- 1Alzheimer’s Disease Convergence Research Center, Samsung Medical Center, Seoul, Republic of Korea
- 2Department of Neurology, Samsung Medical Center, Sungkyunkwan University School of Medicine, Seoul, Republic of Korea
- 3Neuroscience Center, Samsung Medical Center, Seoul, Republic of Korea
- 4Department of Neurology, Seoul National University Hospital, Seoul, Republic of Korea
- 5Department of Neurology, Soonchunhyang University Bucheon Hospital, Gyeonggi-do, Republic of Korea
- 6Artificial Intelligence Graduate School, Gwangju Institute of Science and Technology, Gwangju, Republic of Korea
Introduction: Alzheimer’s disease (AD) is a progressive neurodegenerative disease characterized by cerebral cortex atrophy. In this study, we used sparse canonical correlation analysis (SCCA) to identify associations between single nucleotide polymorphisms (SNPs) and cortical thickness in the Korean population. We also investigated the role of the SNPs in neurological outcomes, including neurodegeneration and cognitive dysfunction.
Methods: We recruited 1125 Korean participants who underwent neuropsychological testing, brain magnetic resonance imaging, positron emission tomography, and microarray genotyping. We performed group-wise SCCA in Aβ negative (−) and Aβ positive (+) groups. In addition, we performed mediation, expression quantitative trait loci, and pathway analyses to determine the functional role of the SNPs.
Results: We identified SNPs related to cortical thickness using SCCA in Aβ negative and positive groups and identified SNPs that improve the prediction performance of cognitive impairments. Among them, rs9270580 was associated with cortical thickness by mediating Aβ uptake, and three SNPs (rs2271920, rs6859, rs9270580) were associated with the regulation of CHRNA2, NECTIN2, and HLA genes.
Conclusion: Our findings suggest that SNPs potentially contribute to cortical thickness in AD, which in turn leads to worse clinical outcomes. Our findings contribute to the understanding of the genetic architecture underlying cortical atrophy and its relationship with AD.
1 Introduction
Alzheimer’s disease (AD) is a progressive neurodegenerative disorder caused by the accumulation of β-amyloid (Aβ) plaques and neurofibrillary tangles, with subsequent neurodegeneration and cognitive decline (Serrano-Pozo et al., 2011). The amyloid hypothesis of AD, which suggests that Aβ accumulations in the brain are a central event in disease pathology, remains a dominant theory of disease causation (Goate et al., 1991; Selkoe, 1991; Karran et al., 2011). Neurodegeneration is the downstream pathologic process of Aβ accumulation and can be detected by cerebral atrophy or hypometabolism. Although neurodegeneration is not an AD-specific process, it is closely associated with its clinical symptoms and prognosis. Therefore, neurodegeneration is still recognized as a nonspecific but important biomarker in the National Institute on Aging-Alzheimer’s Association (NIA-AA) criteria for AD diagnosis and staging (Jack et al., 2018).
Several imaging genetic association studies have identified genetic biomarkers associated with cortical atrophy that serve as typical surrogate markers for neurodegeneration (Bakken et al., 2011; Wolthusen et al., 2015; Kim et al., 2020; Brouwer et al., 2022). However, the genetic mechanisms underlying the cortical atrophy in AD are complex. Further genetic studies are necessary to elucidate the intricate genetic mechanisms that contribute to cortical atrophy in AD. In recent years, advanced imaging genetics approaches have utilized complex machine-learning models (Kim et al., 2017, 2021; Arslan, 2018; Jacobs and Voineskos, 2020). These approaches not only identify genetic variants that affect brain structure and functional activities, but also provide a comprehensive understanding of the genetic mechanisms underlying these disorders using multivariate algorithms (Sim et al., 2013; Fang et al., 2016; Lorenzi et al., 2016; Kim et al., 2022; Kong et al., 2023).
Over the past few decades, several multivariate studies have emerged that integrate multiple data modalities, including partial least squares, parallel independent component analysis, and canonical correlation analysis (CCA), which have been used for genetic imaging studies (Witten and Tibshirani, 2009; Chi et al., 2013; Pearlson et al., 2015; Beaton et al., 2016; Kim et al., 2022). CCA has been widely used in the field of imaging genetics as a multivariate approach (Chi et al., 2013; Du et al., 2016; Fang et al., 2016; Liu et al., 2017). The fundamental concept underlying CCA is transforming the embedding space to maximize the cross-correlation between two sets of data (Hotelling, 1992). Researchers have employed L1 regularization in CCA, referred to as sparse CCA (SCCA), to identify genetic variants and address overfitting issues commonly encountered in high-dimensional datasets (Waaijenborg et al., 2008; Chu et al., 2013; Fang et al., 2016).
Cortical thickness is one of the sensitive markers of cortical atrophy and global and regional abnormality of cortical thickness in AD compared with cognitive unimpaired subjects have been widely reported (Lerch et al., 2005; Du et al., 2007; Dickerson et al., 2009). The pattern of brain atrophy in AD is different from that observed in normal aging, and cortical atrophy due to aging may be locally severe as in AD (Pini et al., 2016). Numerous studies support that Aβ pathophysiology may function as a trigger/facilitator of downstream molecular pathways that leads to cortical neurodegeneration (He et al., 2018; Busche and Hyman, 2020; Hampel et al., 2021) and cortical thinning is regarded as an indicator of the burden of neurofibrillary tangles and plaques, and neuronal loss that are related to AD (Dickerson et al., 2009).
The purpose of this study is to identify genetic variants related to cortical thickness in the Korean population. In addition, we hypothesized that genetic mechanisms affecting cortical atrophy may differ depending on Aβ accumulation. Thus, we performed SCCA with genetic variants and cortical thickness independently in Aβ positive (Aβ (+)) and negative (Aβ (−)) and identified cortical thickness related SNPs in each group. Next, we evaluated whether selected single nucleotide polymorphisms (SNPs) contribute to improving the predictive performance of cognitive function. In addition, we performed functional effects of selected SNPs and evaluated the indirect effect of SNPs on the cortical thickness through Aβ (Figure 1).
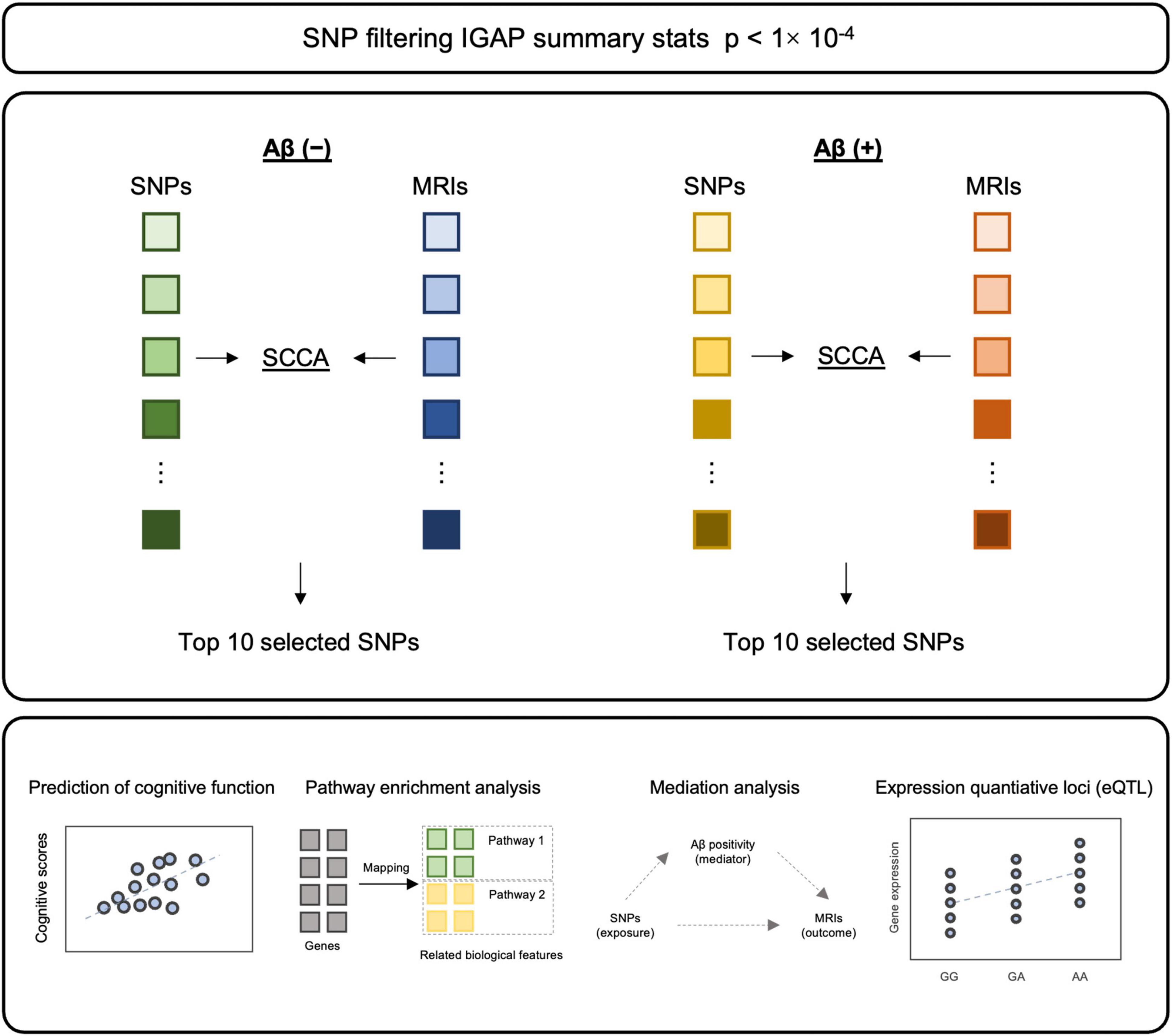
Figure 1. The schematic diagram of methodology. After filtering SNPs with p-value from IGAP summary statistics, SCCA was applied to each Aβ (–) and Aβ (+) for SNP selection. Top 10 SNPs from each model were selected, followed by validation and post-hoc analyses.
