- 1Department of Food Science and Human Nutrition, Iowa State University, Ames, IA, United States
- 2Interdepartmental Graduate Program in Nutritional Sciences, Iowa State University, Ames, IA, United States
- 3Department of Biomedical Sciences, Iowa State University College of Veterinary Medicine, Ames, IA, United States
- 4Department of Kinesiology, Iowa State University, Ames, IA, United States
Eggs are protein-rich, nutrient-dense, and contain bioactive ingredients that have been shown to modify gene expression and impact health. To understand the effects of egg consumption on tissue-specific mRNA and microRNA expression, we examined the role of whole egg consumption (20% protein, w/w) on differentially expressed genes (DEGs) between rat (n = 12) transcriptomes in the prefrontal cortex (PFC), liver, kidney, and visceral adipose tissue (VAT). Principal component analysis with hierarchical clustering was used to examine transcriptome profiles between dietary treatment groups. We performed Gene Ontology and Kyoto Encyclopedia of Genes and Genomes (KEGG) pathway analysis as well as genetic network and disease enrichment analysis to examine which metabolic pathways were the most predominantly altered in each tissue. Overall, our data demonstrates that whole egg consumption for 2 weeks modified the expression of 52 genes in the PFC, 22 genes in VAT, and two genes in the liver (adj p < 0.05). Additionally, 16 miRNAs were found to be differentially regulated in the PFC, VAT, and liver, but none survived multiple testing correction. The main pathways influenced by WE consumption were glutathione metabolism in VAT and cholesterol biosynthesis in the PFC. These data highlight key pathways that may be involved in diseases and are impacted by acute consumption of a diet containing whole eggs.
Introduction
Eggs are a low-cost, nutrient-dense food comprised of numerous vitamins and bioactive compounds, and have been proposed to play a role in disease prevention (1, 2). Dietary whole eggs and their derived compounds (3) have been linked to several mechanisms of modulating gene expression, such as vitamin D-mediated transcriptional regulation and methyl group metabolism, by supplying choline, methionine, folate, B12, B6, and B2 (4). Despite the beneficial components of eggs, they remain one of the most controversial foods (5), due to their cholesterol content (6, 7). Observational studies examining the role of long-term egg intake on the risk of developing cardiovascular disease (CVD) have reported inconsistent results (8), but most recently, Dehghan and others reported no significant association between whole egg intake and major CVD events in a conglomerate of 50 studies (9). Although the role of whole egg (WE) consumption has been extensively examined in population-based studies (10, 11), relatively few studies have addressed the role of whole egg consumption in health and disease experimentally (12, 13).
Some studies have addressed the mechanistic role of individual egg components. For instance, egg yolk peptides have been shown to display anti-oxidative properties (14) and lutein, a carotenoid that is rich in egg yolk, has been demonstrated to protect dopaminergic neurons from oxidative damage in a chemically-induced mouse model of Parkinson's Disease (15). Similar effects of lutein administration have also been shown in other animal models of aging and cognitive impairment (16, 17); however, the role of these egg components and their influence on global gene expression remains not well-understood. In addition to WE decreasing oxidative stress, our laboratory has previously reported that consuming a WE-based diet reduced body weight gain in rats with type 2 diabetes (12, 13). To date, very few studies have focused on identifying the molecular mechanisms that are influenced by WE consumption in addition to the global gene expression changes in rats fed WE vs. casein (CAS)-based diets. By gaining an in-depth understanding of gene-diet interactions, we can understand how genes regulating molecular pathways are modified by short-term dietary WE consumption, allowing for future research to identify more mechanistic approaches examining the relationship between dietary WE and chronic disease.
In this study, male Sprague Dawley rats were fed WE-based diets to examine the influence of short-term WE consumption on mRNA and microRNA expression in comparison with CAS-based diets. We examined the transcriptome profiles of the prefrontal cortex (PFC), visceral adipose (VAT), liver, and kidney tissues to identify metabolic pathways that may be altered by WE consumption, and mapped these changes to microRNAs. The primary aim of this study was to initially determine if short-term WE consumption had a significant impact on gene expression in specific tissues of interest given the relevance of these tissues in disease. Furthermore, we sought to examine if changes in expression of miRNAs could be upstream of observed gene expression changes.
Materials and Methods
The data discussed and presented in this publication have been made publicly available and deposited in NCBI's Gene Expression Omnibus (18) and can be found through GEO Series accession number GSE163193 (https://www.ncbi.nlm.nih.gov/geo/query/acc.cgi?acc=GSE163193).
Animals and Diets
This animal study was approved by the Institutional Animal Care and Use Committee (IACUC) at Iowa State University and was performed according to the Iowa State University Laboratory Animal Resources Guidelines. Male Sprague Dawley rats (n = 12) were obtained at 6 week of age (151–175 g) from Charles River Laboratories (Wilmington, MA). Current studies were limited to males, building on previous studies that provide the foundation for this study. Rats were individually housed in conventional cages in a temperature-controlled environment (22 ± 2°C) using a 12-h light-dark cycle. All rats were acclimated for 1 week on a standard rat chow diet, after which they were randomly assigned to one of two dietary intervention groups. There were no significant differences in baseline body weight between the two dietary groups (p = 0.62). Rats were placed on either a control CAS-or WE-based diet (Research Diets, New Brunswick, NJ; Table 1) matched for 20% protein (w/w). For 72 h, animals underwent a controlled fasted-refeeding protocol to train them to consume food ad libitum within a 4 h time period (Figure 1) for a serum collection. After training, 7-week old animals were fasted overnight for 10 h with water provided ad libitum followed by controlled feeding (4 g) of either the CAS- or WE-based diet. Serum was collected via the tail vein at 0, 2, 4, 6, and 8 h immediately following refeeding. Following the serum time curve collection, rats were given respective dietary treatments, ad libitum for 2 weeks. Food intake and body weight gain were monitored daily. Following the dietary intervention, 9-week old rats underwent a 12 h overnight fast with water provided ad libitum; rats were anesthetized with a ketamine:xylazine cocktail (90:10 mg/kg bw) via a single intraperitoneal injection. Following anesthesia, whole blood was collected via cardiac puncture for serum sample, followed by procurement of vital organs. Euthanasia was confirmed by performing a bilateral thoracotomy. The epididymal VAT, kidney and liver, were procured, weighed, and stored in RNAlater (Thermo Fisher, Waltham, MA). The fresh PFC was excised and rapidly micro dissected on a chilled platform. A flat edge razor was used to remove and discard the olfactory bulbs and make coronal slices through the brain. Anterior forceps of the corpus collosum (AFCC) was used as a visual landmark to identify the section from which to sub-dissect the PFC. Specifically, the darker tissue between the AFCC and the genus corpus callosum in roughly a diamond shape was dissected as the PFC. After sub-dissection the tissue was weighed and stored in RNAlater (Thermo Fisher, Waltham, MA).
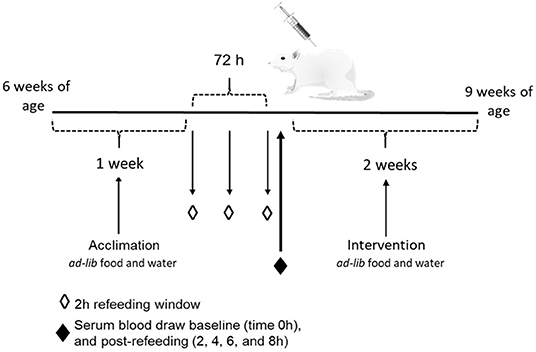
Figure 1. Schematic of the study design to measure the effects of whole egg consumption in a serum response curve and after a 2 week dietary intervention.
