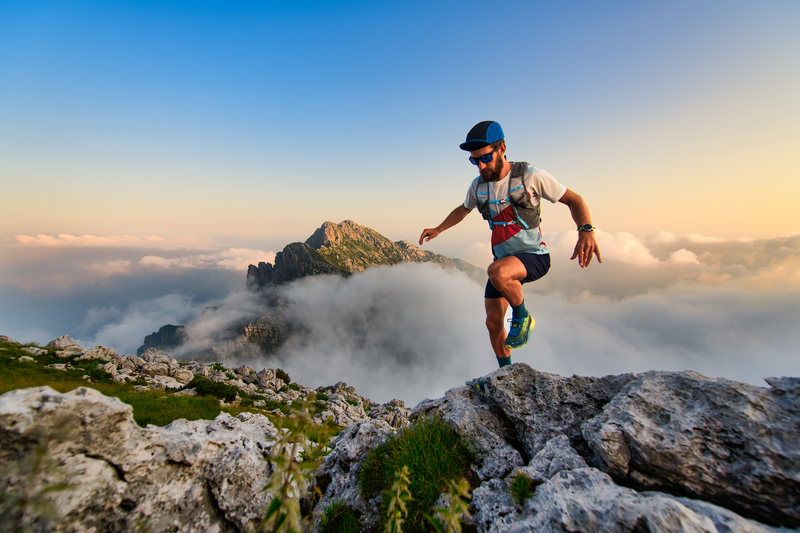
95% of researchers rate our articles as excellent or good
Learn more about the work of our research integrity team to safeguard the quality of each article we publish.
Find out more
ORIGINAL RESEARCH article
Front. Nutr. , 06 December 2022
Sec. Clinical Nutrition
Volume 9 - 2022 | https://doi.org/10.3389/fnut.2022.1048206
This article is part of the Research Topic Biomarkers: Precision Nutrition in Chronic Diseases View all 26 articles
Objective: We aimed to investigate the association between the GNRI and the risk of stroke in elderly patients with hypertension.
Methods: A total of 5312 elderly hypertensive patients free of history of stroke were included. Multivariate Cox models were used to calculate hazard ratios (HRs) and their 95% confidence intervals (CIs) for stroke and its subtypes.
Results: The average time of follow-up was 3.8 years, and the median time was 3.2 years. We identified 640 individuals with stroke, of whom 526 had an ischemic stroke (IS) and 114 had a hemorrhagic stroke (HS). After adjusting for confounding variables, compared with participants in the lowest quartile of the GNRI, those in the third and fourth quartiles were associated with a decreased risk of stroke (adjusted HR 0.72, 95% CI 0.58–0.90, and adjusted HR 0.58, 95% CI 0.46–0.74, respectively, P for trend < 0.001). Similar results were found for IS and HS. Moreover, there were L-shaped associations of GNRI with new-onset HS (P for non-linearity = 0.034). Multiple sensitivity analyses and stratified analyses did not materially change the results.
Conclusions: In summary, we found that a lower GNRI was associated with a higher risk of incident stroke in elderly hypertensive patients. Additional prospective data collection is required to confirm our findings.
Stroke, including ischemic stroke (IS) and hemorrhagic stroke (HS), is the leading cause of the global burden of disease (1). China has the highest burden of strokes in the world (2). There are still 250 million new instances of stroke each year in China, and that number is rising, even though the incidence and frequency of stroke have decreased globally (3). Hypertension has now been identified as the primary variable risk factor for stroke (4). Several large epidemiological surveys in China have shown that more than 50% of people over 60 years of age have hypertension (5). Therefore, identifying the residual risk of stroke and early risk stratification in elderly patients with hypertension is essential to more effectively tailoring risk reduction strategies.
Malnutrition is associated with a poor clinical prognosis in patients with various diseases (6). According to studies, malnutrition is significantly linked to increased levels of inflammatory response, arterial calcification, and atherosclerosis progression, which raises the possibility that it plays a key role in the emergence of cardiovascular disease (7, 8). The geriatric nutritional risk index (GNRI) is a simple, well-established nutrition assessment tool that uses serum albumin and body mass index (BMI) (9). Recent studies have shown that GNRI is associated with the development of atherosclerosis and an increased risk of cardiovascular mortality in older patients (10, 11). However, studies on GNRI as a predictor of new-onset stroke are still limited. Until now, only one cohort study has reported lower GNRI in hemodialysis patients as an independent risk factor for cerebral infarction and hemorrhage, and it is unclear whether this effect can be extended to older patients with hypertension (10). Therefore, GNRI may have important clinical implications for stroke risk stratification in hypertensive patients. In addition, the status of the dose-response relationship between GNRI and the risk of stroke and its subtypes in elderly hypertensive patients is uncertain.
