- 1State Key Laboratory of Sheep Genetic Improvement and Healthy Production, Xinjiang Academy of Agricultural and Reclamation Sciences, Shihezi, China
- 2Institute of Animal Science, State Key Laboratory of Animal Nutrition, Chinese Academy of Agricultural Sciences (CAAS), Beijing, China
- 3College of Animal Science and Technology, Yangzhou University, Yangzhou, China
- 4Chinese Academy of Agricultural Sciences-World Agroforestry Centre (CAAS-ICRAF) Joint Lab on Agroforestry and Sustainable Animal Husbandry, World Agroforestry Center, East and Central Asia, Beijing, China
- 5Department of Animal Sciences and Division of Nutritional Sciences, University of Illinois, Urbana, IL, United States
Gut hormones are not only able to regulate digestive, absorptive, and immune mechanisms of the intestine through biological rhythms, but impact the host through their interactions with intestinal microorganisms. Whether hormones in ruminal fluid have an association with the ruminal ecology is unknown. Objectives of the study were to examine relationships between the diurnal change in ruminal hormones and microbiota in lactating cows, and their associations in vivo and in vitro. For the in vivo study, six cows of similar weight (566.8 ± 19.6 kg), parity (3.0 ± 0.0), and milk performance (8,398.7 ± 1,392.9 kg/y) were used. They were adapted to natural light for 2 weeks before sampling and fed twice daily at 07:00 a.m. and 14:00 p.m. Serum, saliva, and ruminal fluid samples were collected at 02:00, 10:00, and 18:00 on the first day and 06:00, 14:00, and 22:00 on the second day of the experimental period. The concentrations of melatonin (MLT), growth hormone (GH), and prolactin (PRL) were measured via radioimmunoassay, whereas amplicon sequencing data were used to analyze relative abundance of microbiota in ruminal fluid. JTK_CYCLE analysis was performed to analyze circadian rhythms of hormone concentrations as well as the relative abundance of microbiota. For the in vitro study, exogenous MLT (9 ng) was added into ruminal fluid incubations to investigate the impacts of MLT on ruminal microbiota. The results not only showed that rumen fluid contains MLT, but the diurnal variation of MLT and the relative abundance of 9% of total rumen bacterial operational taxonomic units (OTUs) follow a circadian rhythm. Although GH and PRL were also detected in ruminal fluid, there was no obvious circadian rhythm in their concentrations. Ruminal MLT was closely associated with Muribaculaceae, Succinivibrionaceae, Veillonellaceae, and Prevotellaceae families in vivo. In vitro, these families were significantly influenced by melatonin treatment, as melatonin treatment increased the relative abundance of families Prevotellaceae, Muribaculaceae while it reduced the relative abundance of Succinivibrionaceae, Veillonellaceae. Collectively, ruminal microbes appear to maintain a circadian rhythm that is associated with the profiles of melatonin. As such, data suggest that secretion of melatonin into the rumen could play a role in host-microbe interactions in ruminants.
Introduction
Intestinal microbial research has achieved great success in humans and mice. A host of studies demonstrated that gut microbes play an important role in regulating digestion, absorption and metabolism of the host, and these microbes are also able to induce or prevent inflammation of the digestive tract (1–3). Intestinal microorganisms can modulate lipid, sugar and protein metabolism in the host, hence, are closely associated with a variety of diseases such as type 2 diabetes (T2D) (4), Parkinson's disease (5), colon cancer (6, 7) and even human immunodeficiency virus (HIV) infection (8).
Gut microbes exhibit robust circadian rhythms, and their circadian patterns of relative abundance, absolute abundance and metabolomics were shown to be affected by the biological rhythm of the host (9, 10), which are largely regulated by circadian secretion of several hormones including Melatonin (MLT) (11, 12).
The intestinal tract, in particular, is capable of hormonal secretion according to a circadian rhythm, and some of these hormones are able to influence the intestinal bacteria. For instance, some of these hormones could induce locally resident microbes to release functional cytokines or chemokines (13). In turn, the microbiota is able to stimulate intestinal endocrine cells to release gut peptides and hormones, effectively resulting in interactions with the host circadian clock (14). Clearly, a growing body of literature is highlighting the existence of crosstalk between host and intestinal microbiota partly due to gut hormones.
The MLT is one of the most-essential hormones involved in regulating circadian rhythms in the host, and the complex effects of this hormone on the gut microbiome has just begun to be studied (15). For instance, Ma et al. (16) demonstrated a firm connection among MLT, gut microbiota and mucosal immune cells. Paulose et al. (17) and Paulose and Cassone (18) also reported that MLT modulated in a circadian fashion intestinal microorganisms such as Enterobacter aerogenes in humans. In addition, Zhu et al. (19) reported that MLT treatment could reduce the relative abundance of Bacteroidetes from 58.93 to 41.63% while increasing Firmicutes in the intestine of mice with colitis.
Although host-microbe communication is an integral component of normal physiological mechanisms in the human body, specific factors that coordinate this communication in ruminants remain to be fully established. Microbial density in ruminal fluid can be as high as 109 cells/mL (20), and a number of studies have associated specific microbiota profiles with phenotypes such as milk yield in dairy cows (21–23). For example, Mu et al. (24) reported that abundance of Prevotella spp. in ruminal fluid was negatively correlated with milk production, whereas abundance of Succinivibrionaceae family was positively associated with milk yield (25). Furthermore, hormones such as growth hormone (GH), prolactin (PRL) and MLT in ruminants are closely linked with lactation performance (26, 27). We hypothesized that, similar to humans, hormones and ruminal microbes in ruminants follow a circadian rhythm, and the rhythmic hormones can modulate the profiles of specific bacteria. To address this hypothesis, we investigated the diurnal variation of GH, PRL, and MLT concentrations along with ruminal microbiota profiles. The linkages between these hormones and bacteria were explored in vivo and in vitro.
Results
Diurnal Variation of MLT, GH, and PRL in Serum, Saliva, and Ruminal Fluid
As shown in Table 1, MLT was detectable in serum, saliva as well as ruminal fluid, and JTK_CYCLE analysis indicated that concentrations followed a circadian rhythm (Benjamini–Hochberg q-value, BH.Q < 0.05, Bonferroni-adjusted p-value, ADJ. P < 0.05). The trend of diurnal variation of MLT concentrations in these three biological fluids was similar, with higher concentrations at 2:00 and 22:00 compared with 10:00 and 14:00 (P < 0.001, Figure 1A). The concentrations of GH and PRL (Figures 1A,B) in serum, saliva, and ruminal fluid did not follow an obvious circadian oscillation (BH. Q > 0.05, ADJ. P > 0.05), despite the fact that the concentration of PRL in rumen fluid at 2:00 and 22:00 was significantly higher than that at 10:00 and 14:00.
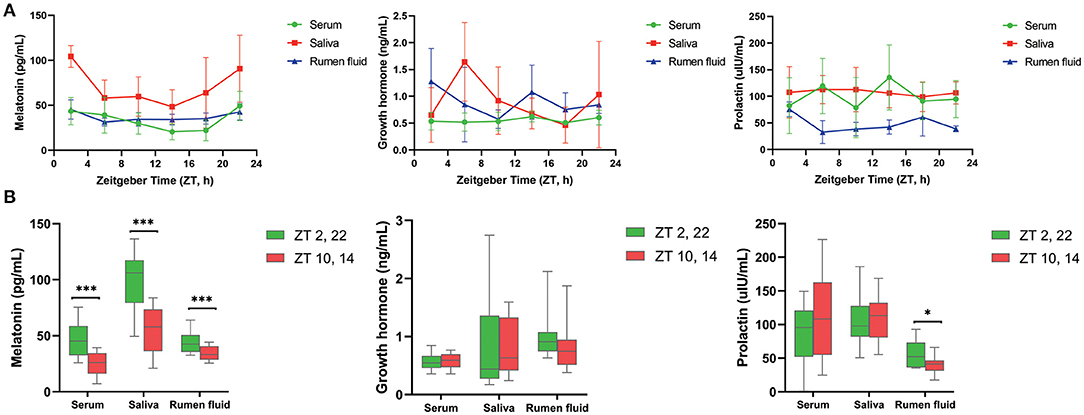
Figure 1. Diurnal change of concentrations of three hormones in different samples. (A) Line graphs show the diurnal concentration of hormones comprising melatonin (left), growth hormone (middle) and prolactin (right) in serum, saliva and rumen fluid. (B) Boxplot figures show the difference of three hormones concentration between dark (ZT2, 22) and light conditions (ZT10, 14) in serum, saliva and rumen fluid of dairy cows. Conditions are color coded (see legend). ZT, Zeitgeber time. *P < 0.05, **P < 0.01, and ***P < 0.001.