Large and Small RNA Extraction and Sequencing
Large- and smallRNAs were extracted using a SPLIT Total RNA Extraction Kit (Lexogen, Greenland, NH). RNA was quantified using a Qubit Fluorometer (ThermoFisher Scientific), and integrity was assessed using a Bioanalyzer 2100 (Agilent Technologies). Large RNA libraries were prepared using an automated protocol for the QuantSeq 3′ mRNA-Seq Library Prep Kit (Lexogen, Greenland, NH) and small RNA libraries were prepared using Small RNA Library Prep Kits (Lexogen, Greenland, NH). Total RNA samples were multiplexed across two lanes using an Illumina High-Seq 3000 resulting in an average of 7.5 million reads per sample prior to quality control. Small RNA libraries were also multiplexed and run on a separate lane on an Illumina High-Seq 3000 (San Diego, CA).
Quality Control and Adapter Trimming
All large and small RNA reads were inspected using Fastqc and were trimmed to remove adapter sequences using BBDUK (BBMap: A Fast, Accurate, Splice-Aware Aligner)1. Read segments matching common Illumina Truseq or Nextera adapter sequences were removed for the reverse-complement or forward sequence of the adapters during processing.
Read Alignment and Quantification
Rattus norvegicus reference genome (fasta) and genome annotation files (gtf) were obtained from the Ensembl genome browser2. Reads were aligned to the RNO version 6 release 94 of the Ensembl genome using the STAR v2.5.2 aligner (19). Transcripts aligning to specific genes were counted using the STAR-quantMode geneCounts function to map transcripts to each genome. Files containing microRNA counts and gene counts are located in the GEO database. Small RNA samples were processed using the smallrnaseq python tool (20), which aligns samples using Bowtie and quantifies small RNA read counts using reference fasta and gtf files from RNAcentral.org.
Data Filtering and Quality Control
Genes were filtered out if they were not expressed in any samples or had fewer than 10 counts in half of the samples for each gene. Data filtering and alignment settings were adapted from Lexogen's QuantSeq 3′ mRNA-Seq Kit and integrated Data Analysis Pipeline on Bluebee® platform according to the manufacturer's instructions. Following quality control, analysis included 10,101 liver genes, 9,217 adipose genes, 11,607 kidney genes, and 99,36 brain genes.
Differential Expression Analysis Using DESeq2
Read normalization was conducted using a weighted trimmed mean of the log expression ratios [trimmed mean of M values (TMM)] method to account for variable sequencing depth between samples. Differential expression analysis was conducted using the DESeq2 (21) package in the R programming language.
Heatmaps, Principal Component Analysis, and Volcano Plots
Principal Component Analysis (PCA) was conducted for the initial clustering and characterization of RNAseq data. Hierarchical clustering was used to create a dendrogram classifying samples according to similar transcriptomic profiles using Pearson correlation coefficients. Volcano plots were constructed to visualize genes which surpass a log-fold change of >1.0 (upregulated) or <-1.0 (downregulated) to assess biological significance at adjusted p < 0.05. PCA and volcano plots were all constructed using MatplotLib in python version 3.2.0rc1. Heatmaps and hierarchical clustering were constructed using “heatmapper” package in R software.
Functional Enrichment Annotations
Pathway-based analysis was performed using the Kyoto Encyclopedia of Genes and Genomes (KEGG) database that contains annotated biological functions for genes (22). All differentially expressed genes (DEGs) were additionally categorized into biological pathways using KEGG pathway analysis and Gene Ontology (GO) analysis based on cellular localization, function, and processes using the Database for Annotation, Visualization and Integrated Discovery (DAVID) v6.8 database through the online web application (DAVID Functional Annotation Bioinformatics Microarray Analysis)3. MicroRNA target genes were predicted through miRPath v.3 analysis tools (23). Genetic Interactome Analysis was completed on DEGs from the VAT and PFC using Cytoscape version 3.8.0 (24). STRING application—Protein Query generated a node network that allowed functional enrichment data of all DEGs to be generated. The Kyoto Encyclopedia of Genes and Genomes (KEGG) database was queried to identify the functional pathways corresponding to each gene in each species. All DEGs were cross-referenced to String—disease, specifically Diabetes, Parkinson's and Alzheimer's with a confidence interval of 0.95 and a maximum number of proteins of 500 (25).
qRT-PCR Validation Analyses
Total RNA from each tissue was aliquoted and reverse transcribed into cDNA using the High-Capacity cDNA Reverse Transcription Kit (Applied Biosystems, Catalog # 4368813). The cDNA was diluted to 100 ng/μL and qPCR reactions were performed using 200 ng of total cDNA with primers at 300 nM concentration in 10 μL FastStart Sybr Green Master (Roche) according to the manufacturer's instructions. All qPCR reactions were conducted in a Roche LightCycler 96 Real-Time PCR System. Primers sequences for qPCR (Supplementary Table 1) were obtained from Integrated DNA Technology (IDT) and 18s RNA was used as an internal control for normalization in each tissue.
Statistical Analysis
Data analysis was conducted in Python within an IPython notebook unless otherwise specified. Body weight and food intake group means were analyzed using an unpaired t-test to analyze the difference at p < 0.05 between casein and whole egg treatment groups. When applying DESeq2 for differential gene expression analysis, DESeqDataSetFromMatrix was used to generate p-values and adjusted p-values were calculated using the Benjamini-Hochberg method (26) of false discovery rate (FDR) correction. For all analyses, FDR was controlled at 1% and all adjusted p < 0.05 were considered significant. For functional enrichment analysis, gene identifiers were cross-validated through our differentially expressed genes on the basis of significance at FDR correction of <0.05. All qRT-PCR samples and genes were analyzed in triplicate using the Livak Delta-Delta CT method (27).
Results
RNA Seq Differential Expression
Differential gene expression analyses of the tissues identified two DEGs in the liver, 22 in VAT, 52 in the PFC, and none in the kidney. Of the 76 DEGs that surpassed multiple testing corrections (adjusted p < 0.05), one gene was differentially upregulated across both the PFC (5.6-fold increase) and VAT (3.2-fold increase) tissue: indolethylamine N-methyltransferase (INMT). Supplementary Table 2 describes all of the DEGs in each tissue, whereas Supplementary Table 3 contains only those with a significant p-value after correction in all tissues.
KEGG and GO Functional Enrichment Analysis
To examine the functional pathways for the DEGs, mRNAs were mapped to KEGG/GO pathway terms, which are described in Supplementary Table 4. In the PFC, GO function analysis releveled genes modified by whole egg consumption were significantly enriched in Cholesterol biosynthetic pathways and lipid metabolism. Furthermore, a large genetic network of 16 genes in these two pathways were all down regulated (Supplementary Figure 1A). Of the 12 genes differentially regulated which were involved in Oxidation-reduction process, 9 were downregulated in response to whole egg consumption. In the VAT, Gsta3, Gstz1, and Gstp1, all members of the glutathione transferase activity pathway, were found to be up-regulated by whole egg consumption (Supplementary Figure 1B).
