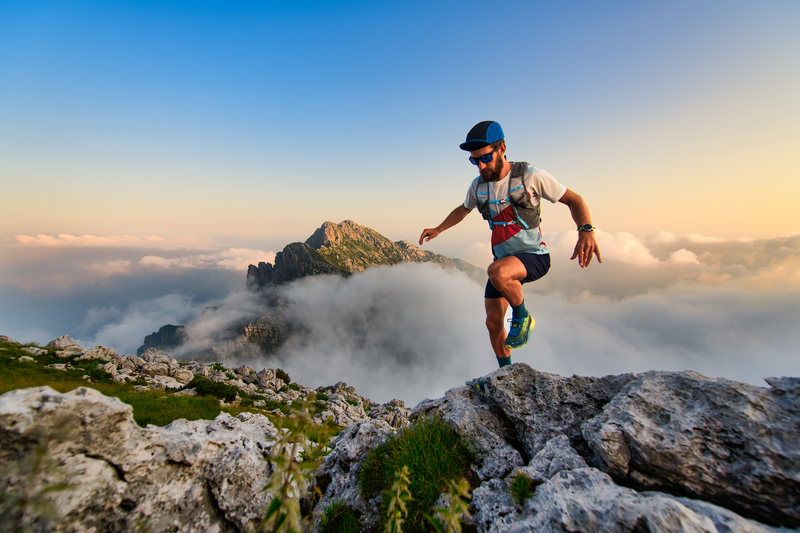
94% of researchers rate our articles as excellent or good
Learn more about the work of our research integrity team to safeguard the quality of each article we publish.
Find out more
MINI REVIEW article
Front. Nutr. , 23 September 2022
Sec. Nutrition and Microbes
Volume 9 - 2022 | https://doi.org/10.3389/fnut.2022.1006747
The investigation of the human gut microbiome during recent years has permitted us to understand its relevance for human health at a systemic level, making it possible to establish different functional axes (e.g., the gut-brain, gut-liver, and gut-lung axes), which support the organ-like status conferred to this microecological component of our body. The human gut microbiota is extremely variable but modifiable via diet, a fact that allows targeting of microbes through defined dietary strategies to uncover cost-effective therapies to minimize the burden of non-communicable diseases such as pandemic obesity and overweight and its metabolic comorbidities. Nevertheless, randomly controlled dietary interventions regularly exhibit low to moderate degrees of success in weight control, making their implementation difficult in clinical practice. Here, we review the predictive value of the baseline gut microbiota configurations to anticipate the success of dietary interventions aimed at weight loss, mostly based on caloric restriction regimes and oral fiber supplementation. This emergent research concept fits into precision medicine by considering different diet patterns and adopting the best one, based on the individual microbiota composition, to reach significant adiposity reduction and improve metabolic status. We review the results from this fresh perspective of investigation, taking into account studies released very recently. We also discuss some future outlooks in the field and potential pitfalls to overcome with the aim of gaining knowledge in the field and achieving breakthroughs in personalized nutrition.
The human gut microbiota is gaining increasing attention in clinical practice, given the hundreds of reports published in recent years highlighting its contributing role in a wide variety of health states (1, 2). After almost two decades of intensive and rapidly evolving microbiome research, two major traits can undoubtedly be attributed to the gut microbiota. The first one is its profound interindividual influence, and the second one is the enormous influence that diet exerts on it. Regarding the first trait, the great interpersonal variability found in hundreds of human-associated gut microbiota surveys released in the scientific literature makes it difficult to distinguish unique microbial signatures associated with health and disease. Consequently, the so-called “dysbiosis” states presumably associated with several gastrointestinal, mental, and inflammatory maladies–for which causal agents have been examined in the gut microbiota–have an empty and ambiguous meaning (3–5) due to the impossibility of distinguishing concrete microbial communities underlying the disease from the vast variability expected across multiple human populations and settlements.
On the other hand, diet is probably the most important variable–over genetics and other environmental factors (6)–shaping the human intestinal microbiota. Therefore, dietary-based clinical trials constitute a promising scenario to tackle a wide variety of metabolic and inflammatory dysfunctions on the basis of modulating the gut microbiota and thus improving the host-microbe molecular interactions mechanistically demonstrated to modulate human health [e.g., short-chain fatty acids (SCFAs), which have effector roles]. Current investigation paradigms indicate that the Western diet (hypercaloric and enriched in saturated fat and refined sugars) increases opportunistic bacteria, bacterial metabolites (e.g., LPS, TMAO) and inflammatory cytokines while decreasing beneficial bacteria and SCFA production (7). Such a detrimental dietary pattern not only results in an increased risk of chronic diseases (e.g., cardiovascular diseases), but also represents a serious risk for neurodegenerative diseases due to the long-term neuroinflammation favoring the amyloid-β expression and deposition (8, 9). Conversely, plant-based diets, including the Mediterranean diet, are rich in fiber acting as prebiotics stimulating microbial growth in the human gut and are shown to increase SFCAs production and have anti-inflammatory properties, thus decreasing the risk of infectious and non-communicable diseases (7, 10).
Among the multiple macronutrients and dietary compounds and ingredients regularly used in randomized placebo-controlled clinical trials (RCTs) to assess their impact on human health (e.g., unsaturated fat, proteins of plant and animal origin, polyphenols, and whole microorganisms), complex carbohydrates, namely, oligo- and polysaccharides of plant origin, are one of the preferable strategies to modulate the gut microbiota. This preference is based on the lack of genes encoded in the human genome that produce enzymes that break these complex carbohydrates and the contrasting massive molecular circuits for degrading such compounds present in the gut microbiota genomes (11, 12). Such an expanded content of genes encoding carbohydrate-degrading enzymes grants some particular gut microbiota species with outstanding glycolytic versatility (13–15). Moreover, the metabolic products resulting from the microbial fermentation of those complex carbohydrates present in the diet, that is, the SCFAs, have been demonstrated to exert important modulation on host physiology, highlighting this wide variety of dietary fiber compounds as a therapeutic proxy to improve metabolic and immune dysfunctions through the gut microbiota. Nevertheless, the response to dietary interventions with fiber exhibits the same interindividual variability associated with gut microbiota profiling.