Circadian Rhythm in Ruminal Microbiota Profiles
In vivo, amplicon sequencing generated a total of 3,156,355 high-quality sequences and an average of 87,677 ± 2,506 sequences per sample, which were assigned to 2,965 OTUs. Furthermore, JTK_CYCLE analysis identified 262 OTUs exhibited a significant circadian rhythm (BH. Q < 0.05, ADJ. P < 0.05) and underscored that ~9% of ruminal microbes followed an obvious circadian rhythm (Figure 2A). At the phylum level, JTK_CYCLE analysis revealed that three predominant ruminal species, Proteobacteria, Bacteroidetes, and Firmicutes followed a circadian pattern, with relative abundance of Bacteroidetes and Firmicutes being higher in dark (Zeitgeber time, ZT 2,22) compared with light conditions (ZT 10,14; P < 0.05; Figures 2B,C). In addition, the phyla Cyanobacteria, Melainabacteria, Gracilibacteria, and Tenericutes also followed a circadian rhythm (BH. Q < 0.05, ADJ. P < 0.05). Except for Gracilibacteria, relative abundance of these microorganisms was clearly influenced by the light/dark cycle (Figures 2B,C). At the family level, JTK_CYCLE analysis revealed that Prevotellaceae, Succinivibrionaceae, Ruminococcaceae, Muribaculaceae, and Veillonellaceae followed a clear circadian oscillation (BH. Q < 0.05, ADJ. P < 0.05). Furthermore, apart from Succinibrionaceae that exhibited markedly higher abundance during light conditions (P < 0.001), the other four bacterial families substantially increased in dark conditions (P < 0.05; Figure 2D).
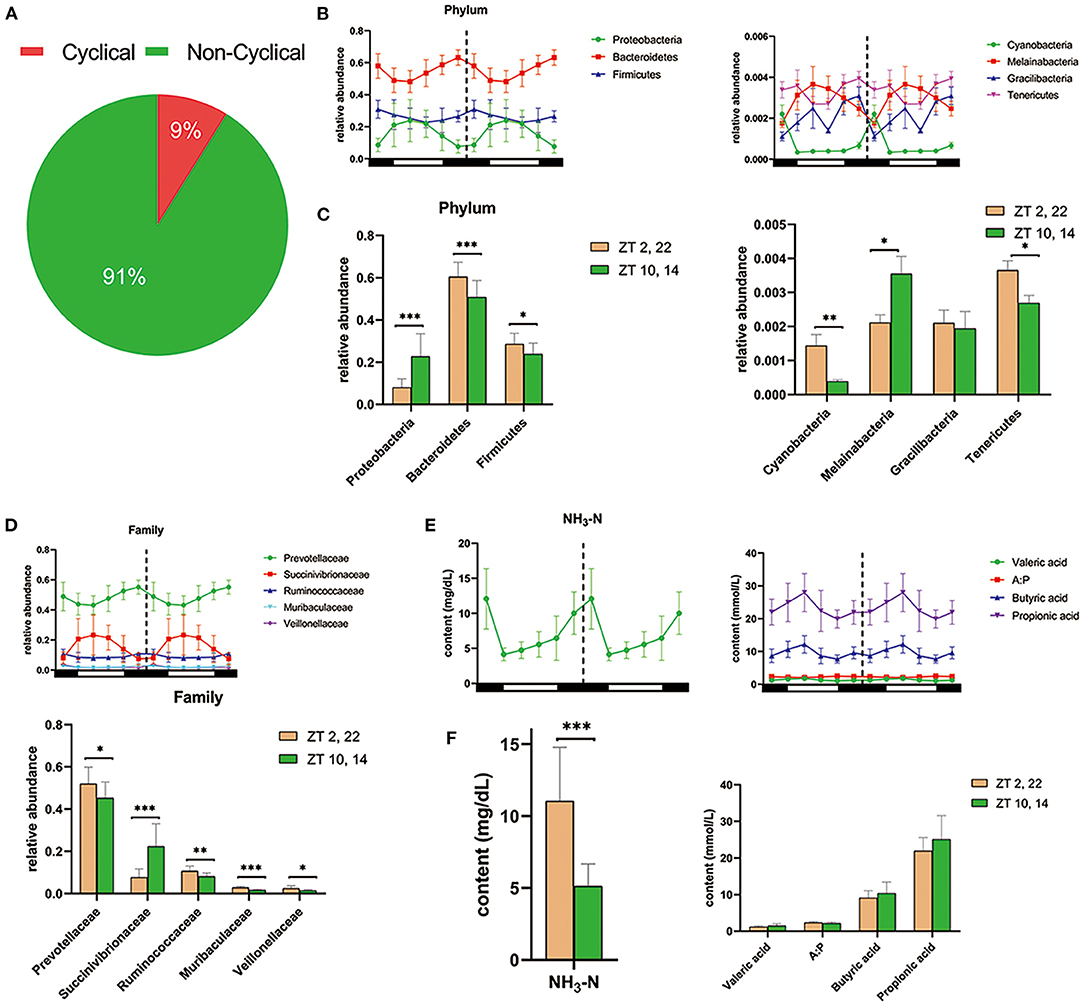
Figure 2. Diurnal rhythms of ruminal microbiota and fermentation parameters in dairy cows. (A) Pie chart shows the percentages of cyclical OTUs and non-cyclical OTUs based on the results of JTK_CYCLE analysis. (B) The double-plot line graph—where the second cycle is a duplicate of the first cycle following the dashed line—shows the diurnal change in relative abundance of the three most predominant phylum (left) and four other phyla (right) at each time point (n = 6 per time point). All of these phyla were cycling based on the JTK_CYCLE analysis (that is ADJ. P < 0.05 and BH. Q < 0.05). Black and white boxes indicate day and night, respectively. Different colored lines represent different phylum (see legend) (C) Bar graphs show the average relative abundance of the seven phyla depicted in (B) during dark (ZT2, 22) and light conditions (ZT10, 14). Conditions are color coded (see legend). *P < 0.05, **P < 0.01, and ***P < 0.001. (D) Upper double-plot line graph shows the diurnal relative abundance of five predominant family, which were cyclical according to the JTK_CYCLE analysis. Lower bar graph shows the mean relative abundance of the five predominant circadian family under the dark condition and light condition. (E) Colored line graphs present the content of dominant circadian rumen fermentation parameters in JTK_CYCLE analysis at each time point (n = 6 per time point). NH3-N, ammonia nitrogen; A:P, the ratio of acetate to propionate. (F) The average content of rhythmical rumen fermentation parameters under the dark condition (yellow) and light condition (green). *P < 0.05, **P < 0.01, and ***P < 0.001.
Circadian Rhythms in Ruminal Fermentation Parameters
As shown in Figure 2E, there was a clear circadian rhythm (BH. Q < 0.05, ADJ. P < 0.05) for ruminal ammonia nitrogen (NH3-N), valerate, butyrate, propionate as well as the ratio of acetate to propionate (A:P). Concentrations of NH3-N in ruminal fluid during light conditions were markedly lower than that during dark conditions (P < 0.001) (Figure 2F). Furthermore, the content of propionate, butyrate, and valerate was numerically higher during light conditions (P > 0.05; Figure 2F).
Linkages Between Ruminal Microbes and Melatonin in vivo
Correlations between ruminal bacterial families and ruminal melatonin or volatile fatty acids are reported in Figure 3. Families Muribaculaceae, Rikenellaceae, unidentified Cyanobacteria, Defluviitaleaceae, Veillonellaceae, Spirochaetaceae, and Protevotellaceae were positively correlated with ruminal melatonin. In contrast, unidentified Bacteria, Anaeroplasmataceae, and Rhodobacteraceae were negatively correlated with ruminal melatonin (Figure 3); genus Succinivibrio, Ruminobacter, Selenomonas, and Moryella also had a markedly negative correlation with ruminal melatonin (Supplementary Figure S1). In addition, in contrast to Rhodobacteraceae, the families Muribaculaceae, Rikenellaceae, unidentified Cyanobacteria and Protevotellaceae were negatively associated with propionate (Figure 3).