2 Materials and methods
2.1 Study participants
The study participants were enrolled from the Korea-Registries to Overcome and Accelerate Dementia Research Project (K-ROAD). The K-ROAD aims to develop a genotype–phenotype cohort to accelerate the development of novel diagnostic and therapeutic techniques for Alzheimer’s and concomitant cerebrovascular diseases, and the 25 university-affiliated hospitals in South Korea have participated. All participants underwent neuropsychological testing, high-resolution T1-weighted magnetic resonance imaging (MRI), and microarray genotyping. The 1125 participants consisted of individuals diagnosed with dementia of Alzheimer’s type (DAT) (n = 447), amnestic mild cognitive impairment (aMCI) (n = 368), and the cognitively unimpaired (CU) (n = 310). All participants with CU met the following criteria: (1) no medical history that was likely to affect cognitive function based on Christensen’s health screening criteria; (2) no objective cognitive impairment in any cognitive domain on a comprehensive neuropsychological test battery (at least −1.0 SD above age-adjusted norms on any cognitive test); and (3) independence in daily living activities. All participants with aMCI met the criteria for aMCI with the following modifications (Albert et al., 2011): (1) subjective cognitive complaints by the participants or caregivers; (2) objective memory impairment below −1.0 SD on verbal or visual memory tests; (3) no significant impairment in daily living activities; and (4) non-demented status. The participants with dementia of the Alzheimer’s type met the NIA-AA criteria of probable AD (McKhann et al., 2011).
Participants with significant white matter hyperintensities (cap or band > 10 mm and longest diameter of deep white matter lesion > 25 mm); structural lesions, including cerebral infarction, intracranial hemorrhage, brain tumors, or hydrocephalus on MRI; abnormal laboratory results on complete blood count; electrolyte, vitamin B12, or folate levels; syphilis serology; or abnormal liver, kidney, or thyroid function tests were excluded from the study.
The Institutional Review Board of the Samsung Medical Center approved this study. Written informed consent was obtained from all the participants.
2.2 Genotyping and imputation
SNP data were genotyped using the Illumina Asian Screening Array BeadChip (Illumina, CA, USA). Quality control (QC) steps were performed using PLINK software (Purcell et al., 2007). The samples with call rate < 95%, sex-mismatch, excess heterozygosity rate (five standard deviations from the mean), and identify-by-descent ≥ 0.125 were excluded. The markers with call rate < 98%, minor allele frequency (MAF) < 1% and Hardy-Weinberg equilibrium p < 10–6 were excluded. After performing QC, un-genotyped markers were imputed using Minimac4 and reference haplotypes from HRC-r1.1 on the University of Michigan Imputation Server (Das et al., 2016). After performing imputation, SNPs with poor imputation quality r2 ≤ 0.8 and MAF < 1% were excluded. Finally, 4, 906, 407 biallelic SNPs in autosomal chromosomes (sex chromosome, mitochondrial, and pseudo-autosomal SNPs were excluded) were used for subsequent analyses.
2.3 Amyloid positron emission tomography (PET) acquisition and visual assessment
All participants underwent either 18F-florbetaben (FBB) or 18F-flutemetamol (FMM) PET at the SMC using a Discovery STe PET/CT scanner (GE Medical Systems, Milwaukee, WI, USA) in 3-dimensional (3D) scanning mode that examined 47 slices of 3.3 mm thickness spanning the entire brain (Jang et al., 2019). A visual assessment was performed to determine Aβ peptide deposition positivity, and the detailed process has been described previously (Cho et al., 2020). Briefly, tracer uptake was assessed according to the regional cortical tracer uptake system in four brain regions (frontal cortex, posterior cingulate cortex/precuneus, parietal cortex, and lateral temporal cortex) for FBB scans and in five regions (frontal, temporoparietal/insula, posterior cingulate/precuneus, lateral temporal, and striatum) for FMM scans. Aβ positivity was defined as whether tracer uptake was observed in any of these regions (Cho et al., 2020).
2.4 MRI acquisition and processing
Three-dimensional T1-weighted MR images were acquired using a 3.0T MRI scanner (Philips 3.0T Achieva; Philips Healthcare, Andover, MA, USA), as previously described (Kang et al., 2022). Images were processed using the CIVET anatomical pipeline to measure cortical thickness (Zijdenbos et al., 2002). The detailed processing pipeline has been described previously (Kang et al., 2022). Briefly, T1-weighted MR images were registered to the MNI-152 template (Collins et al., 1994) and corrected for intensity non-uniformities (Sled et al., 1998). Tissue classification was then performed (Zijdenbos et al., 2002), and the hemispheric inner and outer cortical surfaces were extracted using constrained Laplacian-based automated segmentation with a proximity algorithm (MacDonald et al., 2000; June et al., 2005). Cortical thickness was measured by calculating the Euclidean distance between the corresponding vertices on the inner and outer cortical surfaces (Lerch and Evans, 2005).
2.5 SNP identification based on SCCA
For genetic biomarker identification, we performed SCCA. Given datasets X ∈ ℝn × p, Y ∈ ℝn × q with n samples, X denotes p features of SNP data, and Y denotes q features of imaging data. Witten et al. proposed the SCCA model. The model aims to identify the best association between two datasets, and is defined as follows:
where u and v denote the corresponding canonical vectors. The l1 regularization was applied to control model sparsity (Witten and Tibshirani, 2009).
In our experiments, we first selected candidate SNPs and brain regions to mitigate overfitting. For the genotyping data, we filtered SNPs with p value < 1 × 10–4 in the International Genomics of Alzheimer’s Project (IGAP) summary statistics (Lambert et al., 2013), a meta-analysis of genome-wide association (GWA) data for AD. Additionally, we applied a clumping technique to prune redundant correlated effects resulting from linkage disequilibrium among the SNPs. For imaging data, the average cortical thickness in the temporal, frontal, parietal, and occipital lobes and the global mean cortical thickness adjusted for age, sex, education, and intracranial volume (ICV) were used. Then, we performed SCCA implemented in the Python package ‘cca-zoo’ (Parkhomenko et al., 2009) to identify genetic variants associated with cortical thickness. This analysis was performed separately for the Aβ (+) and Aβ (−) groups, as well as for the total samples. We selected a total of 20 SNPs (i.e., 10 SNPs from each of the Aβ (+) and Aβ (−) groups) in terms of the absolute weight of the canonical vectors for further analysis. The identified SNPs were used for subsequent analyses.
2.6 Validation of SNP selection model
The clinical efficacy of the identified SNPs was evaluated by examining their ability to predict global cortical thickness and cognitive function. First, the focus of the prediction tasks was to validate the clinical efficacy of the SNPs across different amyloid positivity groups (Aβ (+) and Aβ (−)). To accomplish this, the predictive models were trained for one group with repeated 5-fold cross-validation 10 times and tested on the other group. The elastic net regression was initially trained using the top 10 SNPs identified from SCCA within the Aβ (−) group and tested on the Aβ (+) samples. Subsequently, the model was trained using the same set of 10 SNPs from SCCA within the Aβ (+) groups and tested on Aβ (−) samples. Secondly, we evaluated the performance of SNPs in predicting cortical thickness and cognitive function for the Aβ (+) and Aβ (−) groups, as well as for the total samples, using elastic net regression.