On the other hand, calorie-reduced dietary interventions are also repeatedly used in clinical trials to investigate weight loss strategies and to assess to what extent their physiological therapeutic benefits are derived from or translated to the gut microbiome. Caloric restriction diets (CRDs) are known to provide efficacy in reducing adiposity and improving organ function, thereby reducing the risk of non-communicable comorbid diseases (16, 17). Additionally, CRDs protect against host aging and age-related perturbations of the gut microbiota, which are changes that are often associated with pathogen boosting and a proinflammatory status and are therefore detrimental for the progression of several diseases, such as obesity, diabetes, neurological diseases and inflammatory bowel disease (IBD) (18, 19).
Although dietary patterns seem to influence to a larger extent the composition of the gut microbiota and host health by extension, the outcomes have thus far not provided a consensus regarding a particular dietary regimen that is optimal for weight-loss strategies and improving metabolic health. Consequently, the conceptualization of one-diet-fits-all becomes deprecated. Thus, a new perspective of analysis should be adopted with a personalized medicine approach, which can be ascertained via the evaluation of predefined or individualized microbiota profiles in subjects prior to starting dietary regimes aiming for weight loss. Therefore, establishing the links between microbes and dietary ingredients deserves special attention to design such a prospect.
According to the Codex Alimentarius definition, “dietary fiber consists of carbohydrate polymers with ten or more monomeric units, which are not hydrolyzed by the endogenous enzymes in the small intestine of humans” (20). Dietary fiber is present in whole grains, fruits, legumes, vegetables, seeds, and nuts. Nevertheless, it is also manufactured [e.g., most oligosaccharides (OSs)] by enzymatic processing of polysaccharides extracted from the food mentioned above or their transformation subproducts. Bacterial utilization preferences for certain oligo- and polysaccharide carbohydrates, their utilization priorities and their respective degradation kinetics not only support species coexistence from an ecological point of view (Bacteroides thetaiotaomicron vs. Akkermansia muciniphila) (21) but can also inform about the type of complex carbohydrates that can be used in dietary interventions depending on the microbiota present and their abundance. A particular line of investigation is emerging to comprehensively understand the metabolic circuits activated in a species-specific manner by single and complex carbohydrates, and this is of massive relevance to designing personalized medicine strategies based on nutrition.
Fiber is assumed to confer health benefits due to its physicochemical and structural properties (e.g., indigestibility and viscosity) that delay gastric emptying, inhibit nutrient absorption, decrease postprandial glycemic levels, and reduce body cholesterol stores (22). Since it is indigestible by human enzymes, dietary fiber reaches distal portions of the human colon, where it is fermented rapidly by microbes that thrive there to produce different SCFAs. Currently, this biotransformation process is the cornerstone for the design of dietary RCTs aiming to tackle a wide array of maladies affecting the human gastrointestinal tract (GIT), other critical organs, and health as a whole in several ways. The molecular basis underlying the therapeutic transversal approach of dietary fiber is that SCFAs produced in the colon modulate gut barrier integrity, glucose, and lipid metabolism; regulate the immune system, the inflammatory response, and blood pressure; and control enteroendocrine cell networks (23–25).
Conventional RCT design aims to test the effect of particular dietary fiber components and consequently utilizes high purity preparations to isolate their individual effect primarily on clinical parameter indicators of health states (e.g., body weight, blood pressure, glycemia, lipidemia, and motor and cognitive function), and secondary outcomes are frequently used to assess microbiota alterations. In this particular context, manufactured and purified OSs distributed in defined dosages for easy serving preparation are the classic clinical strategy to evaluate diet-based therapies on different diseases with altered gastrointestinal function. The wide diversity of dietary fiber compounds, for which health effects have been tested via RCTs, usually also exhibit an individual-specific response in terms of primary clinical outcomes assessed. Conversely, there is a unique quasi-consensus signal regarding secondary outcomes circumscribed to gut microbiota appraisal. As a general response, Bifidobacterium species seem to be predominantly boosted by dietary fiber, with no other consensus signatures captured for alternative species [reviewed in Benítez-Páez et al. (26) and Portuneet al. (27)]. This consistent pattern seen for Bifidobacterium species suggests that such beneficial-considered microbes would be the primary gut bacteria degrading the dietary fiber ingested. The above is supported by the fact that Bifidobacterium species contain a large number of specialized carbohydrate-active enzymes (CAZy) encoded in their genomes (28). Nevertheless, Bacteroides species exhibit the largest glycolytic capability observed in the human gut microbiota (26, 28, 29), which makes their null response in RCTs with dietary fiber perplexing. Hence, the difficulty in discerning other common patterns of gut microbiota modulation through dietary fiber intake could be explained by the Bifidobacteria themselves. Such species increase acetate production after dietary fiber fermentation and can lead to growth stimulation of other bacteria and SCFA production (30, 31). This cross-feeding process could also explain the variation in the gut microbiota response accounting for other multiple species that, in addition to being subjected to Bifidobacteria metabolic-driven interactions, would be strongly conditioned by pre-treatment individual-specific configurations in every RCT.
Although the paradigm of the interactions among the host, diet and microbiota seems to be sound and elegant because of its simplicity and its implications at the systemic level, there is still a great deal of complexity to be unraveled, where the production of the different SCFAs and their relative proportions depend strongly and jointly on the individual composition of the microbiota and the type of dietary fiber administered (32–34).