STRING: Diseases database Enrichment Analysis
Using STRING: disease query analysis to examine disease related genes that were differentially expressed in response to WE diet, we identified one gene in VAT which is known to be associated with Diabetes. Specifically, Secreted Phosphoprotein 1 (SPP1) also known as Osteopontin was found to be down regulated in the VAT (−3.14 log2 fold change, Supplementary Table 3).
microRNA Sequencing Differential Expression
Differential expression analyses of microRNAs identified six upregulated microRNAs and 10 downregulated microRNAs across all four tissues based on non-adjusted p < 0.05 (Supplementary Table 5). No microRNAs survived multiple testing correction with FDR correction at 5% value (Supplementary Table 2). miRNAs largely changed in a tissue specific manner, but two miRNAs changed in multiple tissues including miR-10b-5p which was downregulated in both the VAT and PFC (non-adjusted; p = 0.03 and p = 0.02, respectively) and miR-192-5p which was downregulated in both the liver and PFC (non-adjusted; p = 0.02 and p = 0.05, respectively).
microRNA Gene Target Analysis
To gain a better understanding of the possible regulatory role of miRNAs, all 16 differentially expressed microRNAs (Supplementary Table 5) were mapped against their putative human genetic targets using miRPath, and cross-referenced against the DEGs in the corresponding tissue (Supplementary Table 3). Based on this mapping, three miRNAs were identified whose predicted target genes which were also differentially expressed in response to diet in the corresponding tissues (Table 2). These included miR-10b-5p which was downregulated in the PFC. Its corresponding predicted target, Arrestin Domain Containing 3 protein (ARRDC3), was upregulated. miR-192-5p was also suppressed in the PFC with nine putative targets which were upregulated. These included: SPC Membrane Recruitment Protein 1 (Amer 1), Fatty Acid Binding Protein 3 (Fabp3), Protocadherin 17 (Pcdh 17), FERM Domain Containing 4B (Frmd4b), Kinesin Family Member 1B (Kif1b), Collagen type V alpha 1 chain (Col5a1), zinc finger protein 36, C3H type-like 1 (Zfp3611), NIPA Like Domina Containing 1 (Nipal 1), and Sorting Nexin 33 (Snx33, Table 2). Additional gene targets of miR-192-5p in the PFC and miR-125b-5p in the VAT were predicted, but did not follow traditional patterns of miRNA regulation as they and the miRNAs were all down regulated (Table 2).
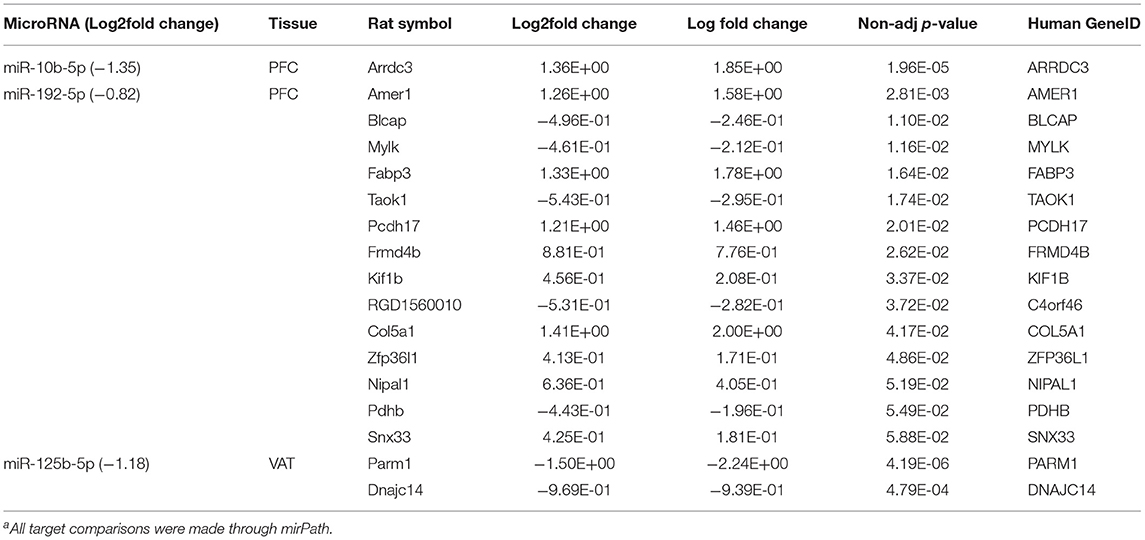
Table 2. Differentially expressed miRNAs and putative target genes in the PFC and VAT of Sprague Dawley rats fed dietary whole egg vs. caseina.
Serum microRNA Refeeding Analysis
There was no significant effect of dietary treatment on serum microRNA at 0, 2, 4, 6, or 8 h immediately following refeeding based on multiple testing corrective measures using FDR adjusted p < 0.05 (data not shown).
Principal Component Analysis (PCA) and Hierarchical Clustering
To examine the similarity between transcriptomic profiles, low expression genes across all tissues were filtered and data illustrated in a PCA, as shown in Figure 2A. The PCA plots indicate that these rat samples cluster by diet (WE vs. CAS). Subsequently, the top DEGs were visualized using a hierarchical clustering heatmap for each tissue that displays distinct differential expression patterns according to each diet, as shown in Figures 2B–D. To visualize the magnitude of log-fold changes, all DEGs were plotted for each corresponding tissue using volcano plots. Generally an equal distribution of both up and down regulated genes were observed in each tissue in response to WE diet. Very few genes were differentially expressed at greater than 2 log2 (Fold Change) in either a positive or negative direction (Figure 3A, Liver; Figure 3B, VAT; Figure 3C, PFC).
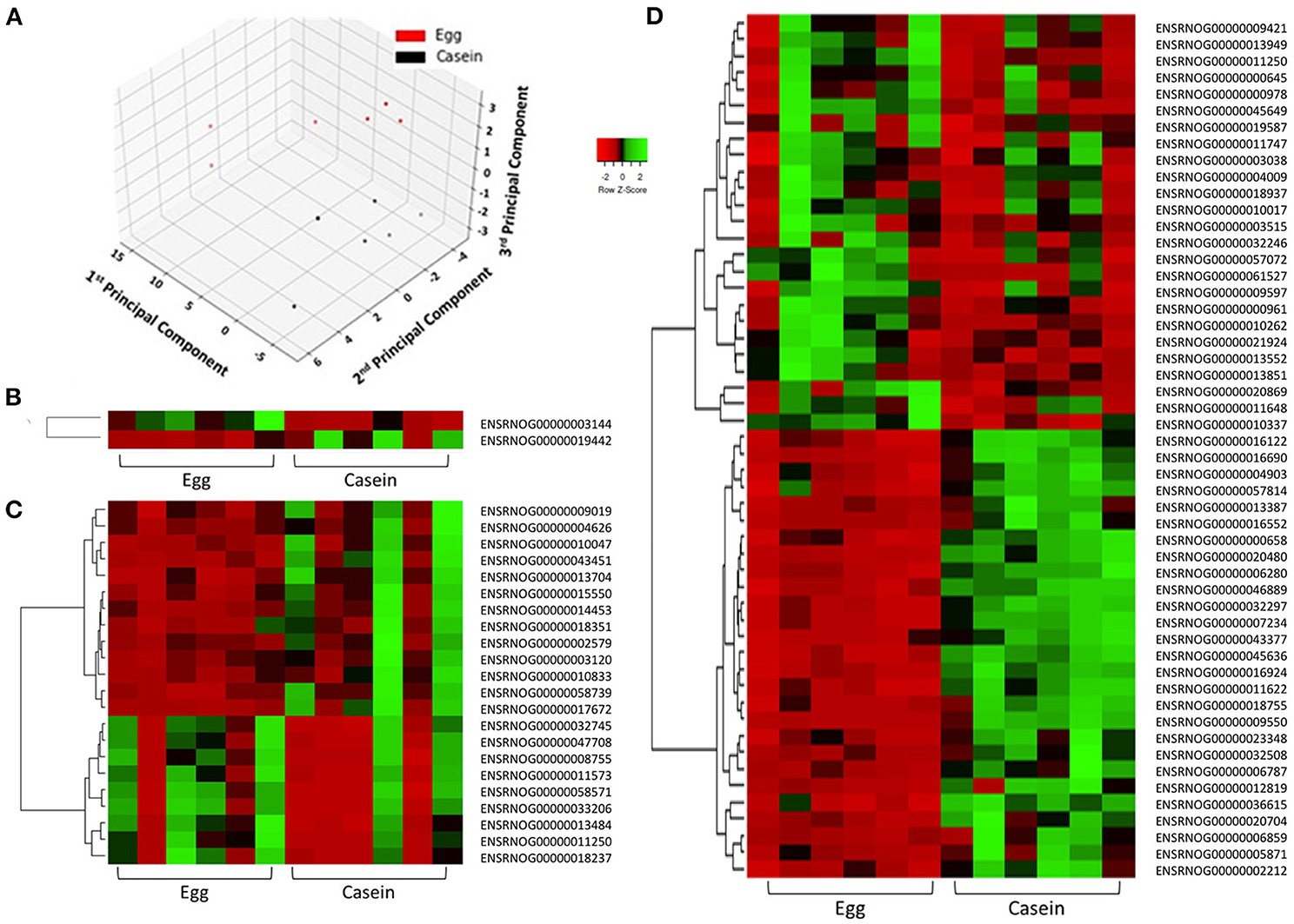
Figure 2. Principle component analysis demonstrates distinct separation of samples according to dietary intervention. Samples are colored in red (WE) or black (CAS, A). Differentially expressed genes in response to dietary intervention in: liver (B), visceral adipose tissue (C), and prefrontal cortex (D) of Sprague Dawley rats demonstrates individual and group transcriptomic response to diet using Pearson correlation coefficients (row z-score scale is indicated −2 Red, 0 Black, and 2 Green).