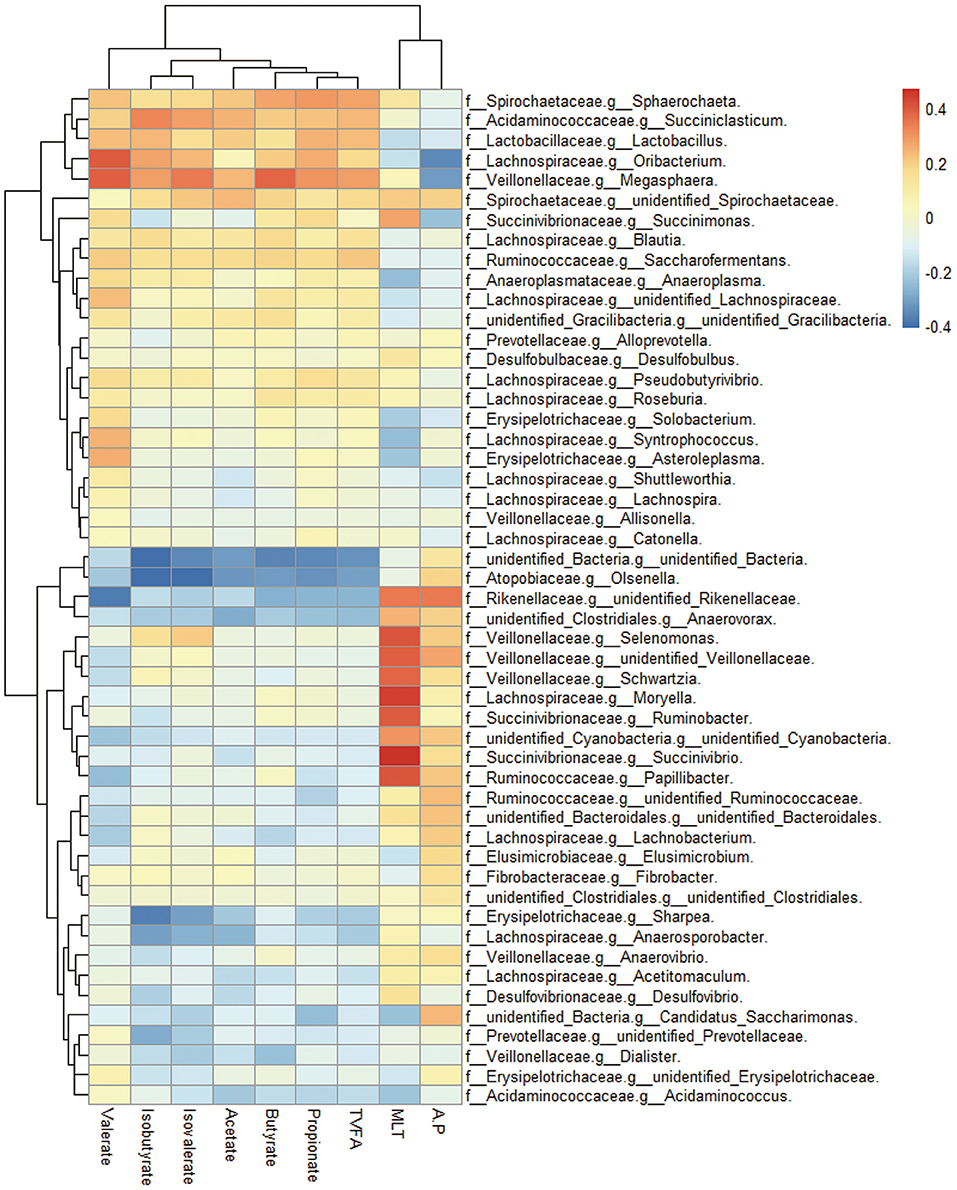
Figure 3. The ruminal microbes correlated with ruminal melatonin and fermentation parameters. Cluster heatmap figure shows the correlation between bacterial family in the rumen and several ruminal metabolites compassing melatonin and volatile fatty acids. MLT, melatonin; TVFA, total volatile fatty acids; A:P, the ratio of acetate to propionate; f, family; g, genus.
Effect of MLT on Prevotellaceae and Succinivibrionaceae in vitro
The in vitro simulated fermentation experiment was conducted to investigate the impacts of melatonin on ruminal microbiota ex vivo. A total of three different treatments were included: (i) non-rumen fluid + non-melatonin (CK0); (ii) rumen fluid + non-melatonin (CK1); (iii) rumen fluid + melatonin (MLT). The result showed that melatonin concentration was influenced by both of time and treatment in vitro and, compared with CK0, the decrease rate of melatonin in the CK1 and MLT groups was significantly greater (Table 2). As for bacteria, amplicon sequencing of the partial 16S rRNA gene generated a total of 2,532,697 high-quality sequences and an average of 60,302 ± 1,856 sequences per sample, which were assigned to 1,639 OTUs. However, the average distinct OTUs count in CK1 was 1,158 ± 42, which was significantly different from MLT (1,226 ± 52; P = 0.001).
Although the Shannon index and Beta diversity in vitro were not altered by melatonin treatment (Supplementary Figure S2), this hormone increased the relative abundance of phyla Bacteridetes and decreased Proteobacteria, Kiritimatiellaeota and Spirochaetes (P < 0.05; Figure 4A). At the family level, melatonin treatment increased the relative abundance of Prevotellaceae, F082, Muribaculaceae, and Saccharimonadaceae; however, it reduced the relative abundance of Succinivibrionaceae, Veillonellaceae, and Spirochaetaceae (P < 0.05; Figure 4B). Furthermore, at the genus level, the relative abundance of Prevotella, unidentified F082 was increased by melatonin treatment, in contrast to that of unidentified Succinivibrionaceae and Quinella (P < 0.05; Figure 4C).
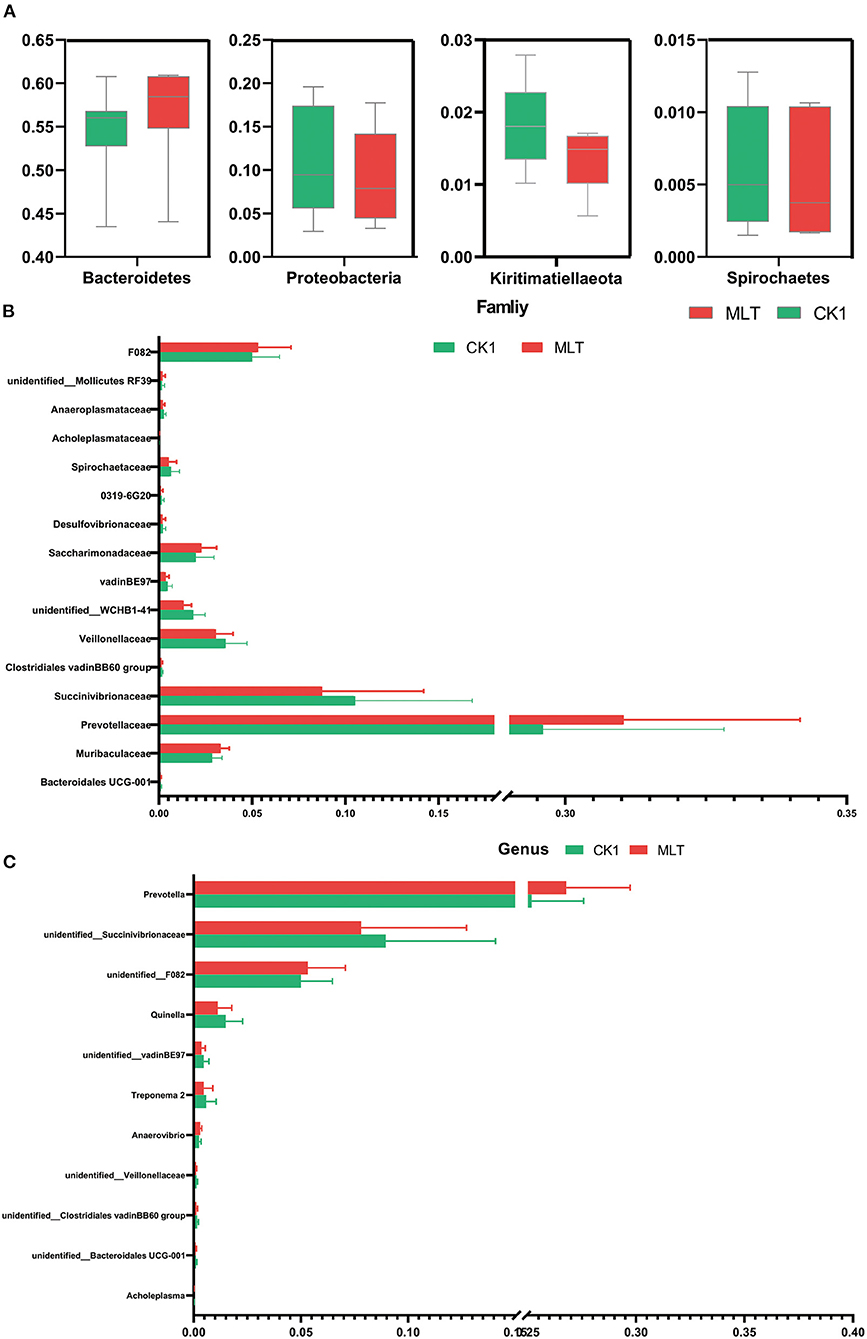
Figure 4. Melatonin impacted the ruminal microbiota in vitro fermentation. (A) Boxplot graphs show the average relative abundance of bacterial phyla that were significantly different (P < 0.05) based on paired T-tests between CK1 (green box) and MLT (red box) treatments. (B) Bar graph shows the average relative abundance of bacterial family that were significantly different (P < 0.05) based on the paired T-tests between CK1 and MLT treatments. (C) Bar graph shows the average relative abundance of bacterial genus that were significantly different (P < 0.05) based on the paired T-tests between CK1 and MLT treatments. CK0, non-rumen fluid + non-melatonin; CK1, rumen fluid + non-melatonin; MLT, rumen fluid + melatonin.