A Western diet is becoming the preferred dietary pattern in Western countries and is widespread in Middle-East and Eastern countries, representing a chronically overfed state and increasing health risk factors and non-communicable chronic diseases (35). These health conditions could lead to metabolic disorders related to the human microbiome, such as an increase in pathogenic gut bacteria, undesirable metabolites and gut permeability, as well as inflammation in the intestine, peripheral organs, and systemically (36). The CRD is one of the commonly used approaches for the treatment of obesity with notable weight loss success (37). The CRD is a nutritional approach that only reduces average daily calorie intake compared to ad libitum intake while maintaining acceptable macronutrient proportions (45–65% carbohydrates, 20–35% lipids, and proteins 10–35% in average), sometimes with an additional increase in protein or fiber intake. In general terms, CRD adopted in clinical trials consists in a reduction of 10–30% of caloric intake leaving an average energy intake 1,200–1,800 kcal/day. Globally, the deficit should be of 500–600 kcal/day over baseline caloric intake estimates. Evidence indicates that a CRD may provide a positive impact on gut microbiota diversity (38–40), barrier function, immune and inflammatory responses (41–43), and the production of postbiotic metabolites such as SCFAs (40). Moreover, a CRD has been shown to have an impact on the abundance of all the main phyla of the gut bacteria present in humans, such as Firmicutes, Bacteroidetes, Proteobacteria, Verrucomicrobia, and Actinobacteria [reviewed in Rinninella et al. (18)]. Nevertheless, as the long-term compliance with a CRD has its limitations, intermittent fasting (IF) or time-restricted feeding regimes consisting of alternating periods of food intake and periods of energy restriction, and limiting feeding to a few hours daily, respectively, have gained increasing attention for implementation during recent years, as they have revealed similar clinical benefits (44–47). Moreover, following a CRD for obese subjects has been shown to have the ability to adjust the gut microbiota composition to those of lean subjects, thus reducing the enormous baseline differences observed (48). Such treatment led to a shift in the gut microbiota composition, increasing the levels of Firmicutes while decreasing most other phyla and consequently increasing the production of SCFAs compared to those of the ad libitum controls (49). Clinical trials report that a CRD reduces the Bacteroidetes population in favor of Firmicutes (50). Additionally, in CRD-treated mammals, an overall increase in the relative abundance of probiotic microbes (e.g., Bifidobacterium and former Lactobacillus species) was detected, which may explain some of the benefits observed in the host (38, 51, 52). On the other hand, some inflammation-inducing microbes could be inhibited with CRD treatment (53), whereas other butyrate-producing microbial strains, such as Coprobacillus, Holdemania, Eubacterium cellulosolvens, and Clostridium saccharolyticum, seem to be boosted by CRDs (52).
The clinical reports reviewed here and listed in Table 1 were selected as follows: monthly searching in PubMed from October 2021 to July 2022 using the terms “baseline microbiota” OR “pre-treatment microbiota” AND “weight loss.” The retrieved list of publications was manually inspected to assess and explicitly disclose microbiome-based predictive traits for weight loss success of dietary interventions. From the final selected list of publications, their references citing microbiota-based predictive features for weight loss, not recovered from our regular searching, were also incorporated into this review.
Table 1. Summary of human studies investigating the predictive potential of the baseline microbiota on dietary intervention success.
As summarized in the previous sections, caloric restriction and fiber-based orally administered ingredients are the preferred weight-loss strategies in RCTs and seem to exert profound effects on gut microbiota configurations. However, the prominent feature of such controlled interventions is that no consensus outcomes are reached, and an elevated variability in weight reduction is seen among participants. Consequently, these results are prominently linked with gut microbiota profiling, given the strong influence of diet on intestinal microbiota structure.
Accumulating evidence regarding the interaction between the microbiota and weight-loss interventions in overweight (generally classified as BMI >25 kg/m2) or obese (BMI 28–30 kg/m2) adults reveals that differences in the intrinsic baseline gut microbial profile could likely play a conditioning role in the success of health interventions (54). In this regard, a novel concept in microbiome research that has emerged in the last 4 years includes considering the baseline microbiota as a predictor of weight loss success following a low-calorie diet in RCTs. However, some studies have looked at other perspectives, such as IF or fiber supplementation. The characteristics of the collected studies and their specific results are listed in Table 1, and an outline of the primary outcomes with the related taxa is presented in Figure 1.
Figure 1. Schematic results of all the clinical trials reviewed. Taxa in green indicate a positive predictive manner, and taxa in red indicate a negative predictive manner. Dietary interventions and their influence on obesity-related metabolic parameters with a focus on weight loss as well as the correlated gut microbiota taxa at the genus or species level. CR, caloric restriction; IF, intermittent fasting; LCD, low-carbohydrate diet.
Bacteroides and Prevotella species are the most studied microbial genera, probably because they are the dominant stable clusters of bacterial communities in the human gut. Researchers from the University of Copenhagen were pioneers who inferred weight-loss success in RCTs using such features and have focused their research on personalized dietary strategies with an “enterotype” perspective, emphasizing the importance of the Prevotella/Bacteroides balance (54). From the information gathered from genomic analyses and targeted in vitro studies, Prevotella species seem to favor fiber degradation over their Bacteroides counterparts, and they are associated with better weight modulation in response to increased dietary fiber intake. As a proof of concept, the Prevotella abundance at baseline has been shown to be a positive predictive biomarker for the success of weight loss (55). In contrast, a high abundance of Bacteroides cellulosilyticus has been found to be a potential predictor for failure of weight-loss intervention following oral administration of arabinoxylan-oligosaccharides (AXOS) (56). Similarly, the baseline abundance of Bacteroides fragilis or Bacteroides ovatus was negatively related to weight loss after a calorie restriction intervention with fiber supplementation in a previous multiomics study completed by our group (40). Nevertheless, other authors have described Bacteroides species as a positive predictor of individual weight loss after a short-term low-carbohydrate dietary intervention (57). In accordance with this, Bacteroides dorei (together with Blautia wexlerae) was found to be a strong predictor for weight loss when present in higher relative abundance at baseline before following a high-carbohydrate and high-protein CRD (58). These contrasting results regarding the Bacteroides genus may be due to differences in methodology, study design and population characteristics, but it is also necessary to obtain a more detailed microbiota profiling outlook at the species level.
Bearing this in mind, to obtain more precise insight into how the baseline microbiota can be predictive, we focused on studies aimed at the evidence at the species level. Nonetheless, research that tackles that perspective is currently insufficient, and there are considerable heterogeneities in study design, population and methodologies. Furthermore, current methods are not sufficiently discriminatory to discern between closely related species, and the genetic and metabolic variability revealed in recent years indicates that identification at the subspecies level could also be a critical aspect to take into account for predictive aims (e.g., Prevotella copri clades, Eubacterium hallii clusters and A. muciniphila subspecies) (59–61) and should offer a more intricate perspective on predictions aimed at designing personalized medicine strategies.