Therefore, the present study is based on a cohort study aiming to investigate the association between GNRI and the risk of stroke and its subtypes in elderly hypertensive patients and to characterize the nature of the dose-response relationship.
We conducted a cohort study at the People's Hospital of Xinjiang Uygur Autonomous Region, Xinjiang, China. All patients were either older than 60 years of age and were recruited between January 1, 2010, and December 31, 2021. First, we excluded patients who had < 6 months of follow-up or had the outcome at baseline. Second, we further excluded individuals with missing data on body height, body weight, or serum albumin level. Third, we excluded participants with severe wasting diseases (e.g., malignancy, autoimmune diseases, severe hepatic disease, and severe renal insufficiency). Finally, this left a final study population of 5,312 patients. Participant flow is shown in Figure 1. A comparison of baseline characteristics for in- and excluded participants are presented in Supplementary Table S1. This study was approved by the ethics committee of the People's Hospital of Xinjiang Uygur Autonomous Region (No. KY2021031901). A waiver of informed consent was granted due to the retrospective data collection. The study was reported as per the STROBE statement for observational cohort studies (12).
Data were abstracted electronically from the patient's medical records, including demographic characteristics, diagnoses according to the International Classification of Diseases 10th Revision (ICD-10), prescribed medications, and laboratory reports. Weight, height, heart rate, and blood pressure (BP) were measured using standard protocols. The BMI (kg/m2) was computed from the measured weight and height. Smoking status included categories of current smokers and non-smokers. Participants are classified as current drinkers and non-smokers. Blood samples were drawn after an overnight fast. The participants' prior medical histories were evaluated using ICD-10 codes. To ensure the accuracy of diagnoses, coronary heart disease (CHD) (I24 and I25), diabetes (E10-E14), atrial fibrillation (I48), and dyslipidemia (E78) were regarded as present if a participant was treated ≥ 2 times. To quantify the burden of comorbidities, the Charlson Comorbidity Index (CCI) was calculated as described previously (13). Prescription claims within the last year before the baseline defined concomitant medications. The list of concomitant medications included in the study is shown in Supplementary Table S2. The GNRI formula used was as follows: GNRI = (1.489 × albumin, g/l) + (41.7 × present/ideal body weight). Ideal weight was calculated using the Lorenz formulas: For males: height - 100 - [(height - 150)/4]. For females: height - 100 - [(height - 150)/2.5] (14).
The primary outcome was the first occurrence of stroke (ischemic or hemorrhagic), either nonfatal or fatal. Secondary outcomes included the first ischemic stroke and the first hemorrhagic stroke. Methods of determination of incident stroke are described in the Supplementary material. Outcomes of events since participants enrolled in the study were determined through medical records, patient and family interview, contact with local disease and death registries, or access to the database of basic medical insurance. These data sources are linked using an individual national identification number assigned to each Chinese person for life. This number is replaced by a series number when provided for personal data analysis to anonymize the individual participant's data. Patients were followed from the date of enrollment to the end of the observation period, defined as the date of the last follow-up visit, the date of the first appearance of any study outcome, the date of death, or the end of the study period (December 31, 2021).
Details of the missing covariates are shown in Supplementary Table S3. Missing values of covariates (all covariates were missing in < 6%) were imputed using multiple imputations by chained equations. Characteristics of study participants were expressed by GNRI quartiles. For differences in cumulative incidence between groups, we used Kaplan-Meier curves and the log-rank test. The multicollinearity test suggested that the variance inflation factors of all variables were less than five, confirming that the regression model was not affected by multicollinearity. The hazard ratio (HR) estimates and 95% confidence intervals (CI) were determined by the Cox regression models. Tests for non-linear associations were performed using restricted cubic spline regressions. We also performed subgroup analyses stratified by potential confounders. Sensitivity analyses were undertaken to evaluate the robustness of the results. First, to minimize the chance of reverse causation, we excluded events that occurred within 1 or 3 years after the baseline visit. Second, sensitivity analysis determined whether event risks remained stable after accounting for competing risks. Third, participants with CCI ≥2 were excluded to reduce confounding factors caused by associated comorbidity. Fourth, participants with atrial fibrillation were excluded. Lastly, to evaluate potential unmeasured confounding, we calculated E-values. Further analysis details are provided in the Supplementary material. Statistical analyses were performed using R software, version 4.1.1. Two-sided P-values < 0.05 were considered statistically significant.
