEukaryotGeneExpr.2021037971
13. Van Gossum A, Neve J. Trace element deficiency and toxicity. Curr Opin Clin Nutr Metab Care. (1998) 1:499–507. doi: 10.1097/00075197-199811000-00004
14. Ma W, Jia L, Xiong Q, Feng Y, Du H. The role of iron homeostasis in adipocyte metabolism. Food Funct. (2021) 12:4246–53. doi: 10.1039/D0FO03442H
15. Aigner E, Weiss G, Datz C. Dysregulation of iron and copper homeostasis in nonalcoholic fatty liver. World J Hepatol. (2015) 7:177–88. doi: 10.4254/wjh.v7.i2.177
16. Cui Z, Zhou H, Liu K, Wu M, Li S, Meng S, et al. Dietary copper and selenium intakes and the risk of type 2 diabetes mellitus: findings from the china health and nutrition survey. Nutrients. (2022) 14:2055. doi: 10.3390/nu14102055
17. Li B, Huang Y, Luo C, Peng X, Jiao Y, Zhou L, et al. Inverse association of plasma molybdenum with metabolic syndrome in a Chinese adult population: a case-control study. Nutrients. (2021) 13:4544. doi: 10.3390/nu13124544
18. Fontenelle LC, Cardoso de Araújo DS, da Cunha Soares T, Clímaco Cruz KJ, Henriques GS, Marreiro DDN. Nutritional status of selenium in overweight and obesity: a systematic review and meta-analysis. Clin Nutr. (2022) 41:862–84. doi: 10.1016/j.clnu.2022.02.007
19. Sandström B. Micronutrient interactions: effects on absorption and bioavailability. Br J Nutr. (2001) 85 (Suppl. 2):S181–5. doi: 10.1079/BJN2000312
20. Schwalfenberg GK, Genuis SJ. Vitamin D, essential minerals, and toxic elements: exploring interactions between nutrients and toxicants in clinical medicine. ScientificWorldJournal. (2015) 2015:318595. doi: 10.1155/2015/318595
21. Kondaiah P, Yaduvanshi PS, Sharp PA, Pullakhandam R. Iron and zinc homeostasis and interactions: does enteric zinc excretion cross-talk with intestinal iron absorption? Nutrients. (2019) 11:1885. doi: 10.3390/nu11081885
22. Vinceti M, Bonaccio M, Filippini T, Costanzo S, Wise LA, Di Castelnuovo A, et al. Dietary selenium intake and risk of hospitalization for type 2 diabetes in the Moli-sani study cohort. Nutr Metab Cardiovasc Dis. (2021) 31:1738–46. doi: 10.1016/j.numecd.2021.02.016
23. Salt DE, Baxter I, Lahner B. Ionomics and the study of the plant ionome. Annu Rev Plant Biol. (2008) 59:709–33. doi: 10.1146/annurev.arplant.59.032607.092942
24. Baxter I. Ionomics: studying the social network of mineral nutrients. Curr Opin Plant Biol. (2009) 12:381–6. doi: 10.1016/j.pbi.2009.05.002
25. Baxter I. Ionomics: the functional genomics of elements. Brief Funct Genomics. (2010) 9:149–56. doi: 10.1093/bfgp/elp055
26. Eide DJ, Clark S, Nair TM, Gehl M, Gribskov M, Guerinot ML, et al. Characterization of the yeast ionome: a genome-wide analysis of nutrient mineral and trace element homeostasis in Saccharomyces cerevisiae. Genome Biol. (2005) 6:R77. doi: 10.1186/gb-2005-6-9-r77
27. Malinouski M, Hasan NM, Zhang Y, Seravalli J, Lin J, Avanesov A, et al. Genome-wide RNAi ionomics screen reveals new genes and regulation of human trace element metabolism. Nat Commun. (2014) 5:3301. doi: 10.1038/ncomms4301
28. Chaparro JM, Holm DG, Broeckling CD, Prenni JE, Heuberger AL. Metabolomics and ionomics of potato tuber reveals an influence of cultivar and market class on human nutrients and bioactive compounds. Front Nutr. (2018) 5:36. doi: 10.3389/fnut.2018.00036
29. Arruda MAZ, de Jesus JR, Blindauer CA, Stewart AJ. Speciomics as a concept involving chemical speciation and omics. J Proteomics. (2022) 263:104615. doi: 10.1016/j.jprot.2022.104615
30. Mahan B, Chung RS, Pountney DL, Moynier F, Turner S. Isotope metallomics approaches for medical research. Cell Mol Life Sci. (2020) 77:3293–309. doi: 10.1007/s00018-020-03484-0
31. Zhang Y. Trace elements and healthcare: a bioinformatics perspective. Adv Exp Med Biol. (2017) 1005:63–98. doi: 10.1007/978-981-10-5717-5_4
32. Zhang Y, Xu Y, Zheng L. Disease ionomics: understanding the role of ions in complex disease. Int J Mol Sci. (2020) 21:8646. doi: 10.3390/ijms21228646
33. Ali S, Tyagi A, Bae H. Ionomic approaches for discovery of novel stress-resilient genes in plants. Int J Mol Sci. (2021) 22:7182. doi: 10.3390/ijms22137182
34. Misra BB, Reichman SM, Chen S. The guard cell ionome: understanding the role of ions in guard cell functions. Prog Biophys Mol Biol. (2019) 146:50–62. doi: 10.1016/j.pbiomolbio.2018.11.007
35. Rahman SU, Nawaz MF, Gul S, Yasin G, Hussain B, Li Y, et al. State-of-the-art OMICS strategies against toxic effects of heavy metals in plants: a review. Ecotoxicol Environ Saf. (2022) 242:113952. doi: 10.1016/j.ecoenv.2022.113952
36. Yang HS, LaFrance DR, Hao Y. Elemental testing using inductively coupled plasma mass spectrometry in clinical laboratories. Am J Clin Pathol. (2021) 156:167–75. doi: 10.1093/ajcp/aqab013
37. Leclercq A, Nonell A, Todolí Torró JL, Bresson C, Vio L, Vercouter T, et al. Introduction of organic/hydro-organic matrices in inductively coupled plasma optical emission spectrometry and mass spectrometry: a tutorial review. Part I. Theoretical considerations. Anal Chim Acta. (2015) 885:33–56. doi: 10.1016/j.aca.2015.03.049
38. Ma S, Lee SG, Kim EB, Park TJ, Seluanov A, Gorbunova V, et al. Organization of the mammalian ionome according to organ origin, lineage specialization, and longevity. Cell Rep. (2015) 13:1319–26. doi: 10.1016/j.celrep.2015.10.014
39. Rudman SM, Goos JM, Burant JB, Brix KV, Gibbons TC, Brauner CJ, et al. Ionome and elemental transport kinetics shaped by parallel evolution in threespine stickleback. Ecol Lett. (2019) 22:645–53. doi: 10.1111/ele.13225
40. Zhang B, Podolskiy DI, Mariotti M, Seravalli J, Gladyshev VN. Systematic age-, organ-, and diet-associated ionome remodeling and the development of ionomic aging clocks. Aging Cell. (2020) 19:e13119. doi: 10.1111/acel.13119
41. Pushie MJ, Sylvain NJ, Hou H, Hackett MJ, Kelly ME, Webb SM. X-ray fluorescence microscopy methods for biological tissues. Metallomics. (2022) 14:mfac032. doi: 10.1093/mtomcs/mfac032
42. Grochowski C, Blicharska E, Krukow P, Jonak K, Maciejewski M, Szczepanek D, et al. Analysis of trace elements in human brain: its aim, methods, and concentration levels. Front Chem. (2019) 7:115. doi: 10.3389/fchem.2019.00115
43. Baxter I, Ouzzani M, Orcun S, Kennedy B, Jandhyala SS, Salt DE. Purdue ionomics information management system. An integrated functional genomics platform. Plant Physiol. (2007) 143:600–11. doi: 10.1104/pp.106.092528
44. Colmsee C, Mascher M, Czauderna T, Hartmann A, Schlüter U, Zellerhoff N, et al. OPTIMAS-DW: a comprehensive transcriptomics, metabolomics, ionomics, proteomics and phenomics data resource for maize. BMC Plant Biol. (2012) 12:245. doi: 10.1186/1471-2229-12-245
45. Whitt L, Ricachenevsky FK, Ziegler GZ, Clemens S, Walker E, Maathuis FJM, et al. A curated list of genes that affect the plant ionome. Plant Direct. (2020) 4:e00272. doi: 10.1002/pld3.272
46. Morel JD, Sauzéat L, Goeminne LJE, Jha P, Williams E, Houtkooper RH, et al. The mouse metallomic landscape of aging and metabolism. Nat Commun. (2022) 13:607. doi: 10.1038/s41467-022-28060-x
47. Li Q, Hu C, Lin J, Yang Z, Zhou Q, Yang R, et al. Urinary ionomic analysis reveals new relationship between minerals and longevity in a Han Chinese population. J Trace Elem Med Biol. (2019) 53:69–75. doi: 10.1016/j.jtemb.2019.02.002
48. Shen Z, Lin J, Teng J, Zhuang Y, Zhang H, Wang C, et al. Association of urinary ionomic profiles and acute kidney injury and mortality in patients after cardiac surgery. J Thorac Cardiovasc Surg. (2020) 159:918–26.e5. doi: 10.1016/j.jtcvs.2019.02.095
49. Golasik M, Jawień W, Przybyłowicz A, Szyfter W, Herman M, Golusiński W, et al. Classification models based on the level of metals in hair and nails of laryngeal cancer patients: diagnosis support or rather speculation? Metallomics. (2015) 7:455–65. doi: 10.1039/C4MT00285G
50. Iacovacci J, Lin W, Griffin JL, Glen RC. IonFlow: a galaxy tool for the analysis of ionomics data sets. Metabolomics. (2021) 17:91. doi: 10.1007/s11306-021-01841-z