Beyond the Prevotella/Bacteroides ratio, a low abundance of the Dorea genus at baseline has been shown to be predictive of subsequent weight loss following intermittent calorie restriction, nullifying the predictive capability of the Prevotella/Bacteroides ratio or gut microbiota richness in this particular nutritional context (62). The predictive value of the Dorea species abundance (Dorea longicatena) was also supported, but in different directions, by its positive correlation with the BMI loss ratio after CRD intervention (63). This type of disparity in results is quite frequent in microbiota assessment under particular nutritional regimes and could be the result of the technical limitations when defining species-level taxonomy associations. In addition, when CRD and IF interventions are compared, they also produce discrepancies in the predictive value of certain taxonomy features. For instance, the abundance of Subdoligranulum species was associated with more significant weight loss only after IF intervention (64). A short-term weight loss intervention based on a CRD reducing caloric intake by 34% showed improved weight reduction linked to Coprococcus species baseline abundance, while Bacteroides and Lachnospiraceae species were negatively related to waist circumference reduction (65).
From a different perspective, Dong and coworkers reported significantly different outcomes related to the variability between patients with at least 5% weight loss on a calorie-restricted diet compared to those with less response. The former group had less Escherichia/Shigella, Klebsiella, Megasphaera, Sellimonas, and Lactobacillus and more Collinsella as well as an unidentified genus from the Christensenellaceae family compared to those that did not respond as well to a calorie-restricted diet. In addition, Actinomyces was shown to be a negative predictor for weight loss following the given intervention, and it seemed to be predictive of the development of hepatic steatosis (66).
A recently published study did not find baseline microbiota to be a good predictor of weight loss, but it showed that the baseline microbiota was a good predictor of indirect measures of weight loss, such as fat mass lost, after a high-calorie restriction regimen with a high-protein diet. Jian et al. (67) observed that high relative abundances of Clostridium sensu stricto, Erysipelotrichaceae and Parabacteroides species were negatively correlated with fat mass loss. Similarly, other authors have focused more on different outcomes that frequently accompany weight loss as the metabolic parameters improve. However, those clinical outcomes of interest also seem predictable using baseline gut microbiota and diet configurations, which allows us to broaden the possibilities of where the basal microbiota could play an important role as a biomarker. For instance, Tettamanzi et al. detected at least ten microbial species, including Eubacterium xylanophilum, Clostridium sensu stricto, E. hallii, and Lachnoclostridium, suggesting that they play an important role in host glucose homeostasis. Coprococcus, for example, might exert a positive effect through the production of SCFAs. Moreover, Lachnoclostridium species might metabolize precursors of trimethylamine and its oxide, which negatively regulate glucose metabolism and insulin sensitivity (68). Another study assessed baseline characteristics to predict the postprandial glucose response and revealed that the relative abundance of Faecalibacterium is negatively associated with plasma glucose after the consumption of high- and low-resistant starch (69). This finding could be of special interest since glucose metabolism impairment is one of the most common and problematic issues in overweight and obese patients.
The well-recognized beneficial human gut microbe A. muciniphila has also been identified as a diagnostic or prognostic tool in the context of predicting dietary intervention success. Consequently, A. muciniphila abundance at baseline showed correlations with improvements in body fat distribution, fasting plasma glucose, plasma triglycerides and insulin sensitivity after a CRD (70).
Nevertheless, not all studies are directed toward clarifying the relationship between the baseline gut microbiota and CRD or weight loss strategies. Strikingly, a randomized crossover trial published in 2021 aimed to study the effects of short-term overfeeding on the human gut microbiota and liver steatosis and showed that fat accumulation in the liver is linked to a higher baseline abundance of Bilophila species (71).
Current research indicates that the baseline microbiota may act as a predictor of weight loss, which could have implications for clinical practice such that we could use the microbiota as a biomarker for predicting successful clinical interventions in the future. In summary, we have observed that elucidation of predictive baseline microbiota traits for weight loss dietary strategies is variable, and no consensus signals can be intuited. Undoubtedly, such an observation likely results from the recognized inter-individual variability of gut microbiota profiles and the variability of weight loss diets explored in this review. The literature reviewed allows us to hypothesize that we can target the microbiota with some dietary interventions using high-fiber or calorie restriction regimes to modulate the microbiota and improve the outcomes of proposed cost-effective therapies for weight loss. However, the association between the predictive traits of the baseline gut microbiota and the success of dietary interventions requires more research. In this regard, we have recently described that a sex-based perspective should be adopted in all RCTs to improve the precision of predictions. We have found, from a multiomic perspective, that women and men responded disparately to a CRD regimen with fiber supplementation (72). Furthermore, the recent work published by Cuevas-Sierra and coworkers analyzed the baseline microbiota predictive value for weight loss dietary strategies upon low-fat and high-protein regimes separately. When sex was included in the data modeling, the observed predictions were notably improved (73). The above results are strongly suggestive of directions to adopt in future studies. The recognized influence of specific covariates, directly or indirectly linked to dietary patterns, stresses the importance of considering factors such as sex, age and nutritional habits to improve biomarker predictions. Altogether, integration of such covariates into the predictive models will permit the creation of more robust associations and defining discrete diets for individuals depending on their gut microbiota configuration to reach weight loss; thus we will move toward abandoning of the one-diet-fits-all concept, as well as the delineation of personalized nutrition in the context of precision medicine to improve weight loss strategies and reduce the burden of non-communicable disease associated with obesity and overweight in human populations.
We want to express our conviction that there is an evident need to improve microbiota profiling in clinical and preclinical studies toward describing taxonomy inventories at the species level and minimizing potential issues during biocomputational processing of microbiome data. The level of technical and methodological information found in most microbiome studies is insufficient and does not contribute positively to solving contrasting and disparate results frequently found in the literature. Important initiatives have emerged to tackle such pitfalls, including the introduction of the STORMS Checklist as a guideline for microbiota assessments in clinical studies (74). However, beyond the issues the above-claimed standard will solve, there is an underlying technical limitation to microbiota profiling assessments, which makes it unsuitable to reach the level of detail currently required and for the advances in this research. For example, data generated via the short-read sequencing of 16S rRNA gene amplicons, widely used in microbiome surveys, cannot reach a remarkable proportion of annotations at the species level. The only technology able to provide high-quality information in this regard is shotgun sequencing, which is more expensive and makes studies with a large set of samples unfeasible. Consequently, steps forward are needed to establish a middle-ground approach that will permit taxonomy resolution with lower costs. In this regard, our group has proposed a cost-effective strain-level assessment using rrn long-amplicons as a promising technique, which could help to solve the issues stated above (72, 75). Moreover, a very comprehensive repository is also available to complete this advanced method of analysis (76).
