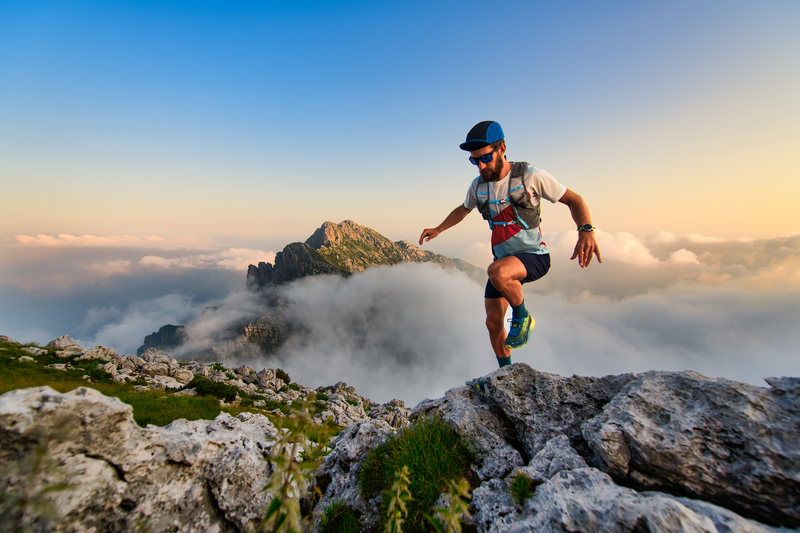
94% of researchers rate our articles as excellent or good
Learn more about the work of our research integrity team to safeguard the quality of each article we publish.
Find out more
ORIGINAL RESEARCH article
Front. Neurosci.
Sec. Brain Imaging Methods
Volume 19 - 2025 | doi: 10.3389/fnins.2025.1532433
This article is part of the Research Topic Current Challenges and Future Perspectives in Neuro-Oncological Imaging View all 3 articles
The final, formatted version of the article will be published soon.
You have multiple emails registered with Frontiers:
Please enter your email address:
If you already have an account, please login
You don't have a Frontiers account ? You can register here
Background: Patients undergoing brain tumor resection experience neurological and cognitive (i.e., neurocognitive) changes reflected in altered performance on neuropsychological tests. These changes can be difficult to explain or predict. Brain connectivity, measured with neuroimaging, offers one potential model for examining these changes. In this study, we evaluated whether longitudinal changes in brain connectivity correlated with changes in neurocognitive abilities in patients before and after brain tumor resection. Methods: Patients underwent functional and diffusion MR scanning and neuropsychological evaluation before tumor resection followed by repeat scanning and evaluation two weeks postresection. Using this functional and diffusion imaging data, we measured changes in the topology of the functional and structural networks. From the neuropsychological testing scores, we derived a composite score that described a patient's overall level of neurocognitive functioning. We then used a multiple linear regression model to test whether structural and functional connectivity measures were correlated with changes in composite scores. Results: Multiple linear regression on 21 subjects showed that connectivity changes were highly correlated with changes in neuropsychological evaluation scores (R 2 adjusted = 0.79, p<0.001). Changes in functional local efficiency (p<0.001) and global efficiency (p<0.05) were inversely correlated with changes in composite score, while changes in modularity (p<0.01) as well as the patient's age (p<0.05) were directly correlated with changes in composite score. Conclusion: Short interval changes in brain connectivity markers were strongly correlated with changes in the composite neuropsychological test scores in brain tumor resection patients. Our findings support the need for further exploration of brain connectivity as a biomarker relevant to brain tumor patients.
Keywords: brain connectivity, brain tumor, graph theory, connectome, neuropsychological evaluation
Received: 22 Nov 2024; Accepted: 24 Feb 2025.
Copyright: © 2025 Ellis, Warren, Garlinghouse and Aizenberg. This is an open-access article distributed under the terms of the Creative Commons Attribution License (CC BY). The use, distribution or reproduction in other forums is permitted, provided the original author(s) or licensor are credited and that the original publication in this journal is cited, in accordance with accepted academic practice. No use, distribution or reproduction is permitted which does not comply with these terms.
* Correspondence:
Michele R Aizenberg, University of Nebraska Medical Center, Omaha, United States
Disclaimer: All claims expressed in this article are solely those of the authors and do not necessarily represent those of their affiliated organizations, or those of the publisher, the editors and the reviewers. Any product that may be evaluated in this article or claim that may be made by its manufacturer is not guaranteed or endorsed by the publisher.
Research integrity at Frontiers
Learn more about the work of our research integrity team to safeguard the quality of each article we publish.