2.7 Pathway analysis
We examined the biological concordance of specific SNPs identified through the aforementioned analysis by performing pathway analysis using Enrichr1 (Xie et al., 2021). For our pathway analysis, we utilized comprehensive and well-curated annotations provided by the Gene Ontology (GO) resources.2 By integrating information from the GO annotations, we aimed to gain deeper insights into the potential biological pathways and functional relationships implicated by the selected SNPs.
2.8 Statistical analysis
We performed statistical analyses to examine the impact of specific SNPs in two distinct aspects: (1) examination of the effects of the selected SNPs on AD (i.e., CU and AD) using logistic regression after controlling for age, sex, and education, and (2) examination of the effects of selected SNPs on cortical atrophy, with amyloid positivity as a mediator. Specifically, we employed structural equation modeling adjusted for potential confounding variables, including age, sex, education, and ICV, as implemented in R (mediation package). The significance of the results was determined using a p-value cutoff of < 0.05.
2.9 Expression quantitative trait loci (eQTL) analysis
We performed expression quantitative trait loci (eQTL) analysis to determine the functional effects of SNPs on gene expression using the GTEx database.3 To investigate whether any of the variants were eQTLs in brain tissues and whole blood, we used GTEx Analysis Release V8 (dbGaP Accession phs000424.v8.p2) with default parameters.
3 Results
3.1 Study participants
The demographic information and genotype characteristics of the participants are listed in Table 1. The age (mean [ ± standard deviation]) of the participants was 70.2 ( ± 8.5) years. The proportions of female and APOE ε4 carriers were 58.4% and 44.6%, respectively. Among 1125 participants, 647 (57.5%) were Aβ (+) and 478 (42.5%) were Aβ (−).
3.2 SNP identification based on SCCA
The SNP data were filtered based on the p value obtained from the summary statistics of the IGAP (Lambert et al., 2013). Furthermore, linkage disequilibrium clumping was performed, resulting in the selection of 344 uncorrelated SNPs. The selected SNPs were used for subsequent analyses (Supplementary Table 1). The cortical thickness of 12 ROIs and cortical thickness after adjusting for covariates are displayed in Supplementary Figure 1. Both measures showed a significant difference between Aβ (+) and Aβ (−) groups, with p values < 0.001.
Next, we used SCCA to select genetic variants associated with cortical thickness. The SCCA has been trained for the Aβ (−) and Aβ (+) groups and evaluated in terms of canonical correlation coefficients. The canonical correlation coefficients of those selected variants were 0.55 and 0.45 in the Aβ (−) and Aβ (+) groups, respectively. For the Aβ (−) group, we identified rs6743470 located near the BIN1 gene on chromosome 2 (i.e., a canonical weight of −0.13), and rs141622900 located near the APOC1 gene on chromosome 19 (i.e., a canonical weight of −0.14) as associated with imaging features. For the Aβ (+) group, rs157580 located near the TOMM40 and rs7550917 and rs9270850, located near the HLA-DQA1 gene, have been identified as genetic variants associated with imaging features. Detailed canonical loading weights and identified SNPs are shown in Figure 2 and Table 2. Among the top 20 SNPs selected from the two groups, five (rs73281586, rs6859, rs35879138, rs157580, and rs141622900) showed marginal associations with CU-AD diagnosis in our cohort (p < 0.05, Supplementary Table 2). The SCCA was trained on the total samples, and the canonical correlation was 0.42. Detailed canonical loading weights and identified SNPs are shown in Supplementary Figure 2 and Supplementary Table 3.
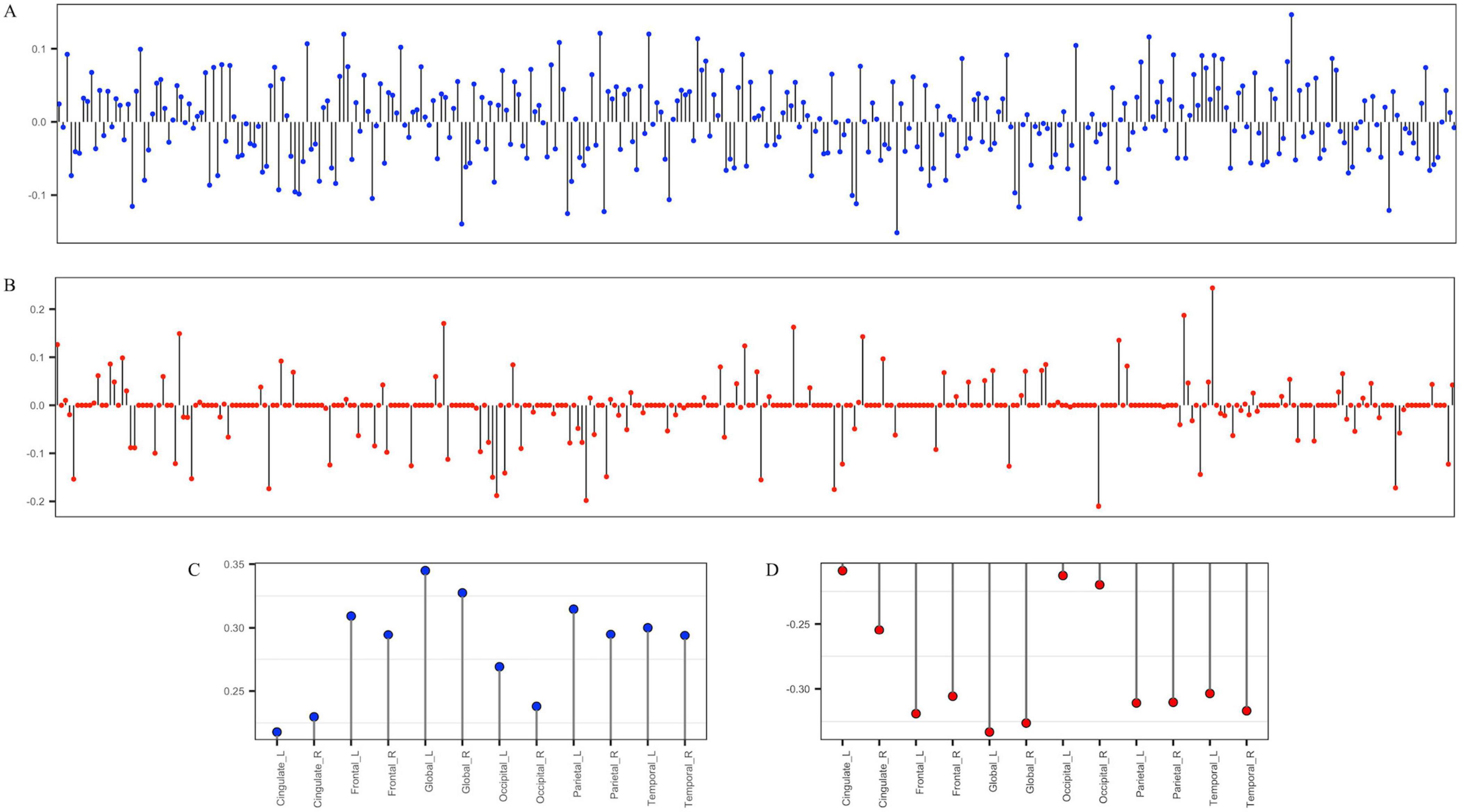
Figure 2. The stem plot of SNPs and regions of interest (ROIs) weights estimated by group-wise SCCA. (A,C) are weights of 344 SNPs and cortical thickness ROIs in the Aβ (–) group. (B,D) are the weights of 344 SNPs and cortical thickness ROIs in the Aβ (+) group. The y-axis represents the weights of variables, and the x-axis represents SNP indices (A,B) or the names of ROIs (C,D).
3.3 Prediction of AD biomarkers
To validate the efficiency of the SNPs, a model based on elastic net regression was constructed to predict AD biomarkers. First, we validated the efficiency of SNPs across different amyloid positivity groups (Aβ (+) and Aβ (−)). The prediction model employing the top 10 SNPs from SCCA within the Aβ (−) group showed high predictive performance for cortical thickness (r = 0.76) in Aβ (−) samples, whereas the low predictive performance of r = 0.18 in Aβ (+) samples. Similarly, the prediction model employing the top 10 SNPs from SCCA within the Aβ (+) group showed high predictive performance for cortical thickness (r = 0.50) in Aβ (+), whereas the low predictive performance of r = 0.36 in Aβ (−) (Table 3).