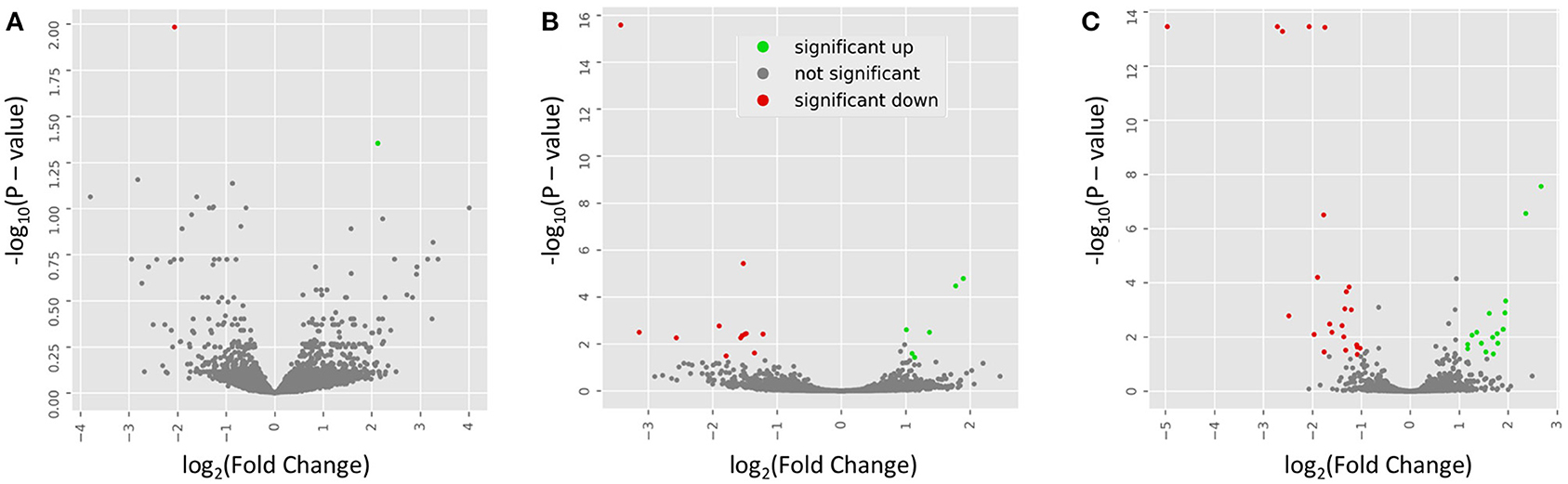
Figure 3. Volcano plots indicate the directionality of significant differentially expressed genes. Genes upregulated (green) or downregulated (red) by whole egg consumption, correspond to a 1.0 decrease or increase in log2 fold change with a log10 P > 1.0. Each panel corresponds to a tissue: (A) liver; (B) visceral adipose tissue; and (C) prefrontal cortex.
qRT-PCR Validation
To validate the RNAseq data, five DEGs were randomly selected for qRT-PCR analysis across each tissue. The PCR gene expression validation data are contained in Supplementary Figure 2. suggesting that RNAseq and PCR results followed the same relative fold trend for each corresponding gene that was validated. A value of 1 indicates no fold change where as those values above the line indicate an increase in fold change and those below indicate a decrease.
Food Intake and Body Weight Gain
Descriptive data indicated no significant effect of dietary treatment on food intake or total energy intake throughout the study (data not shown). Additionally, there was no statistically significant effect of dietary treatment on final body weight or cumulative body weight gain at 9 week of age after 2 weeks and 3 days on the WE diet. There were also no significant differences in organ weights following tissue collection except for the liver, where rats on the WE-based diets had 16% increased relative liver weight (p < 0.01).
Discussion
Specific egg components, such as hen egg lysozymes, have been previously studied in altering gene expression in pig intestinal tissues (28), but very little information is known about how dietary WE affects endogenous gene expression across specific tissues. The beneficial role of a WE-based diet in maintaining vitamin D status and modulating adverse phenotypic outcomes in both a type 1 diabetes (T1D) and type 2 diabetes (T2D) animal models has been consistently demonstrated (12, 29); however, we have not investigated how WE consumption modulates gene expression in a standard rodent model. RNA-sequencing is a powerful tool that can elucidate the influence of dietary patterns at the level of the transcriptome. It is important to examine the global effects of WE consumption in a healthy rodent model to better understand the pathways that link WE and chronic diseases like type 2 diabetes. By assessing the genes that are influenced by WE, we can contribute to scientifically sound intervention strategies using dietary WE as a means of disease mitigation. In this study, next generation sequencing revealed that consuming WE for 2-weeks significantly modified the expression of 76 different genes across the PFC, liver, and VAT.