Melatonin Alters Predicted Ruminal Microbial Metabolic Pathways in vitro
A total of 12 significantly different metabolic pathways in ruminal microbiota between the CK1 and MLT treatments were predicted by CowPI. For instance, melatonin facilitated Cell motility and secretion, Atrazine degradation, Phosphotransferase system (PTS), Protein folding and associated processing, Phosphonate and phosphinate metabolism, and Glycerphospholipid metabolism, while inhibited the Chlorcyclohexane and chlorobenzene degradation, Lysine biosynthesis, Transcription machinery, Gyanoamino acid metabolism, Phenylpropanoid biosynthesis and Flavane and flavonol biosynthesis functions of ruminal bacteria in vitro (Figure 5A). Additionally, family Prevotellaceae and genus Prevotella were negatively correlated with Atrazine degradation, Cell motility and secretion, and Phosphonate and phosphinate metabolism pathways (P < 0.05), but positively correlated with Chlorocyclohexane and chlorobenzene degradation, Cyanoamino acid metabolism, Flavone and flavonol biosynthesis, Phenylpropanoid biosynthesis and Transcription machinery pathways (P < 0.01; Figure 5B and Supplementary Figure S3). The family Succinivibrionaceae and genus unidentified Succinivibrionaceae had a positive correlation with Cell motility and secretion, Glycerphospholipid metabolism, Lysine biosynthesis, Phosphotransferase system (PTS), and Protein folding and associated processing of ruminal microbiota in vitro (P < 0.05; Figure 5B and Supplementary Figure S3).
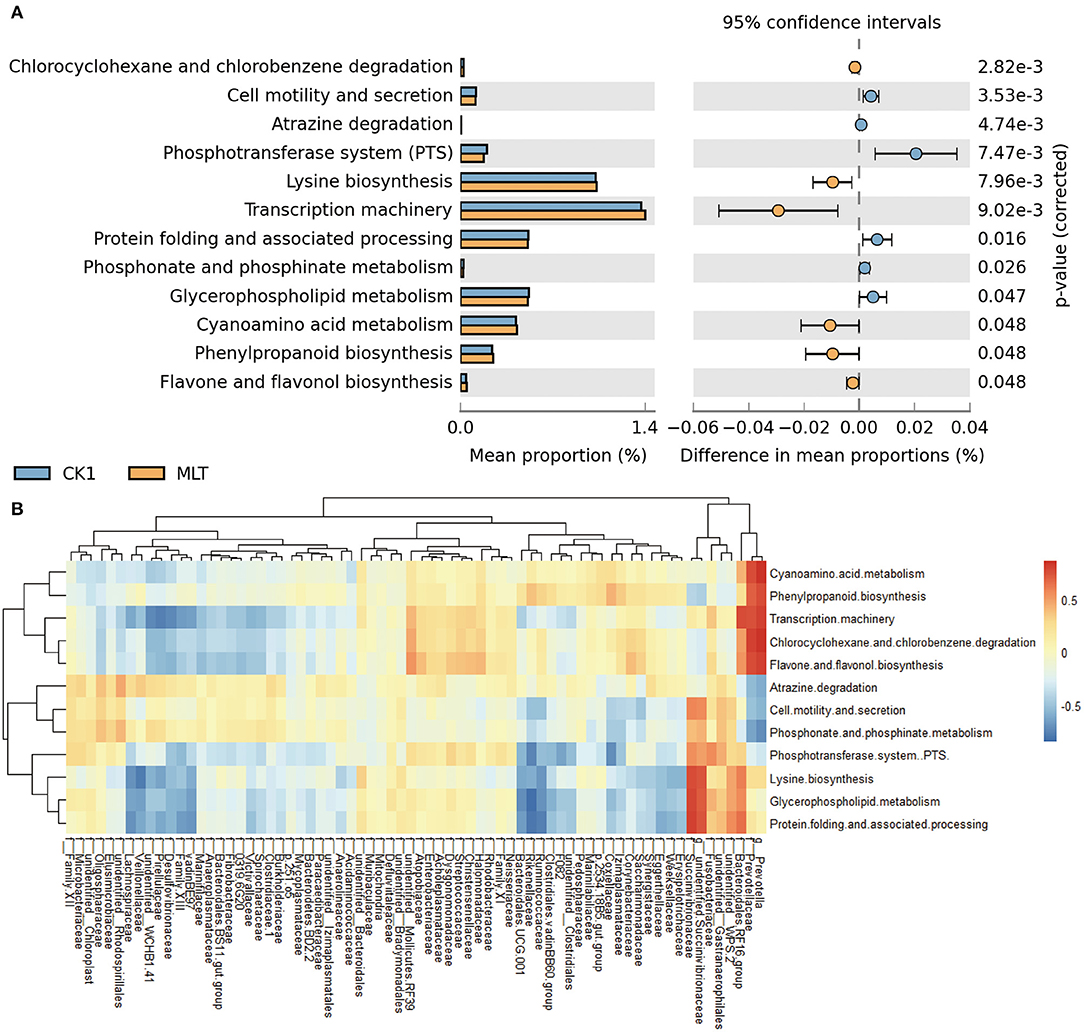
Figure 5. Melatonin mediated the metabolism pathway of ruminal microbiota. (A) Bar chart shows different metabolic pathways predicted through applying the CowPI analysis between the CK1 and MLT treatments. The blue bar and yellow bar represent CK1 and MLT, respectively. (B) Heatmap shows the correlation between ruminal bacteria family (contain two extra genus Prevotella and unidentified Succinivibrionaceae) and the predominant metabolic pathway affected by melatonin during in vitro fermentation. f for family taxa, g for genus taxa. CK0, non-rumen fluid + non-melatonin; CK1, rumen fluid + non-melatonin; MLT, rumen fluid + melatonin.
Discussion
Melatonin is an indispensable hormone regulating biological rhythms that is mainly synthesized and secreted by the pineal gland in response to environmental darkness, but limited during light conditions, which agrees with the findings that greater serum melatonin concentrations were observed at night (28). In turn, these circadian patterns regulate the rhythmicity of the biological clock in the body. At least in humans, other biological fluids such as saliva contain variable amounts of steroid hormones such as GH and PRL, and peptide hormones such as MLT partly due to diffusion from blood (29). Salivary MLT participates in the regulation of inflammatory processes, promoting antioxidant responses and cell proliferation, and contributes to the rapid healing of oral epithelial wounds (29).
The present results demonstrating that MLT in saliva of ruminants also displays circadian rhythm is similar to published work in humans and rodents. Bubenik et al. (30) were the earliest to report the presence of MLT in the ruminal muscularis and ruminal fluid of dairy cows with MLT concentrations in muscularis being greater than fluid. The origin of MLT was not evaluated. The present study not only confirmed MLT in ruminal fluid, but also that it exhibits a certain circadian rhythm, which was similar with the pattern in the serum and saliva. In addition, our detection of GH and PRL in saliva and ruminal fluid (without obvious circadian rhythms) was consistent with the findings of Koprowski et al. (31), Koyama et al. (32), and Lindell et al. (33).
Circadian oscillations of the gut microflora have been widely studied in human and mouse (34). For instance, Thaiss et al. (35) reported more than 15% of total OTUs that could be detected in mouse fecal microorganisms exhibited a circadian rhythm. This was followed by a study of Zarrinpar et al. (36), which demonstrated that 17% of total OTUs in the mouse gut microbiota exhibited a clear circadian rhythm. Unlike those previous studies, only 9% of the total OTUs in ruminal fluid demonstrated a clear and robust circadian rhythm. In fact, taking into account the fact the substantially greater number of total OTUs in rumen than intestine of human or rodents, the number of cyclical OTUs in the rumen is clearly quite considerable.
Using phyla-level assignments revealed that relative abundance of Bacteroidetes and Firmicutes oscillated diurnally in mice (36, 37). This was in accordance with the present study, as the relative abundance of Prevotellaceae, Muribaculaceae and Veillonellaceae, three dominant families of Bacteroidetes, and the Ruminococcaceae, a vital family of Firmicutes exhibited clear circadian rhythms. While the relative abundance of Proteobacteria phyla exhibited unclear rhythms in mice, the work of Liang et al. (37) inferred that the absolute abundance of Proteobacteria oscillated during the light-cycle. In contrast, the relative abundance of Proteobacteria in ruminal fluid exhibited a strong diurnal rhythm according in the present study. Succinivibrionaceae, a major family of Proteobacteria also oscillated regularly. In addition, because the phyla Bacteroidetes, Firmicutes and Proteobacteria together comprise most of the ruminal bacteria (over 97% in our study) (38), there is no doubt that nearly the entire microbiota maintains a robust circadian rhythm. Profiles of ruminal fermentation parameters, particularly the ammonia nitrogen, propionate, butyrate, and the ratio of acetate to propionate, also supported the existence of a circadian pattern.
