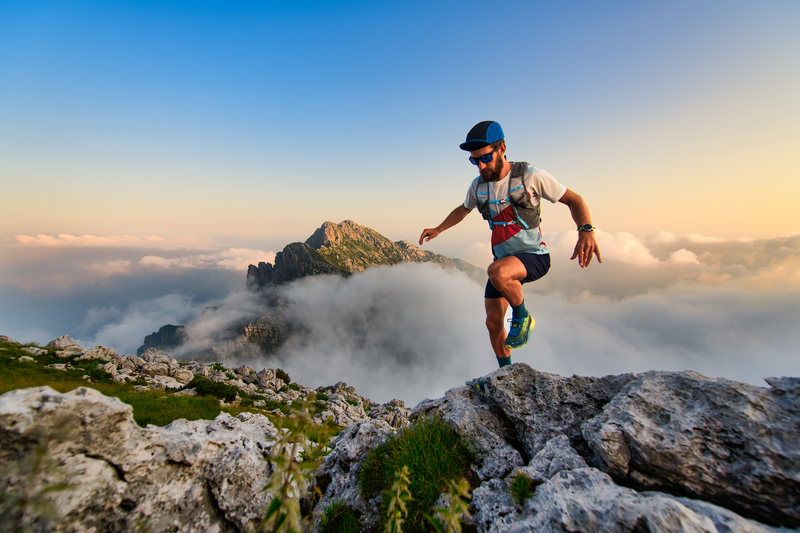
95% of researchers rate our articles as excellent or good
Learn more about the work of our research integrity team to safeguard the quality of each article we publish.
Find out more
OPINION article
Front. Neurosci. , 01 November 2023
Sec. Brain Imaging Methods
Volume 17 - 2023 | https://doi.org/10.3389/fnins.2023.1302069
During the first years of life, the human brain undergoes rapid structural and functional development, laying the foundation for cognitive behavior's progression throughout the individual's life. The structural covariance network (SCN), constructed based on varying cortical morphological features extracted from structural MRI, reflects the coordinated brain maturation between cortical regions (Alexander-Bloch et al., 2013; Gilmore et al., 2018). It is associated with white matter networks, functional networks, cognitive functions, and genetic heritability, making it valuable for early brain development studies (Chen et al., 2011; Gong et al., 2012; Alexander-Bloch et al., 2013; Seidlitz et al., 2018; Fenchel et al., 2020). Regarding the construction of SCN, the most representative method is to estimate the similarity between cortical regions by calculating the correlation of their morphological features [such as cortical thickness (CT), surface area (SA), gray matter volume (GMV), sulcal depth (SD), etc.] at the population level (He et al., 2007; Alexander-Bloch et al., 2013; Gilmore et al., 2018), which has been widely applied in adult and adolescent populations. In recent years, some studies have proposed SCNs constructed at the individual level (Kong et al., 2015; Meng et al., 2015; Li et al., 2017, 2021; Seidlitz et al., 2018; Yu et al., 2018; Sebenius et al., 2023), which can capture individual difference information. However, current studies of early brain development based on cortical morphology mainly focus on statistical analysis of morphological features, while exploration of the SCN is relatively limited, especially at the individual level. The neuroimaging research for early brain development based on SCN is still in its infancy, more work needs to be done to better explore the neural mechanisms of brain development.
This opinion paper aims to comprehensively analyze the relevant literature on early brain development from the perspectives of cortical morphological indicators and SCNs during both the fetal period and the first 5 years of life. We will delineate the research significance of SCNs in the context of early brain development, while also addressing the existing challenges and outlining potential directions for future research. It is expected that these analyses will shed light on the field of early brain development based on morphological SCN.