As illustrated in the flow chart (Figure 1), a total of 5312 participants were included in the current study. The average age of the study population was 66.5 years (SD 4.8). The GNRI was approximately normally distributed (Figure 2). Baseline characteristics of the study participants by GNRI quartiles are shown in Table 1. Participants with a lower GNRI tended to be women, have a higher duration of hypertension, a higher prevalence of dyslipidemia, take more statins and aspirin, have higher HbA1c, TC, TG, and LDL-C levels, and have lower HDL-C compared with participants in the quartile 4 group.
The average time of follow-up was 3.8 years, and the median time was 3.2 years. We identified 640 individuals with stroke, of which 526 had IS and 114 had HS. The Kaplan-Meier curve showed that participants in the quartile 1 group had a higher risk of total stroke, IS instead of HS than those in other groups (log-rank test, P < 0.001, Figure 3A; P = 0.001, Figure 3B; P = 0.001, Figure 3C). Overall, there was a significant inverse association of GNRI with the risk of first total stroke (Figure 4A) (per SD increment; full adjusted HR: 0.80; 95% CI: 0.73, 0.87). Consistently, when GNRI was assessed as quartiles, the full adjusted HRs of first stroke for participants in quartile 2, quartile 3, and quartile 4 were 0.94 (95% CI: 0.77, 1.15), 0.72 (95% CI: 0.58, 0.90), and 0.58 (95% CI: 0.46, 0.74) respectively, compared with those in quartile 1 (P for trend < 0.001) (Table 2). Similarly, a significant inverse association between GNRI and both IS and HS (Figure 4). Moreover, there were L-shaped associations of GNRI with new-onset HS (P for non-linearity = 0.034). Sensitivity analyses were conducted to verify the robustness of the reported findings. The results of sensitivity analyses were similar to those observed in the main analysis (Supplementary Tables S4–S9 and Supplementary Figure S1 in the Supplement).
Figure 3. Kaplan-Meier survival curves for total stroke and individual outcomes based on GNRI quartiles. (A) Total stroke, (B) ischemic stroke, and (C) hemorrhagic stroke.
Figure 4. Dose-response association between GNRI and risk of stroke events. (A) Total stroke, (B) ischemic stroke, and (C) hemorrhagic stroke.
Stratified analyses were performed to assess the association of GNRI (per SD increment) with total stroke and its subtypes, as provided in Figure 5. No interaction was found between subgroup variables and the association of GNRI with the risk of total stroke. Similar results were found for IS and HS.
Figure 5. Subgroup analyses of the relationship between GNRI and risk of stroke events. (A) Total stroke, (B) ischemic stroke, and (C) hemorrhagic stroke.
As illustrated in Table 3, according to C-statistic, risk prediction was improved by adding the GNRI to established risk factors (C-statistic increased from 0.613 to 0.648, P < 0.001). Moreover, according to continuous NRI and IDI, the GNRI significantly improved risk discrimination for total stroke [continuous NRI (95% CI): 0.118 (0.066–0.172), P < 0.001; IDI (95% CI): 0.017 (0.009–0.029), P < 0.001]. Furthermore, the DCA for the different models is shown in Supplementary Figure S2. The decision curves show that using a combination of GNRI features to predict total stroke increases the net benefit more than using established risk factors alone. Similar results were observed in IS and HS.
This study investigated the relationship between GNRI and incident stroke in elderly hypertensive patients. The findings revealed that the risk of stroke was significantly associated with baseline GNRI after adjusting for multiple confounders. In addition, a significant L-shaped dose-response relationship between GNRI and the risk of incident HS was observed, indicating a rapid increase in the risk of HS when GNRI was below 103. These findings were reliable in subgroup and multiple sensitivity analyses. Overall, the present study revealed that low CNRI was associated with a higher risk of incident stroke. To our knowledge, this is the first study to show an association between GNRI and the risk of incident stroke in a large retrospective cohort.