The capacity of MLT to have a negative impact on Gram-negative bacteria is well-established (39–41). However, several recent studies have reported that MLT is not only essential to regulate the circadian rhythm of the host, but regulates the intestine by mediating metabolism of the microbiota as well as their circadian rhythms (16). Paulose and Cassone (18) demonstrated that Enterobacter aerogenes was sensitive to MLT and this hormone contributes to regulation of the circadian clock in E. aneogenes. Ma et al. (42) concluded that MLT can impact gut microbes through cytokines (e.g., IFN-γ) generated by intestinal epithelium. In addition, O'Keeffe et al. (43) demonstrated that MLT is capable to alter the metabolism of intestinal microbes in mice through NF-κB. Furthermore, Yin et al. (44) reported that administration of exogenous MLT was able to recover the disrupted diurnal rhythms of the gut microbiota in mice fed a high-fat diet.
In the present study, the families Muribaculaceae, Veillonellaceae, and Prevotellaceae, and two genera of family Succinivibrionaceae, unidentified Succinivibrionaceae spp. and Quinella spp. correlated robustly with ruminal MLT in vivo. Intriguingly, these bacterial taxa were dramatically influenced by supplemented MLT in vitro, suggesting that this hormone can mediate these cyclical microbes in the rumen. Both of the families Muribaculaceae and Prevotellaceae belong to the Bacteroidetes phylum, which demonstrated a robust circadian rhythm in response to MLT treatment in mice (44). That work also uncovered that the circadian pattern of phyla Firmicutes (contains Veillonellacea family) was impacted by MLT. Together, these findings confirm that MLT is capable of influencing circadian patterns of “rhythmic microbes” in the digestive tract including the rumen.
The fact that MLT enhanced the relative abundance of phyla Bacteroidetes and decreased Proteobacteria phyla was similar to the studies of Kim et al. (15) and Yin et al. (44) in mice. Thus, we speculate that a variety of crucial metabolic pathways within ruminal microbiota were likely to be regulated by MLT treatment. For instance, Flavonoids are a large and diverse group of secondary metabolites that are synthesized through a specific branch of the phenylpropanoid pathway (45). Flavonoids not only regulate lipid metabolism (46), but play an important role in preventing intestinal inflammation (47). Thus, the flavone biosynthesis pathway in microbiota plays a significant role on maintaining health of the host. Collectively, these results underscored the potential significance of ruminal MLT in terms of host-microbe interactions in dairy cows.
The genus Prevotella spp. and family Succinivibrionacae, localized to mucosal sites, have attracted much attention due to their association with a variety of systemic diseases including periodontitis, bacterial vaginosis, rheumatoid arthritis, metabolic disorders, and low-grade systemic inflammation (48). During gut dysbiosis, increased numbers of Prevotella were detected during persistent inflammation with HIV infections (49), metabolic syndrome (insulin-resistance) (50) as well as bowel inflammatory disease induced by NLRP6-inflammation (51). In the present study, the negative correlation between Prevotella numbers and atrazine concentrations suggested this microorganism could enhance atrazine accumulation. If, in fact, atrazine concentrations in the rumen are associated with changes in a major microbial species such as Prevotella, it could potentially lead to serious negative effects on the animal and other microbes as reported previously (52).
Beyond toxic effects, atrazine can regulate IL-1 mRNA abundance (53) and activate STAT3 signaling (54), which are well-known pro-inflammatory signals (55). Thus, we speculate that Prevotella could drive gut tissue inflammation through facilitating atrazine accumulation. Further studies appear warranted to study more closely the role of Prevotella species on physiological responses in the ruminant animal.
Recent studies provided evidence that Succinivibrionaceae species have a positive impact on milk production of lactating cows (56, 57) through their ability to utilize hydrogen and reduce production of methane (58). As such, increases in the numbers of Succinivibrionaceae should enhance availability of energy and nutrients to the host. The fact that in our study the abundance of Succinivibrionaceae was positively correlated with lysine biosynthesis suggested that this species could play a role in reducing methane. Lysine maintains the capacity to significantly inhibit both methane and carbon dioxide by shifting the equilibrium phase boundary condition to higher pressures and/or region of lower temperatures (59). Furthermore, Mu et al. (24) reported that the abundance of Prevotella spp. in ruminal fluid was negatively correlated with milk production. While the abundance of Succinivibrionaceae family was likely to positively associate with milk yield (25). Thus, the linkages between melatonin and these two species in this study might demonstrate that melatonin could affect milk production of dairy cows by regulating their ruminal microorganisms. Further research in this area is warranted.
Conclusions
In summary, the present research revealed a link between melatonin and the rhythmic oscillation of the three dominant phyla Firmicutes, Proteobacteria, and Bacteroidetes in vivo; these diurnal cyclical bacteria were closely related with melatonin in vivo and were sensitive to melatonin in vitro, indicating melatonin is likely to play a vital role in mediating ruminal microbes and their metabolic pathway.
Methods
Cow Management
All experimental procedures were approved by the Animal Management Committee (in charge of animal welfare issue) of the Institute of Animal Science, Chinese Academy of Agricultural Sciences (IAS-CAAS, Beijing, China). Six lactating Holstein cows of similar weight (566.8 ± 19.6 kg), parity (3.0 ± 0.0) and milk performance (8,398.7 ± 1,392.9 kg/y) from Youran Dairy Farm (Inner Mongolia, China; Table 3). Cows were housed in individual 10-m2 concrete-floor pens, each of which had a separate feed bunk and watering point, feed and water was available for ad libitum consumption. The CALAN broadbent feeding system (American CALAN Inc., Northwood, USA) was used for feeding. Cows were trained to use the feeding system 3 weeks before the experiment to ensure that feeding behavior was normal. Cows were adapted to natural light for 2 weeks (from 12 to 25 April), fed twice a day (07:00 and 14:00), milk yield and feed intake recorded daily (Supplementary Tables S1, S2). Diet composition is reported in Table 4 (60).
In vitro Culture
The in vitro simulated fermentation solutions were shown in Table 5. A total of three different treatments were included: (i) non-rumen fluid + non-melatonin (CK0); (ii) rumen fluid + non-melatonin (CK1); (iii) rumen fluid + melatonin (MLT). Three replicates for each treatment. The 1.2 g feed provided by Youran Dairy Farm (Inner Mongolia, China; Table 4) was weighed and used as fermentation substrates for every fermentation bottle. The standard solutions of MLT (9 ng/mL) were prepared according to Özcan and Bagci (61) using melatonin powder purchased from Sigma-Aldrich company (Sigma-Aldrich, USA). The rumen fluid was collected from four different directions of rumen of three fistula healthy Holstein cows after 2 h of morning feeding. Artificial saliva in vitro culture experiments were prepared according to Cone et al. (62). All incubation procedures were performed in a thermostatic water bath shaker (SHA-A, Hengfeng Instrument, China) at 39°C (63). After 24 h of cultivation, the fermentation bottle was taken out and placed in an ice water bath to stop the fermentation.
Sample Collection
For the in vivo study, a total of 10 mL saliva, 20 mL blood, and 100 mL ruminal fluid were collected at 02:00, 10:00, and 18:00 on the first day and 06:00, 14:00, 22:00 on the second day of the experimental period following details in Negrao et al. (64), Bu et al. (65), and Wang et al. (66), respectively. For the in vitro study, the culture fluid samples were collected at 0, 4, 8, 12, 16, 20, and 24 h of fermentation, and immediately measure the pH value of these samples. All samples were protected from light during the collection and packaging process. For sample collection at night, a dark red light (Kodak Spa, NY, USA) with light intensity <3 lx was used to account for the fact that photosensitive intensity of cattle is 3 lx (67). All samples were packed in brown tubes (Corning Inc., NY, USA) and immediately frozen prior to storage at −80°C until analyzed. Details of ruminal fermentation parameter determination are described in our previous publication (68).
Light Intensity, Humidity, and Temperature
The UT382 illuminance meter (UNI-T Inc., Dongguan, China) was used to measure light intensity of the barn at the sampling time. An RC-4 automatic temperature and humidity recorder (Elitech Inc., Jiangsu, China) was also used. All these records during experimental days are reported in Supplementary Table S3.