The fetal period is a crucial stage in early brain development, marked by the prolific formation of dendrites and synapses within the cortical plate and significant transformations in cerebral cortical morphology (Dubois et al., 2007; Cao et al., 2017). During this phase, the cerebral cortex rapidly develops from an initial smooth structure without gyri and sulci into an extremely complex folded structure (Dubois et al., 2007). With the formation of sulci-gyri, the complexity of the cerebral cortex also increases. This cerebral developmental process unfolds progressively, with primary cortical folding emerging around 20 gestational weeks, followed by the initiation of secondary cortical folding at approximately 32 gestational weeks (Feess-Higgins and Larroche, 1987; Dubois et al., 2019). It has been found that the total brain tissue volume undergoes a nearly 3-fold linear augmentation from 29 to 41 gestational weeks, among which, GMV demonstrates an almost 4-fold increase (Hüppi et al., 1998; Anderson et al., 2015). Concurrently, SA undergoes a substantial expansion, expanding nearly 4-fold between 27 and 40 gestational weeks (Dubois et al., 2019), with the lateral surface generally showing faster growth rates than the ventral and medial surfaces. Furthermore, the developmental pace of SA manifests heterogeneity among distinct cortical regions. Notably, SA of most primary cortices develops faster than the high-order association cortices, such as sensorimotor areas and auditory areas, showing a weekly average increment of more than 25% during 26–29 gestational weeks (Xia et al., 2019).
Currently, research on fetal development using the cortical morphology-based SCN remains relatively understudied. It has been shown that the SCN exhibits dynamic topological reorganization during this period. Notably, due to the challenges associated with obtaining high-quality fetal brain images, premature infants are often utilized as a means to simulate early brain development in the prenatal stage. A study involving premature infants that established the SCN based on SD demonstrated that in premature infants without or with slight injuries, the global efficiency of SCN increases gradually with age during 26.7–43 gestational weeks (age at scan), whereas the characteristic path length decreases with age (Kim et al., 2020). By 40 gestational weeks, premature infants without or with slight injuries exhibited no significant differences in SD and network connection strength compared to full-term infants. These findings suggest that the information integration capacity of the brain network is continuously enhancing during the third trimester.
The first 5 years of life is an important period for early postnatal development. At full-term birth, the cortical folding pattern of neonatal brain largely resembles that of adults (Duan et al., 2017b, 2019), and exhibits sex differences, hemispheric asymmetry, and individual differences (Hill et al., 2010; Li et al., 2014a; Duan et al., 2019, 2020). Notably, 0–2 years old is the most dynamic stage of postnatal brain development. Specifically, the brain volume expands rapidly and new tertiary cortical folds appear (Feess-Higgins and Larroche, 1987; Gilmore et al., 2007; Dubois et al., 2019). Various cortical morphological features, e.g., GMV, CT, SA, SD, folding index, and multiscale decompositions of cortical curvature, exhibit dramatic developmental changes during this period. The cortical development rate for most of these features is substantially higher during the first year than in the second year, with growth rates slowing down after 2 years of age (Li et al., 2014b, 2015; Lyall et al., 2015; Duan et al., 2017a, 2020). Specifically, during 0–1 years old, infant GMV increases about 108–149%; during 1–2 years old, GMV increases by about 14–19%; after 2 years old, GMV undergoes minor growth throughout childhood and exhibits a decline during adolescence (Knickmeyer et al., 2008; Groeschel et al., 2010; Gilmore et al., 2011, 2018). Regarding CT of infant brain, there is an average increase of 31% during 0–1 years old, followed by a growth of 4.3% during 1–2 years old, ultimately reaching 97% of adult thickness by 2 years old (Li et al., 2015; Lyall et al., 2015; Gilmore et al., 2018). It is noteworthy that CT peaks at around 14 months, and subsequently undergoes a gradual thinning process during adolescence (Lyall et al., 2015; Wang et al., 2019).
Currently, research on SCN-based early brain development patterns of children across the first 5 years of life is still in a nascent stage. Studies have found that in full-term neonates, SCN already exhibits the small-world topology and ordered modular organization (Fan et al., 2011; Meng et al., 2015). During 0–2 years old, the global efficiency, local efficiency, clustering coefficient, and modularity index of SCN constructed based on CT increases with age, and the shortest path length decreases with age (Fan et al., 2011; Meng et al., 2015), which suggest that both network segregation and integration enhances with age. However, a study on infants aged 0–2 years old found that in SCNs constructed based on CT, the global efficiency increases while the local efficiency decreases with age; in SCNs constructed based on curvedness, the global efficiency decreases while the local efficiency increases with age (Nie et al., 2014). Another study on 3–5-year old infants showed that the global efficiency of SCN constructed based on CT and curvedness decreases to varying degrees, while local efficiency increases to varying degrees (Nie et al., 2013). The inconsistencies among these findings may arise from variations in the morphological features and analysis methodologies used for network construction. In addition, a cross-sectional study of infants at 37–44 gestational weeks found age-related associations in the average structural connectivity strength (SCS) of network nodes. Among them, the SCS of sensorimotor cortex, auditory cortex, and visual cortex increases significantly with age (Fenchel et al., 2020; Galdi et al., 2020), while the SCS of cingulate gyrus and limbic cortex declines with age. These findings collectively indicate that after birth, the information integration and transmission capacity of the infant brain networks are significantly enhanced, leading to a more efficient organization.