51. Emmett SE. ICP-MS: a new look at trace elements in Alzheimer's disease. Prog Clin Biol Res. (1989) 317:1077–86.
52. Krachler M, Lindschinger M, Eber B, Watzinger N, Wallner S. Trace elements in coronary heart disease: impact of intensified lifestyle modification. Biol Trace Elem Res. (1997) 60:175–85. doi: 10.1007/BF02784438
53. Nyman DW, Suzanne Stratton M, Kopplin MJ, Dalkin BL, Nagle RB, Jay Gandolfi A. Selenium and selenomethionine levels in prostate cancer patients. Cancer Detect Prev. (2004) 28:8–16. doi: 10.1016/j.cdp.2003.11.002
54. Huang J, Xie L, Song A, Zhang C. Selenium status and its antioxidant role in metabolic diseases. Oxid Med Cell Longev. (2022) 2022:7009863. doi: 10.1155/2022/7009863
55. Li L, Yang X. The essential element manganese, oxidative stress, and metabolic diseases: links and interactions. Oxid Med Cell Longev. (2018) 2018:7580707. doi: 10.1155/2018/7580707
56. Poucheret P, Verma S, Grynpas MD, McNeill JH. Vanadium and diabetes. Mol Cell Biochem. (1998) 188:73–80. doi: 10.1007/978-1-4615-5763-0_9
57. Yamaguchi M. Role of nutritional zinc in the prevention of osteoporosis. Mol Cell Biochem. (2010) 338:241–54. doi: 10.1007/s11010-009-0358-0
58. Planchart A, Green A, Hoyo C, Mattingly CJ. Heavy metal exposure and metabolic syndrome: evidence from human and model system studies. Curr Environ Health Rep. (2018) 5:110–24. doi: 10.1007/s40572-018-0182-3
59. Rodríguez-Gutiérrez R, González-Saldivar G, González-González JG. Diagnosis of diabetes. N Engl J Med. (2013) 368:192–3. doi: 10.1056/NEJMc1212738
60. Skalnaya MG, Demidov VA. Hair trace element contents in women with obesity and type 2 diabetes. J Trace Elem Med Biol. (2007) 21 (Suppl. 1):59–61. doi: 10.1016/j.jtemb.2007.09.019
61. Flores CR, Puga MP, Wrobel K, Garay Sevilla ME, Wrobel K. Trace elements status in diabetes mellitus type 2: possible role of the interaction between molybdenum and copper in the progress of typical complications. Diabetes Res Clin Pract. (2011) 91:333–41. doi: 10.1016/j.diabres.2010.12.014
62. Sun L, Yu Y, Huang T, An P, Yu D, Yu Z, et al. Associations between ionomic profile and metabolic abnormalities in human population. PLoS ONE. (2012) 7:e38845. doi: 10.1371/journal.pone.0038845
63. Badran M, Morsy R, Soliman H, Elnimr T. Assessment of trace elements levels in patients with type 2 diabetes using multivariate statistical analysis. J Trace Elem Med Biol. (2016) 33:114–9. doi: 10.1016/j.jtemb.2015.10.006
64. Li XT, Yu PF, Gao Y, Guo WH, Wang J, Liu X, et al. Association between plasma metal levels and diabetes risk: a case-control study in China. Biomed Environ Sci. (2017) 30:482–91. doi: 10.3967/bes2017.064
65. Hansen AF, Simić A, Åsvold BO, Romundstad PR, Midthjell K, Syversen T, et al. Trace elements in early phase type 2 diabetes mellitus-A population-based study. The HUNT study in Norway. J Trace Elem Med Biol. (2017) 40:46–53. doi: 10.1016/j.jtemb.2016.12.008
66. Jac A, Csl B, Bps B, Tls B, Gld A. Combining elemental analysis of toenails and machine learning techniques as a non-invasive diagnostic tool for the robust classification of type-2 diabetes. Exp Syst Applic. (2019) 115:245–55. doi: 10.1016/j.eswa.2018.08.002
67. Lv Y, Xie L, Dong C, Yang R, Long T, Yang H, et al. Co-exposure of serum calcium, selenium and vanadium is nonlinearly associated with increased risk of type 2 diabetes mellitus in a Chinese population. Chemosphere. (2021) 263:128021. doi: 10.1016/j.chemosphere.2020.128021
68. Nie H, Hu H, Li Z, Wang R, He J, Li P, et al. Associations of plasma metal levels with type 2 diabetes and the mediating effects of microRNAs. Environ Pollut. (2022) 292:118452. doi: 10.1016/j.envpol.2021.118452
69. Luan F, Chen Y, Xu Y, Jiang X, Liu B, Wang Y. Associations between whole blood trace elements concentrations and HbA1c levels in patients with type 2 diabetes. Biometals. (2022) 35:1011–22. doi: 10.1007/s10534-022-00419-z
70. Joda BA, Ward NI. Use of human teardrop fluid for the determination of trace elements in healthy individuals and diabetic patients. J Trace Elem Med Biol. (2021) 65:126733. doi: 10.1016/j.jtemb.2021.126733
71. Chen H, Tan C. Prediction of type-2 diabetes based on several element levels in blood and chemometrics. Biol Trace Elem Res. (2012) 147:67–74. doi: 10.1007/s12011-011-9306-4
72. Chen H, Tan C, Lin Z, Wu T. The diagnostics of diabetes mellitus based on ensemble modeling and hair/urine element level analysis. Comput Biol Med. (2014) 50:70–5. doi: 10.1016/j.compbiomed.2014.04.012
73. Hasanato RM. Trace elements in type 2 diabetes mellitus and their association with glycemic control. Afr Health Sci. (2020) 20:287–93. doi: 10.4314/ahs.v20i1.34
74. Liu G, Sun L, Pan A, Zhu M, Li Z, ZhenzhenWang Z, et al. Nickel exposure is associated with the prevalence of type 2 diabetes in Chinese adults. Int J Epidemiol. (2015) 44:240–8. doi: 10.1093/ije/dyu200
75. Rangel-Moreno K, Gamboa-Loira B, López-Carrillo L, Cebrián ME. Prevalence of type 2 diabetes mellitus in relation to arsenic exposure and metabolism in Mexican women. Environ Res. (2022) 210:112948. doi: 10.1016/j.envres.2022.112948
76. González de Vega R, Fernández-Sánchez ML, Fernández JC, Álvarez Menéndez FV, Sanz-Medel A. Selenium levels and glutathione peroxidase activity in the plasma of patients with type II diabetes mellitus. J Trace Elem Med Biol. (2016) 37:44–9. doi: 10.1016/j.jtemb.2016.06.007
77. Ying H, Zhang Y. Systems biology of selenium and complex disease. Biol Trace Elem Res. (2019) 192:38–50. doi: 10.1007/s12011-019-01781-9
78. Marín-Martínez L, Molino Pagán D, López Jornet P. Trace elements in saliva as markers of type 2 diabetes mellitus. Biol Trace Elem Res. (2018) 186:354–60. doi: 10.1007/s12011-018-1326-x
79. Yakout S, Faqeeh F, Al-Attas O, Hussain SD, Saadawy GM, Al-Daghri NM, et al. Patterns of essential trace elements (Cr, Mn, Ni, and Se) in Saudi patients with type 2 diabetes mellitus. Am J Transl Res. (2022) 14:8175–82.