Aging is a condition that affects all people. One of the most susceptible demographics and one that is more likely to experience nutritional issues is the elderly (15). Similarly, malnutrition is an important independent risk factor for stroke, as is hypertension (16–19). In contrast to other clinical variables, nutritional status is a modifiable risk factor that physicians can act on. Therefore, appropriate tools are needed to assess the nutritional status of elderly patients with hypertension and to identify patients at risk to reduce their risk of stroke. Among multiple proxies of nutritional status, serum albumin levels and BMI are often used to evaluate nutritional status (20, 21). Hypoalbuminemia has been linked to acute and chronic inflammation, low BMI may be a sign of malnutrition, and both conditions may be a result of the loss of muscle and adipose tissue (22). In the general population, hypoalbuminemia and higher BMI have been reported as independent risk factors for stroke (23–26). Systemic edema, hepatic failure, and inflammation all have a negative influence on serum albumin levels. The status of body fluids also impacts body weight (27, 28). Consequently, assessing nutritional risk and prognosis solely based on albumin or BMI may not be sufficient. Simple hematological data (serum albumin) and anthropometric data can be used to compute the GNRI, a simplified form of the nutritional risk index (including height and weight) (14, 15). These indicators are readily available and can reduce information bias. Because of its objective nature, GNRI overcomes the problems of traditional nutrition indicators, including subjective issues such as mini-nutritional assessments (29). And GNRI correlates well with malnutrition-inflammation scores and has been regarded as one of the gold standards for nutritional assessment of elderly patients with chronic diseases (10, 30). There is evidence that patients with chronic illnesses, including chronic hemodialysis and peripheral vascular disease, have a lower GNRI (31–35). The results of Xiong et al. demonstrated that low GNRI levels were a strong predictor of cardiovascular and cerebrovascular events in patients with CKD (36). GNRI has also been reported to predict cardiovascular events, including cardiovascular disease mortality, in patients with heart failure (37). Furthermore, a study by Anzaki et al. (38) showed that low GNRI levels were associated with all-cause mortality and major adverse cardiovascular events after elective percutaneous coronary intervention (PCI). Cheng et al. (11) shown that in patients with chronic coronary artery occlusion (CTO) following PCI, the GNRI score at admission was a reliable predictor of adverse cardiovascular events. The prediction of cardiovascular events following PCI in patients with CTO was greatly enhanced by including the GNRI score into existing risk prediction algorithms (11). Elderly patients with hypertension are prone to multiple chronic diseases and may focus more on the primary disease, but nutritional support is mostly neglected. Our findings suggest that physicians may incorporate the identification of nutritional status into their daily practice. From prior research and the findings of the current study, we suggest that modestly increasing calorie and protein intake in malnourished elderly patients with hypertension may reduce the risk of stroke (39, 40). Further prospective intervention trials are needed to establish causality.
Although the underlying mechanisms remain unclear, there are some possible explanations. Oxidative stress and inflammation play key roles in the pathogenesis of stroke (41). First, serum albumin is a multifunctional protein that exerts neuroprotective effects in ischemic strokes, such as resisting antioxidants and reducing erythrocyte pressure levels (42, 43). According to Dziedzic et al. (43) stroke patients with decreased serum albumin levels had worse prognoses. Low albumin levels significantly enhanced the probability of recurrence in stroke patients and were related with poor outcome in all stroke subtypes (43, 44). Second, another potential mechanism may be attributed to the inflammatory response. According to a number of studies, inflammation frequently fosters a catabolic state that increases protein breakdown and slows protein synthesis, resulting in malnutrition and a decrease in GNRI (45). Additionally, it has been proven in the past that malnutrition is associated with higher than normal levels of inflammatory markers (46). Lower albumin levels are a result of the catabolic cytokines, muscle catabolism, and hunger suppression that are linked to chronic inflammatory disorders (47, 48). As a result, inflammation may be a key relationship between dietary status and the risk of cardiovascular disease (49). Severe malnutrition is closely associated with high levels of inflammation, and inflammation can increase the burden of atherosclerosis (50). At the same time, the inflammatory response reduces albumin synthesis, further inducing malnutrition (51). There may be a positive feedback loop between inflammation, malnutrition, immune defense, and adverse events, resulting in a vicious cycle. Therefore, the link between these three entities is also described as the malnutrition-inflammation-atherosclerosis syndrome (52, 53).
The strengths of this study lie in the novelty, the long observational period, and the well-characterized participants. Despite the aforementioned merits, several limitations of the present study merit discussion. First, it was observational and cannot establish causation. Second, the time-dependent changes of GNRI during the follow-up period were not assessed. Third, we didn't examine the predictive value of GNRI against more thorough nutrition evaluations. Despite adjustment for major confounding factors, the risk of residual unmeasured confounding remains possible. Finally, this study is limited to China and needs to be replicated in other different populations. Given the limitations inherent in this study, these results should be interpreted with caution but warrant further investigation in subsequent studies.
In summary, we demonstrated that a lower GNRI was associated with a higher risk of incident stroke in elderly hypertensive patients. In addition, a significant L-shaped dose-response relationship between GNRI and the risk of incident HS was observed. Additional prospective data collection is required to confirm our findings.
The original contributions presented in the study are included in the article/Supplementary material, further inquiries can be directed to the corresponding author/s.