With the proper control of covariation in microbiota data using host-associated variables and improvements in the annotation of species, we should advance the knowledge and better explain the variability in the baseline characteristics predictive of success of different strategies. Although such variability could correspond and be representative of the well-known individual characteristics of the microbiota configurations, the metabolic landscape set and guided by those predictive traits cannot be disregarded since functional redundancy is also characteristic of gut microbiomes (77). With the resolution, at the species or strain level, of predictive microbes that permit us to anticipate the result of a diet-based weight-loss strategy, we could start to define carbohydrate (simple or complex) preferences in such species to disclose the metabolic landscapes in different nutritional environments guiding adiposity reduction. Consequently, future studies to define complex carbohydrate utilization preferences by gut microbes (15, 78), evaluate different effector molecules produced by gut microbes (33, 34), and assess the production of SCFAs depending on fiber ingredients and the physiological environment (32) will all be necessary to integrate such knowledge into clinical practice.
AB-P conceived and coordinated this mini-review. All authors compiled and critically analyzed the clinical trial studies reviewed in this work.
We thank the Spanish Carlos III Health Institute (ISCIII) and its funds from the European Social Fund (ESF/FSE) for the CP19/00132 grant to AB-P. We also thank the CIPF Predoctoral Training Fellowships 2022, funded by Janssen-Cilag–supporting the PHC contract–and the European Union ERASMUS+ Program supporting the LW fellowship at the CIPF Institute.
The authors declare that the research was conducted in the absence of any commercial or financial relationships that could be construed as a potential conflict of interest.
All claims expressed in this article are solely those of the authors and do not necessarily represent those of their affiliated organizations, or those of the publisher, the editors and the reviewers. Any product that may be evaluated in this article, or claim that may be made by its manufacturer, is not guaranteed or endorsed by the publisher.
1. De Vos WM, Tilg H, Van Hul M, Cani PD. Gut microbiome and health: mechanistic insights. Gut. (2022) 71:1020–32. doi: 10.1136/gutjnl-2021-326789
2. Sorbara MT, Pamer EG. Microbiome-based therapeutics. Nat Rev Microbiol. (2022) 20:365–80. doi: 10.1038/s41579-021-00667-9
3. Olesen SW, Alm EJ. Dysbiosis is not an answer. Nat Microbiol. (2016) 1:16228. doi: 10.1038/nmicrobiol.2016.228
4. Hooks KB, O’malley MA. Dysbiosis and its discontents. mBio. (2017) 8:e1492–1417. doi: 10.1128/mBio.01492-17
5. Brussow H. Problems with the concept of gut microbiota dysbiosis. Microb Biotechnol. (2020) 13:423–34. doi: 10.1111/1751-7915.13479
6. Rothschild D, Weissbrod O, Barkan E, Kurilshikov A, Korem T, Zeevi D, et al. Environment dominates over host genetics in shaping human gut microbiota. Nature. (2018) 555:210–5. doi: 10.1038/nature25973
7. Beam A, Clinger E, Hao L. Effect of diet and dietary components on the composition of the gut microbiota. Nutrients. (2021) 13:2795. doi: 10.3390/nu13082795
8. Bianchi VE, Herrera PF, Laura R. Effect of nutrition on neurodegenerative diseases. A systematic review. Nutr Neurosci. (2021) 24:810–34.
9. Bianchi VE, Rizzi L, Somaa F. The role of nutrition on Parkinson’s disease: a systematic review. Nutr Neurosci. (2022) 25:1–24. doi: 10.1080/1028415X.2022.2073107
10. Merra G, Noce A, Marrone G, Cintoni M, Tarsitano MG, Capacci A, et al. Influence of mediterranean diet on human gut microbiota. Nutrients. (2020) 13:7. doi: 10.3390/nu13010007
11. El Kaoutari A, Armougom F, Gordon JI, Raoult D, Henrissat B. The abundance and variety of carbohydrate-active enzymes in the human gut microbiota. Nat Rev Microbiol. (2013) 11:497–504. doi: 10.1038/nrmicro3050
12. Grondin JM, Tamura K, Dejean G, Abbott DW, Brumer H. Polysaccharide utilization loci: fueling microbial communities. J Bacteriol. (2017) 199:e860–816. doi: 10.1128/JB.00860-16
13. Martens EC, Lowe EC, Chiang H, Pudlo NA, Wu M, Mcnulty NP, et al. Recognition and degradation of plant cell wall polysaccharides by two human gut symbionts. PLoS Biol. (2011) 9:e1001221. doi: 10.1371/journal.pbio.1001221
14. Benitez-Paez A, Gomez Del Pulgar EM, Sanz Y. The glycolytic versatility of Bacteroides uniformis CECT 7771 and its genome response to oligo and polysaccharides. Front Cell Infect Microbiol. (2017) 7:383. doi: 10.3389/fcimb.2017.00383
15. Tuncil YE, Xiao Y, Porter NT, Reuhs BL, Martens EC, Hamaker BR. Reciprocal prioritization to dietary glycans by gut bacteria in a competitive environment promotes stable coexistence. mBio. (2017) 8:e1068–1017. doi: 10.1128/mBio.01068-17
16. Fontana L, Partridge L. Promoting health and longevity through diet: from model organisms to humans. Cell. (2015) 161:106–18. doi: 10.1016/j.cell.2015.02.020
17. Pifferi F, Terrien J, Perret M, Epelbaum J, Blanc S, Picq JL, et al. Promoting healthspan and lifespan with caloric restriction in primates. Commun Biol. (2019) 2:107. doi: 10.1038/s42003-019-0348-z
18. Rinninella E, Cintoni M, Raoul P, Ianiro G, Laterza L, Lopetuso LR, et al. Gut microbiota during dietary restrictions: new insights in non-communicable diseases. Microorganisms. (2020) 8:1140. doi: 10.3390/microorganisms8081140