80. Sobczak AIS, Stefanowicz F, Pitt SJ, Ajjan RA, Stewart AJ. Total plasma magnesium, zinc, copper and selenium concentrations in type-I and type-II diabetes. Biometals. (2019) 32:123–38. doi: 10.1007/s10534-018-00167-z
81. Marín-Martínez L, Molino-Pagán D, López-Jornet P. Trace elements in saliva and plasma of patients with type 2 diabetes: association to metabolic control and complications. Diabetes Res Clin Pract. (2019) 157:107871. doi: 10.1016/j.diabres.2019.107871
82. Long T, Wang R, Wang J, Wang F, Xu Y, Wei Y, et al. Plasma metals and cardiovascular disease in patients with type 2 diabetes. Environ Int. (2019) 129:497–506. doi: 10.1016/j.envint.2019.05.038
83. Temurer Afşar Z, Ayçiçek B, Tütüncü Y, Çavdar Ü, Sennaroglu E. Relationships between microvascular complications of diabetes mellitus and levels of macro and trace elements. Minerva Endocrinol. (2020). doi: 10.23736/S0391-1977.20.03139-9
84. Forte G, Bocca B, Peruzzu A, Tolu F, Asara Y, Farace C, et al. Blood metals concentration in type 1 and type 2 diabetics. Biol Trace Elem Res. (2013) 156:79–90. doi: 10.1007/s12011-013-9858-6
85. Squitti R, Negrouk V, Perera M, Llabre MM, Ricordi C, Rongioletti MCA, et al. Serum copper profile in patients with type 1 diabetes in comparison to other metals. J Trace Elem Med Biol. (2019) 56:156–61. doi: 10.1016/j.jtemb.2019.08.011
86. Peruzzu A, Solinas G, Asara Y, Forte G, Bocca B, Tolu F, et al. Association of trace elements with lipid profiles and glycaemic control in patients with type 1 diabetes mellitus in northern Sardinia, Italy: an observational study. Chemosphere. (2015) 132:101–7. doi: 10.1016/j.chemosphere.2015.02.052
87. Roverso M, Berté C, Di Marco V, Lapolla A, Badocco D, Pastore P, et al. The metallome of the human placenta in gestational diabetes mellitus. Metallomics. (2015) 7:1146–54. doi: 10.1039/C5MT00050E
88. Roverso M, Di Marco V, Badocco D, Pastore P, Calanducci M, Cosmi E, et al. Maternal, placental and cordonal metallomic profiles in gestational diabetes mellitus. Metallomics. (2019) 11:676–85. doi: 10.1039/c8mt00331a
89. Wang X, Gao D, Zhang G, Zhang X, Li Q, Gao Q, et al. Exposure to multiple metals in early pregnancy and gestational diabetes mellitus: a prospective cohort study. Environ Int. (2020) 135:105370. doi: 10.1016/j.envint.2019.105370
90. Onat T, Demir Caltekin M, Turksoy VA, Baser E, Aydogan Kirmizi D, Kara M, et al. The relationship between heavy metal exposure, trace element level, and monocyte to hdl cholesterol ratio with gestational diabetes mellitus. Biol Trace Elem Res. (2021) 199:1306–15. doi: 10.1007/s12011-020-02499-9
91. Jia X, Zhang L, Zhao J, Ren M, Li Z, Wang J, et al. Associations between endocrine-disrupting heavy metals in maternal hair and gestational diabetes mellitus: a nested case-control study in China. Environ Int. (2021) 157:106770. doi: 10.1016/j.envint.2021.106770
92. Zhu G, Zheng T, Xia C, Qi L, Papandonatos GD, Ming Y, et al. Plasma levels of trace element status in early pregnancy and the risk of gestational diabetes mellitus: a nested case-control study. J Trace Elem Med Biol. (2021) 68:126829. doi: 10.1016/j.jtemb.2021.126829
93. Zhang Q, Li X, Liu X, Dong M, Xiao J, Wang J, et al. Association between maternal antimony exposure and risk of gestational diabetes mellitus: a birth cohort study. Chemosphere. (2020) 246:125732. doi: 10.1016/j.chemosphere.2019.125732
94. Lian S, Zhang T, Yu Y, Zhang B. Relationship of circulating copper level with gestational diabetes mellitus: a meta-analysis and systemic review. Biol Trace Elem Res. (2021) 199:4396–409. doi: 10.1007/s12011-020-02566-1
95. Tatsuta N, Iwai-Shimada M, Nakayama SF, Iwama N, Metoki H, Arima T, et al. Association between whole blood metallic elements concentrations and gestational diabetes mellitus in Japanese women: the Japan environment and children's study. Environ Res. (2022) 212:113231. doi: 10.1016/j.envres.2022.113231
96. Zhou M, Peng L, Wang J, Cao R, Ou Z, Fang Y. Cadmium exposure and the risk of GDM: evidence emerging from the systematic review and meta-analysis. Environ Sci Pollut Res Int. (2022) 29:77253–74. doi: 10.1007/s11356-022-21171-4
97. Vekic J, Zeljkovic A, Stefanovic A, Jelic-Ivanovic Z, Spasojevic-Kalimanovska V. Obesity and dyslipidemia. Metabolism. (2019) 92:71–81. doi: 10.1016/j.metabol.2018.11.005
98. Pan XF, Wang L, Pan A. Epidemiology and determinants of obesity in China. Lancet Diabetes Endocrinol. (2021) 9:373–92. doi: 10.1016/S2213-8587(21)00045-0
99. García OP, Long KZ, Rosado JL. Impact of micronutrient deficiencies on obesity. Nutr Rev. (2009) 67:559–72. doi: 10.1111/j.1753-4887.2009.00228.x
100. Di Martino G, Matera MG, De Martino B, Vacca C, Di Martino S, Rossi F. Relationship between zinc and obesity. J Med. (1993) 24:177–83.
101. Zafon C, Lecube A, Simó R. Iron in obesity. An ancient micronutrient for a modern disease. Obes Rev. (2010) 11:322–8. doi: 10.1111/j.1467-789X.2009.00638.x
102. Lima SC, Arrais RF, Sales CH, Almeida MG, de Sena KC, Oliveira VT, et al. Assessment of copper and lipid profile in obese children and adolescents. Biol Trace Elem Res. (2006) 114:19–29. doi: 10.1385/BTER:114:1:19
103. Tascilar ME, Ozgen IT, Abaci A, Serdar M, Aykut O. Trace elements in obese Turkish children. Biol Trace Elem Res. (2011) 143:188–95. doi: 10.1007/s12011-010-8878-8
104. Yerlikaya FH, Toker A, Aribaş A. Serum trace elements in obese women with or without diabetes. Indian J Med Res. (2013) 137:339–45.
105. Suliburska J, Cofta S, Gajewska E, Kalmus G, Sobieska M, Samborski W, et al. The evaluation of selected serum mineral concentrations and their association with insulin resistance in obese adolescents. Eur Rev Med Pharmacol Sci. (2013) 17:2396–400.
106. Cayir A, Doneray H, Kurt N, Orbak Z, Kaya A, Turan MI, et al. Thyroid functions and trace elements in pediatric patients with exogenous obesity. Biol Trace Elem Res. (2014) 157:95–100. doi: 10.1007/s12011-013-9880-8
107. Azab SF, Saleh SH, Elsaeed WF, Elshafie MA, Sherief LM, Esh AM. Serum trace elements in obese Egyptian children: a case-control study. Ital J Pediatr. (2014) 40:20. doi: 10.1186/1824-7288-40-20
108. Fatani SH, Saleh SA, Adly HM, Abdulkhaliq AA. Trace element alterations in the hair of diabetic and obese women. Biol Trace Elem Res. (2016) 174:32–9. doi: 10.1007/s12011-016-0691-6
109. Shao W, Liu Q, He X, Liu H, Gu A, Jiang Z. Association between level of urinary trace heavy metals and obesity among children aged 6-19 years: NHANES 1999-2011. Environ Sci Pollut Res Int. (2017) 24:11573–81. doi: 10.1007/s11356-017-8803-1
110. Luciano-Mateo F, Cabré N, Nadal M, García-Heredia A, Baiges-Gaya G, Hernández-Aguilera A, et al. Serum concentrations of trace elements and their relationships with paraoxonase-1 in morbidly obese women. J Trace Elem Med Biol. (2018) 48:8–15. doi: 10.1016/j.jtemb.2018.02.023
111. Skalnaya MG, Skalny AV, Grabeklis AR, Serebryansky EP, Demidov VA, Tinkov AA. Hair trace elements in overweight and obese adults in association with metabolic parameters. Biol Trace Elem Res. (2018) 186:12–20. doi: 10.1007/s12011-018-1282-5
112. Adnan MT, Amin MN, Uddin MG, Hussain MS, Sarwar MS, Hossain MK, et al. Increased concentration of serum MDA, decreased antioxidants and altered trace elements and macro-minerals are linked to obesity among Bangladeshi population. Diabetes Metab Syndr. (2019) 13:933–8. doi: 10.1016/j.dsx.2018.12.022
113. Xu Y, Wei Y, Long T, Wang R, Li Z, Yu C, et al. Association between urinary metals levels and metabolic phenotypes in overweight and obese individuals. Chemosphere. (2020) 254:126763. doi: 10.1016/j.chemosphere.2020.126763
114. Amin MN, Siddiqui SA, Uddin MG, Ibrahim M, Uddin SMN, Adnan MT, et al. Increased oxidative stress, altered trace elements, and macro-minerals are associated with female obesity. Biol Trace Elem Res. (2020) 197:384–93. doi: 10.1007/s12011-019-02002-z