The studies involving human participants were reviewed and approved by People's Hospital of Xinjiang Uygur Autonomous Region. Written informed consent for participation was not required for this study in accordance with the national legislation and the institutional requirements.
XC and JHu analyzed the data and wrote the manuscript. JHo, QZ, MW, YD, and WY helped with copyediting. XC and NL audited the data. SL, JHu, and XC conducted research. NL had primary responsibility for the final content of the manuscript. All authors read and approved the final manuscript.
This research was supported by the Chinese Academy of Medical Sciences (2020-RW330-002).
We are grateful to all the participants.
The authors declare that the research was conducted in the absence of any commercial or financial relationships that could be construed as a potential conflict of interest.
All claims expressed in this article are solely those of the authors and do not necessarily represent those of their affiliated organizations, or those of the publisher, the editors and the reviewers. Any product that may be evaluated in this article, or claim that may be made by its manufacturer, is not guaranteed or endorsed by the publisher.
The Supplementary Material for this article can be found online at: https://www.frontiersin.org/articles/10.3389/fnut.2022.1048206/full#supplementary-material
1. Global Global burden of 369 diseases and injuries in 204 countries and territories 1990–2019: 1990–2019: a systematic analysis for the global burden of disease study 2019. Lancet. (2020) 396:1204-22. doi: 10.1016/S0140-6736(20)30925-9
2. Feigin VL, Nguyen G, Cercy K, Johnson CO, Alam T, Parmar PG, et al. Global, regional, and country-specific lifetime risks of stroke, 1990 and 2016. N Engl J Med. (2018) 379:2429–37. doi: 10.1056/NEJMoa1804492
3. Wang W, Jiang B, Sun H, Ru X, Sun D, Wang L, et al. Prevalence, incidence, and mortality of stroke in China: results from a nationwide population-based survey of 480, 687 adults. Circulation. (2017) 135:759–71. doi: 10.1161/CIRCULATIONAHA.116.025250
4. Xu J, Jiang F, Wang A, Zhi H, Gao Y, Tian J, et al. Ambulatory blood pressure profile and stroke recurrence. Stroke Vasc Neurol. (2021) 6:352–8. doi: 10.1136/svn-2020-000526
5. Zhang WL, Cai J, STEP. to blood pressure management of elderly hypertension: evidence from Asia. Hypertens Res. (2022) 45:576–82. doi: 10.1038/s41440-022-00875-7
6. Norman K, Pichard C, Lochs H, Pirlich M. Prognostic impact of disease-related malnutrition. Clin Nutr. (2008) 27:5–15. doi: 10.1016/j.clnu.2007.10.007
7. Raposeiras Roubín S, Abu Assi E, Cespón Fernandez M, Barreiro Pardal C, Lizancos Castro A, Parada JA, et al. Prevalence and prognostic significance of malnutrition in patients with acute coronary syndrome. J Am Coll Cardiol. (2020) 76:828–40. doi: 10.1016/j.jacc.2020.06.058
8. Zhang G, Pan Y, Zhang R, Wang M, Meng X, Li Z, et al. Prevalence and prognostic significance of malnutrition risk in patients with acute ischemic stroke: results from the third china national stroke registry. Stroke. (2022) 53:111–9. doi: 10.1161/STROKEAHA.121.034366
9. Seoudy H, Al-Kassou B, Shamekhi J, Sugiura A, Frank J, Saad M, et al. Frailty in patients undergoing transcatheter aortic valve replacement: prognostic value of the geriatric nutritional risk index. J Cachexia Sarcopenia Muscle. (2021) 12:577–85. doi: 10.1002/jcsm.12689
10. Tsuneyoshi S, Matsukuma Y, Kawai Y, Hiyamuta H, Yamada S, Kitamura H, et al. Association between geriatric nutritional risk index and stroke risk in hemodialysis patients: 10-Years outcome of the Q-Cohort study. Atherosclerosis. (2021) 323:30–6. doi: 10.1016/j.atherosclerosis.2021.03.006
11. Cheng L, Rong J, Zhuo X, Gao K, Meng Z, Wen X, et al. Prognostic value of malnutrition using geriatric nutritional risk index in patients with coronary chronic total occlusion after percutaneous coronary intervention. Clin Nutr. (2021) 40:4171–9. doi: 10.1016/j.clnu.2021.01.042