Morphological SCNs provide valuable insights into the complex organization of the human brain. These networks reveal organized co-variation of cortical regions' morphological properties, to some extent resembling white matter and functional networks, and have been reported to be associated with specific cognitive and behavioral functions (Chen et al., 2011; Gong et al., 2012; Alexander-Bloch et al., 2013; Seidlitz et al., 2018; Fenchel et al., 2020). In addition, SCNs exhibit genetic heritability and undergo changes across the lifespan, with structure alterations observed in neuropsychiatric disorders, such as Alzheimer's disease and schizophrenia (He et al., 2008; Seeley et al., 2009; Yao et al., 2010). Individual-level morphological networks offer a novel perspective on understanding brain development and neuropsychiatric conditions (Cai et al., 2023). The individual SCNs assess inter-regional morphological similarity in single subjects mainly by estimating the similarity/divergence of regional feature distributions (Li et al., 2021; Sebenius et al., 2023) or by evaluating the correlation of regional feature vectors (Li et al., 2017; Seidlitz et al., 2018). This approach enables the investigation of individual topological brain changes in both healthy and diseased states. The relationship between morphological similarity and gene co-expression enriches our understanding of brain organization. Consequently, SCNs hold substantial neuroscientific significance in studying early brain development, contributing to the comprehension of cognitive development and risk for neuropsychiatric disorders in early childhood.
Despite pioneer studies on early brain development from the perspective of SCN, there are still many challenges to overcome. Notably, due to the considerable challenges in image acquisition and processing for fetal and infant populations, the corresponding datasets are scarce compared with adults, which also leads to the current research remaining in a nascent stage. Besides, most present studies are based on group-level SCN, which could not describe the individual information for further deep analysis of individual development. Compounding this, the development patterns from diverse studies are not very consistent, attributable to the heterogeneity in SCN construction methodologies and distinct morphological features employed (Alexander-Bloch et al., 2013; Nie et al., 2014). Furthermore, the relationship between cortical morphological development and cognitive function development is still unknown. More work needs to be done to further understand the early brain development.
Morphological SCNs hold great potential for exploring the neural mechanism of early brain development as well as neurodevelopment disorders. Many directions in the future require more attention. For example, (1) Propose better segmentation and registration methods to accurately analyze the fetal images with low SNR to construct SCNs to reveal the network properties, such as small-worldness, modularity, and hub distributions, during the fetal stage. (2) Appropriately combine the worldwide human brain development datasets, such as the Developing Human Connectome Project (dHCP, http://www.developingconnectome.org/project/), Baby Connectome Project (BCP, https://babyconnectomeproject.org/), Adolescent Brain Cognitive Development (ABCD, https://abcdstudy.org/) study, and upcoming datasets from the China Brain Project, to comprehensively explore the brain development patterns over a wide range of age durations. (3) Evaluate the influences of various factors and analysis methodologies in network construction, and explore reliable early brain developmental patterns. (4) Apply new individual-level SCN methods (Li et al., 2021; Sebenius et al., 2023) to early brain development studies that estimate the structural connectivity between cortical regions based on the similarity of feature distributions, thereby further analyzing the individual brain development of brain maturation. (5) Explore the relationships of developmental patterns among SCNs, functional networks, and white matter connectivity networks, as well as the relationships between the development of individual SCNs and cognitive functions. These studies will benefit the understanding of the neural mechanism of early brain development in both healthy individuals and individuals with neurodevelopment disorders; and combined with the brain-computer interface (BCI) control technology, which may contribute to the evaluation and rehabilitation of children with conditions such as developmental delays and movement disorders.