115. Tinkov AA, Skalnaya MG, Ajsuvakova OP, Serebryansky EP, Chao JC, Aschner M, et al. Selenium, zinc, chromium, and vanadium levels in serum, hair, and urine samples of obese adults assessed by inductively coupled plasma mass spectrometry. Biol Trace Elem Res. (2021) 199:490–9. doi: 10.1007/s12011-020-02177-w
116. Tinkov AA, Bogdański P, Skrypnik D, Skrypnik K, Skalny AV, Aaseth J, et al. Trace element and mineral levels in serum, hair, and urine of obese women in relation to body composition, blood pressure, lipid profile, and insulin resistance. Biomolecules. (2021) 11:689. doi: 10.3390/biom11050689
117. Nasab H, Rajabi S, Eghbalian M, Malakootian M, Hashemi M, Mahmoudi-Moghaddam H. Association of As, Pb, Cr, and Zn urinary heavy metals levels with predictive indicators of cardiovascular disease and obesity in children and adolescents. Chemosphere. (2022) 294:133664. doi: 10.1016/j.chemosphere.2022.133664
118. Skalny AV, Chang JS, Bobrovnitsky IP, Kopylov PY, Skalnaya MG, Huang SY, et al. Relationship between elevated hair mercury levels, essential element status, and metabolic profile in overweight and obese adults. Biol Trace Elem Res. (2021) 199:2874–81. doi: 10.1007/s12011-020-02430-2
119. Yang H, Liu CN, Wolf RM, Ralle M, Dev S, Pierson H, et al. Obesity is associated with copper elevation in serum and tissues. Metallomics. (2019) 11:1363–71. doi: 10.1039/C9MT00148D
120. Ge W, Liu W, Liu G. The relationships between serum copper levels and overweight/total obesity and central obesity in children and adolescents aged 6-18 years. J Trace Elem Med Biol. (2020) 61:126557. doi: 10.1016/j.jtemb.2020.126557
121. Rios-Lugo MJ, Madrigal-Arellano C, Gaytán-Hernández D, Hernández-Mendoza H, Romero-Guzmán ET. Association of serum zinc levels in overweight and obesity. Biol Trace Elem Res. (2020) 198:51–7. doi: 10.1007/s12011-020-02060-8
122. Hernández-Mendoza H, Martínez-Navarro I, Hernández-Ochoa E, Espinoza-Ruiz M, Lugo-Trampe A, Trujillo-Murillo KDC, et al. Serum zinc levels are associated with obesity and low-density lipoprotein cholesterol in Mexican adults. J Trace Elem Med Biol. (2022) 73:127002. doi: 10.1016/j.jtemb.2022.127002
123. Lokvencová L, Zvěrina O, Kuta J. Different trends of Cr, Fe and Zn contents in hair between obese, overweight and normal-weight men. Cent Eur J Public Health. (2021) 29:301–4. doi: 10.21101/cejph.a6912
124. Xu R, Chen C, Zhou Y, Zhang X, Wan Y. Fingernail selenium levels in relation to the risk of obesity in Chinese children: a cross-sectional study. Medicine (Baltimore). (2018) 97:e0027. doi: 10.1097/MD.0000000000010027
125. Tinkov AA, Skalnaya MG, Aaseth J, Ajsuvakova OP, Aschner M, Skalny AV. Aluminium levels in hair and urine are associated with overweight and obesity in a non-occupationally exposed population. J Trace Elem Med Biol. (2019) 56:139–45. doi: 10.1016/j.jtemb.2019.08.005
126. Jeon J, Morris JS, Park K. Toenail mercury levels positively correlate with obesity and abdominal obesity among Korean adults. J Trace Elem Med Biol. (2021) 64:126678. doi: 10.1016/j.jtemb.2020.126678
127. Hernández-Mendoza H, Rios-Lugo MJ, Álvarez-Loredo HE, Romero-Guzmán ET, Gaytán-Hernández D, Martínez-Navarro I, et al. Serum lead levels and its association with overweight and obesity. J Trace Elem Med Biol. (2022) 72:126984. doi: 10.1016/j.jtemb.2022.126984
128. Hernández-Mendoza H, Álvarez-Loredo HE, Romero-Guzmán ET, Gaytán-Hernández D, Chang-Rueda C, Martínez-Navarro I, et al. Relationship between serum levels of arsenic, cadmium, and mercury and body mass index and fasting plasma glucose in a Mexican adult population. Biol Trace Elem Res. (2022) 200:4916–23. doi: 10.1007/s12011-021-03081-7
129. Zohal M, Jam-Ashkezari S, Namiranian N, Moosavi A, Ghadiri-Anari A. Association between selected trace elements and body mass index and waist circumference: a cross sectional study. Diabetes Metab Syndr. (2019) 13:1293–7. doi: 10.1016/j.dsx.2019.01.019
130. Kim HN, Song SW. Concentrations of chromium, selenium, and copper in the hair of viscerally obese adults are associated with insulin resistance. Biol Trace Elem Res. (2014) 158:152–7. doi: 10.1007/s12011-014-9934-6
131. Jaksic M, Martinovic M, Gligorovic-Barhanovic N, Vujacic A, Djurovic D, Nedovic-Vukovic M. Association between inflammation, oxidative stress, vitamin D, copper and zinc with pre-obesity and obesity in school children from the city of podgorica, montenegro. J Pediatr Endocrinol Metab. (2019) 32:951–7. doi: 10.1515/jpem-2019-0086
132. Aguilera CM, Olza J, Gil A. Genetic susceptibility to obesity and metabolic syndrome in childhood. Nutr Hosp. (2013) 28 (Suppl. 5):44–55. doi: 10.3305/nh.2013.28.sup5.6917
133. Robberecht H, Bruyne T, Hermans N. Biomarkers of the metabolic syndrome: influence of minerals, oligo- and trace elements. J Trace Elem Med Biol. (2017) 43:23–8. doi: 10.1016/j.jtemb.2016.10.005
134. Datz C, Felder TK, Niederseer D, Aigner E. Iron homeostasis in the metabolic syndrome. Eur J Clin Invest. (2013) 43:215–24. doi: 10.1111/eci.12032
135. Panchal SK, Wanyonyi S, Brown L. Selenium, vanadium, and chromium as micronutrients to improve metabolic syndrome. Curr Hypertens Rep. (2017) 19:10. doi: 10.1007/s11906-017-0701-x
136. Shi Y, Zhong H, Pang L. Maternal micronutrient disturbance as risks of offspring metabolic syndrome. J Trace Elem Med Biol. (2022) 75:127097. doi: 10.1016/j.jtemb.2022.127097
137. Bozzini C, Girelli D, Olivieri O, Martinelli N, Bassi A, De Matteis G, et al. Prevalence of body iron excess in the metabolic syndrome. Diabetes Care. (2005) 28:2061–3. doi: 10.2337/diacare.28.8.2061
138. Aguilar MV, Saavedra P, Arrieta FJ, Mateos CJ, González MJ, Meseguer I, et al. Plasma mineral content in type-2 diabetic patients and their association with the metabolic syndrome. Ann Nutr Metab. (2007) 51:402–6. doi: 10.1159/000108108
139. Obeid O, Elfakhani M, Hlais S, Iskandar M, Batal M, Mouneimne Y, et al. Plasma copper, zinc, and selenium levels and correlates with metabolic syndrome components of lebanese adults. Biol Trace Elem Res. (2008) 123:58–65. doi: 10.1007/s12011-008-8112-0
140. Seo JA, Song SW, Han K, Lee KJ, Kim HN. The associations between serum zinc levels and metabolic syndrome in the Korean population: findings from the 2010 Korean national health and nutrition examination survey. PLoS ONE. (2014) 9:e105990. doi: 10.1371/journal.pone.0105990
141. Tajaddini MH, Keikha M, Razzazzadeh A, Kelishadi R. A systematic review on the association of serum selenium and metabolic syndrome. J Res Med Sci. (2015) 20:782–9. doi: 10.4103/1735-1995.168403
142. Choi WS, Kim SH, Chung JH. Relationships of hair mineral concentrations with insulin resistance in metabolic syndrome. Biol Trace Elem Res. (2014) 158:323–9. doi: 10.1007/s12011-014-9946-2
143. Rotter I, Kosik-Bogacka D, Dołegowska B, Safranow K, Lubkowska A, Laszczyńska M. Relationship between the concentrations of heavy metals and bioelements in aging men with metabolic syndrome. Int J Environ Res Public Health. (2015) 12:3944–61. doi: 10.3390/ijerph120403944
144. Guo X, Yang Q, Zhang W, Chen Y, Ren J, Gao A. Associations of blood levels of trace elements and heavy metals with metabolic syndrome in Chinese male adults with microRNA as mediators involved. Environ Pollut. (2019) 248:66–73. doi: 10.1016/j.envpol.2019.02.015
145. Wen WL, Wang CW, Wu DW, Chen SC, Hung CH, Kuo CH. Associations of heavy metals with metabolic syndrome and anthropometric indices. Nutrients. (2020) 12:2666. doi: 10.3390/nu12092666
146. Huang M, Chen J, Yan G, Yang Y, Luo D, Chen X, et al. Plasma titanium level is positively associated with metabolic syndrome: a survey in China's heavy metal polluted regions. Ecotoxicol Environ Saf. (2021) 208:111435. doi: 10.1016/j.ecoenv.2020.111435
147. Wang X, Karvonen-Gutierrez CA, Herman WH, Mukherjee B, Park SK. Metals and risk of incident metabolic syndrome in a prospective cohort of midlife women in the United States. Environ Res. (2022) 210:112976. doi: 10.1016/j.envres.2022.112976