19. Schmidt NS, Lorentz A. Dietary restrictions modulate the gut microbiota: implications for health and disease. Nutr Res. (2021) 89:10–22. doi: 10.1016/j.nutres.2021.03.001
20. Jones JM. CODEX-aligned dietary fiber definitions help to bridge the ‘fiber gap’. Nutr J. (2014) 13:34. doi: 10.1186/1475-2891-13-34
21. Kostopoulos I, Aalvink S, Kovatcheva-Datchary P, Nijsse B, Backhed F, Knol J, et al. A continuous battle for host-derived glycans between a mucus specialist and a glycan generalist in vitro and in vivo. Front Microbiol. (2021) 12:632454. doi: 10.3389/fmicb.2021.632454
22. Dikeman CL, Fahey GC. Viscosity as related to dietary fiber: a review. Crit Rev Food Sci Nutr. (2006) 46:649–63. doi: 10.1080/10408390500511862
23. Modasia A, Parker A, Jones E, Stentz R, Brion A, Goldson A, et al. Regulation of enteroendocrine cell networks by the major human gut symbiont Bacteroides thetaiotaomicron. Front Microbiol. (2020) 11:575595. doi: 10.3389/fmicb.2020.575595
24. Nogal A, Valdes AM, Menni C. The role of short-chain fatty acids in the interplay between gut microbiota and diet in cardio-metabolic health. Gut Microbes. (2021) 13:1–24. doi: 10.1080/19490976.2021.1897212
25. Rauf A, Khalil AA, Rahman UU, Khalid A, Naz S, Shariati MA, et al. Recent advances in the therapeutic application of short-chain fatty acids (SCFAs): an updated review. Crit Rev Food Sci Nutr. (2021) 62:1–21. doi: 10.1080/10408398.2021.1895064
26. Benítez-Páez A, Gómez Del Pulgar EM, Kjølbæk L, Brahe LK, Astrup A, Larsen L, et al. Impact of dietary fiber and fat on gut microbiota re-modeling and metabolic health. Trends Food Sci Technol. (2016) 57:201–12. doi: 10.1016/j.tifs.2016.11.001
27. Portune KJ, Benítez-Páez A, Del Pulgar EM, Cerrudo V, Sanz Y Gut microbiota, diet, and obesity-related disorders-the good, the bad, and the future challenges. Mol Nutr Food Res. (2017) 61:e1600252. doi: 10.1002/mnfr.201600252
28. Bhattacharya T, Ghosh TS, Mande SS. Global profiling of carbohydrate active enzymes in human gut microbiome. PLoS One. (2015) 10:e0142038. doi: 10.1371/journal.pone.0142038
29. Aakko J, Pietila S, Toivonen R, Rokka A, Mokkala K, Laitinen K, et al. A carbohydrate-active enzyme (CAZy) profile links successful metabolic specialization of Prevotella to its abundance in gut microbiota. Sci Rep. (2020) 10:12411. doi: 10.1038/s41598-020-69241-2
30. Rios-Covian D, Gueimonde M, Duncan SH, Flint HJ, De Los Reyes-Gavilan CG. Enhanced butyrate formation by cross-feeding between Faecalibacterium prausnitzii and Bifidobacterium adolescentis. FEMS Microbiol Lett. (2015) 362:fnv176. doi: 10.1093/femsle/fnv176
31. Louis P, Flint HJ. Formation of propionate and butyrate by the human colonic microbiota. Environ Microbiol. (2017) 19:29–41. doi: 10.1111/1462-2920.13589
32. Reichardt N, Vollmer M, Holtrop G, Farquharson FM, Wefers D, Bunzel M, et al. Specific substrate-driven changes in human faecal microbiota composition contrast with functional redundancy in short-chain fatty acid production. ISME J. (2018) 12:610–22. doi: 10.1038/ismej.2017.196
33. Deehan EC, Yang C, Perez-Munoz ME, Nguyen NK, Cheng CC, Triador L, et al. Precision microbiome modulation with discrete dietary fiber structures directs short-chain fatty acid production. Cell Host Microbe. (2020) 27:389–404e6. doi: 10.1016/j.chom.2020.01.006
34. Delannoy-Bruno O, Desai C, Raman AS, Chen RY, Hibberd MC, Cheng J, et al. Evaluating microbiome-directed fibre snacks in gnotobiotic mice and humans. Nature. (2021) 595:91–5. doi: 10.1038/s41586-021-03671-4
35. Pressler M, Devinsky J, Duster M, Lee JH, Glick CS, Wiener S, et al. Dietary transitions and health outcomes in four populations - systematic review. Front Nutr. (2022) 9:748305. doi: 10.3389/fnut.2022.748305
36. Mohr AE, Gumpricht E, Sears DD, Sweazea KL. Recent advances and health implications of dietary fasting regimens on the gut microbiome. Am J Physiol Gastrointest Liver Physiol. (2021) 320:G847–63. doi: 10.1152/ajpgi.00475.2020
37. Chao AM, Quigley KM, Wadden TA. Dietary interventions for obesity: clinical and mechanistic findings. J Clin Invest. (2021) 131:e140065. doi: 10.1172/JCI140065
38. Fraumene C, Manghina V, Cadoni E, Marongiu F, Abbondio M, Serra M, et al. Caloric restriction promotes rapid expansion and long-lasting increase of Lactobacillus in the rat fecal microbiota. Gut Microbes. (2018) 9:104–14. doi: 10.1080/19490976.2017.1371894
39. Tanca A, Abbondio M, Palomba A, Fraumene C, Marongiu F, Serra M, et al. Caloric restriction promotes functional changes involving short-chain fatty acid biosynthesis in the rat gut microbiota. Sci Rep. (2018) 8:14778. doi: 10.1038/s41598-018-33100-y
40. Benitez-Paez A, Hess AL, Krautbauer S, Liebisch G, Christensen L, Hjorth MF, et al. Sex, food, and the gut microbiota: disparate response to caloric restriction diet with fiber supplementation in women and men. Mol Nutr Food Res. (2021) 65:e2000996. doi: 10.1002/mnfr.202000996
41. Meydani SN, Das SK, Pieper CF, Lewis MR, Klein S, Dixit VD, et al. Long-term moderate calorie restriction inhibits inflammation without impairing cell-mediated immunity: a randomized controlled trial in non-obese humans. Aging (Albany NY). (2016) 8:1416–31. doi: 10.18632/aging.100994
42. Ott B, Skurk T, Hastreiter L, Lagkouvardos I, Fischer S, Buttner J, et al. Effect of caloric restriction on gut permeability, inflammation markers, and fecal microbiota in obese women. Sci Rep. (2017) 7:11955. doi: 10.1038/s41598-017-12109-9