In conclusion, this study reviewed the recent literature on early brain development based on morphological SCNs. Specifically, the morphology of cerebral cortex develops dramatically during the first few years of life, with various morphological features, e.g., GMV, CT, and SA, significantly multiplying in this period. In addition, the SCN of full-term newborns exhibits small-world topology and ordered modular organization, showing dynamic changes in network separation and integration, suggesting that the brain is moving toward an organization with higher information integration and transmission capacity during early brain development. However, this field is still in a nascent stage, more work needs to be done. Our opinion is that it is necessary to apply new individual-level SCNs instead of traditional group-level SCNs and utilize databases spanning multiple age groups, to facilitate extensive analyses on a large sample level to explore early brain development patterns and individual differences; to reveal the relationship between developmental patterns among SCN, functional networks, and white matter connectivity networks; and to investigate the relationship between individual morphological development and cognition development. These studies based on SCN will deepen our understanding of the neural mechanism underlying early brain development as well as neurodevelopment disorders. This will contribute to optimizing the design of effective BCI systems, which could ultimately provide substantial help for children with neurodevelopmental disorders, e.g., developmental delays and movement disorders, to restart normal life in the near future.
DD: Writing—original draft, Writing—review & editing. DW: Writing—review & editing, Supervision.
The author(s) declare financial support was received for the research, authorship, and/or publication of this article. This work was supported by the National Natural Science Foundation of China (82001908 and 62276022) and the China Postdoctoral Science Foundation (2021T140065 and 2020M680419).
The authors declare that the research was conducted in the absence of any commercial or financial relationships that could be construed as a potential conflict of interest.
All claims expressed in this article are solely those of the authors and do not necessarily represent those of their affiliated organizations, or those of the publisher, the editors and the reviewers. Any product that may be evaluated in this article, or claim that may be made by its manufacturer, is not guaranteed or endorsed by the publisher.
Alexander-Bloch, A., Giedd, J. N., and Bullmore, E. (2013). Imaging structural co-variance between human brain regions. Nat. Rev. Neurosci. 14, 322–336. doi: 10.1038/nrn3465
Anderson, P. J., Cheong, J. L., and Thompson, D. K. (2015). The predictive validity of neonatal MRI for neurodevelopmental outcome in very preterm children. Semin. Perinatol. 39, 147–158. doi: 10.1053/j.semperi.2015.01.008
Cai, M., Ma, J., Wang, Z., Zhao, Y., Zhang, Y., Wang, H., et al. (2023). Individual-level brain morphological similarity networks: current methodologies and applications. CNS Neurosci. Ther. doi: 10.1111/cns.14384
Cao, M., He, Y., Dai, Z., Liao, X., Jeon, T., Ouyang, M., et al. (2017). Early development of functional network segregation revealed by connectomic analysis of the preterm human brain. Cereb. Cortex 27, 1949–1963. doi: 10.1093/cercor/bhw038
Chen, Z. J., He, Y., Rosaneto, P., Gong, G., and Evans, A. C. (2011). Age-related alterations in the modular organization of structural cortical network by using cortical thickness from MRI. NeuroImage 56, 235–245. doi: 10.1016/j.neuroimage.2011.01.010
Duan, D., Rekik, I., Xia, S., Lin, W., Gilmore, J. H., Shen, D., et al. (2017a). “Longitudinal multi-scale mapping of infant cortical folding using spherical wavelets. Biomedical imaging (ISBI 2017),” in 2017 IEEE 14th International Symposium (Melbourne, VIC: IEEE), 93–96.
Duan, D., Xia, S., Meng, Y., Wang, L., Lin, W., Gilmore, J. H., et al. (2017b). “Exploring gyral patterns of infant cortical folding based on multi-view curvature information,” in International Conference onMedical Image Computing and Computer- Assisted Intervention (Quebec City, QC: Springer), 12–20.