148. Feng X, Li L, Huang L, Zhang H, Mo Z, Yang X. Associations between serum multiple metals exposures and metabolic syndrome: a longitudinal cohort study. Biol Trace Elem Res. (2021) 199:2444–55. doi: 10.1007/s12011-020-02371-w
149. Ngu YJ, Skalny AV, Tinkov AA, Tsai CS, Chang CC, Chuang YK, et al. Association between essential and non-essential metals, body composition, and metabolic syndrome in adults. Biol Trace Elem Res. (2022) 200:4903–15. doi: 10.1007/s12011-021-03077-3
150. Huang S, Zhong D, Lv Z, Cheng J, Zou X, Wang T, et al. Associations of multiple plasma metals with the risk of metabolic syndrome: a cross-sectional study in the mid-aged and older population of China. Ecotoxicol Environ Saf. (2022) 231:113183. doi: 10.1016/j.ecoenv.2022.113183
151. Piuri G, Zocchi M, Della Porta M, Ficara V, Manoni M, Zuccotti GV, et al. Magnesium in obesity, metabolic syndrome, and type 2 diabetes. Nutrients. (2021) 13:320. doi: 10.3390/nu13020320
152. Ruz M, Carrasco F, Rojas P, Basfi-Fer K, Hernández MC, Pérez A. Nutritional effects of zinc on metabolic syndrome and type 2 diabetes: mechanisms and main findings in human studies. Biol Trace Elem Res. (2019) 188:177–88. doi: 10.1007/s12011-018-1611-8
153. Chung JH, Yum KS. Correlation of hair mineral concentrations with insulin resistance in Korean males. Biol Trace Elem Res. (2012) 150:26–30. doi: 10.1007/s12011-012-9474-x
154. Zhang H, Man Q, Song P, Li S, Liu X, Wang L, et al. Association of whole blood copper, magnesium and zinc levels with metabolic syndrome components in 6-12-year-old rural Chinese children: 2010-2012 China national nutrition and health survey. Nutr Metab. (2021) 18:67. doi: 10.1186/s12986-021-00593-w
155. Kelishadi R, Ataei E, Motlagh ME, Yazdi M, Tajaddini MH, Heshmat R, et al. Association of serum concentrations of magnesium and some trace elements with cardiometabolic risk factors and liver enzymes in adolescents: the CASPIAN-III study. Biol Trace Elem Res. (2015) 163:97–102. doi: 10.1007/s12011-014-0180-8
156. Yuan Z, Xu X, Ye H, Jin L, Zhang X, Zhu Y. High levels of plasma selenium are associated with metabolic syndrome and elevated fasting plasma glucose in a Chinese population: a case-control study. J Trace Elem Med Biol. (2015) 32:189–94. doi: 10.1016/j.jtemb.2015.07.009
157. La SA, Lee JY, Kim DH, Song EL, Park JH, Ju SY. Low magnesium levels in adults with metabolic syndrome: a meta-analysis. Biol Trace Elem Res. (2016) 170:33–42. doi: 10.1007/s12011-015-0446-9
158. Freitas EP, Cunha AT, Aquino SL, Pedrosa LF, Lima SC, Lima JG, et al. Zinc status biomarkers and cardiometabolic risk factors in metabolic syndrome: a case control study. Nutrients. (2017) 9:175. doi: 10.3390/nu9020175
159. Yary T, Virtanen JK, Ruusunen A, Tuomainen TP, Voutilainen S. Association between serum zinc and later development of metabolic syndrome in middle aged and older men: the kuopio ischaemic heart disease risk factor study. Nutrition. (2017) 37:43–7. doi: 10.1016/j.nut.2016.09.004
160. Lu CW, Chang HH, Yang KC, Chiang CH, Yao CA, Huang KC. Gender differences with dose(-)response relationship between serum selenium levels and metabolic syndrome-a case-control study. Nutrients. (2019) 11:477. doi: 10.3390/nu11020477
161. Fang C, Wu W, Gu X, Dai S, Zhou Q, Deng H, et al. Association of serum copper, zinc and selenium levels with risk of metabolic syndrome: a nested case-control study of middle-aged and older Chinese adults. J Trace Elem Med Biol. (2019) 52:209–15. doi: 10.1016/j.jtemb.2018.12.017
162. Chen S, Zhou L, Guo Q, Fang C, Wang M, Peng X, et al. Association of plasma chromium with metabolic syndrome among Chinese adults: a case-control study. Nutr J. (2020) 19:107. doi: 10.1186/s12937-020-00625-w
163. Lu CW, Lee YC, Kuo CS, Chiang CH, Chang HH, Huang KC. Association of serum levels of zinc, copper, and iron with risk of metabolic syndrome. Nutrients. (2021) 13:548. doi: 10.3390/nu13020548
164. Ayoub N, Mantash H, Dhaini HR, Mourad A, Hneino M, Daher Z. Serum cadmium levels and risk of metabolic syndrome: a cross-sectional study. Biol Trace Elem Res. (2021) 199:3625–33. doi: 10.1007/s12011-020-02502-3
165. Arnaud J, de Lorgeril M, Akbaraly T, Salen P, Arnout J, Cappuccio FP, et al. Gender differences in copper, zinc and selenium status in diabetic-free metabolic syndrome European population - the IMMIDIET study. Nutr Metab Cardiovasc Dis. (2012) 22:517–24. doi: 10.1016/j.numecd.2010.09.005
166. Park K, Seo E. Association between toenail mercury and metabolic syndrome is modified by selenium. Nutrients. (2016) 8:424. doi: 10.3390/nu8070424
167. Byrne CD, Targher G. NAFLD: a multisystem disease. J Hepatol. (2015) 62:S47–64. doi: 10.1016/j.jhep.2014.12.012
168. Ekstedt M, Nasr P, Kechagias S. Natural history of NAFLD/NASH. Curr Hepatol Rep. (2017) 16:391–7. doi: 10.1007/s11901-017-0378-2
169. Ma C, Han L, Zhu Z, Heng Pang C, Pan G. Mineral metabolism and ferroptosis in non-alcoholic fatty liver diseases. Biochem Pharmacol. (2022) 205:115242. doi: 10.1016/j.bcp.2022.115242
170. Feldman A, Aigner E, Weghuber D, Paulmichl K. The potential role of iron and copper in pediatric obesity and nonalcoholic fatty liver disease. Biomed Res Int. (2015) 2015:287401. doi: 10.1155/2015/287401
171. Eslam M, Sanyal AJ, George J, International Consensus P. MAFLD: a consensus-driven proposed nomenclature for metabolic associated fatty liver disease. Gastroenterology. (2020) 158:1999–2014 e1. doi: 10.1053/j.gastro.2019.11.312
172. Fargion S, Mattioli M, Fracanzani AL, Sampietro M, Tavazzi D, Fociani P, et al. Hyperferritinemia, iron overload, and multiple metabolic alterations identify patients at risk for nonalcoholic steatohepatitis. Am J Gastroenterol. (2001) 96:2448–55. doi: 10.1111/j.1572-0241.2001.04052.x
173. Aigner E, Theurl I, Theurl M, Lederer D, Haufe H, Dietze O, et al. Pathways underlying iron accumulation in human nonalcoholic fatty liver disease. Am J Clin Nutr. (2008) 87:1374–83. doi: 10.1093/ajcn/87.5.1374
174. Aigner E, Theurl I, Haufe H, Seifert M, Hohla F, Scharinger L, et al. Copper availability contributes to iron perturbations in human nonalcoholic fatty liver disease. Gastroenterology. (2008) 135:680–8. doi: 10.1053/j.gastro.2008.04.007
175. Aigner E, Strasser M, Haufe H, Sonnweber T, Hohla F, Stadlmayr A, et al. A role for low hepatic copper concentrations in nonalcoholic fatty liver disease. Am J Gastroenterol. (2010) 105:1978–85. doi: 10.1038/ajg.2010.170
176. Sorrentino P, D'Angelo S, Ferbo U, Micheli P, Bracigliano A, Vecchione R. Liver iron excess in patients with hepatocellular carcinoma developed on non-alcoholic steato-hepatitis. J Hepatol. (2009) 50:351–7. doi: 10.1016/j.jhep.2008.09.011
177. Nobili V, Siotto M, Bedogni G, Ravà L, Pietrobattista A, Panera N, et al. Levels of serum ceruloplasmin associate with pediatric nonalcoholic fatty liver disease. J Pediatr Gastroenterol Nutr. (2013) 56:370–5. doi: 10.1097/MPG.0b013e31827aced4
178. Kowdley KV, Belt P, Wilson LA, Yeh MM, Neuschwander-Tetri BA, Chalasani N, et al. Serum ferritin is an independent predictor of histologic severity and advanced fibrosis in patients with nonalcoholic fatty liver disease. Hepatology. (2012) 55:77–85. doi: 10.1002/hep.24706
179. Moya D, Baker SS, Liu W, Garrick M, Kozielski R, Baker RD, et al. Novel pathway for iron deficiency in pediatric non-alcoholic steatohepatitis. Clin Nutr. (2015) 34:549–56. doi: 10.1016/j.clnu.2014.06.011
180. Asprouli E, Kalafati IP, Sakellari A, Karavoltsos S, Vlachogiannakos J, Revenas K, et al. Evaluation of plasma trace elements in different stages of nonalcoholic fatty liver disease. Biol Trace Elem Res. (2019) 188:326–33. doi: 10.1007/s12011-018-1432-9
181. Li L, Huang L, Huang S, Luo X, Zhang H, Mo Z, et al. Non-linear association of serum molybdenum and linear association of serum zinc with nonalcoholic fatty liver disease: multiple-exposure and Mendelian randomization approach. Sci Total Environ. (2020) 720:137655. doi: 10.1016/j.scitotenv.2020.137655