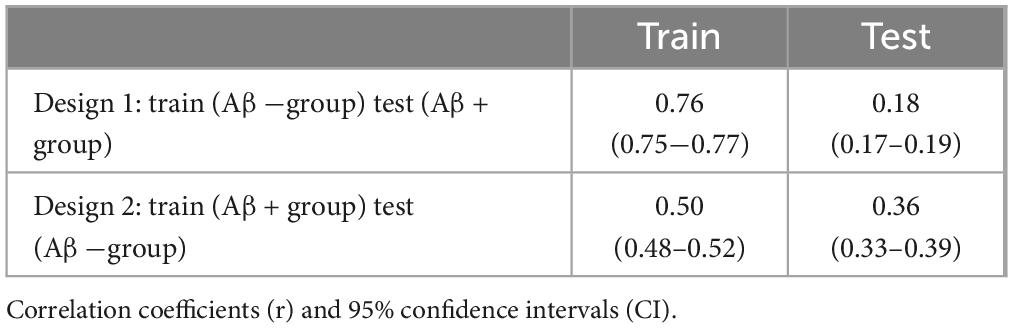
Table 3. Comparison of correlation coefficients (r) of predication models with top 10 SNPs for Aβ (+) and Aβ (−) groups.
Subsequently, we compared the prediction performances of the models trained with the identified SNPs from SCCA and randomly selected SNPs. The prediction model with the top 10 SNPs (model 2) from SCCA within Aβ (−) group showed highest predictive performance in Aβ (−) samples (cortical thickness r = 0.72, MMSE r = 0.57, CDR-SB r = 0.36), whereas the model with randomly selected SNPs (model 3) (cortical thickness r = 0.68, MMSE r = 0.56, CDR-SB r = 0.35) and model with age, sex, and APOE ε4, ICV (model 1) (cortical thickness r = 0.67, MMSE r = 0.54, CDR-SB r = 0.31) exhibit relatively lower performance. Similarly, the prediction model employing the top 10 SNPs from SCCA within Aβ (+) showed the highest predictive performance in Aβ (+) samples compared to models 1 and 3 (Table 4). When evaluating the predictive performance in the total samples using the top 10 SNPs from each Aβ positivity group, for a total of 20 SNPs, the correlation coefficients were as follows: cortical thickness r = 0.44, MMSE r = 0.40, CDR-SB r = 0.31. In addition, the prediction performance of models with selected SNPs from total samples were shown in Supplementary Table 4. The prediction model employing the top 10 selected SNPs showed highest predictive performance.
3.4 Pathway analysis
Pathway analyses were conducted using Enrichr, resulting in the enrichment of 134 and 106 gene sets in Aβ (−) and Aβ (+) groups, respectively. Table 5 presents the top 10 enriched gene sets in each group with a q value threshold of < 0.05. In the Aβ (−) group, the enriched gene sets included those associated with the regulation of synaptic transmission, postsynaptic potential, and dendrites. In the Aβ (+) group, enriched gene sets were related to MHC class protein binding and assembly. The results of gene set enrichment analysis for the selected SNPs in total samples were shown in Supplementary Table 5.
3.5 Mediation analysis
To assess the mediating effects of Aβ on the association between the identified SNPs and cortical atrophy, we performed a mediation analysis with Aβ positivity (Aβ (−) or Aβ (+)) as a mediator variable. Twenty SNPs (the top 10 SNPs in each amyloid positivity group) selected from SCCA were included in this analysis. Figure 3 shows that seven SNPs (rs2271920, rs1949100, rs9270850, rs35879138, rs157580, rs6859, and rs7550917) had significant direct or indirect effects on cortical thickness. Among these seven, rs9270850 selected from SCCA in the Aβ (+) group had significant indirect effects (Figure 3B).
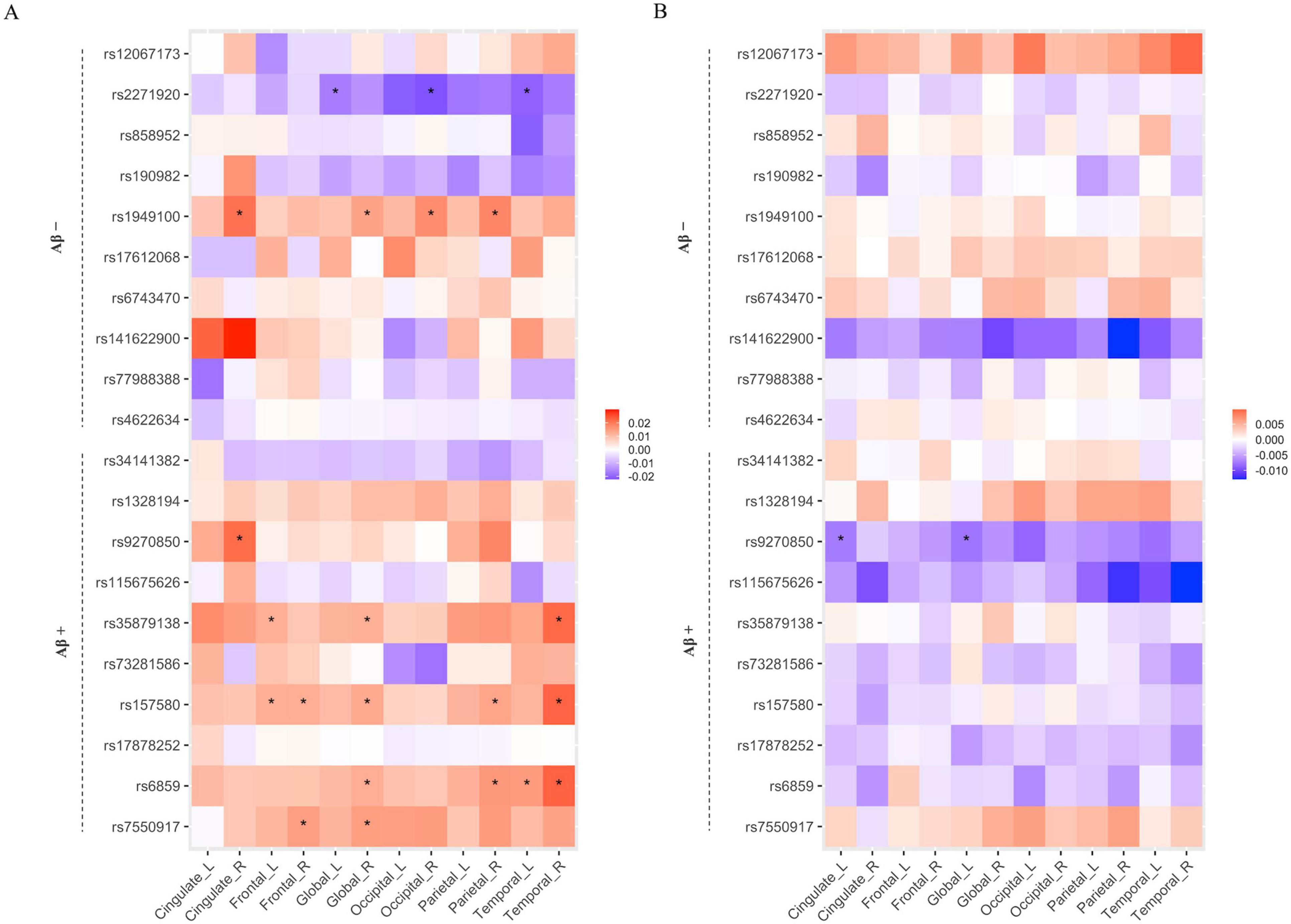
Figure 3. The results of mediation analysis for the relation of SNP with cortical thickness via Aβ positivity. The heatmaps of direct (A) and indirect (B) effects. The x-axis represents the name of ROIs, and the y-axis represents the selected SNPs from Aβ positive and negative groups. The associations with a p-value < 0.05 are indicated with an asterisk.
3.6 eQTL analysis
We investigated whether the seven identified SNPs have eQTLs in brain tissues and whole blood using data from the GTEx eQTL database. Three of the seven SNPs, rs2271920, rs6859, and rs9270850, significantly affected the regulation of gene expression. The eQTL plots of the four SNPs were downloaded from the GTEx portal (Figure 4). The rs2271920 on chromosome 8 significantly regulated the expression of CHRNA2 in the cerebellum (p value = 2.10 × 10–6). The rs9270850 significantly regulated HLA-DRB5 (p value = 1.80 × 10–18) and HLA-DRB1 (p value = 2.50 × 10–15) expressions in the brain cortex and rs6859 on chromosome 19 regulated the expression of NECTIN2 in whole blood (p value = 7.50 × 10–15).

Figure 4. The violin plots of the eQTL results. The x-axis indicated the genotype of SNPs, and y-axis indicated the normalized expression levels of genes. All eQTL plots are downloaded from the GTEx portal.