Determination of Hormonal Concentrations
A radioimmunoassay (RIA) was used to detect the concentration of MLT, PRL, and GH in saliva, serum, and ruminal fluid. The MLT levels were measured by means of a commercial RIA kit (RE29301, IBL, Germany) that consisted of 125I-melatonin (69). The PRL and GH levels were tested according to the RIA methods of Schams et al. (70) and Bubenik et al. (71) using a bovine PRL antibody (AS1003.1, Immundiagnostik AG, Germany) and a bovine GH antibody (AS1006.1, Immundiagnostik AG, Germany). The intra-assay coefficients of variation (CV) for MLT, PRL, and GH were 5.4, 7.4, and 8%, and the inter-assay CV for MLT, PRL, and GH were 11.1, 14.3, and 11.4%, respectively. All samples were determined by Beijing North China Biotechnology Research Institute (Beijing, China). Ruminal fluid samples were centrifuged at 4,000 × g for 30 min at 4°C before hormonal tests.
DNA Extraction and Sequencing
After thawing ruminal fluid, total DNA was extracted using the QIAamp DNA Stool Mini kit (Qiagen, Hilden, Germany) according to the manufacturer's protocols. The NanoDrop 1000 spectrophotometer (Thermo Fisher Scientific, MA, USA) was used to measure quality and quantity of DNA samples. The V3–V4 regions of 16S rRNA gene were amplified using the 341F/806R primer set (5′-CCTAYGGGRBGCASCAG-3′/5′-GGACTACNNGGGTATCTAAT-3′) according to Xue et al. (72). Subsequently, amplicons were purified by QIAquick gel extraction kit (Qiagen, Hilden, Germany). The Illumina Hiseq-PE 250 sequencing platform (SanDiego, USA) was used to conduct amplicon sequencing by Novogene Bioinformatics Technology Co., Ltd. (Beijing, China). The raw in vivo and in vitro amplicon sequence data generated in the study are available at the NCBI sequence read archive (SRA) under the accession number PRJNA666225 and PRJNA666235, respectively.
Sequencing Data Analysis
The QIIME software package was used to process raw reads (73). Operational taxonomic units (OTUs) were clustered at 97% similarity cutoff (74), and the taxonomy was analyzed with the Greengenes database. JTK_CYCLE is a nonparametric algorithm to identify rhythmic components in large group size data sets (75). This experiment refers to Thaiss et al. (35), and using JTK_CYCLE source in R program to analyse circadian rhythms of bacteria. Both Bonferroni-adjusted p-value (ADJ.P) and Benjamini–Hochberg q-values (BH.Q) <0.05 were considered significant (36). CowPI analysis was used to predict functional information (PICRUSt) within the rumen microflora (76). We used the online analysis platform developed by Aberystwyth University for CowPI analysis (https://share-galaxy.ibers.aber.ac.uk). STAMP (V.2.1.3) then was used to compare the metabolic pathway predicted by CowPI and visualized the results.
Statistical Analysis
Data analysis was carried out in Excel 2019, SAS 9.4, QIIME, GraphPad Prism software (V.8.0.2), R program (V.3.6.1), STAMP (V.2.1.3), and the Galaxy cloud platform. Hormone concentrations, relative abundance of rhythmical bacterial taxa and ruminal fermentation parameters between light/dark conditions in vivo, and the relative abundance of bacteria taxa in vitro were analyzed using paired t-tests, with P < 0.05 considered as significant. The predicted pathways were compared using the Welch's t-test performed by STAMP with a P < 0.05 being considered significant. Correlation analysis was performed using Spearman's method in R software.
Data Availability Statement
The datasets generated for this study can be found in the NCBI sequence read archive, accession numbers PRJNA666225 and PRJNA666235.
Ethics Statement
The animal study was reviewed and approved by Animal Management Committee (in charge of animal welfare issue) of the Institute of Animal Science, Chinese Academy of Agricultural Sciences (IAS-CAAS, Beijing, China).
Author Contributions
JO, MW, and DB conceived and designed the study. JO, CX, and CD conducted the experiment and analyzed the animal data. JO and FL did the metataxonomic analysis. JO drafted the manuscript. AA and JL contributed to data interpretation and writing of the manuscript. JO, MW, DB, and LM contributed to the writing of the manuscript. JL edited the final version of the manuscript. All the authors revised and edited the manuscript.
Funding
This work was supported by the Open subject of State Key Laboratory of Animal Nutrition (Grant 2004DA125184F1715), Key program of State Key Laboratory of Sheep Genetic Improvement and Healthy Production (2021ZD07 and 2021ZD01), Science and Technology Innovation Project of Chinese Academy of Agricultural Sciences (Grants ASTIP-IAS07 and CAAS-XTCX2016011-01), and Jiangsu Province Graduate Research and Innovation Project (Grant XKYCX19_121).
Conflict of Interest
The authors declare that the research was conducted in the absence of any commercial or financial relationships that could be construed as a potential conflict of interest.
Publisher's Note
All claims expressed in this article are solely those of the authors and do not necessarily represent those of their affiliated organizations, or those of the publisher, the editors and the reviewers. Any product that may be evaluated in this article, or claim that may be made by its manufacturer, is not guaranteed or endorsed by the publisher.
Supplementary Material
The Supplementary Material for this article can be found online at: https://www.frontiersin.org/articles/10.3389/fnut.2021.760578/full#supplementary-material
Abbreviations
MLT, Melatonin; GH, Growth hormone; PRL, Prolactin; BH.Q, Benjamini-Hochberg q-value; ADJ.P, Bonferroni-adjusted p-value; OTUs, Operational taxonomic units; NH3-N, Ammonia nitrogen; A:P, Ratio of acetate to propionate; SRA, Sequence read archive; RIA, Radioimmunoassay.
References
1. Chen XY, Eslamfam S, Fang LY, Qiao SY, Ma X. Maintenance of gastrointestinal glucose homeostasis by the gut-brain axis. Curr Protein Pept Sci. (2017) 18:541–7. doi: 10.2174/1389203717666160627083604
2. Coleman OI, Haller D. Bacterial signaling at the intestinal epithelial interface in inflammation and cancer. Front Immunol. (2017) 8:1927. doi: 10.3389/fimmu.2017.01927
3. Wong S-Y, Ramanan D, Cadwell K. Host-microbe and intra-microbiome interactions leading to small intestinal inflammation in a genetically-susceptible host. Gastroenterology. (2018) 154:S1357. doi: 10.1016/S0016-5085(18)34438-X
4. Sabatino A, Regolisti G, Cosola C, Gesualdo L, Fiaccadori E. Intestinal microbiota in Type 2 diabetes and chronic kidney disease. Curr Diab Rep. (2017) 17:16. doi: 10.1007/s11892-017-0841-z
5. Sampson T. The impact of indigenous microbes on Parkinson's disease. Neurobiol Dis. (2020) 135:104426. doi: 10.1016/j.nbd.2019.03.014
6. Kim M, Friesen L, Park J, Kim HM, Kim CH. Microbial metabolites, short-chain fatty acids, restrain tissue bacterial load, chronic inflammation, and associated cancer in the colon of mice. Eur J Immunol. (2018) 48:1235–47. doi: 10.1002/eji.201747122
7. Song MY, Chan AT, Sun J. Influence of the gut microbiome, diet, and environment on risk of colorectal cancer. Gastroenterology. (2020) 158:322–40. doi: 10.1053/j.gastro.2019.06.048
8. Vujkovic-Cvijin I, Somsouk M. HIV and the gut microbiota: composition, consequences, and avenues for amelioration. Curr HIV/AIDS Rep. (2019) 16:204–13. doi: 10.1007/s11904-019-00441-w
9. Leone V, Gibbons SM, Martinez K, Hutchison AL, Huang EY, Cham CM, et al. Effects of diurnal variation of gut microbes and high-fat feeding on host circadian clock function and metabolism. Cell Host and Microbe. (2015) 17:681–9. doi: 10.1016/j.chom.2015.03.006
10. Voigt RM, Forsyth CB, Green SJ, Engen PA, Keshavarzian A. Chapter 9: circadian rhythm and the gut microbiome. In: Cryan JF, Clarke G, editors. International Review of Neurobiology. London: Academic Press (2016). p. 193–205.