In the PFC, where the largest number of genes were changed, the top three enriched GO pathways were cholesterol biosynthetic process, fatty acid biosynthetic process and oxidation-reduction process. A genetic network of 16 genes highly represented in the cholesterol biosynthetic process and lipid metabolism were all down regulated. In addition, we found a large number of genes (9/12) in the oxidation-reduction process pathway to be downregulated. Dysregulation of oxidation-reduction pathways is a common thread in dementia, and modulation of this pathway is thought to be important in brain health (30). The observed reduction in expression of oxidation-reduction pathway genes in our study could be due to the high choline content of WE. Recently, associations have been observed between dietary choline intake and reduced risk of incident dementia and improved cognitive performance (31). One gene found to be highly down regulated in the oxidation-reduction pathway was Squalene epoxidase (Sqle), a rate-limiting gene in the sterol biosynthesis pathway (32), which was significantly downregulated (−31-fold) within the PFC of rats fed WE-based vs. CAS-based diets. Sqle is important for steroidal synthesis, and previous studies have demonstrated that ablation of Sqle may disrupt tumorigenesis, owing to blunted cholesterol biosynthesis (33). Moreover, the dysregulation of Sqle has been observed during the onset of diabetes (34, 35). Ge et al. (36) indicated a significant upregulation in the expression of Sqle, as well as an abundance in the protein in a chemically-induced diabetic animal model (37). Interestingly Ding et al. (35), conducted a cross-sectional study in an obese population of men, examining the relationship between body mass index and DEGs. They reported the close association between body mass index and increased expression of Fads1, Sqle, Scd, Cyp51a1, whereas in a weight-loss intervention study, Sqle expression was significantly reduced. Similar to these findings, we observed downregulation of Fads1 and Sqle in animals fed the WE- as compared to the CAS-based diets. A small number of genes included in the oxidation-reduction pathway were upregulated including two cytochrome P450 genes, Cyp2c22, and Cyp4A1 in the PFC of the animals fed WE- vs. CAS-based diets. While additional research is needed, CYPS are known to play a role in susceptibility to neurotoxins, and the risk of developing certain CNS diseases (38).
In the VAT, functional annotation of the DEGs to GO terms indicated a significant enrichment of genes involved in the glutathione S-transferase (GST) activity pathway including glutathione S-transferase zeta 1 (Gstz1), glutathione S-transferase A3 (Gsta3), and glutathione S-transferase pi 1 (Gstp1), which were upregulated 1.9-, 2.6-, and 3.7-fold, respectively, in the VAT of the Sprague Dawley animals fed WE-based diets. Previously, it was reported that deficiency of glutathione-related pathways alters antioxidant responses (39), suggesting that our data may indicate an upregulation of the catalytic GST enzymes, which function in glutathione conjugation for detoxification reactions, with WE consumption providing protection against oxidative stress. In a recent study, a Gstp1 polymorphism was associated with increased glucose intolerance and greater androgen production in non-obese women with polycystic ovary syndrome (40). Moreover, the literature indicates that dysregulated GST production has been implicated in conditions of obesity and T2D (41). It was previously reported that glutathione metabolism is regulated by egg yolk peptide consumption in a porcine model of oxidative stress (42), while in Zucker Diabetic Fatty rats WE consumption led to an upregulation in 14 GST-related enzymes across numerous tissues (43). In a meta-analysis of clinical studies with long-term egg consumption, there were no observed effects of WE consumption on blood inflammatory markers (44). Other researchers have reported elevations in endothelial and arterial inflammation from WE consumption (45) and some report vascular inflammation to be exclusive to egg white consumption and not WE (46, 47). It is important that we examined these differences in gene expression, as we identified upregulation not only in VAT, but also observed an increase of glutathione S-transferase mu 2 (Gstm2) expression in the PFC of rats fed WE-based diet. These are important considerations, as the data from clinical trials regarding WE consumption on inflammation-mediated cardiovascular disease are nuanced and remain contradictory (7, 48–50). When specifically examining genes associated with disease we identified SPP1 which was down regulated 8.8-fold in VAT after consumption of the WE diet. SPP1 also known as Osteopontin is an inflammatory cytokine which is highly upregulated in obesity and has been shown to be a key player for local adipose tissue macrophage proliferation which underlies insulin resistance and type 2 diabetes (51). Importantly Osteopontin has been found to be required for the early onset of high fat diet-induced insulin resistance in mice (52). The down regulation of this gene may account for some of the benefits observed in longer term consumption of WE diet in the Zucker Diabetic Fatty Rats (12, 13) and requires further mechanistic study.
To better understand what may be driving the changes in gene expression in response to WE diet, we performed small RNA sequencing to examine miRNAs. Previous research by Zempleni et al. has reported robust findings for meaningful dietary microRNA absorption from milk and egg sources. They report a postparandial increase in plasma miRNAs in humans suggesting biological value of dietary-derived microRNAs on downstream target genes (53, 54). While it is controversial whether dietary consumption directly influences circulating microRNA status (55), using a more highly processed whole egg product, we did not observe any serum changes in circulating microRNAs between the two dietary treatments in the 8 h post meal consumption. However, we hypothesized that over a longer period of diet consumption, the tissue microRNA profile may change due to diet; thus, we examined the microRNA expression changes in rats following a 2-week consumption period.
Six upregulated and ten down regulated miRNAs were identified across all tissues profiled. However, when the miRNAs putative gene targets were cross-referenced against the differentially expressed genes within each respective tissue, only three microRNAs had putative targets which were also changed in expression. Of these three, two were identified in the PFC (miR-10b-5p and miR192-5p) and one was in the VAT (miR-125b-5p) all three miRNAs were down regulated. ARRDC3, a target gene for miR-10b-5p exhibited a ~1.9-fold change as a result of WE consumption with a non-adjusted p-value of (p = 0.027) was the most relevant to observed phenotypes in our work with the Zucker Diabetic fatty rats. The biological significance of ARRDC3 has been examined in both human and animal studies (56, 57). It has been suggested that ARRDC3 is critically involved in obesity and energy expenditure, owing to its function in fat pad development and role in thermoregulation (58). Although there are no mechanistic studies to date examining the direct role of miR-10b-5p on ARRDC3 as it relates to obesity or subsequent diseases, to date, one study explored the association between miR-10b-5p in children with obesity (59). In a population of normal weight and overweight or obese adolescents, they assessed circulating microRNAs and identified that miR-10b-5p is an obesity-associated microRNA, as it highly correlated with body mass index z-scores (59). Based on our consistent results of WE consumption on reduced weight gain during type 2 diabetes, the results of this study could provide insight into the mechanisms involved in weight reduction. Future mechanistic studies exploring the relationship between miR-10b-5p, ARRDC3 as it relates to obesity, and type 2 diabetes are warranted.
There are several strengths and limitations to consider in our research study. Given the limited data on the mechanisms related to whole egg consumption and disease, our profiling experiments provide valuable data on the altering effects of whole egg consumption on gene expression. Data of this magnitude will help better define target pathways and genes that may be used to further examine the influence of nutrition on disease progression. Limitations such as the duration of dietary intervention should be noted. Furthermore, our functional pathways analysis does have limitations. The first being that pathway analysis does not account for the tissue- and cell-specific function of genes in a given pathway. Additionally, not all gene variants are represented in pathway analysis tools, limiting the scope of tissue specificity in related pathway changes.
Conclusion
In this study, we examined the effects of consuming a WE-based diet for 2 weeks on gene expression across multiple tissues in healthy rats. Our main results revealed 76 novel DEGs across the PFC, liver, VAT, and kidney transcriptomes. For VAT, GO analyses highlighted that glutathione metabolism was upregulated by WE consumption. Furthermore, SPP1 was identified to be highly down regulated in the VAT after WE consumption and should be further explored as it relates to outcomes of WE consumption and Diabetes. In the PFC, a large network of genes from the cholesterol biosynthetic and lipid metabolic process were all down regulated in the PFC. In addition, multiple genes involved in the oxidation-reduction process in the PFC were also altered by WE consumption. These results indicate that, even short-term, WE-based diets modulates the expression of genes. Together, these results may provide information for follow up in models where these genes and pathways are involved in the pathogenesis of disease.
Data Availability Statement
The datasets presented in this study can be found in online repositories. The names of the repository/repositories and accession number(s) can be found at: https://www.ncbi.nlm.nih.gov/geo/query/acc.cgi?acc$=$GSE163193.