12. von Elm E, Altman DG, Egger M, Pocock SJ, Gøtzsche PC, Vandenbroucke JP. The strengthening the reporting of observational studies in epidemiology (STROBE) statement: guidelines for reporting observational studies. Lancet. (2007) 370:1453–7. doi: 10.1016/S0140-6736(07)61602-X
13. Sundararajan V, Henderson T, Perry C, Muggivan A, Quan H, Ghali WA. New ICD-10 version of the Charlson comorbidity index predicted in-hospital mortality. J Clin Epidemiol. (2004) 57:1288–94. doi: 10.1016/j.jclinepi.2004.03.012
14. Bouillanne O, Morineau G, Dupont C, Coulombel I, Vincent JP, Nicolis I, et al. Geriatric Nutritional Risk Index: a new index for evaluating at-risk elderly medical patients. Am J Clin Nutr. (2005) 82:777–83. doi: 10.1093/ajcn/82.4.777
15. Ruan GT, Zhang Q, Zhang X, Tang M, Song MM, Zhang XW, et al. Geriatric nutrition risk index: prognostic factor related to inflammation in elderly patients with cancer cachexia. J Cachexia Sarcopenia Muscle. (2021) 12:1969–82. doi: 10.1002/jcsm.12800
16. Chen N, Li Y, Fang J, Lu Q, He L. Risk factors for malnutrition in stroke patients: a meta-analysis. Clin Nutr. (2019) 38:127–35. doi: 10.1016/j.clnu.2017.12.014
17. Mosselman MJ, Kruitwagen CL, Schuurmans MJ, Hafsteinsdóttir TB. Malnutrition and risk of malnutrition in patients with stroke: prevalence during hospital stay. J Neurosci Nurs. (2013) 45:194–204. doi: 10.1097/JNN.0b013e31829863cb
18. Hankey GJ. Nutrition and the risk of stroke. Lancet Neurol. (2012) 11:66–81. doi: 10.1016/S1474-4422(11)70265-4
19. Howard G, Banach M, Cushman M, Goff DC, Howard VJ, Lackland DT, et al. Is blood pressure control for stroke prevention the correct goal? The lost opportunity of preventing hypertension. Stroke. (2015) 46:1595–600. doi: 10.1161/STROKEAHA.115.009128
20. Zhang Z, Pereira SL, Luo M, Matheson EM. Evaluation of blood biomarkers associated with risk of malnutrition in older adults: a systematic review and meta-analysis. Nutrients. (2017) 9:829. doi: 10.3390/nu9080829
21. Cederholm T, Bosaeus I, Barazzoni R, Bauer J, Van Gossum A, Klek S, et al. Diagnostic criteria for malnutrition - An ESPEN Consensus Statement. Clin Nutr. (2015) 34:335–40. doi: 10.1016/j.clnu.2015.03.001
22. Kovesdy CP, Kalantar-Zadeh K. Why is protein-energy wasting associated with mortality in chronic kidney disease. Semin Nephrol. (2009) 29:3–14. doi: 10.1016/j.semnephrol.2008.10.002
23. Morotti A, Marini S, Lena UK, Crawford K, Schwab K, Kourkoulis C, et al. Significance of admission hypoalbuminemia in acute intracerebral hemorrhage. J Neurol. (2017) 264:905–11. doi: 10.1007/s00415-017-8451-x
24. Zhang Q, Lei YX, Wang Q, Jin YP, Fu RL, Geng HH, et al. Serum albumin level is associated with the recurrence of acute ischemic stroke. Am J Emerg Med. (2016) 34:1812–6. doi: 10.1016/j.ajem.2016.06.049
25. Park JW, Lee SY, Kim SY, Choe H, Jee SH, BMI. and stroke risk in Korean women. Obesity. (2008) 16:396–401. doi: 10.1038/oby.2007.67
26. Huang K, Liu F, Han X, Huang C, Huang J, Gu D, et al. Association of BMI with total mortality and recurrent stroke among stroke patients: a meta-analysis of cohort studies. Atherosclerosis. (2016) 253:94–101. doi: 10.1016/j.atherosclerosis.2016.08.042
27. Franch-Arcas G. The meaning of hypoalbuminaemia in clinical practice. Clin Nutr. (2001) 20:265–9. doi: 10.1054/clnu.2001.0438
28. Honda Y, Nagai T, Iwakami N, Sugano Y, Honda S, Okada A, et al. Usefulness of geriatric nutritional risk index for assessing nutritional status and its prognostic impact in patients aged ≥65 years with acute heart failure. Am J Cardiol. (2016) 118:550–5. doi: 10.1016/j.amjcard.2016.05.045
29. Lee S, Fujita K, Morishita T, Negoro E, Oiwa K, Tsukasaki H, et al. Prognostic utility of a geriatric nutritional risk index in combination with a comorbidity index in elderly patients with diffuse large B cell lymphoma. Br J Haematol. (2021) 192:100–9. doi: 10.1111/bjh.16743