Duan, D., Xia, S., Rekik, I., Meng, Y., Wu, Z., Wang, L., et al. (2019). Exploring folding patterns of infant cerebral cortex based on multi-view curvature features: Methods and applications. NeuroImage 185, 575–592. doi: 10.1016/j.neuroimage.2018.08.041
Duan, D., Xia, S., Rekik, I., Wu, Z., Wang, L., Lin, W., et al. (2020). Individual identification and individual variability analysis based on cortical folding features in developing infant singletons and twins. Hum. Brain Map. 41, 1985–2003. doi: 10.1002/hbm.24924
Dubois, J., Benders, M., Cachia, A., Lazeyras, F., Ha-Vinh Leuchter, R., Sizonenko, S., et al. (2007). Mapping the early cortical folding process in the preterm newborn brain. Cereb. Cortex 18, 1444–1454. doi: 10.1093/cercor/bhm180
Dubois, J., Lefèvre, J., Angleys, H., Leroy, F., Fischer, C., Lebenberg, J., et al. (2019). The dynamics of cortical folding waves and prematurity-related deviations revealed by spatial and spectral analysis of gyrification. Neuroimage 185, 934–946. doi: 10.1016/j.neuroimage.2018.03.005
Fan, Y., Shi, F., Smith, J. K., Lin, W., Gilmore, J. H., and Shen, D. (2011). Brain anatomical networks in early human brain development. Neuroimage 54, 1862–1871. doi: 10.1016/j.neuroimage.2010.07.025
Feess-Higgins, A., and Larroche, J.-C. (1987). Development of the Human Foetal Brain: An Anatomical Atlas. Institut Natl De LA Sante. Paris: Masson.
Fenchel, D., Dimitrova, R., Seidlitz, J., Robinson, E. C., Batalle, D., Hutter, J., et al. (2020). Development of microstructural and morphological cortical profiles in the neonatal brain. Cereb. Cortex 30, 5767–5779. doi: 10.1093/cercor/bhaa150
Galdi, P., Blesa, M., Stoye, D. Q., Sullivan, G., Lamb, G. J., Quigley, A. J., et al. (2020). Neonatal morphometric similarity mapping for predicting brain age and characterizing neuroanatomic variation associated with preterm birth. NeuroImage Clin. 25, 102195. doi: 10.1016/j.nicl.2020.102195
Gilmore, J. H., Knickmeyer, R. C., and Gao, W. (2018). Imaging structural and functional brain development in early childhood. Nat. Rev. Neurosci. 19, 123. doi: 10.1038/nrn.2018.1
Gilmore, J. H., Lin, W., Prastawa, M. W., Looney, C. B., Vetsa, Y. S. K., Knickmeyer, R. C., et al. (2007). Regional gray matter growth, sexual dimorphism, and cerebral asymmetry in the neonatal brain. J. Neurosci. 27, 1255–1260. doi: 10.1523/JNEUROSCI.3339-06.2007
Gilmore, J. H., Shi, F., Woolson, S. L., Knickmeyer, R. C., Short, S. J., Lin, W., et al. (2011). Longitudinal development of cortical and subcortical gray matter from birth to 2 years. Cereb. Cortex 22, 2478–2485. doi: 10.1093/cercor/bhr327
Gong, G., He, Y., Chen, Z. J., and Evans, A. C. (2012). Convergence and divergence of thickness correlations with diffusion connections across the human cerebral cortex. Neuroimage 59, 1239–1248. doi: 10.1016/j.neuroimage.2011.08.017
Groeschel, S., Vollmer, B., King, M., and Connelly, A. (2010). Developmental changes in cerebral grey and white matter volume from infancy to adulthood. Int. J. Dev. Neurosci. 28, 481–489. doi: 10.1016/j.ijdevneu.2010.06.004
He, Y., Chen, Z., and Evans, A. (2008). Structural insights into aberrant topological patterns of large-scale cortical networks in Alzheimer's disease. J. Neurosci. 28, 4756–4766. doi: 10.1523/JNEUROSCI.0141-08.2008
He, Y., Chen, Z. J., and Evans, A. C. (2007). Small-world anatomical networks in the human brain revealed by cortical thickness from MRI. Cereb. Cortex 17, 2407–2419. doi: 10.1093/cercor/bhl149