4 Discussion
In this study, we conducted an imaging genetic association analysis to identify the genetic biomarkers associated with cortical thickness. Our major findings are summarized as follows: First, through the implementation of the SCCA algorithm, we identified SNPs that demonstrated strong associations with cortical thickness. Second, our results indicate that the identified SNPs exhibit promising predictive performance for neurodegeneration and cognitive outcomes. This suggests their potential role as genetic markers associated with disease progression in AD. Third, our pathway enrichment analysis indicated that identified SNPs from the Aβ (−) and Aβ (+) groups were enriched for different gene sets. Additionally, one SNP identified in the Aβ (+) group exhibited associations with cortical thickness mediated by Aβ accumulation in the brain. These suggest that the genetic mechanisms that affect cortical atrophy may differ between the Aβ (−) and Aβ (+) groups. Overall, our findings highlighted the potential significance of the identified SNPs as novel genetic targets for understanding neurodegeneration in AD. These findings contribute to a growing body of research on genetic biomarkers associated with cortical thickness and their implications in AD pathology.
From the SCCA analysis, the global mean cortical thicknesses of the left and right hemispheres were the most prominent, followed by that of the frontal and parietal cortex. Global atrophy of the cortex, as well as regional atrophy, has been widely studied and demonstrated an abnormal reduction in patients with AD compared to cognitively normal control subjects (Braak and Braak, 1991; Lerch et al., 2005; Dickerson et al., 2009; Querbes et al., 2009). For the genetic data, we identified the top 10 SNPs for each Aβ (−) and Aβ (+) group. Twenty identified SNPs have been reported to be associated with the risk of clinically diagnosed AD in a European population (Lambert et al., 2013), but only five of them have a potential relationship with AD (p < 0.05). These results may be attributed to insufficient statistical power and ethnic differences. Recent genetic studies of AD have highlighted ethnic differences that may have affected our results.
We found that the identified SNPs improved the predictive performance for cortical thickness and cognitive function. Furthermore, we observed divergent patterns of these SNPs between the two groups. Specifically, the identified SNPs exhibited robust predictive capabilities for global cortical thickness in one group while facing challenges in achieving accurate predictions in the other group. These findings suggest that the genetic mechanisms affecting cortical atrophy may differ depending on amyloid accumulation. Our hypothesis was supported by the following observations: (1) The top 10 SNPs selected in each Aβ (−) and Aβ (+) group were not shared between groups. (2) The top 10 SNPs selected in one group showed poor predictive performance for cortical thickness and cognitive function in the other group. (3) These SNPs selected from the two groups were clustered into gene sets with different functional roles.
eQTL analysis revealed that these SNPs were significantly involved in the regulation of four genes (CHRNA2, NECTIN2, HLA-DRB5, and HLA-DRB1). Previous studies have reported that these genes are associated with AD pathophysiology. In particular, CHRNA2 (cholinergic receptor nicotinic alpha 2 subunit) encodes neuronal acetylcholine receptor subunit alpha-2 (nAChRα2). Nicotinic acetylcholine receptors (nAChRs) are ligand-gated ion channels that produce neuronal receptors widely found in the central nervous system and are involved in synaptic transmission. CHRNA2 is expressed in brain tissues and hippocampal CA1 region and previous studies have reported the effect of nAChRα2 on hippocampus-dependent learning and memory (Lotfipour et al., 2017) and plasticity of CA1 hippocampal synapses (Demontis et al., 2019). In addition, a variant of CHRNA2 has been reported to be relevant to Alzheimer’s disease in the Chinese population (Ding et al., 2023), and CHRNA2 is currently one of the targets of AD drug research (Xu et al., 2021). NECTIN2 (nectin cell adhesion molecule 2) is expressed in astrocytes and neurons in the brain and plays important roles in the homeostasis of astrocytes and neurons and the formation of synapses (Mizutani et al., 2022). In addition, previous genetic association studies in European, Japanese, and African Americans reported that variations in NECTIN2 are associated with AD (Harold et al., 2009; Takei et al., 2009; Logue et al., 2011), MCI to AD conversion in APOE ε4 non-carriers (Xiao et al., 2022), and cognitive trajectory (Rajendrakumar et al., 2024). Human leukocyte antigen (HLA) is a family of genes that encodes cell-surface proteins that play vital roles in immune system regulation (Shiina et al., 2009). The activities involved in immune responses, including infection, brain development, and plasticity, in AD pathogenesis may be determined by HLA genes (Wang et al., 2020). Moreover, the expression of HLA-DRB1 and HLA-DRB5 in microglia is positively correlated with measures of AD pathology (Mathys et al., 2019). Furthermore, many previous GWA and haplotype studies have suggested an association between variations in HLA genes and AD risk (Neill et al., 1999; Lambert et al., 2013; Mansouri et al., 2015; Zhang et al., 2022). HLA-DRB1 has protective effects in APOE ε4 carriers against AD susceptibility (Ding et al., 2023), and CpGs of the HLA-DRB5 gene are associated with AD pathological diagnosis (Yu et al., 2015). Although previous studies have reported associations between genes and the risk of AD, to date, no study has shown an association between the SNPs mapped to these genes and cortical thickness. Our findings can help achieve a better understanding of AD pathophysiology and uncover novel therapeutic targets for AD.
Pathway analysis revealed that genes identified in the Aβ (−) group were enriched for pathways related to dendrites, synaptic transmission, potassium ion transport, and Aβ formation. Meanwhile, genes identified in the Aβ (+) group were enriched for pathways related to the immune response, which are related to AD pathophysiology. Dendritic abnormalities in AD are widespread and occur in the early stages of the disease (Weigeldt, 1922; Cochran et al., 2014). Synaptic loss may lead to brain atrophy and the abnormalities in synaptic transmission and postsynaptic function are associated with cognitive decline (Ardiles et al., 2012; Subramanian et al., 2020; Tzioras et al., 2023). It is interesting that Aβ-related pathways were identified from SNPs identified in Aβ (−) group. This could be because subthreshold Aβ pathology is associated with worse pathological and clinical outcomes (Bischof and Jacobs, 2019). We also identified the potassium transport pathway. Potassium channels play an important physiological role in signaling mechanisms and are linked to the development of neurodegenerative diseases (Colom et al., 1998; Annunziato et al., 2002; Cordaro et al., 2022), and dysfunction of calcium-activated potassium ion channel activity is related to memory impairment (Trombetta-Lima et al., 2020). The peripheral and central immune systems are dysregulated in AD and are related to cognitive function and AD pathology (Bettcher et al., 2021; Wu et al., 2021). The pathological proteins of AD, such as Aβ peptides, can be swallowed by the microglia, which are presented to T lymphocytes after combination with particular HLA classes I and II. Furthermore, B lymphocytes secrete antibodies against Aβ peptides (Wang et al., 2020; Wu et al., 2021).
The strength of our study is the recruitment of participants using a standardized diagnostic protocol, including detailed neuropsychological tests, Aβ PET, and brain MRI. However, the present study had some limitations. We identified SNPs related to cortical thickness in AD using SCCA; however, the sample size was moderate. Although we used candidate SNPs to overcome the overfitting problem that leads to false-positive findings, replicating our findings in a larger independent dataset is still required. In addition, we used only a Korean population; further studies with racially diverse samples are needed to generalize our findings. Nevertheless, the fact that little research has been conducted on Asian populations makes the current study notable. In this study, we used candidate SNPs that showed higher associations with AD in the European population. However, these SNPs showed weaker associations in our study than in previous studies in the European population. Our findings highlight the importance of genetic association studies in diverse populations. In this study, we investigated the role of SNPs in neurodegeneration and Aβ neurological outcomes. However, because candidate SNPs were used, the genetic mechanisms of each pathway could not be fully elucidated.
In conclusion, using SCCA, we identified the SNPs that contributed to the prediction of cortical thickness in a Korean cohort. We investigated the involvement of these SNPs in AD by examining their association with cortical atrophy and Aβ-mediated cortical atrophy. AD is a complex multifactorial neurodegenerative disorder. Genetic association studies using AD biomarkers are needed to elucidate the complex genetic architecture of AD. Our findings contribute to our understanding of the genetic architecture underlying cortical atrophy and its relationship with AD.
Data availability statement
The original contributions presented in this study are publicly available. This data can be found here: https://www.ebi.ac.uk/eva/?eva-study=PRJEB72796.
Ethics statement
The studies involving humans were approved by the Institutional Review Board of the Samsung Medical Center approved this study. Written informed consent was obtained from all the participants. The studies were conducted in accordance with the local legislation and institutional requirements. The participants provided their written informed consent to participate in this study. Written informed consent was obtained from the individual(s) for the publication of any potentially identifiable images or data included in this article.