182. Sabrina N, Bai CH, Chang CC, Chien YW, Chen JR, Chang JS. Serum iron: ferritin ratio predicts healthy body composition and reduced risk of severe fatty liver in young adult women. Nutrients. (2017) 9:833. doi: 10.3390/nu9080833
183. Eder SK, Feldman A, Strebinger G, Kemnitz J, Zandanell S, Niederseer D, et al. Mesenchymal iron deposition is associated with adverse long-term outcome in non-alcoholic fatty liver disease. Liver Int. (2020) 40:1872–82. doi: 10.1111/liv.14503
184. Ma B, Sun H, Zhu B, Wang S, Du L, Wang X, et al. Hepatic steatosis is associated with elevated serum iron in patients with obesity and improves after laparoscopic sleeve gastrectomy. Obes Facts. (2021) 14:64–71. doi: 10.1159/000511736
185. Yang HH, Chen GC, Li DM, Lan L, Chen LH, Xu JY, et al. Serum iron and risk of nonalcoholic fatty liver disease and advanced hepatic fibrosis in US adults. Sci Rep. (2021) 11:10387. doi: 10.1038/s41598-021-89991-x
186. Yu YC, Luu HN, Wang R, Thomas CE, Glynn NW, Youk AO, et al. Serum biomarkers of iron status and risk of hepatocellular carcinoma development in patients with nonalcoholic fatty liver disease. Cancer Epidemiol Biomarkers Prev. (2022) 31:230–5. doi: 10.1158/1055-9965.EPI-21-0754
187. Mendoza M, Caltharp S, Song M, Collin L, Konomi JV, McClain CJ, et al. Low hepatic tissue copper in pediatric nonalcoholic fatty liver disease. J Pediatr Gastroenterol Nutr. (2017) 65:89–92. doi: 10.1097/MPG.0000000000001571
188. Lee SH, Kim MJ, Kim YS, Chun H, Won BY, Lee JH, et al. Low hair copper concentration is related to a high risk of nonalcoholic fatty liver disease in adults. J Trace Elem Med Biol. (2018) 50:28–33. doi: 10.1016/j.jtemb.2018.06.001
189. Zhang H, Zheng KI, Zhu PW, Chen SD, Li G, Ma HL, et al. Lower serum copper concentrations are associated with higher prevalence of nonalcoholic steatohepatitis: a matched case-control study. Eur J Gastroenterol Hepatol. (2022) 34:838–43. doi: 10.1097/MEG.0000000000002392
190. Lan Y, Wu S, Wang Y, Chen S, Liao W, Zhang X, et al. Association between blood copper and nonalcoholic fatty liver disease according to sex. Clin Nutr. (2021) 40:2045–52. doi: 10.1016/j.clnu.2020.09.026
191. Nasr P, Ignatova S, Lundberg P, Kechagias S, Ekstedt M. Low hepatic manganese concentrations in patients with hepatic steatosis—a cohort study of copper, iron and manganese in liver biopsies. J Trace Elem Med Biol. (2021) 67:126772. doi: 10.1016/j.jtemb.2021.126772
192. Zhang D, Wu S, Lan Y, Chen S, Wang Y, Sun Y, et al. Blood manganese and nonalcoholic fatty liver disease: a cohort-based case-control study. Chemosphere. (2022) 287:132316. doi: 10.1016/j.chemosphere.2021.132316
193. Van Campenhout S, Hastuti A, Lefere S, Van Vlierberghe H, Vanhaecke F, Costas-Rodriguez M, et al. Lighter serum copper isotopic composition in patients with early non-alcoholic fatty liver disease. BMC Res Notes. (2020) 13:225. doi: 10.1186/s13104-020-05069-3
194. Ito T, Ishigami M, Ishizu Y, Kuzuya T, Honda T, Ishikawa T, et al. Serum nutritional markers as prognostic factors for hepatic and extrahepatic carcinogenesis in japanese patients with nonalcoholic fatty liver disease. Nutr Cancer. (2020) 72:884–91. doi: 10.1080/01635581.2019.1653474
195. Akdas S, Yazihan N. Serum zinc level and dietary zinc intake status in non-alcoholic fatty liver disease: a meta-analysis and systematic review. Hepatol Forum. (2020) 1:59–67. doi: 10.14744/hf.2020.2020.0006
196. Kim MC, Lee JI, Kim JH, Kim HJ, Cho YK, Jeon WK, et al. Serum zinc level and hepatic fibrosis in patients with nonalcoholic fatty liver disease. PLoS ONE. (2020) 15:e0240195. doi: 10.1371/journal.pone.0240195
197. Chen SD, Zhang H, Rios RS, Li YY, Zhu PW, Jin Y, et al. J-shaped relationship between serum zinc levels and the severity of hepatic necro-inflammation in patients with MAFLD. Nutr Metab Cardiovasc Dis. (2022) 32:1259–65. doi: 10.1016/j.numecd.2022.01.035
198. Polyzos SA, Kountouras J, Goulas A, Duntas L. Selenium and selenoprotein P in nonalcoholic fatty liver disease. Hormones. (2020) 19:61–72. doi: 10.1007/s42000-019-00127-3
199. Yang Z, Yan C, Liu G, Niu Y, Zhang W, Lu S, et al. Plasma selenium levels and nonalcoholic fatty liver disease in Chinese adults: a cross-sectional analysis. Sci Rep. (2016) 6:37288. doi: 10.1038/srep37288
200. Reja M, Makar M, Visaria A, Marino D, Rustgi V. Increased serum selenium levels are associated with reduced risk of advanced liver fibrosis and all-cause mortality in NAFLD patients: national health and nutrition examination survey (NHANES) III. Ann Hepatol. (2020) 19:635–40. doi: 10.1016/j.aohep.2020.07.006
201. Spaur M, Nigra AE, Sanchez TR, Navas-Acien A, Lazo M, Wu HC. Association of blood manganese, selenium with steatosis, fibrosis in the national health and nutrition examination survey, 2017-18. Environ Res. (2022) 213:113647. doi: 10.1016/j.envres.2022.113647
202. Zhai H, Chen C, Wang N, Chen Y, Nie X, Han B, et al. Blood lead level is associated with non-alcoholic fatty liver disease in the Yangtze River Delta region of China in the context of rapid urbanization. Environ Health. (2017) 16:93. doi: 10.1186/s12940-017-0304-7
203. Chen R, Xu Y, Xu C, Shu Y, Ma S, Lu C, et al. Associations between mercury exposure and the risk of nonalcoholic fatty liver disease (NAFLD) in US adolescents. Environ Sci Pollut Res Int. (2019) 26:31384–91. doi: 10.1007/s11356-019-06224-5
204. Reja D, Makar M, Visaria A, Karanfilian B, Rustgi V. Blood lead level is associated with advanced liver fibrosis in patients with non-alcoholic fatty liver disease: a nationwide survey (NHANES 2011-2016). Ann Hepatol. (2020) 19:404–10. doi: 10.1016/j.aohep.2020.03.006
205. Han S, Sung GH, Lee S, Han KJ, Han HJ. Serum cadmium is associated with hepatic steatosis and fibrosis: Korean national health and nutrition examination survey data IV-VII. Medicine. (2022) 101:e28559. doi: 10.1097/MD.0000000000028559
206. Wan H, Wang Y, Zhang H, Zhang K, Chen Y, Chen C, et al. Chronic lead exposure induces fatty liver disease associated with the variations of gut microbiota. Ecotoxicol Environ Saf. (2022) 232:113257. doi: 10.1016/j.ecoenv.2022.113257
207. Yang C, Li Y, Ding R, Xing H, Wang R, Zhang M. Lead exposure as a causative factor for metabolic associated fatty liver disease (MAFLD) and a lead exposure related nomogram for MAFLD prevalence. Front Public Health. (2022) 10:1000403. doi: 10.3389/fpubh.2022.1000403
208. Liu C, Liu W, Zhang G, Wang Y, Jiang J, Yang Z, et al. Conjunctional relationship between serum uric acid and serum nickel with non-alcoholic fatty liver disease in men: a cross-sectional study. Int J Environ Res Public Health. (2022) 19:6424. doi: 10.3390/ijerph19116424
209. Shin JY, Kim MJ, Kim ES, Mo EY, Moon SD, Han JH, et al. Association between serum calcium and phosphorus concentrations with non-alcoholic fatty liver disease in Korean population. J Gastroenterol Hepatol. (2015) 30:733–41. doi: 10.1111/jgh.12832
210. Nelson RH. Hyperlipidemia as a risk factor for cardiovascular disease. Prim Care. (2013) 40:195–211. doi: 10.1016/j.pop.2012.11.003
211. Narang RK, Dalbeth N. Pathophysiology of gout. Semin Nephrol. (2020) 40:550–63. doi: 10.1016/j.semnephrol.2020.12.001
212. Borghi C, Agabiti-Rosei E, Johnson RJ, Kielstein JT, Lurbe E, Mancia G, et al. Hyperuricaemia and gout in cardiovascular, metabolic and kidney disease. Eur J Intern Med. (2020) 80:1–11. doi: 10.1016/j.ejim.2020.07.006
213. Ezeh KJ, Ezeudemba O. Hyperlipidemia: a review of the novel methods for the management of lipids. Cureus. (2021) 13:e16412. doi: 10.7759/cureus.16412
214. Nishizawa H, Maeda N, Shimomura I. Impact of hyperuricemia on chronic kidney disease and atherosclerotic cardiovascular disease. Hypertens Res. (2022) 45:635–40. doi: 10.1038/s41440-021-00840-w
215. Thuillier-Juteau Y, Jaudon MC, Clavel JP, Delattre J, Galli A. Serum zinc and copper in hypercholesterolemia. Pathol Biol. (1987) 35:387–90.