Ethics Statement
The animal study was reviewed and approved by Institutional Animal Care and Use Committee (IACUC) at Iowa State University.
Author Contributions
AB, JW, TD, MK, MR, SC, KS, and EM conceptualized the original research. AB, JW, and EM curated and analyzed the data. Methodology of the research studies was developed by KS, EM, AB, and JW. Investigation, experiments, and animal handling was performed by AB, JW, BV, and CC. The original draft of the manuscript was written and prepared by AB and JW. Funding acquisition, project administration and writing, reviewing, and editing was carried out by AB, JW, TD, MK, MR, SC, KS, and EM. All authors contributed to the article and approved the submitted version.
Funding
This work was supported by the College of Human Sciences Intramural Collaborative Grant Program and the Presidential Interdisciplinary Research Seed Grant Program from Iowa State University.
Conflict of Interest
The authors declare that the research was conducted in the absence of any commercial or financial relationships that could be construed as a potential conflict of interest.
Acknowledgments
We would like to thank Simon Moe, for assistance with cytoscape and STRING Disease query analysis and Dr. Peng Liu, Department of Statistics, for assisting in the initial statistical planning of this project. Additionally, the authors would like to thank the ISU DNA facility staff members Kevin Cavallin, Tanya Murtha and Dr. Michael Baker for their assistance sequencing our samples.
Supplementary Material
The Supplementary Material for this article can be found online at: https://www.frontiersin.org/articles/10.3389/fnut.2021.652192/full#supplementary-material
Supplementary Figure 1. Network of interactome representing STRING Network interactions of DEGs in specific tissues. Prefrontal Cortex (A) GO indicated enrichment of involved network genes in cholesterol biosynthetic process (FDR 4.96E−16) and lipid metabolic process (FDR 4.09E−13) and Visceral Adipose Tissue (B) GO indicated enrichment of involved network in Glutathione Transferase activity (FDR 5.1 E−04). Networks connected through “edge” lines with thickness represented by STRING confidence score. Colors in nodes represent gene expression changes, upregulated orange and downregulated blue. Red border indicates gene is identified in STRING network as a Diabetes associated gene.
Supplementary Figure 2. Fold change comparisons between qPCR and mRNA sequencing of several genes validating the directionality of sequencing results. Those above 1 indicate a fold increase in expression due to diet. Those below 1 indicated a decrease fold expression in relation to diet. Fold changes are significant at p < 0.05 unless otherwise indicated. Blue bars represent qPCR data and orange bars represent RNAseq data. Each tissue is indicated by different shading with the name of the tissue at the top. PFC, Prefrontal Cortex; VAT, Visceral Adipose Tissue; ns, not significant.
Supplementary Table 1. Primer sequence as used for qPCR.
Supplementary Table 2. Raw differentially expressed genes in the PFC, liver, adipose, and kidney.
Supplementary Table 3. List of significantly differentially expressed genes in the PFC, liver, adipose, and kidney.
Supplementary Table 4. Kegg pathway and gene ontology (GO) analysis.a,b.
Supplementary Table 5. Differentially expressed microRNAs between Sprague Dawley rats fed dietary whole egg compared to casein control.
Footnotes
1. ^BBMap: A Fast, Accurate, Splice-Aware Aligner. Available online at: https://escholarship.org/uc/item/1h3515gn (accessed January 28, 2020).
2. ^Ensembl genome browser 99. Available online at: https://useast.ensembl.org/index.html (accessed March 25, 2020).
3. ^DAVID Functional Annotation Bioinformatics Microarray Analysis. Available online at: https://david.ncifcrf.gov/home.jsp (accessed March 23, 2020).
References
1. Andersen CJ. Bioactive egg components and inflammation. Nutrients. (2015) 7:7889–913. doi: 10.3390/nu7095372
2. Park SJ, Jung JH, Choi SW, Lee HJ. Association between egg consumption and metabolic disease. Korean J Food Sci Anim Resour. (2018) 38:209–23. doi: 10.5851/kosfa.2018.38.2.209
3. Nimalaratne C, Wu J. Hen egg as an antioxidant food commodity: a review. Nutrients. (2015) 7:8274–93. doi: 10.3390/nu7105394
4. Wallace TC, Fulgoni VL. Usual choline intakes are associated with egg and protein food consumption in the United States. Nutrients. (2017) 9:839. doi: 10.3390/nu9080839
5. Miranda JM, Anton X, Redondo-Valbuena C, Roca-Saavedra P, Rodriguez JA, Lamas A, et al. Egg and egg-derived foods: effects on human health and use as functional foods. Nutrients. (2015) 7:706–29. doi: 10.3390/nu7010706
6. Alexander DD, Miller PE, Vargas AJ, Weed DL, Cohen SS. Meta-analysis of Egg Consumption and Risk of Coronary Heart Disease and Stroke. J Am Coll Nutr. (2016) 35:704–16. doi: 10.1080/07315724.2016.1152928
7. Zhong VW, Van Horn L, Cornelis MC, Wilkins JT, Ning H, Carnethon MR, et al. Associations of dietary cholesterol or egg consumption with incident cardiovascular disease and mortality. J Am Med Assoc. (2019) 321:1081–95. doi: 10.1001/jama.2019.1572
8. Drouin-Chartier JP, Chen S, Li Y, Schwab AL, Stampfer MJ, Sacks FM, et al. Egg consumption and risk of cardiovascular disease: three large prospective US cohort studies, systematic review, updated meta-analysis. BMJ. (2020) 368:m513 368. doi: 10.1136/bmj.m513
9. Dehghan M, Mente A, Rangarajan S, Mohan V, Lear S, Swaminathan S, et al. Association of egg intake with blood lipids, cardiovascular disease, mortality in 177,000 people in 50 countries. Am J Clin Nutr. (2020) 111:795–803. doi: 10.1093/ajcn/nqz348
10. DiMarco DM, Missimer A, Murillo AG, Lemos BS, Malysheva OV, Caudill MA, et al. Intake of up to 3 eggs/day increases HDL cholesterol and plasma choline while plasma trimethylamine-N-oxide is unchanged in a healthy population. Lipids. (2017) 52:255–63. doi: 10.1007/s11745-017-4230-9
11. Fuller NR, Sainsbury A, Caterson ID, Denyer G, Fong M, Gerofi J, et al. Effect of a high-egg diet on cardiometabolic risk factors in people with type 2 diabetes: The Diabetes and Egg (DIABEGG) Study - randomized weight-loss and follow-up phase. Am J Clin Nutr. (2018) 107:921–31. doi: 10.1093/ajcn/nqy048
12. Saande CJ, Jones SK, Hahn KE, Reed CH, Rowling MJ, Schalinske KL. Dietary whole egg consumption attenuates body weight gain is more effective than supplemental cholecalciferol in maintaining vitamin D balance in type 2 diabetic rats. J Nutr. (2017) 31:S1436.2 jn254193. doi: 10.3945/jn.117.254193
13. Saande CJ, Webb JL, Curry PE, Rowling MJ, Schalinske KL. Dietary whole egg reduces body weight gain in a dose-dependent manner in zucker diabetic fatty rats. J Nutr. (2019) 149:1766–75. doi: 10.1093/jn/nxz143
14. Yousr M, Howell N. Antioxidant and ACE inhibitory bioactive peptides purified from egg yolk proteins. Int J Mol Sci. (2015) 16:29161–78. doi: 10.3390/ijms161226155
15. Nataraj J, Manivasagam T, Justin Thenmozhi A, Essa MM. Lutein protects dopaminergic neurons against MPTP-induced apoptotic death and motor dysfunction by ameliorating mitochondrial disruption and oxidative stress. Nutr Neurosci. (2016) 19:237–46. doi: 10.1179/1476830515Y.0000000010
16. Akbaraly NT, Faure H, Gourlet V, Favier A, Berr C. Plasma carotenoid levels and cognitive performance in an elderly population: results of the EVA study. J Gerontol A Biol Sci Med Sci. (2007) 62:308–16. doi: 10.1093/gerona/62.3.308
17. Binawade Y, Jagtap A. Neuroprotective effect of lutein against 3-nitropropionic acid-induced huntington's disease-like symptoms: possible behavioral, biochemical, cellular alterations. J Med Food. (2013) 16:934–43. doi: 10.1089/jmf.2012.2698
18. Edgar R, Domrachev M, Lash AE. Gene expression omnibus: NCBI gene expression and hybridization array data repository. Nucleic Acids Res. (2002) 30:207–10. doi: 10.1093/nar/30.1.207
19. Dobin A, Davis CA, Schlesinger F, Drenkow J, Zaleski C, Jha S, et al. STAR: Ultrafast universal RNA-seq aligner. Bioinformatics. (2013) 29:15–21. doi: 10.1093/bioinformatics/bts635
20. Farrell D. smallrnaseq : short non coding RNA-seq analysis with Python. Bioarxiv. (2017) 110585. doi: 10.1101/110585
21. Love MI, Huber W, Anders S. Moderated estimation of fold change and dispersion for RNA-seq data with DESeq2. Genome Biol. (2014) 15:550. doi: 10.1186/s13059-014-0550-8
22. Kanehisa M, Goto S. KEGG: Kyoto Encyclopedia of Genes and Genomes. Available online at: http://www.genome.ad.jp/kegg/ (accessed March 23, 2020).