Author contributions
B-HK: Conceptualization, Formal analysis, Methodology, Software, Visualization, Writing – original draft. SS: Conceptualization, Supervision, Writing – review and editing. YP: Formal analysis, Methodology, Writing – original draft. JiK: Data curation, Formal analysis, Writing – original draft. HK: Data curation, Writing – review and editing. HJ: Data curation, Formal analysis, Writing – review and editing. JY: Data curation, Writing – review and editing. MK: Conceptualization, Supervision, Writing – review and editing. JPK: Data curation, Supervision, Writing – review and editing.
Funding
The authors declare that they received financial support for the research, authorship, and publication of this article. This work was partly supported by an Institute of Information and Communications Technology Planning and Evaluation (IITP) grant funded by the Korean government (MSIT) [No. 2019-0-01842, Artificial Intelligence Graduate School Program (GIST); No. RS-2021-II212068, Artificial Intelligence Innovation Hub] and the National Research Foundation of Korea under grant NRF-2022R1F1A1068529, Future Medicine 2030 Project of the Samsung Medical Center (#SMX1240011), National Research Foundation of Korea(NRF) grant funded by the Korea government (MSIT) (No. RS-2023-00247408), and Korea Dementia Research Project through the Korea Dementia Research Center (KDRC), funded by the Ministry of Health and Welfare and Ministry of Science and ICT, Republic of Korea (grant number: RS-2020-KH106434).
Conflict of interest
The authors declare that the research was conducted in the absence of any commercial or financial relationships that could be construed as a potential conflict of interest.
Publisher’s note
All claims expressed in this article are solely those of the authors and do not necessarily represent those of their affiliated organizations, or those of the publisher, the editors and the reviewers. Any product that may be evaluated in this article, or claim that may be made by its manufacturer, is not guaranteed or endorsed by the publisher.
Supplementary material
The Supplementary Material for this article can be found online at: https://www.frontiersin.org/articles/10.3389/fnins.2024.1428900/full#supplementary-material
Footnotes
References
Albert, M., DeKosky, S., Dickson, D., Dubois, B., Feldman, H., Fox, N., et al. (2011). The diagnosis of mild cognitive impairment due to Alzheimer’s disease: Recommendations from the national institute on aging-Alzheimer’s association workgroups on diagnostic guidelines for Alzheimer’s disease. Alzheimers Dement. 7, 270–279. doi: 10.1016/j.jalz.2011.03.008
Annunziato, L., Pannaccione, A., Cataldi, M., Secondo, A., Castaldo, P., Di Renzo, G., et al. (2002). Modulation of ion channels by reactive oxygen and nitrogen species: A pathophysiological role in brain aging? Neurobiol. Aging. 23, 819–834. doi: 10.1016/s0197-4580(02)00069-6
Ardiles, A., Tapia-Rojas, C., Mandal, M., Alexandre, F., Kirkwood, A., Inestrosa, N., et al. (2012). Postsynaptic dysfunction is associated with spatial and object recognition memory loss in a natural model of Alzheimer’s disease. Proc. Natl. Acad. Sci. U.S.A. 109, 13835–13840. doi: 10.1073/pnas.1201209109
Arslan, A. (2018). Imaging genetics of schizophrenia in the post-GWAS era. Prog. Neuropsychopharmacol. Biol. Psychiatry 80, 155–165. doi: 10.1016/j.pnpbp.2017.06.018
Bakken, T., Bloss, C., Roddey, J., Joyner, A., Rimol, L., Djurovic, S., et al. (2011). Association of genetic variants on 15q12 with cortical thickness and cognition in schizophrenia. Arch. Gen. Psychiatry 68, 781–790. doi: 10.1001/archgenpsychiatry.2011.81
Beaton, D., Kriegsman, M., Dunlop, J., Filbey, F. M., and Abdi, H. (2016). “Imaging genetics with partial least squares for mixed-data types (MiMoPLS),” in Springer proceedings in mathematics and statistics, eds H. Abdi, V. Esposito Vinzi, G. Russolillo, G. Saporta, and L. Trinchera (Cham: Springer), doi: 10.1007/978-3-319-40643-5_6
Bettcher, B., Tansey, M., Dorothée, G., and Heneka, M. (2021). Peripheral and central immune system crosstalk in Alzheimer disease - a research prospectus. Nat. Rev. Neurol. 17, 689–701. doi: 10.1038/s41582-021-00549-x
Bischof, G., and Jacobs, H. (2019). Subthreshold amyloid and its biological and clinical meaning: Long way ahead. Neurology 93, 72–79. doi: 10.1212/WNL.0000000000007747
Braak, H., and Braak, E. (1991). Neuropathological stageing of Alzheimer-related changes. Acta Neuropathol. 82, 239–259. doi: 10.1007/BF00308809
Brouwer, R., Klein, M., Grasby, K., Schnack, H., Jahanshad, N., Teeuw, J., et al. (2022). Genetic variants associated with longitudinal changes in brain structure across the lifespan. Nat. Neurosci. 25, 421–432. doi: 10.1038/s41593-022-01042-4
Busche, M., and Hyman, B. (2020). Synergy between amyloid-β and tau in Alzheimer’s disease. Nat. Neurosci. 23, 1183–1193. doi: 10.1038/s41593-020-0687-6
Chi, E., Allen, G., Zhou, H., Kohannim, O., Lange, K., and Thompson, P. M. (2013). IMAGING GENETICS VIA SPARSE CANONICAL CORRELATION ANALYSIS. Proc. IEEE Int. Symp. Biomed. Imaging 2013, 740–743. doi: 10.1109/ISBI.2013.6556581
Cho, S., Choe, Y., Kim, Y., Lee, B., Kim, H., Jang, H., et al. (2020). Concordance in detecting amyloid positivity between 18F-florbetaben and 18F-flutemetamol amyloid PET using quantitative and qualitative assessments. Sci. Rep. 10:19576. doi: 10.1038/s41598-020-76102-5
Chu, D., Liao, L., Ng, M., and Zhang, X. (2013). Sparse canonical correlation analysis: New formulation and algorithm. IEEE Trans. Pattern Anal. Mach. Intell. 35, 3050–3065. doi: 10.1109/TPAMI.2013.104
Cochran, J., Hall, A., and Roberson, E. D. (2014). The dendritic hypothesis for Alzheimer’s disease pathophysiology. Brain Res. Bull. 103, 18–28. doi: 10.1016/j.brainresbull.2013.12.004
Collins, D., Neelin, P., Peters, T., and Evans, A. (1994). Automatic 3D intersubject registration of MR volumetric data in standardized Talairach space. J. Comput. Assist. Tomogr. 18, 192–205.
Colom, L., Diaz, M., Beers, D., Neely, A., Xie, W., and Appel, S. (1998). Role of potassium channels in amyloid-induced cell death. J. Neurochem. 70, 1925–1934. doi: 10.1046/j.1471-4159.1998.70051925.x
Cordaro, M., Cuzzocrea, S., and Di Paola, R. (2022). Ion channels and neurodegenerative disease aging related. London: IntechOpen, doi: 10.5772/intechopen.103074
Das, S., Forer, L., Schönherr, S., Sidore, C., Locke, A., Kwong, A., et al. (2016). Next-generation genotype imputation service and methods. Nat. Genet. 48, 1284–1287. doi: 10.1038/ng.3656
Demontis, D., Rajagopal, V., Thorgeirsson, T., Als, T., Grove, J., Leppälä, K., et al. (2019). Genome-wide association study implicates CHRNA2 in cannabis use disorder. Nat. Neurosci. 22, 1066–1074. doi: 10.1038/s41593-019-0416-1
Dickerson, B., Bakkour, A., Salat, D., Feczko, E., Pacheco, J., Greve, D., et al. (2009). The cortical signature of Alzheimer’s disease: Regionally specific cortical thinning relates to symptom severity in very mild to mild AD dementia and is detectable in asymptomatic amyloid-positive individuals. Cereb. Cortex 19, 497–510. doi: 10.1093/cercor/bhn113
Ding, Y., Chen, H., Yan, Y., Qiu, Y., Zhao, A., Li, B., et al. (2023). Relationship between FERMT2, CELF1, COPI, CHRNA2, and ABCA7 genetic polymorphisms and Alzheimer’s disease risk in the southern Chinese population. J. Alzheimers Dis. Rep. 7, 1247–1257. doi: 10.3233/ADR-230072
Du, A., Schuff, N., Kramer, J., Rosen, H., Gorno-Tempini, M., Rankin, K., et al. (2007). Different regional patterns of cortical thinning in Alzheimer’s disease and frontotemporal dementia. Brain 130, 1159–1166. doi: 10.1093/brain/awm016
Du, L., Huang, H., Yan, J., Kim, S., Risacher, S., Inlow, M., et al. (2016). Structured sparse CCA for brain imaging genetics via graph OSCAR. BMC Syst. Biol. 10:68. doi: 10.1186/s12918-016-0312-1
Fang, J., Lin, D., Schulz, S., Xu, Z., Calhoun, V., and Wang, Y. (2016). Joint sparse canonical correlation analysis for detecting differential imaging genetics modules. Bioinformatics 32, 3480–3488. doi: 10.1093/bioinformatics/btw485
Goate, A., Chartier-Harlin, M., Mullan, M., Brown, J., Crawford, F., Fidani, L., et al. (1991). Segregation of a missense mutation in the amyloid precursor protein gene with familial Alzheimer’s disease. Nature 349, 704–706. doi: 10.1038/349704a0
Hampel, H., Hardy, J., Blennow, K., Chen, C., Perry, G., Kim, S., et al. (2021). The amyloid-β pathway in Alzheimer’s disease. Mol. Psychiatry 26, 5481–5503. doi: 10.1038/s41380-021-01249-0
Harold, D., Abraham, R., Hollingworth, P., Sims, R., Gerrish, A., Hamshere, M., et al. (2009). Genome-wide association study identifies variants at CLU and PICALM associated with Alzheimer’s disease. Nat. Genet. 41, 1088–1093. doi: 10.1038/ng.440
He, Z., Guo, J., McBride, J., Narasimhan, S., Kim, H., Changolkar, L., et al. (2018). Amyloid-β plaques enhance Alzheimer’s brain tau-seeded pathologies by facilitating neuritic plaque tau aggregation. Nat. Med. 24, 29–38. doi: 10.1038/nm.4443
Hittner, J., May, K., and Silver, N. C. A. (2003). Monte Carlo evaluation of tests for comparing dependent correlations. J. Gen. Psychol. 130, 149–168. doi: 10.1080/00221300309601282
Hotelling, H. (1992). “Relations between two sets of variates,” in Breakthroughs in statistics: Methodology and distribution, eds S. Kotz and N. L. Johnson (New York, NY: Springer), 162–190.