30. Kalantar-Zadeh K, Kopple JD, Block G, Humphreys MH, A. malnutrition-inflammation score is correlated with morbidity and mortality in maintenance hemodialysis patients. Am J Kidney Dis. (2001) 38:1251–63. doi: 10.1053/ajkd.2001.29222
31. Wada H, Dohi T, Miyauchi K, Doi S, Naito R, Konishi H, et al. Prognostic impact of the geriatric nutritional risk index on long-term outcomes in patients who underwent percutaneous coronary intervention. Am J Cardiol. (2017) 119:1740–5. doi: 10.1016/j.amjcard.2017.02.051
32. Minamisawa M, Seidelmann SB, Claggett B, Hegde SM, Shah AM, Desai AS, et al. Impact of malnutrition using geriatric nutritional risk index in heart failure with preserved ejection fraction. JACC Heart Fail. (2019) 7:664–75. doi: 10.1016/j.jchf.2019.04.020
33. Sze S, Pellicori P, Kazmi S, Rigby A, Cleland J, Wong K, et al. Prevalence and prognostic significance of malnutrition using 3 scoring systems among outpatients with heart failure: a comparison with body mass index. JACC Heart Fail. (2018) 6:476–86. doi: 10.1016/j.jchf.2018.02.018
34. Matsuo Y, Kumakura H, Kanai H, Iwasaki T, Ichikawa S. The geriatric nutritional risk index predicts long-term survival and cardiovascular or limb events in peripheral arterial disease. J Atheroscler Thromb. (2020) 27:134–43. doi: 10.5551/jat.49767
35. Matsukuma Y, Tanaka S, Taniguchi M, Nakano T, Masutani K, Hirakata H, et al. Association of geriatric nutritional risk index with infection-related mortality in patients undergoing hemodialysis: the Q-Cohort Study. Clin Nutr. (2019) 38:279–87. doi: 10.1016/j.clnu.2018.01.019
36. Xiong J, Wang M, Wang J, Yang K, Shi Y, Zhang J, et al. Geriatric nutrition risk index is associated with renal progression, cardiovascular events and all-cause mortality in chronic kidney disease. J Nephrol. (2020) 33:783–93. doi: 10.1007/s40620-019-00676-1
37. Takahashi H, Ito Y, Ishii H, Aoyama T, Kamoi D, Kasuga H, et al. Geriatric nutritional risk index accurately predicts cardiovascular mortality in incident hemodialysis patients. J Cardiol. (2014) 64:32–6. doi: 10.1016/j.jjcc.2013.10.018
38. Anzaki K, Kanda D, Ikeda Y, Takumi T, Tokushige A, Ohmure K, et al. Impact of malnutrition on prognosis and coronary artery calcification in patients with stable coronary artery disease. Curr Probl Cardiol. (2022) 3:101185. doi: 10.1016/j.cpcardiol.2022.101185
39. Schuetz P, Fehr R, Baechli V, Geiser M, Deiss M, Gomes F, et al. Individualized nutritional support in medical inpatients at nutritional risk: a randomized clinical trial. Lancet. (2019) 393:2312–21. doi: 10.1016/S0140-6736(18)32776-4
40. Rozentryt P, von Haehling S, Lainscak M, Nowak JU, Kalantar-Zadeh K, Polonski L, et al. The effects of a high-caloric protein-rich oral nutritional supplement in patients with chronic heart failure and cachexia on quality of life, body composition, and inflammation markers: a randomized, double-blind pilot study. J Cachexia Sarcopenia Muscle. (2010) 1:35–42. doi: 10.1007/s13539-010-0008-0
41. Chamorro Á, Dirnagl U, Urra X, Planas AM. Neuroprotection in acute stroke: targeting excitotoxicity, oxidative and nitrosative stress, and inflammation. Lancet Neurol. (2016) 15:869–81. doi: 10.1016/S1474-4422(16)00114-9
42. Zhou H, Wang A, Meng X, Lin J, Jiang Y, Jing J, et al. Low serum albumin levels predict poor outcome in patients with acute ischaemic stroke or transient ischaemic attack. Stroke Vasc Neurol. (2021) 6:458–66. doi: 10.1136/svn-2020-000676
43. Dziedzic T, Slowik A, Szczudlik A. Serum albumin level as a predictor of ischemic stroke outcome. Stroke. (2004) 35:e156–8. doi: 10.1161/01.STR.0000126609.18735.be
44. Babu MS, Kaul S, Dadheech S, Rajeshwar K, Jyothy A, Munshi A. Serum albumin levels in ischemic stroke and its subtypes: correlation with clinical outcome. Nutrition. (2013) 29:872–5. doi: 10.1016/j.nut.2012.12.015