23. Vlachos IS, Zagganas K, Parakevopoulou MD, Georgakilas G, Karagkouni D, Vergoulis T, et al. Diana-miRPath v3.0: deciphering microRNA function with experimental support. Nucleic Acids Res. (2015) 43:W460–6. doi: 10.1093/nar/gkv403
24. Shannon P, Markiel A, Ozier O, Baliga NS, Wang JT, Ramage D, et al. Cytoscape: a software Environment for integrated models of biomolecular interaction networks. Genome Res. (2003) 13:2498–504. doi: 10.1101/gr.1239303
25. Doncheva NT, Morris JH, Gorodkin J, Jensen LJ. Cytoscape StringApp: network analysis and visualization of proteomics data. J Proteome Res. (2019) 18:623–32. doi: 10.1021/acs.jproteome.8b00702
26. Benjamini Y, Hochberg Y. Controlling the False Discovery Rate: a Practical and powerful Approach to Multiple Testing. J R Stat Soc B. (1995) 57:289–300. doi: 10.1111/j.2517-6161.1995.tb02031.x
27. Livak KJ, Schmittgen TD. Analysis of relative gene expression data using real-time quantitative PCR and the 2-ΔΔCT method. Methods. (2001) 25:402–8. doi: 10.1006/meth.2001.1262
28. Lee M, Kovacs-Nolan J, Yang C, Archbold T, Fan MZ, Mine Y. Hen egg lysozyme attenuates inflammation and modulates local gene expression in a porcine model of dextran sodium sulfate (DSS)-induced colitis. J Agric Food Chem. (2009) 57:2233–40. doi: 10.1021/jf803133b
29. Jones SK, Koh GY, Rowling MJ, Schalinske KL. Whole egg consumption prevents diminished serum 25-hydroxycholecalciferol concentrations in type 2 diabetic rats. J Agric Food Chem. (2016) 64:120–4. doi: 10.1021/acs.jafc.5b04347
30. von Bernhardi R, Eugenín J. Alzheimer's disease: Redox dysregulation as a common denominator for diverse pathogenic mechanisms. Antioxidants Redox Signal. (2012) 16:974–1031. doi: 10.1089/ars.2011.4082
31. Ylilauri MPT, Voutilainen S, Lönnroos E, Virtanen HEK, Tuomainen TP, Salonen JT, et al. Associations of dietary choline intake with risk of incident dementia and with cognitive performance: The Kuopio Ischaemic Heart Disease Risk Factor Study. Am J Clin Nutr. (2019) 110:1416–23. doi: 10.1093/ajcn/nqz148
32. Boone LR, Brooks PA, Niesen MI, Ness GC. Mechanism of resistance to dietary cholesterol. J Lipids. (2011) 2011:1–9. doi: 10.1155/2011/101242
33. Garcia-Bermudez J, Baudrier L, Bayraktar EC, Shen Y, La K, Guarecuco R, et al. Squalene accumulation in cholesterol auxotrophic lymphomas prevents oxidative cell death. Nature. (2019) 567:118–22. doi: 10.1038/s41586-019-0945-5
34. Seiki S, Frishman WH. Pharmacologic inhibition of squalene synthase and other downstream enzymes of the cholesterol synthesis pathway: a new therapeutic approach to treatment of hypercholesterolemia. Cardiol Rev. (2009) 17:70–6. doi: 10.1097/CRD.0b013e3181885905
35. Ding J, Reynolds LM, Zeller T, Müller C, Lohman K, Nicklas BJ, et al. Alterations of a cellular cholesterol metabolism network are a molecular feature of obesity-related type 2 diabetes and cardiovascular disease. Diabetes. (2015) 64:3464–74. doi: 10.2337/db14-1314
36. Ge Q, Zhang S, Chen L, Tang M, Liu L, Kang M, et al. Mulberry leaf regulates differentially expressed genes in diabetic mice liver based on RNA-seq analysis. Front Physiol. (2018) 9:1051. doi: 10.3389/fphys.2018.01051
37. Ge Q, Feng F, Liu L, Chen L, Lv P, Ma S, et al. RNA-Seq analysis of the pathogenesis of STZ-induced male diabetic mouse liver. J Diabetes Complicat. (2020) 34:107444. doi: 10.1016/j.jdiacomp.2019.107444
38. Ferguson CS, Tyndale RF. Cytochrome P450 enzymes in the brain: emerging evidence of biological significance. Trends Pharmacol Sci. (2011) 32:708–14. doi: 10.1016/j.tips.2011.08.005
39. Blackburn AC, Matthaei KI, Lim C, Taylor MC, Cappello JY, Hayes JD, et al. Deficiency of glutathione transferase zeta causes oxidative stress and activation of antioxidant response pathways. Mol Pharmacol. (2006) 69:650–7. doi: 10.1124/mol.105.018911
40. Savic-Radojevic A, Mazibrada I, Djukic T, Stankovic ZB, Plješa-Ercegovac M, Sedlecky K, et al. Glutathione S-transferase (GST) polymorphism could be an early marker in the development of PCOS: An insight from non-obese and non-insulin resistant adolescents. Endokrynol Pol. (2018) 69:366–74. doi: 10.5603/EP.a2018.0034
41. Dastidar SG, Jagatheesan G, Haberzettl P, Shah J, Hill BG, Bhatnagar A, et al. Glutathione s-transferase p deficiency induces glucose intolerance via jnk-dependent enhancement of hepatic gluconeogenesis. Am J Physiol Endocrinol Metab. (2018) 315:E1005–18. doi: 10.1152/ajpendo.00345.2017
42. Young D, Fan MZ, Mine Y. Egg Yolk peptides up-regulate glutathione synthesis and antioxidant enzyme activities in a porcine model of intestinal oxidative stress. J Agric Food Chem. (2010) 58:7624–33. doi: 10.1021/jf1011598
43. Webb JL, Bries AE, Vogel B, Carrillo C, Harvison L, Day TA, et al. Whole egg consumption increases gene expression within the glutathione pathway in the liver of Zucker Diabetic Fatty rats. PLoS ONE. (2020) 15:e0240885. doi: 10.1371/journal.pone.0240885
44. Sajadi Hezaveh Z, Sikaroudi MK, Vafa M, Clayton ZS, Soltani S. Effect of egg consumption on inflammatory markers: a systematic review and meta-analysis of randomized controlled clinical trials. J Sci Food Agric. (2019) 99:6663–70. doi: 10.1002/jsfa.9903
45. David Spence J. Dietary cholesterol and egg yolk should be avoided by patients at risk of vascular disease. J Transl Intern Med. (2016) 4:20–4. doi: 10.1515/jtim-2016-0005