11. Tsang AH, Astiz M, Friedrichs M, Oster H. Endocrine regulation of circadian physiology. J Endocrinol. (2016) 230:R1–R11. doi: 10.1530/JOE-16-0051
12. Torres-Ruiz J, Sulli A, Cutolo M, Shoenfeld Y. Air travel, circadian rhythms/hormones, and autoimmunity. Clin Rev Allergy Immunol. (2017) 53:117–25. doi: 10.1007/s12016-017-8599-2
13. Fülling C, Dinan TG, Cryan JF. Gut microbe to brain signaling: what happens in vagus. Neuron. (2019) 101:998–1002. doi: 10.1016/j.neuron.2019.02.008
14. Martin AM, Sun EW, Rogers GB, Keating DJ. The influence of the gut microbiome on host metabolism through the regulation of gut hormone release. Front Physiol. (2019) 10:428. doi: 10.3389/fphys.2019.00428
15. Kim SW, Kim S, Son M, Cheon JH, Park YS. Melatonin controls microbiota in colitis by goblet cell differentiation and antimicrobial peptide production through toll-like receptor 4 signalling. Sci Rep. (2020) 10:2232. doi: 10.1038/s41598-020-59314-7
16. Ma N, Zhang J, Reiter RJ, Ma X. Melatonin mediates mucosal immune cells, microbial metabolism, and rhythm crosstalk: a therapeutic target to reduce intestinal inflammation. Med Res Rev. (2020) 40:606–32. doi: 10.1002/med.21628
17. Paulose JK, Wright JM, Patel AG, Cassone VM. Human gut bacteria are sensitive to melatonin and express endogenous circadian rhythmicity. PLoS One. (2016) 11:e0146643. doi: 10.1371/journal.pone.0146643
18. Paulose JK, Cassone VM. The melatonin-sensitive circadian clock of the enteric bacterium Enterobacter aerogenes. Gut Microbes. (2016) 7:424–7. doi: 10.1080/19490976.2016.1208892
19. Zhu D, Ma Y, Ding S, Jiang H, Fang J. Effects of melatonin on intestinal microbiota and oxidative stress in colitis mice. BioMed Res Int. (2018) 2018:2607679. doi: 10.1155/2018/2607679
20. Li R, Connor E, Li C, Baldwin Vi R, Sparks M. Characterization of the rumen microbiota of pre-ruminant calves using metagenomic tools. Environ Microbiol. (2012) 14:129–39. doi: 10.1111/j.1462-2920.2011.02543.x
21. Weimer P, Waghorn G, Odt C, Mertens D. Effect of diet on populations of three species of ruminal cellulolytic bacteria in lactating dairy cows. J Dairy Sci. (1999) 82:122–34. doi: 10.3168/jds.S0022-0302(99)75216-1
22. Jami E, White BA, Mizrahi I. Potential role of the bovine rumen microbiome in modulating milk composition and feed efficiency. PLoS One. (2014) 9:e85423. doi: 10.1371/journal.pone.0085423
23. Bickhart DM, Weimer PJ. Symposium review: host-rumen microbe interactions may be leveraged to improve the productivity of dairy cows. J Dairy Sci. (2018) 101:7680–9. doi: 10.3168/jds.2017-13328
24. Mu Y, Lin X, Wang Z, Hou Q, Wang Y, Hu Z. High-production dairy cattle exhibit different rumen and fecal bacterial community and rumen metabolite profile than low-production cattle. Microbiologyopen. (2018) 8:e00673. doi: 10.1002/mbo3.673
25. Lima FS, Oikonomou G, Lima SF, Bicalho ML, Ganda EK, de Oliveira Filho JC, et al. Prepartum and postpartum rumen fluid microbiomes: characterization and correlation with production traits in dairy cows. Appl Environ Microbiol. (2015) 81:1327–37. doi: 10.1128/AEM.03138-14
26. Auldist MJ, Turner S-A, McMahon CD, Prosser CG. Effects of melatonin on the yield and composition of milk from grazing dairy cows in New Zealand. J Dairy Res. (2007) 74:52–7. doi: 10.1017/S0022029906002160
27. van Hoeij RJ, Dijkstra J, Bruckmaier RM, Gross JJ, Lam TJGM, Remmelink GJ, et al. Consequences of dietary energy source and energy level on energy balance, lactogenic hormones, and lactation curve characteristics of cows after a short or omitted dry period. J Dairy Sci. (2017) 100:8544–64. doi: 10.3168/jds.2017-12855
28. Armstrong SM. Melatonin and circadian control in mammals. Experientia. (1989) 45:932–8. doi: 10.1007/BF01953050
29. Gröschl M. The physiological role of hormones in saliva. Bioessays. (2009) 31:843–52. doi: 10.1002/bies.200900013
30. Bubenik GA, Hacker RR, Brown GM, Bartos L. Melatonin concentrations in the luminal fluid, mucosa, and muscularis of the bovine and porcine gastrointestinal tract. J Pineal Res. (1999) 26:56–63. doi: 10.1111/j.1600-079X.1999.tb00567.x
31. Koprowski J, Tucker H, Convey E. Prolactin and growth hormone circadian periodicity in lactating cows. Proc Soc Exp Biol Med. (1972) 140:1012–4. doi: 10.3181/00379727-140-36601
32. Koyama T, Omata Y, Saito A. Changes in salivary cortisol concentrations during a 24-hour period in dogs. Horm Metab Res. (2003) 35:355–7. doi: 10.1055/s-2003-41356
33. Lindell SG, Suomi SJ, Shoaf S, Higley JD, Linnoila M. Salivary prolactin as a marker for central serotonin turnover. Biol Psychiatry. (1999) 46:568–72. doi: 10.1016/S0006-3223(99)00037-2
34. Liang X, FitzGerald GA. Timing the microbes: the circadian rhythm of the gut microbiome. J Biol Rhythms. (2017) 32:505–15. doi: 10.1177/0748730417729066
35. Thaiss CA, Zeevi D, Levy M, Zilberman-Schapira G, Suez J, Tengeler AC, et al. Transkingdom control of microbiota diurnal oscillations promotes metabolic homeostasis. Cell. (2014) 159:514–29. doi: 10.1016/j.cell.2014.09.048
36. Zarrinpar A, Chaix A, Yooseph S, Panda S. Diet and feeding pattern affect the diurnal dynamics of the gut microbiome. Cell Metab. (2014) 20:1006–17. doi: 10.1016/j.cmet.2014.11.008
37. Liang X, Bushman FD, FitzGerald GA. Rhythmicity of the intestinal microbiota is regulated by gender and the host circadian clock. Proc Natl Acad Sci U S A. (2015) 112:10479–84. doi: 10.1073/pnas.1501305112
38. Bi Y, Zeng S, Zhang R, Diao Q, Tu Y. Effects of dietary energy levels on rumen bacterial community composition in Holstein heifers under the same forage to concentrate ratio condition. BMC Microbiol. (2018) 18:69. doi: 10.1186/s12866-018-1213-9
39. Rahman MA, Azuma Y, Fukunaga H, Murakami T, Sugi K, Fukushi H, et al. Serotonin and melatonin, neurohormones for homeostasis, as novel inhibitors of infections by the intracellular parasite chlamydia. J Antimicrob Chemother. (2005) 56:861–8. doi: 10.1093/jac/dki331
40. Tekbas OF, Ogur R, Korkmaz A, Kilic A, Reiter RJ. Melatonin as an antibiotic: new insights into the actions of this ubiquitous molecule. J Pineal Res. (2008) 44:222–6. doi: 10.1111/j.1600-079X.2007.00516.x
41. Srinivasan V, Mohamed M, Kato H. Melatonin in bacterial and viral infections with focus on sepsis: a review. Recent Pat Endocr Metab Immune Drug Discov. (2012) 6:30–9. doi: 10.2174/187221412799015317
42. Ma N, Guo P, Zhang J, He T, Kim SW, Zhang G, et al. Nutrients mediate intestinal bacteria-mucosal immune crosstalk. Front Immunol. (2018) 9:5. doi: 10.3389/fimmu.2018.00005
43. O'Keeffe S, Beynon A, Davies J, Moynagh PN, Coogan A. NF-κB signalling is involved in immune-modulation, but not basal functioning, of the mouse suprachiasmatic circadian clock. Eur J Neurosci. (2017) 45:1111–23. doi: 10.1111/ejn.13553
44. Yin J, Li Y, Han H, Ma J, Liu G, Wu X, et al. Administration of exogenous melatonin improves the diurnal rhythms of the gut microbiota in mice fed a high-fat diet. mSystem. (2020) 5:e00002–20. doi: 10.1128/mSystems.00002-20
45. Li X, Zhang L, Ahammed GJ, Li ZX, Wei JP, Shen C, et al. Stimulation in primary and secondary metabolism by elevated carbon dioxide alters green tea quality in Camellia sinensis L. Sci Rep. (2017) 7:7937. doi: 10.1038/s41598-017-08465-1
46. Su DX, Liu HS, Qi XY, Dong LH, Zhang RF, Zhang J. Citrus peel flavonoids improve lipid metabolism by inhibiting miR-33 and miR-122 expression in HepG2 cells. Biosci Biotechnol Biochem. (2019) 83:1747–55. doi: 10.1080/09168451.2019.1608807