43. Park CY, Park S, Kim MS, Kim HK, Han SN. Effects of mild calorie restriction on lipid metabolism and inflammation in liver and adipose tissue. Biochem Biophys Res Commun. (2017) 490:636–42. doi: 10.1016/j.bbrc.2017.06.090
44. Trepanowski JF, Kroeger CM, Barnosky A, Klempel MC, Bhutani S, Hoddy KK, et al. Effect of alternate-day fasting on weight loss, weight maintenance, and cardioprotection among metabolically healthy obese adults: a randomized clinical trial. JAMA Intern Med. (2017) 177:930–8. doi: 10.1001/jamainternmed.2017.0936
45. Sundfor TM, Svendsen M, Tonstad S. Effect of intermittent versus continuous energy restriction on weight loss, maintenance and cardiometabolic risk: a randomized 1-year trial. Nutr Metab Cardiovasc Dis. (2018) 28:698–706. doi: 10.1016/j.numecd.2018.03.009
46. Rynders CA, Thomas EA, Zaman A, Pan Z, Catenacci VA, Melanson EL. Effectiveness of intermittent fasting and time-restricted feeding compared to continuous energy restriction for weight loss. Nutrients. (2019) 11:2442. doi: 10.3390/nu11102442
47. Liu D, Huang Y, Huang C, Yang S, Wei X, Zhang P, et al. Calorie restriction with or without time-restricted eating in weight loss. N Engl J Med. (2022) 386:1495–504. doi: 10.1056/NEJMoa2114833
48. Ruiz A, Cerdo T, Jauregui R, Pieper DH, Marcos A, Clemente A, et al. One-year calorie restriction impacts gut microbial composition but not its metabolic performance in obese adolescents. Environ Microbiol. (2017) 19:1536–51. doi: 10.1111/1462-2920.13713
49. Klement RJ, Pazienza V. Impact of different types of diet on gut microbiota profiles and cancer prevention and treatment. Medicina (Kaunas). (2019) 55:84. doi: 10.3390/medicina55040084
50. Damms-Machado A, Mitra S, Schollenberger AE, Kramer KM, Meile T, Konigsrainer A, et al. Effects of surgical and dietary weight loss therapy for obesity on gut microbiota composition and nutrient absorption. Biomed Res Int. (2015) 2015:806248. doi: 10.1155/2015/806248
51. Zhang C, Li S, Yang L, Huang P, Li W, Wang S, et al. Structural modulation of gut microbiota in life-long calorie-restricted mice. Nat Commun. (2013) 4:2163. doi: 10.1038/ncomms3163
52. Russo M, Fabersani E, Abeijon-Mukdsi MC, Ross R, Fontana C, Benitez-Paez A, et al. Lactobacillus fermentum CRL1446 ameliorates oxidative and metabolic parameters by increasing intestinal feruloyl esterase activity and modulating microbiota in caloric-restricted mice. Nutrients. (2016) 8:415. doi: 10.3390/nu8070415
53. Hess AL, Benitez-Paez A, Blaedel T, Larsen LH, Iglesias JR, Madera C, et al. The effect of inulin and resistant maltodextrin on weight loss during energy restriction: a randomised, placebo-controlled, double-blinded intervention. Eur J Nutr. (2020) 59:2507–24. doi: 10.1007/s00394-019-02099-x
54. Roager HM, Christensen LH. Personal diet-microbiota interactions and weight loss. Proc Nutr Soc. (2022) 81:1–12. doi: 10.1017/S0029665122000805
55. Christensen L, Vuholm S, Roager HM, Nielsen DS, Krych L, Kristensen M, et al. Prevotella abundance predicts weight loss success in healthy, overweight adults consuming a whole-grain diet ad libitum: a post hoc analysis of a 6-Wk randomized controlled trial. J Nutr. (2019) 149:2174–81. doi: 10.1093/jn/nxz198
56. Christensen L, Sorensen CV, Wohlk FU, Kjolbaek L, Astrup A, Sanz Y, et al. Microbial enterotypes beyond genus level: Bacteroides species as a predictive biomarker for weight change upon controlled intervention with arabinoxylan oligosaccharides in overweight subjects. Gut Microbes. (2020) 12:1847627. doi: 10.1080/19490976.2020.1847627
57. Zhang S, Wu P, Tian Y, Liu B, Huang L, Liu Z, et al. Gut microbiota serves a predictable outcome of short-term low-carbohydrate diet (LCD) intervention for patients with obesity. Microbiol Spectr. (2021) 9:e0022321. doi: 10.1128/Spectrum.00223-21
58. Jie Z, Yu X, Liu Y, Sun L, Chen P, Ding Q, et al. The baseline gut microbiota directs dieting-induced weight loss trajectories. Gastroenterology. (2021) 160:2029–2042e16. doi: 10.1053/j.gastro.2021.01.029
59. Tett A, Huang KD, Asnicar F, Fehlner-Peach H, Pasolli E, Karcher N, et al. The Prevotella copri complex comprises four distinct clades underrepresented in westernized populations. Cell Host Microbe. (2019) 26:666–679e7. doi: 10.1016/j.chom.2019.08.018
60. Karcher N, Pasolli E, Asnicar F, Huang KD, Tett A, Manara S, et al. Analysis of 1321 Eubacterium rectale genomes from metagenomes uncovers complex phylogeographic population structure and subspecies functional adaptations. Genome Biol. (2020) 21:138. doi: 10.1186/s13059-020-02042-y
61. Karcher N, Nigro E, Puncochar M, Blanco-Miguez A, Ciciani M, Manghi P, et al. Genomic diversity and ecology of human-associated Akkermansia species in the gut microbiome revealed by extensive metagenomic assembly. Genome Biol. (2021) 22:209. doi: 10.1186/s13059-021-02427-7
62. Sowah SA, Milanese A, Schubel R, Wirbel J, Kartal E, Johnson TS, et al. Calorie restriction improves metabolic state independently of gut microbiome composition: a randomized dietary intervention trial. Genome Med. (2022) 14:30. doi: 10.1186/s13073-022-01030-0
63. Zou H, Wang D, Ren H, Cai K, Chen P, Fang C, et al. Effect of caloric restriction on bmi, gut microbiota, and blood amino acid levels in non-obese adults. Nutrients. (2020) 12:631. doi: 10.3390/nu12030631
64. Stanislawski MA, Frank DN, Borengasser SJ, Ostendorf DM, Ir D, Jambal P, et al. The gut microbiota during a behavioral weight loss intervention. Nutrients. (2021) 13:3248. doi: 10.3390/nu13093248