46. Njike V, Faridi Z, Dutta S, Gonzalez-Simon AL, Katz DL. Daily egg consumption in hyperlipidemic adults - effects on endothelial function and cardiovascular risk. Nutr J. (2010) 9:28. doi: 10.1186/1475-2891-9-28
47. Jiayu Y, Botta A, Simtchouk S, Winkler J, Renaud LM, Dadlani H, et al. Egg white consumption increases GSH and lowers oxidative damage in 110-week-old geriatric mice hearts. J Nutr Biochem. (2020) 76:108252. doi: 10.1016/j.jnutbio.2019.108252
48. Wang MX, Wong CH, Kim JE. Impact of whole egg intake on blood pressure, lipids and lipoproteins in middle-aged and older population: a systematic review and meta-analysis of randomized controlled trials. Nutr Metab Cardiovasc Dis. (2019) 29:653–64. doi: 10.1016/j.numecd.2019.04.004
49. Zhuang P, Wu F, Mao L, Zhu F, Zhang Y, Chen X, et al. Egg and cholesterol consumption and mortality from cardiovascular and different causes in the United States: a population-based cohort study. PLoS Med. (2021) 18:e1003508. doi: 10.1371/journal.pmed.1003508
50. Xia P, Pan X, Chen C, Yi Y, Ye Y, Pan A. Dietary intakes of eggs and cholesterol in relation to all-cause and heart disease mortality: a prospective cohort study. J Am Heart Assoc. (2020) 9:e015743. doi: 10.1161/JAHA.119.015743
51. Tardelli M, Zeyda K, Moreno-Viedma V, Wanko B, Grün NG, Staffler G, et al. Osteopontin is a key player for local adipose tissue macrophage proliferation in obesity. Mol Metab. (2016) 5:1131–7. doi: 10.1016/j.molmet.2016.09.003
52. Chapman J, Miles PD, Ofrecio JM, Neels JG, Yu JG, Resnik JL, et al. Osteopontin is required for the early onset of high fat diet-induced insulin resistance in mice. PLoS ONE. (2010) 5:e13959. doi: 10.1371/journal.pone.0013959
53. Baier SR, Nguyen C, Xie F, Wood JR, Zempleni J. MicroRNAs are absorbed in biologically meaningful amounts from nutritionally relevant doses of cow milk and affect gene expression in peripheral blood mononuclear cells, HEK-293 kidney cell cultures, mouse livers. J Nutr. (2014) 144:1495–500. doi: 10.3945/jn.114.196436
54. Zempleni J, Baier SR, Howard KM, Cui J. Gene regulation by dietary microRNAs. Can J Physiol Pharmacol. (2015) 93:1097–102. doi: 10.1139/cjpp-2014-0392
55. Witwer KW, Zhang CY. Diet-derived microRNAs: unicorn or silver bullet? Genes Nutr. (2017) 12:15. doi: 10.1186/s12263-017-0564-4
56. Carroll SH, Zhang E, Wang BF, LeClair KB, Rahman A, Cohen DE, et al. Adipocyte arrestin domain-containing 3 protein (Arrdc3) regulates uncoupling protein 1 (Ucp1) expression in white adipose independently of canonical changes in β-adrenergic receptor signaling. PLoS ONE. (2017) 12:e181492. doi: 10.1371/journal.pone.0181492
57. Lin H, Rogers GT, Lunetta KL, Levy D, Miao X, Troy LM, et al. Healthy diet is associated with gene expression in blood: The Framingham Heart Study. Am J Clin Nutr. (2019) 110:742–9. doi: 10.1093/ajcn/nqz060
58. Yates AD, Achuthan P, Akanni W, Allen J, Allen J, Alvarez-Jarreta J, et al. Ensembl 2020. Nucl Acids Res. (2020) 48:D682–8. doi: 10.1093/nar/gkz966
59. Iacomino G, Russo P, Marena P, Lauria F, Venezia A, Ahrens W, et al. Circulating microRNAs are associated with early childhood obesity: results of the I.Family Study. Genes Nutr. (2019) 622–626. doi: 10.1186/s12263-018-0622-6
Nomenclature
Akrc1, aldo-keto reductase family
CAS, casein
Cyp51, Lanosterol 14α demethylase
DAVID, Database for Annotation, Visualization and Integrated Discovery
DEGs, differentially expressed genes
DMT, N,N-dimethyltryptamine
FDR, false discovery rate
GO, gene ontology
GST, glutathione S-transferase
GSTP1, glutathione S-transferase pi 1
GSTZ1, glutathione S-transferase zeta 1
INMT, indolethylamine N-methyltransferase
KEGG, Kyoto Encyclopedia of Genes and Genomes
KNN, K-nearest-neighbor
PCA, Principal Component Analysis
PFC, prefrontal cortex
RIN, RNA integrity number
SAM, S-Adenosyl-methionine
Sqle, Squalene epoxidase
T1D, type 1 diabetes
T2D, type 2 diabetes
TMM, trimmed mean of M values
VAT, visceral adipose tissue
WE, whole egg
Keywords: differential expression analysis, nutrigenetics/nutrigenomics, RNAseq, Sprague Dawley, miRNA–microRNA
Citation: Bries AE, Webb JL, Vogel B, Carrillo C, Day TA, Kimber MJ, Valentine RJ, Rowling MJ, Clark S, Schalinske KL and McNeill EM (2021) RNA Sequencing Reveals Key Metabolic Pathways Are Modified by Short-Term Whole Egg Consumption. Front. Nutr. 8:652192. doi: 10.3389/fnut.2021.652192
Received: 11 January 2021; Accepted: 06 April 2021;
Published: 10 May 2021.
Edited by:
Brian J. Bennett, Western Human Nutrition Research Center, Agricultural Research Service, United States Department of Agriculture (USDA), United StatesReviewed by:
Clara E. Cho, Utah State University, United StatesNatalia Krupenko, University of North Carolina at Chapel Hill, United States
Copyright © 2021 Bries, Webb, Vogel, Carrillo, Day, Kimber, Valentine, Rowling, Clark, Schalinske and McNeill. This is an open-access article distributed under the terms of the Creative Commons Attribution License (CC BY). The use, distribution or reproduction in other forums is permitted, provided the original author(s) and the copyright owner(s) are credited and that the original publication in this journal is cited, in accordance with accepted academic practice. No use, distribution or reproduction is permitted which does not comply with these terms.
*Correspondence: Elizabeth M. McNeill, ZW1jbmVpbGxAaWFzdGF0ZS5lZHU=
†These authors have contributed equally to this work