Jack, C., Bennett, D., Blennow, K., Carrillo, M., Dunn, B., Haeberlein, S., et al. (2018). NIA-AA Research Framework: Toward a biological definition of Alzheimer’s disease. Alzheimers Dement. 14, 535–562. doi: 10.1016/j.jalz.2018.02.018
Jacobs, G. R., and Voineskos, A. N. (2020). “Genetics and neuroimaging in schizophrenia,” in Neuroimaging in schizophrenia, eds M. Kubicki and M. Shenton (Cham: Springer), doi: 10.1007/978-3-030-35206-6_16
Jang, H., Jang, Y., Kim, H., Werring, D., Lee, J., Choe, Y., et al. (2019). Clinical significance of amyloid β positivity in patients with probable cerebral amyloid angiopathy markers. Eur. J. Nucl. Med. Mol. Imaging 46, 1287–1298. doi: 10.1007/s00259-019-04314-7
June, S., Singh, V., Lee, J., Lerch, J., Ad-Dab’bagh, Y., MacDonald, D., et al. (2005). Automated 3-D extraction and evaluation of the inner and outer cortical surfaces using a Laplacian map and partial volume effect classification. Neuroimage 27, 210–221. doi: 10.1016/j.neuroimage.2005.03.036
Kang, S., Park, Y., Shin, J., Kim, H., Yun, J., Jang, H., et al. (2022). Cortical neuroanatomical changes related to specific language impairments in primary progressive aphasia. Front. Aging Neurosci. 14:878758. doi: 10.3389/fnagi.2022.878758
Karran, E., Mercken, M., and De Strooper, B. (2011). The amyloid cascade hypothesis for Alzheimer’s disease: An appraisal for the development of therapeutics. Nat. Rev. Drug Discov. 10, 698–712. doi: 10.1038/nrd3505
Kim, B., Choi, Y., Yang, J., Kim, S., Nho, K., Lee, J., et al. (2020). Identification of novel genes associated with cortical thickness in Alzheimer’s disease: Systems biology approach to neuroimaging endophenotype. J. Alzheimers Dis. 75, 531–545. doi: 10.3233/JAD-191175
Kim, H., Jung, S., Kim, J., Jang, H., Kang, S., Hwangbo, S., et al. (2021). Identifying novel genetic variants for brain amyloid deposition: A genome-wide association study in the Korean population. Alzheimers Res. Ther. 13:117. doi: 10.1186/s13195-021-00854-z
Kim, M., Kim, J., Lee, S., and Park, H. (2017). Imaging genetics approach to Parkinson’s disease and its correlation with clinical score. Sci. Rep. 7:46700. doi: 10.1038/srep46700
Kim, M., Min, E., Liu, K., Yan, J., Saykin, A., Moore, J., et al. (2022). Multi-task learning based structured sparse canonical correlation analysis for brain imaging genetics. Med. Image Anal. 76:102297. doi: 10.1016/j.media.2021.102297
Kong, W., Xu, Y., Wang, S., Wei, K., Wen, G., Yu, Y., et al. (2023). A novel longitudinal phenotype-genotype association study based on deep feature extraction and hypergraph models for Alzheimer’s disease. Biomolecules 13:728. doi: 10.3390/biom13050728
Lambert, J., Ibrahim-Verbaas, C., Harold, D., Naj, A., Sims, R., Bellenguez, C., et al. (2013). Meta-analysis of 74,046 individuals identifies 11 new susceptibility loci for Alzheimer’s disease. Nat. Genet. 45, 1452–1458. doi: 10.1038/ng.2802
Lerch, J., and Evans, A. (2005). Cortical thickness analysis examined through power analysis and a population simulation. Neuroimage 24, 163–173. doi: 10.1016/j.neuroimage.2004.07.045
Lerch, J., Pruessner, J., Zijdenbos, A., Hampel, H., Teipel, S., and Evans, A. (2005). Focal decline of cortical thickness in Alzheimer’s disease identified by computational neuroanatomy. Cereb. Cortex 15, 995–1001. doi: 10.1093/cercor/bhh200
Liu, K., Yao, X., Yan, J., Chasioti, D., Risacher, S., Nho, K., et al. (2017). Transcriptome-guided imaging genetic analysis via a novel sparse CCA algorithm. Graphs. Biomed. Image Anal. Comput. Anat. Imaging Genet. 10551, 220–229. doi: 10.1007/978-3-319-67675-3_20
Logue, M., Schu, M., Vardarajan, B., Buros, J., Green, R., Go, R., et al. (2011). A comprehensive genetic association study of Alzheimer disease in African Americans. Arch. Neurol. 68, 1569–1579. doi: 10.1001/archneurol.2011.646
Lorenzi, M., Gutman, B., Hibar, D. P., Altmann, A., Jahanshad, N., Thompson, P. M., et al. (2016). “Partial least squares modelling for imaging-genetics in Alzheimer’s disease: Plausibility and generalization,” in Proceedings - international symposium on biomedical imaging, (Prague), doi: 10.1109/ISBI.2016.7493396
Lotfipour, S., Mojica, C., Nakauchi, S., Lipovsek, M., Silverstein, S., Cushman, J., et al. (2017). α2* Nicotinic acetylcholine receptors influence hippocampus-dependent learning and memory in adolescent mice. Learn. Mem. 24, 231–244. doi: 10.1101/lm.045369.117
MacDonald, D., Kabani, N., Avis, D., and Evans, A. (2000). Automated 3-D extraction of inner and outer surfaces of cerebral cortex from MRI. Neuroimage 12, 340–356. doi: 10.1006/nimg.1999.0534
Mansouri, L., Messalmani, M., Klai, S., Bedoui, I., Derbali, H., Gritli, N., et al. (2015). Association of HLA-DR/DQ polymorphism with Alzheimer’s disease. Am. J. Med. Sci. 349, 334–337. doi: 10.1097/MAJ.0000000000000416
Mathys, H., Davila-Velderrain, J., Peng, Z., Gao, F., Mohammadi, S., Young, J., et al. (2019). Single-cell transcriptomic analysis of Alzheimer’s disease. Nature 570, 332–337. doi: 10.1038/s41586-019-1195-2
McKhann, G., Knopman, D., Chertkow, H., Hyman, B., Jack, C., Kawas, C., et al. (2011). The diagnosis of dementia due to Alzheimer’s disease: Recommendations from the National Institute on Aging-Alzheimer’s Association workgroups on diagnostic guidelines for Alzheimer’s disease. Alzheimers Dement. 7, 263–269. doi: 10.1016/j.jalz.2011.03.005
Mizutani, K., Miyata, M., Shiotani, H., Kameyama, T., and Takai, Y. (2022). Nectin-2 in general and in the brain. Mol. Cell Biochem. 477, 167–180. doi: 10.1007/s11010-021-04241-y
Neill, D., Curran, M., Middleton, D., Mawhinney, H., Edwardson, J., McKeith, I., et al. (1999). Risk for Alzheimer’s disease in older late-onset cases is associated with HLA-DRB1*03. Neurosci. Lett. 275, 137–140. doi: 10.1016/s0304-3940(99)00761-2
Parkhomenko, E., Tritchler, D., and Beyene, J. (2009). Sparse canonical correlation analysis with application to genomic data integration. Stat. Appl. Genet. Mol. Biol. 8:1. doi: 10.2202/1544-6115.1406
Pearlson, G., Liu, J., and Calhoun, V. (2015). An introductory review of parallel independent component analysis (p-ICA) and a guide to applying p-ICA to genetic data and imaging phenotypes to identify disease-associated biological pathways and systems in common complex disorders. Front. Genet. 6:276. doi: 10.3389/fgene.2015.00276