47. Gil-Cardoso K, Gines I, Pinent M, Ardevol A, Blay M, Terra X. Effects of flavonoids on intestinal inflammation, barrier integrity and changes in gut microbiota during diet-induced obesity. Nutr Res Rev. (2016) 29:234–48. doi: 10.1017/S0954422416000159
48. Larsen JM. The immune response to Prevotella bacteria in chronic inflammatory disease. Immunology. (2017) 151:363–74. doi: 10.1111/imm.12760
49. Dillon SM, Lee EJ, Kotter CV, Austin GL, Gianella S, Siewe B, et al. Gut dendritic cell activation links an altered colonic microbiome to mucosal and systemic T-cell activation in untreated HIV-1 infection. Mucosal Immunol. (2016) 9:24–37. doi: 10.1038/mi.2015.33
50. Li J, Zhao FQ, Wang YD, Chen JR, Tao JE, Tian G, et al. Gut microbiota dysbiosis contributes to the development of hypertension. Microbiome. (2017) 5:14. doi: 10.1186/s40168-016-0222-x
51. Elinav E, Strowig T, Kau AL, Henao-Mejia J, Thaiss CA, Booth CJ, et al. NLRP6 inflammasome regulates colonic microbial ecology and risk for colitis. Cell. (2011) 145:745–57. doi: 10.1016/j.cell.2011.04.022
52. Singh S, Kumar V, Chauhan A, Datta S, Wani AB, Singh N, et al. Toxicity, degradation and analysis of the herbicide atrazine. Environ Chem Lett. (2018) 16:211–37. doi: 10.1007/s10311-017-0665-8
53. Wang X, Xing HJ, Li XL, Xu SW, Wang XL. Effects of atrazine and chlorpyrifos on the mRNA levels of IL-1 and IFN-γ 2b in immune organs of common carp. Fish Shellfish Immunol. (2011) 31:126–33. doi: 10.1016/j.fsi.2011.04.015
54. Hu KB, Tian Y, Du YW, Huang LD, Chen JY, Li N, et al. Atrazine promotes RM1 prostate cancer cell proliferation by activating STAT3 signaling. Int J Oncol. (2016) 48:2166–74. doi: 10.3892/ijo.2016.3433
55. Hu YS, Han X, Liu XH. STAT3: a potential drug target for tumor and inflammation. Curr Top Med Chem. (2019) 19:1305–17. doi: 10.2174/1568026619666190620145052
56. Indugu N, Vecchiarelli B, Baker LD, Ferguson JD, Vanamala JK, Pitta DW. Comparison of rumen bacterial communities in dairy herds of different production. BMC Microbiol. (2017) 17:190. doi: 10.1186/s12866-017-1098-z
57. Ma ZZ, Cheng YY, Wang SQ, Ge JZ, Shi HP, Kou JC. Positive effects of dietary supplementation of three probiotics on milk yield, milk composition and intestinal flora in Sannan dairy goats varied in kind of probiotics. J Anim Physiol Anim Nutr. (2020) 104:44–55. doi: 10.1111/jpn.13226
58. Wallace RJ, Rooke JA, McKain N, Duthie C-A, Hyslop JJ, Ross DW, et al. The rumen microbial metagenome associated with high methane production in cattle. BMC Genomics. (2015) 16:839. doi: 10.1186/s12864-015-2032-0
59. Mannar N, Bavoh CB, Baharudin AH, Lal B, Mellon NB. Thermophysical properties of aqueous lysine and its inhibition influence on methane and carbon dioxide hydrate phase boundary condition. Fluid Phase Equilibria. (2017) 454:57–63. doi: 10.1016/j.fluid.2017.09.012
60. Council NR. Nutrient Requirements of Dairy Cattle. Washington, DC: National Academies Press (2001).
61. Özcan N, Bagci S. Determination of melatonin in cow's milk by liquid chromatography tandem mass spectrometry (LC-MS/MS). Food Anal Methods. (2018) 11:703–8. doi: 10.1007/s12161-017-1037-5
62. Cone JW, van Gelder AH, Visscher GJW, Oudshoorn L. Influence of rumen fluid and substrate concentration on fermentation kinetics measured with a fully automated time related gas production apparatus. Anim Feed Sci Technol. (1996) 61:113–28. doi: 10.1016/0377-8401(96)00950-9
63. Zhang Z, Wang S, Wang M, Shahzad K, Zhang X, Qi R, et al. Effects of urtica cannabina to leymus chinensis ratios on ruminal microorganisms and fiber degradation in vitro. Animals. (2020) 10:335. doi: 10.3390/ani10020335
64. Negrao J, Porcionato M, De Passille A, Rushen J. Cortisol in saliva and plasma of cattle after ACTH administration and milking. J Dairy Sci. (2004) 87:1713–8. doi: 10.3168/jds.S0022-0302(04)73324-X
65. Bu D, Wang J, Dhiman T, Liu S. Effectiveness of oils rich in linoleic and linolenic acids to enhance conjugated linoleic acid in milk from dairy cows. J Dairy Sci. (2007) 90:998–1007. doi: 10.3168/jds.S0022-0302(07)71585-0
66. Wang H, Chen Q, Chen L, Ge R, Wang M, Yu L, et al. Effects of dietary physically effective neutral detergent fiber content on the feeding behavior, digestibility, and growth of 8-to 10-month-old Holstein replacement heifers. J Dairy Sci. (2017) 100:1161–9. doi: 10.3168/jds.2016-10924
67. Carcangiu V, Giannetto C, Luridiana S, Piccione G. Features of the daily rhythms of blood melatonin and glucose in goats during different natural photoperiod. Chronobiol Int. (2018) 35:329–35. doi: 10.1080/07420528.2017.1405968
68. Ouyang J, Wang M, Hou Q, Feng D, Pi Y, Zhao W. Effects of dietary mulberry leaf powder in concentrate on the rumen fermentation and ruminal epithelium in fattening Hu sheep. Animals. (2019) 9:218. doi: 10.3390/ani9050218
69. Ramos A, Laguna I, de Lucía M-LM, Martín-Palomino P, Regodón S, Míguez M-P. Evolution of oxidative/nitrosative stress biomarkers during an open-field vaccination procedure in sheep: effect of melatonin. Vet Immunol Immunopathol. (2010) 133:16–24. doi: 10.1016/j.vetimm.2009.06.016
70. Schams D, Rüsse I, Schallenberger E, Prokopp S, Chan JS. The role of steroid hormones, prolactin and placental lactogen on mammary gland development in ewes and heifers. J Endocrinol. (1984) 102:121–30. doi: 10.1677/joe.0.1020121
71. Bubenik GA, Schams D, White RG, Rowell J, Blake J, Bartos L. Seasonal levels of metabolic hormones and substrates in male and female reindeer (Rangifer tarandus). Comp Biochem Physiol C Pharmacol Toxicol Endocrinol. (1998) 120:307–15. doi: 10.1016/S0742-8413(98)10010-5
72. Xue MY, Sun HZ, Wu XH, Guan LL, Liu JX. Assessment of rumen bacteria in dairy cows with varied milk protein yield. J Dairy Sci. (2019) 102:5031–41. doi: 10.3168/jds.2018-15974
73. Caporaso JG, Kuczynski J, Stombaugh J, Bittinger K, Bushman FD, Costello EK, et al. QIIME allows analysis of high-throughput community sequencing data. Nat Methods. (2010) 7:335–6. doi: 10.1038/nmeth.f.303
74. Edgar RC. UPARSE: highly accurate OTU sequences from microbial amplicon reads. Nat Methods. (2013) 10:996–8. doi: 10.1038/nmeth.2604
75. Hughes ME, Hogenesch JB, Kornacker K. JTK_CYCLE: an Efficient nonparametric algorithm for detecting rhythmic components in genome-scale data sets. J Biol Rhythms. (2010) 25:372–80. doi: 10.1177/0748730410379711
Keywords: lactating cows, rumen, melatonin, flora structure, circadian rhythm
Citation: Ouyang J, Wang M, Bu D, Ma L, Liu F, Xue C, Du C, Aboragah A and Loor JJ (2021) Ruminal Microbes Exhibit a Robust Circadian Rhythm and Are Sensitive to Melatonin. Front. Nutr. 8:760578. doi: 10.3389/fnut.2021.760578
Received: 18 August 2021; Accepted: 28 September 2021;
Published: 25 October 2021.
Edited by:
Yangchun Cao, Northwest A&F University, ChinaReviewed by:
Yanfen Cheng, Nanjing Agricultural University, ChinaIsabelle Wolowczuk, INSERM U1019 Center d'Infection et Immunité de Lille (CIIL), France
Copyright © 2021 Ouyang, Wang, Bu, Ma, Liu, Xue, Du, Aboragah and Loor. This is an open-access article distributed under the terms of the Creative Commons Attribution License (CC BY). The use, distribution or reproduction in other forums is permitted, provided the original author(s) and the copyright owner(s) are credited and that the original publication in this journal is cited, in accordance with accepted academic practice. No use, distribution or reproduction is permitted which does not comply with these terms.
*Correspondence: Mengzhi Wang, mengzhiwangyz@126.com; Dengpan Bu, budengpan@caas.cn