45. Merker M, Felder M, Gueissaz L, Bolliger R, Tribolet P, Kägi-Braun N, et al. Association of baseline inflammation with effectiveness of nutritional support among patients with disease-related malnutrition: a secondary analysis of a randomized clinical trial. JAMA Netw Open. (2020) 3:e200663. doi: 10.1001/jamanetworkopen.2020.0663
46. Fatyga P, Pac A, Fedyk-Łukasik M, Grodzicki T, Skalska A. The relationship between malnutrition risk and inflammatory biomarkers in outpatient geriatric population. Eur Geriatr Med. (2020) 11:383–91. doi: 10.1007/s41999-020-00303-4
47. Ertem AG, Açar B, Ünal S, Yayla Ç. Albumin is an acute or chronic inflammatory marker for in-stent restenosis in patients with coronary artery disease. Angiology. (2017) 68:176. doi: 10.1177/0003319716654079
48. Artigas A, Wernerman J, Arroyo V, Vincent JL, Levy M. Role of albumin in diseases associated with severe systemic inflammation: pathophysiologic and clinical evidence in sepsis and in decompensated cirrhosis. J Crit Care. (2016) 33:62–70. doi: 10.1016/j.jcrc.2015.12.019
49. Arques S. Human serum albumin in cardiovascular diseases. Eur J Intern Med. (2018) 52:8–12. doi: 10.1016/j.ejim.2018.04.014
50. Maraj M, Kuśnierz-Cabala B, Dumnicka P, Gala-Bładzińska A, Gawlik K, Pawlica-Gosiewska D, et al. Malnutrition, inflammation, atherosclerosis syndrome (MIA) and diet recommendations among end-stage renal disease patients treated with maintenance hemodialysis. Nutrients. (2018) 10:69. doi: 10.3390/nu10010069
51. Corti MC, Guralnik JM, Salive ME, Sorkin JD. Serum albumin level and physical disability as predictors of mortality in older persons. JAMA. (1994) 272:1036–42.
52. Sueta D, Hokimoto S, Sakamoto K, Akasaka T, Tabata N, Kaikita K, et al. Validation of the high mortality rate of malnutrition-inflammation-atherosclerosis syndrome: -community-based observational study. Int J Cardiol. (2017) 230:97–102. doi: 10.1016/j.ijcard.2016.12.072
53. Akdag I, Yilmaz Y, Kahvecioglu S, Bolca N, Ercan I, Ersoy A, et al. Clinical value of the malnutrition-inflammation-atherosclerosis syndrome for long-term prediction of cardiovascular mortality in patients with end-stage renal disease: a 5-year prospective study. Nephron Clin Pract. (2008) 108:c99–105. doi: 10.1159/000113526
Keywords: geriatric nutritional risk index, stroke, elderly, hypertension, longitudinal cohort
Citation: Cai X, Hu J, Wen W, Wang M, Zhu Q, Liu S, Yang W, Dang Y, Hong J and Li N (2022) Association between the geriatric nutritional risk index and the risk of stroke in elderly patients with hypertension: A longitudinal and cohort study. Front. Nutr. 9:1048206. doi: 10.3389/fnut.2022.1048206
Received: 19 September 2022; Accepted: 21 November 2022;
Published: 06 December 2022.
Edited by:
Zhenjun Zhu, Jinan University, ChinaReviewed by:
Peicong Ge, Beijing Tiantan Hospital, Capital Medical University, ChinaCopyright © 2022 Cai, Hu, Wen, Wang, Zhu, Liu, Yang, Dang, Hong and Li. This is an open-access article distributed under the terms of the Creative Commons Attribution License (CC BY). The use, distribution or reproduction in other forums is permitted, provided the original author(s) and the copyright owner(s) are credited and that the original publication in this journal is cited, in accordance with accepted academic practice. No use, distribution or reproduction is permitted which does not comply with these terms.
*Correspondence: Nanfang Li, bG5hbmZhbmcyMDE2QHNpbmEuY29t
Disclaimer: All claims expressed in this article are solely those of the authors and do not necessarily represent those of their affiliated organizations, or those of the publisher, the editors and the reviewers. Any product that may be evaluated in this article or claim that may be made by its manufacturer is not guaranteed or endorsed by the publisher.
Research integrity at Frontiers
Learn more about the work of our research integrity team to safeguard the quality of each article we publish.