216. Mahdi GS. Chromium deficiency might contribute to insulin resistance, type 2 diabetes mellitus, dyslipidaemia, and atherosclerosis. Diabet Med. (1996) 13:389–90. doi: 10.1002/(SICI)1096-9136(199604)13:4<389::AID-DIA65>3.0.CO;2-J
217. Jackson GE, Blewet R, Rodgers AL, Wood L, Jacobs P. Trace metal excretion in patients with homozygous hypercholesterolaemia. J Trace Elem Med Biol. (1999) 13:62–7. doi: 10.1016/S0946-672X(99)80025-X
218. Abiaka C, Olusi S, Al-Awadhi A. Serum microminerals and the indices of lipid metabolism in an apparently healthy population. J Clin Lab Anal. (2003) 17:61–5. doi: 10.1002/jcla.10069
219. Lee O, Moon J, Chung Y. The relationship between serum selenium levels and lipid profiles in adult women. J Nutr Sci Vitaminol. (2003) 49:397–404. doi: 10.3177/jnsv.49.397
220. Bunce GE. Hypercholesterolemia of copper deficiency is linked to glutathione metabolism and regulation of hepatic HMG-CoA reductase. Nutr Rev. (1993) 51:305–7. doi: 10.1111/j.1753-4887.1993.tb03061.x
221. Fields M, Lewis CG. Hepatic iron overload may contribute to hypertriglyceridemia and hypercholesterolemia in copper-deficient rats. Metabolism. (1997) 46:377–81. doi: 10.1016/S0026-0495(97)90051-2
222. Mateo-Gallego R, Solanas-Barca M, Burillo E, Cenarro A, Marques-Lopes I, Civeira F. Iron deposits and dietary patterns in familial combined hyperlipidemia and familial hypertriglyceridemia. J Physiol Biochem. (2010) 66:229–36. doi: 10.1007/s13105-010-0029-3
223. Ghayour-Mobarhan M, Lamb DJ, Taylor A, Vaidya N, Livingstone C, Wang T, et al. Effect of statin therapy on serum trace element status in dyslipidaemic subjects. J Trace Elem Med Biol. (2005) 19:61–7. doi: 10.1016/j.jtemb.2005.06.003
224. Yerlikaya FH, Can U, Alpaydin MS, Aribas A. The relationship between plasma microRNAs and serum trace elements levels in primary hyperlipidemia. Bratisl Lek Listy. (2019) 120:344–8. doi: 10.4149/BLL_2019_056
225. Luo X, Liu Z, Ge X, Huang S, Zhou Y, Li D, et al. High manganese exposure decreased the risk of high triglycerides in workers: a cross-sectional study. BMC Public Health. (2020) 20:874. doi: 10.1186/s12889-020-09011-x
226. Cho HW, Kim SH, Park MJ. An association of blood mercury levels and hypercholesterolemia among Korean adolescents. Sci Total Environ. (2020) 709:135965. doi: 10.1016/j.scitotenv.2019.135965
227. Shun CH, Yuan TH, Hung SH, Yeh YP, Chen YH, Chan CC. Assessment of the hyperlipidemia risk for residents exposed to potential emitted metals in the vicinity of a petrochemical complex. Environ Sci Pollut Res Int. (2021) 28:27966–75. doi: 10.1007/s11356-021-12642-1
228. Zhang Y, Liu W, Zhang W, Cheng R, Tan A, Shen S, et al. Association between blood lead levels and hyperlipidemiais: results from the NHANES (1999-2018). Front Public Health. (2022) 10:981749. doi: 10.3389/fpubh.2022.981749
229. Barragán R, Llopis J, Portolés O, Sorlí JV, Coltell O, Rivas-García L, et al. Influence of demographic and lifestyle variables on plasma magnesium concentrations and their associations with cardiovascular risk factors in a mediterranean population. Nutrients. (2020) 12:1018. doi: 10.3390/nu12041018
230. Barragán R, Sánchez-González C, Aranda P, Sorlí JV, Asensio EM, Portolés O, et al. Single and combined associations of plasma and urine essential trace elements (Zn, Cu, Se, and Mn) with cardiovascular risk factors in a mediterranean population. Antioxidants. (2022) 11:1991. doi: 10.3390/antiox11101991
231. Shadick NA, Kim R, Weiss S, Liang MH, Sparrow D, Hu H. Effect of low level lead exposure on hyperuricemia and gout among middle aged and elderly men: the normative aging study. J Rheumatol. (2000) 27:1708–12.
232. Ghio AJ, Ford ES, Kennedy TP, Hoidal JR. The association between serum ferritin and uric acid in humans. Free Radic Res. (2005) 39:337–42. doi: 10.1080/10715760400026088
233. Dai H, Huang Z, Deng Q, Li Y, Xiao T, Ning X, et al. The effects of lead exposure on serum uric acid and hyperuricemia in Chinese adults: a cross-sectional study. Int J Environ Res Public Health. (2015) 12:9672–82. doi: 10.3390/ijerph120809672
234. Liu Z, Ding X, Wu J, He H, Wu Z, Xie D, et al. Dose-response relationship between higher serum calcium level and higher prevalence of hyperuricemia: a cross-sectional study. Medicine. (2019) 98:e15611. doi: 10.1097/MD.0000000000015611
235. Jiang T, Xie D, Wu J, He H, Wang H, Wang N, et al. Association between serum copper levels and prevalence of hyperuricemia: a cross-sectional study. Sci Rep. (2020) 10:8687. doi: 10.1038/s41598-020-65639-0
236. Wang Y, Yang Z, Wu J, Xie D, Yang T, Li H, et al. Associations of serum iron and ferritin with hyperuricemia and serum uric acid. Clin Rheumatol. (2020) 39:3777–85. doi: 10.1007/s10067-020-05164-7
237. Park J, Kim Y. Associations of blood heavy metals with uric acid in the Korean general population: analysis of data from the 2016-2017 Korean national health and nutrition examination survey. Biol Trace Elem Res. (2021) 199:102–12. doi: 10.1007/s12011-020-02152-5
238. Li CC, Lyu YB, Chen C, Zhang XC, Cai JF, Zhou JH, et al. Association of blood arsenic level with hyperuricemia among elderly aged 65 years and older in 9 longevity areas of China. Zhonghua Yu Fang Yi Xue Za Zhi. (2021) 55:60–5. doi: 10.3760/cma.j.cn112150-20200720-01028
239. Zeng C, Wang YL, Wei J, Yang T, Li H, Xie DX, et al. Association between low serum magnesium concentration and hyperuricemia. Magnes Res. (2015) 28:56–63. doi: 10.1684/mrh.2015.0384
240. Lu X, Shi X, Li Y, Chi H, Liao E, Liu C, et al. A negative association between urinary iodine concentration and the prevalence of hyperuricemia and gout: a cross-sectional and population-based study in Mainland China. Eur J Nutr. (2020) 59:3659–68. doi: 10.1007/s00394-020-02199-z
241. Xie DX, Xiong YL, Zeng C, Wei J, Yang T, Li H, et al. Association between low dietary zinc and hyperuricaemia in middle-aged and older males in China: a cross-sectional study. BMJ Open. (2015) 5:e008637. doi: 10.1136/bmjopen-2015-008637
242. Zhang Y, Liu Y, Qiu H. Association between dietary zinc intake and hyperuricemia among adults in the United States. Nutrients. (2018) 10:568. doi: 10.3390/nu10050568
243. Sun H, Wang N, Chen C, Nie X, Han B, Li Q, et al. Cadmium exposure and its association with serum uric acid and hyperuricemia. Sci Rep. (2017) 7:550. doi: 10.1038/s41598-017-00661-3
244. Jung W, Kim Y, Lihm H, Kang J. Associations between blood lead, cadmium, and mercury levels with hyperuricemia in the Korean general population: a retrospective analysis of population-based nationally representative data. Int J Rheum Dis. (2019) 22:1435–44. doi: 10.1111/1756-185X.13632
245. Zeng A, Li S, Zhou Y, Sun D. Association between low-level blood cadmium exposure and hyperuricemia in the American general population: a cross-sectional study. Biol Trace Elem Res. (2022) 200:560–7. doi: 10.1007/s12011-021-02700-7
246. Kawada T. Blood cadmium and hyperuricemia in adults with special reference to sex difference. Biol Trace Elem Res. (2022) 200:1561. doi: 10.1007/s12011-021-02791-2
247. Wang T, Lv Z, Wen Y, Zou X, Zhou G, Cheng J, et al. Associations of plasma multiple metals with risk of hyperuricemia: a cross-sectional study in a mid-aged and older population of China. Chemosphere. (2022) 287:132305. doi: 10.1016/j.chemosphere.2021.132305
248. Ma Y, Hu Q, Yang D, Zhao Y, Bai J, Mubarik S, et al. Combined exposure to multiple metals on serum uric acid in NHANES under three statistical models. Chemosphere. (2022) 301:134416. doi: 10.1016/j.chemosphere.2022.134416
249. Gu T, Cao G, Luo M, Zhang N, Xue T, Hou R, et al. A systematic review and meta-analysis of the hyperuricemia risk from certain metals. Clin Rheumatol. (2022) 41:3641–60. doi: 10.1007/s10067-022-06362-1
250. Kawai M, de Paula FJ, Rosen CJ. New insights into osteoporosis: the bone-fat connection. J Intern Med. (2012) 272:317–29. doi: 10.1111/j.1365-2796.2012.02564.x