Pini, L., Pievani, M., Bocchetta, M., Altomare, D., Bosco, P., Cavedo, E., et al. (2016). Brain atrophy in Alzheimer’s disease and aging. Ageing Res. Rev. 30, 25–48. doi: 10.1016/j.arr.2016.01.002
Purcell, S., Neale, B., Todd-Brown, K., Thomas, L., Ferreira, M., Bender, D., et al. (2007). PLINK: A tool set for whole-genome association and population-based linkage analyses. Am. J. Hum. Genet. 81, 559–575. doi: 10.1086/519795
Querbes, O., Aubry, F., Pariente, J., Lotterie, J., Démonet, J., Duret, V., et al. (2009). Early diagnosis of Alzheimer’s disease using cortical thickness: Impact of cognitive reserve. Brain 132, 2036–2047. doi: 10.1093/brain/awp105
Rajendrakumar, A., Arbeev, K., Bagley, O., Yashin, A., and Ukraintseva, S. (2024). The S rs6859 in NECTIN2 gene is associated with underlying heterogeneous trajectories of cognitive changes in older adults. BMC Neurol. 24:78. doi: 10.1186/s12883-024-03577-4
Selkoe, D. J. (1991). The molecular pathology of Alzheimer’s disease. Neuron 22, 11–22. doi: 10.1016/0896-6273(91)90052-2
Serrano-Pozo, A., Frosch, M., Masliah, E., and Hyman, B. (2011). Neuropathological alterations in Alzheimer disease. Cold Spring Harb. Perspect. Med. 1:a006189. doi: 10.1101/cshperspect.a006189
Shiina, T., Hosomichi, K., Inoko, H., and Kulski, J. (2009). The HLA genomic loci map: Expression, interaction, diversity and disease. J. Hum. Genet. 54, 15–39. doi: 10.1038/jhg.2008.5
Sim, A., Tsagkrasoulis, D., and Montana, G. (2013). Random forests on distance matrices for imaging genetics studies. Stat. Appl. Genet. Mol. Biol. 12, 757–786. doi: 10.1515/sagmb-2013-0040
Sled, J., Zijdenbos, A., and Evans, A. C. (1998). A nonparametric method for automatic correction of intensity nonuniformity in MRI data. IEEE Trans. Med. Imaging 17, 87–97. doi: 10.1109/42.668698
Subramanian, J., Savage, J., and Tremblay, M. È (2020). Synaptic loss in Alzheimer’s disease: Mechanistic insights provided by two-photon in vivo imaging of transgenic mouse models. Front. Cell. Neurosci. 14:592607. doi: 10.3389/fncel.2020.592607
Takei, N., Miyashita, A., Tsukie, T., Arai, H., Asada, T., Imagawa, M., et al. (2009). Genetic association study on in and around the APOE in late-onset Alzheimer disease in Japanese. Genomics 93, 441–448. doi: 10.1016/j.ygeno.2009.01.003
Trombetta-Lima, M., Krabbendam, I., and Dolga, A. (2020). Calcium-activated potassium channels: Implications for aging and age-related neurodegeneration. Int. J. Biochem. Cell Biol. 123:105748. doi: 10.1016/j.biocel.2020.105748
Tzioras, M., McGeachan, R., Durrant, C., and Spires-Jones, T. (2023). Synaptic degeneration in Alzheimer disease. Nat. Rev. Neurol. 19, 19–38. doi: 10.1038/s41582-022-00749-z
Waaijenborg, S., Verselewel de Witt Hamer, P. C., and Zwinderman, A. H. (2008). Quantifying the association between gene expressions and DNA-markers by penalized canonical correlation analysis. Stat. Appl. Genet. Mol. Biol. 7:3. doi: 10.2202/1544-6115.1329
Wang, Z., Wan, Q., and Xing, A. (2020). HLA in Alzheimer’s disease: Genetic association and possible pathogenic roles. Neuromol. Med. 22, 464–473. doi: 10.1007/s12017-020-08612-4
Weigeldt (1922). Monatsschrift für psychiatrie und neurologie. Dtsch. Z. Nervenheilkd. 75:316. doi: 10.1007/bf02549979
Witten, D., and Tibshirani, R. (2009). Extensions of sparse canonical correlation analysis with applications to genomic data. Stat. Appl. Genet. Mol. Biol. 8:28. doi: 10.2202/1544-6115.1470
Wolthusen, R., Hass, J., Walton, E., Turner, J., Rössner, V., Sponheim, S., et al. (2015). Genetic underpinnings of left superior temporal gyrus thickness in patients with schizophrenia. World J. Biol. Psychiatry 7, 1–11.
Wu, K., Zhang, Y., Huang, Y., Dong, Q., Tan, L., and Yu, J. (2021). The role of the immune system in Alzheimer’s disease. Ageing Res. Rev. 70:101409. doi: 10.1016/j.arr.2021.101409
Xiao, Q., Xi, J., Wang, R., Zhao, Q., Liang, X., Wu, W., et al. (2022). the relationship between low-density lipoprotein cholesterol and progression of mild cognitive impairment: The influence of rs6859 in PVRL2. Front. Genet. 13:823406. doi: 10.3389/fgene.2022.823406
Xie, Z., Bailey, A., Kuleshov, M., Clarke, D., Evangelista, J., Jenkins, S., et al. (2021). gene set knowledge discovery with Enrichr. Curr. Protoc. 1:e90. doi: 10.1002/cpz1.90
Xu, Y., Kong, J., and Hu, P. (2021). Computational drug repurposing for alzheimer’s disease using risk genes from GWAS and single-Cell RNA sequencing studies. Front. Pharmacol. 12:617537. doi: 10.3389/fphar.2021.617537
Yu, L., Chibnik, L., Srivastava, G., Pochet, N., Yang, J., Xu, J., et al. (2015). Association of brain DNA methylation in SORL1, ABCA7, HLA-DRB5, SLC24A4, and BIN1 with pathological diagnosis of Alzheimer disease. JAMA Neurol. 72, 15–24. doi: 10.1001/jamaneurol.2014.3049
Zhang, X., Zou, M., Wu, Y., Jiang, D., Wu, T., Zhao, Y., et al. (2022). Regulation of the late onset Alzheimer’s disease associated HLA-DQA1/DRB1 expression. Am. J. Alzheimers Dis. Other Dement. 37:15333175221085066. doi: 10.1177/15333175221085066
Keywords: Alzheimer’s disease, sparse canonical correlation analysis, genetics, cortical thickness, amyloid beta (Ab), single nucleotide polymorphism (SNP)
Citation: Kim B-H, Seo SW, Park YH, Kim J, Kim HJ, Jang H, Yun J, Kim M and Kim JP (2024) Clinical application of sparse canonical correlation analysis to detect genetic associations with cortical thickness in Alzheimer’s disease. Front. Neurosci. 18:1428900. doi: 10.3389/fnins.2024.1428900
Received: 07 May 2024; Accepted: 19 August 2024;
Published: 24 September 2024.
Edited by:
Małgorzata Kujawska, Poznan University of Medical Sciences, PolandReviewed by:
Dmitry Prokopenko, Harvard Medical School, United StatesJiaxing Wang, University of Tennessee Health Science Center (UTHSC), United States
Copyright © 2024 Kim, Seo, Park, Kim, Kim, Jang, Yun, Kim and Kim. This is an open-access article distributed under the terms of the Creative Commons Attribution License (CC BY). The use, distribution or reproduction in other forums is permitted, provided the original author(s) and the copyright owner(s) are credited and that the original publication in this journal is cited, in accordance with accepted academic practice. No use, distribution or reproduction is permitted which does not comply with these terms.
*Correspondence: Mansu Kim, bWFuc3Uua2ltQGdpc3QuYWMua3I=; Jun Pyo Kim, anVucHlvMDcwMy5raW1Ac2Ftc3VuZy5jb20=