65. Siebert JC, Stanislawski MA, Zaman A, Ostendorf DM, Konigsberg IR, Jambal P, et al. Multiomic predictors of short-term weight loss and clinical outcomes during a behavioral-based weight loss intervention. Obesity (Silver Spring). (2021) 29:859–69. doi: 10.1002/oby.23127
66. Dong TS, Luu K, Lagishetty V, Sedighian F, Woo SL, Dreskin BW, et al. The intestinal microbiome predicts weight loss on a calorie-restricted diet and is associated with improved hepatic steatosis. Front Nutr. (2021) 8:718661. doi: 10.3389/fnut.2021.718661
67. Jian C, Silvestre MP, Middleton D, Korpela K, Jalo E, Broderick D, et al. Gut microbiota predicts body fat change following a low-energy diet: a PREVIEW intervention study. Genome Med. (2022) 14:54. doi: 10.1186/s13073-022-01053-7
68. Tettamanzi F, Bagnardi V, Louca P, Nogal A, Monti GS, Mambrini SP, et al. A high protein diet is more effective in improving insulin resistance and glycemic variability compared to a mediterranean diet-a cross-over controlled inpatient dietary study. Nutrients. (2021) 13:4380. doi: 10.3390/nu13124380
69. Nolte Fong JV, Miketinas D, Moore LW, Nguyen DT, Graviss EA, Ajami N, et al. Precision nutrition model predicts glucose control of overweight females following the consumption of potatoes high in resistant starch. Nutrients. (2022) 14:268. doi: 10.3390/nu14020268
70. Dao MC, Everard A, Aron-Wisnewsky J, Sokolovska N, Prifti E, Verger EO, et al. Akkermansia muciniphila and improved metabolic health during a dietary intervention in obesity: relationship with gut microbiome richness and ecology. Gut. (2016) 65:426–36. doi: 10.1136/gutjnl-2014-308778
71. Jian C, Luukkonen P, Sadevirta S, Yki-Jarvinen H, Salonen A. Impact of short-term overfeeding of saturated or unsaturated fat or sugars on the gut microbiota in relation to liver fat in obese and overweight adults. Clin Nutr. (2021) 40:207–16. doi: 10.1016/j.clnu.2020.05.008
72. Benitez-Paez A, Hartstra AV, Nieuwdorp M, Sanz Y. Species- and strain-level assessment using rrn long-amplicons suggests donor’s influence on gut microbial transference via fecal transplants in metabolic syndrome subjects. Gut Microbes. (2022) 14:2078621. doi: 10.1080/19490976.2022.2078621
73. Cuevas-Sierra A, Milagro FI, Guruceaga E, Cuervo M, Goni L, Garcia-Granero M, et al. A weight-loss model based on baseline microbiota and genetic scores for selection of dietary treatments in overweight and obese population. Clin Nutr. (2022) 41:1712–23. doi: 10.1016/j.clnu.2022.06.008
74. Mirzayi C, Renson A, Genomic Standards C, Massive A, Quality Control S, Zohra F, et al. Reporting guidelines for human microbiome research: the STORMS checklist. Nat Med. (2021) 27:1885–92. doi: 10.1038/s41591-021-01552-x
75. Benitez-Paez A, Sanz Y. Multi-locus and long amplicon sequencing approach to study microbial diversity at species level using the MinION portable nanopore sequencer. Gigascience. (2017) 6:1–12. doi: 10.1093/gigascience/gix043
76. Seol D, Lim JS, Sung S, Lee YH, Jeong M, Cho S, et al. Microbial identification using rRNA operon region: database and tool for metataxonomics with long-read sequence. Microbiol Spectr. (2022) 10:e0201721. doi: 10.1128/spectrum.02017-21
77. Moya A, Ferrer M. Functional redundancy-induced stability of gut microbiota subjected to disturbance. Trends Microbiol. (2016) 24:402–13. doi: 10.1016/j.tim.2016.02.002
78. Deehan EC, Zhang Z, Riva A, Armet AM, Perez-Munoz ME, Nguyen NK, et al. Elucidating the role of the gut microbiota in the physiological effects of dietary fiber. Microbiome. (2022) 10:77. doi: 10.1186/s40168-022-01248-5
Keywords: weight loss, dietary interventions, obesity, metabolic disease, gut microbiota, personalized nutrition
Citation: Hernández-Calderón P, Wiedemann L and Benítez-Páez A (2022) The microbiota composition drives personalized nutrition: Gut microbes as predictive biomarkers for the success of weight loss diets. Front. Nutr. 9:1006747. doi: 10.3389/fnut.2022.1006747
Received: 29 July 2022; Accepted: 05 September 2022;
Published: 23 September 2022.
Edited by:
Silvia Turroni, University of Bologna, ItalyReviewed by:
Faiga Magzal, MIGAL - Galilee Research Institute, IsraelCopyright © 2022 Hernández-Calderón, Wiedemann and Benítez-Páez. This is an open-access article distributed under the terms of the Creative Commons Attribution License (CC BY). The use, distribution or reproduction in other forums is permitted, provided the original author(s) and the copyright owner(s) are credited and that the original publication in this journal is cited, in accordance with accepted academic practice. No use, distribution or reproduction is permitted which does not comply with these terms.
*Correspondence: Alfonso Benítez-Páez, YWJlbml0ZXpAY2lwZi5lcw==
†These authors have contributed equally to this work and share first authorship
‡ORCID: Paula Hernández-Calderón, orcid.org/0000-0002-5897-2078; Lara Wiedemann, orcid.org/0000-0003-0301-6721; Alfonso Benítez-Páez, orcid.org/0000-0001-5707-4340
Disclaimer: All claims expressed in this article are solely those of the authors and do not necessarily represent those of their affiliated organizations, or those of the publisher, the editors and the reviewers. Any product that may be evaluated in this article or claim that may be made by its manufacturer is not guaranteed or endorsed by the publisher.
Research integrity at Frontiers
Learn more about the work of our research integrity team to safeguard the quality of each article we publish.