251. Modest JM, Sheth H, Gohh R, Aaron RK. Osteomalacia and renal osteodystrophy. R I Med J. (2022) 105:22–7.
252. Aaseth J, Boivin G, Andersen O. Osteoporosis and trace elements–an overview. J Trace Elem Med Biol. (2012) 26:149–52. doi: 10.1016/j.jtemb.2012.03.017
253. Francis RM, Selby PL. Osteomalacia. Baillieres Clin Endocrinol Metab. (1997) 11:145–63. doi: 10.1016/S0950-351X(97)80569-1
254. Herzberg M, Foldes J, Steinberg R, Menczel J. Zinc excretion in osteoporotic women. J Bone Miner Res. (1990) 5:251–7. doi: 10.1002/jbmr.5650050308
255. Järup L, Alfvén T, Persson B, Toss G, Elinder CG. Cadmium may be a risk factor for osteoporosis. Occup Environ Med. (1998) 55:435–9. doi: 10.1136/oem.55.7.435
256. Gür A, Colpan L, Nas K, Cevik R, Saraç J, Erdogan F, et al. The role of trace minerals in the pathogenesis of postmenopausal osteoporosis and a new effect of calcitonin. J Bone Miner Metab. (2002) 20:39–43. doi: 10.1007/s774-002-8445-y
257. Hyun TH, Barrett-Connor E, Milne DB. Zinc intakes and plasma concentrations in men with osteoporosis: the rancho bernardo study. Am J Clin Nutr. (2004) 80:715–21. doi: 10.1093/ajcn/80.3.715
258. Weinberg ED. Iron loading: a risk factor for osteoporosis. Biometals. (2006) 19:633–5. doi: 10.1007/s10534-006-9000-8
259. Mutlu M, Argun M, Kilic E, Saraymen R, Yazar S. Magnesium, zinc and copper status in osteoporotic, osteopenic and normal post-menopausal women. J Int Med Res. (2007) 35:692–5. doi: 10.1177/147323000703500514
260. Mahdavi-Roshan M, Ebrahimi M, Ebrahimi A. Copper, magnesium, zinc and calcium status in osteopenic and osteoporotic post-menopausal women. Clin Cases Miner Bone Metab. (2015) 12:18–21. doi: 10.11138/ccmbm/2015.12.1.018
261. Scimeca M, Feola M, Romano L, Rao C, Gasbarra E, Bonanno E, et al. Heavy metals accumulation affects bone microarchitecture in osteoporotic patients. Environ Toxicol. (2017) 32:1333–42. doi: 10.1002/tox.22327
262. Arslanca T, Korkmaz V, Arslanca SB, Karadag B, Ergün Y. Body iodine status in women with postmenopausal osteoporosis. Menopause. (2018) 25:320–3. doi: 10.1097/GME.0000000000000987
263. Abdelnour SA, Abd El-Hack ME, Swelum AA, Perillo A, Losacco C. The vital roles of boron in animal health and production: a comprehensive review. J Trace Elem Med Biol. (2018) 50:296–304. doi: 10.1016/j.jtemb.2018.07.018
264. Al EAA, Parsian H, Fathi M, Faghihzadeh S, Hosseini SR, Nooreddini HG, et al. ALOX12 gene polymorphisms and serum selenium status in elderly osteoporotic patients. Adv Clin Exp Med. (2018) 27:1717–22. doi: 10.17219/acem/75689
265. Wang C, Zhu Y, Long H, Ou M, Zhao S. Relationship between blood manganese and bone mineral density and bone mineral content in adults: a population-based cross-sectional study. PLoS ONE. (2022) 17:e0276551. doi: 10.1371/journal.pone.0276551
266. Wei M, Huang Q, Dai Y, Zhou H, Cui Y, Song W, et al. Manganese, iron, copper, and selenium co-exposure and osteoporosis risk in Chinese adults. J Trace Elem Med Biol. (2022) 72:126989. doi: 10.1016/j.jtemb.2022.126989
267. Noor Z, Sumitro SB, Hidayat M, Rahim AH, Sabarudin A, Umemura T. Atomic mineral characteristics of Indonesian osteoporosis by high-resolution inductively coupled plasma mass spectrometry. ScientificWorldJournal. (2012) 2012:372972. doi: 10.1100/2012/372972
268. Okyay E, Ertugrul C, Acar B, Sisman AR, Onvural B, Ozaksoy D. Comparative evaluation of serum levels of main minerals and postmenopausal osteoporosis. Maturitas. (2013) 76:320–5. doi: 10.1016/j.maturitas.2013.07.015
269. Lin S, Yang F, Ling M, Fan Y. Association between bone trace elements and osteoporosis in older adults: a cross-sectional study. Ther Adv Musculoskelet Dis. (2022) 14:1759720X221125984. doi: 10.1177/1759720X221125984
270. Ximenez JPB, Zamarioli A, Kacena MA, Barbosa RM, Barbosa F Jr. Association of urinary and blood concentrations of heavy metals with measures of bone mineral density loss: a data mining approach with the results from the national health and nutrition examination survey. Biol Trace Elem Res. (2021) 199:92–101. doi: 10.1007/s12011-020-02150-7
271. Bingham CT, Fitzpatrick LA. Noninvasive testing in the diagnosis of osteomalacia. Am J Med. (1993) 95:519–23. doi: 10.1016/0002-9343(93)90335-M
272. D'Haese PC, Couttenye MM, Lamberts LV, Elseviers MM, Goodman WG, Schrooten I, et al. Aluminum, iron, lead, cadmium, copper, zinc, chromium, magnesium, strontium, and calcium content in bone of end-stage renal failure patients. Clin Chem. (1999) 45:1548–56. doi: 10.1093/clinchem/45.9.1548
273. D'Haese PC, Schrooten I, Goodman WG, Cabrera WE, Lamberts LV, Elseviers MM, et al. Increased bone strontium levels in hemodialysis patients with osteomalacia. Kidney Int. (2000) 57:1107–14. doi: 10.1046/j.1523-1755.2000.00938.x
274. Järup L, Alfvén T. Low level cadmium exposure, renal and bone effects–the OSCAR study. Biometals. (2004) 17:505–9. doi: 10.1023/B:BIOM.0000045729.68774.a1
275. Zhang YF, Xu JW, Yang Y, Huang X, Yu XQ. The association between body lead levels and childhood rickets: a meta-analysis based on Chinese cohort. Medicine. (2019) 98:e14680. doi: 10.1097/MD.0000000000014680
276. Liu C, Li X, Zhao Z, Chi Y, Cui L, Zhang Q, et al. Iron deficiency plays essential roles in the trigger, treatment, and prognosis of autosomal dominant hypophosphatemic rickets. Osteoporos Int. (2021) 32:737–45. doi: 10.1007/s00198-020-05649-w
277. Honda R, Tsuritani I, Ishizaki M, Yamada Y. Zinc and copper levels in ribs of cadmium-exposed persons with special reference to osteomalacia. Environ Res. (1997) 75:41–8. doi: 10.1006/enrs.1997.3747
278. Miekeley N, de Fortes Carvalho LM, Porto da Silveira CL, Lima MB. Elemental anomalies in hair as indicators of endocrinologic pathologies and deficiencies in calcium and bone metabolism. J Trace Elem Med Biol. (2001) 15:46–55. doi: 10.1016/S0946-672X(01)80026-2
279. Dogan M, Cesur Y, Zehra Dogan S, Kaba S, Bulan K, Cemek M. Oxidant/antioxidant system markers and trace element levels in children with nutritional rickets. J Pediatr Endocrinol Metab. (2012) 25:1129–39. doi: 10.1515/jpem-2012-0153
280. Zhang Y, He J, Jin J, Ren C. Recent advances in the application of metallomics in diagnosis and prognosis of human cancer. Metallomics. (2022) 14:mfac037. doi: 10.1093/mtomcs/mfac037
281. Li M, Chi X, Wang Y, Setrerrahmane S, Xie W, Xu H. Trends in insulin resistance: insights into mechanisms and therapeutic strategy. Signal Transduct Target Ther. (2022) 7:216. doi: 10.1038/s41392-022-01073-0
282. Waseem A, Arshad J. A review of human biomonitoring studies of trace elements in Pakistan. Chemosphere. (2016) 163:153–76. doi: 10.1016/j.chemosphere.2016.08.011
283. Pagano G, Thomas PJ, Di Nunzio A, Trifuoggi M. Human exposures to rare earth elements: present knowledge and research prospects. Environ Res. (2019) 171:493–500. doi: 10.1016/j.envres.2019.02.004
Keywords: trace element, ionome, ionomics, metabolic disease, bioinformatics
Citation: Zhang Y, Huang B, Jin J, Xiao Y and Ying H (2023) Recent advances in the application of ionomics in metabolic diseases. Front. Nutr. 9:1111933. doi: 10.3389/fnut.2022.1111933
Received: 30 November 2022; Accepted: 30 December 2022;
Published: 16 January 2023.
Edited by:
Peng An, China Agricultural University, ChinaReviewed by:
Phitsanu Tulayakul, Kasetsart University, ThailandBernhard Michalke, Research Unit Analytical BioGeoChemistry, Helmholtz Center München (HZ), Germany
Copyright © 2023 Zhang, Huang, Jin, Xiao and Ying. This is an open-access article distributed under the terms of the Creative Commons Attribution License (CC BY). The use, distribution or reproduction in other forums is permitted, provided the original author(s) and the copyright owner(s) are credited and that the original publication in this journal is cited, in accordance with accepted academic practice. No use, distribution or reproduction is permitted which does not comply with these terms.
*Correspondence: Yan Zhang, zhangyan@szu.edu.cn; Huimin Ying,
yinghuimin@139.com