Hill, J., Dierker, D., Neil, J., Inder, T., Knutsen, A., Harwell, J., et al. (2010). A surface-based analysis of hemispheric asymmetries and folding of cerebral cortex in term-born human infants. J. Neurosci. 30, 2268–2276. doi: 10.1523/JNEUROSCI.4682-09.2010
Hüppi, P. S., Warfield, S., Kikinis, R., Barnes, P. D., Zientara, G. P., Jolesz, F. A., et al. (1998). Quantitative magnetic resonance imaging of brain development in premature and mature newborns. Ann. Neurol. 43, 224–235. doi: 10.1002/ana.410430213
Kim, S. Y., Liu, M., Hong, S.-J., Toga, A. W., Barkovich, A. J., Xu, D., et al. (2020). Disruption and compensation of sulcation-based covariance networks in neonatal brain growth after perinatal injury. Cereb. Cortex 30, 6238–6253. doi: 10.1093/cercor/bhaa181
Knickmeyer, R. C., Gouttard, S., Kang, C., Evans, D., Wilber, K., Smith, J. K., et al. (2008). A structural MRI study of human brain development from birth to 2 years. J. Neurosci. 28, 12176–12182. doi: 10.1523/JNEUROSCI.3479-08.2008
Kong, X., Liu, Z., Huang, L., Wang, X., Yang, Z., Zhou, G., et al. (2015). Mapping individual brain networks using statistical similarity in regional morphology from MRI. PLoS ONE 10, e0141840. doi: 10.1371/journal.pone.0141840
Li, G., Lin, W., Gilmore, J. H., and Shen, D. (2015). Spatial patterns, longitudinal development, and hemispheric asymmetries of cortical thickness in infants from birth to 2 years of age. J. Neurosci. 35, 9150–9162. doi: 10.1523/JNEUROSCI.4107-14.2015
Li, G., Nie, J., Wang, L., Shi, F., Lyall, A. E., Lin, W., et al. (2014a). Mapping longitudinal hemispheric structural asymmetries of the human cerebral cortex from birth to 2 years of age. Cereb. Cortex 24, 1289–1300. doi: 10.1093/cercor/bhs413
Li, G., Wang, L., Shi, F., Lyall, A. E., Lin, W., Gilmore, J. H., et al. (2014b). Mapping longitudinal development of local cortical gyrification in infants from birth to 2 years of age. J. Neurosci. 34, 4228–4238. doi: 10.1523/JNEUROSCI.3976-13.2014
Li, W., Yang, C., Shi, F., Wu, S., Wang, Q., Nie, Y., et al. (2017). Construction of individual morphological brain networks with multiple morphometric features. Front. Neuroanat. 11, 34. doi: 10.3389/fnana.2017.00034
Li, Y., Wang, N., Wang, H., Lv, Y., Zou, Q., and Wang, J. (2021). Surface-based single-subject morphological brain networks: effects of morphological index, brain parcellation and similarity measure, sample size-varying stability and test-retest reliability. Neuroimage 235, 118018. doi: 10.1016/j.neuroimage.2021.118018
Lyall, A. E., Shi, F., Geng, X., Woolson, S., Li, G., Wang, L., et al. (2015). Dynamic development of regional cortical thickness and surface area in early childhood. Cerebral Cortex 25, 2204. doi: 10.1093/cercor/bhu027
Meng, Y., Li, G., Lin, W., Gilmore, J. H., and Shen, D. (2015). “Cortical surfacebased construction of individual structural network with application to early brain development study,” in International Conference on Medical Image Computing and Computer-Assisted Intervention (Munich: Springer), 560–568.
Nie, J., Li, G., and Shen, D. (2013). Development of cortical anatomical properties from early childhood to early adulthood. Neuroimage 76, 216–224. doi: 10.1016/j.neuroimage.2013.03.021
Nie, J., Li, G., Wang, L., Shi, F., Lin, W., Gilmore, J. H., et al. (2014). Longitudinal development of cortical thickness, folding, and fiber density networks in the first 2 years of life. Hum. Brain Mapp. 35, 3726–3737. doi: 10.1002/hbm.22432
Sebenius, I., Seidlitz, J., Warrier, V., Bethlehem, R. A., Alexander-Bloch, A., Mallard, T. T., et al. (2023). Robust estimation of cortical similarity networks from brain MRI. Nat. Neurosci. 26, 1461–1471. doi: 10.1038/s41593-023-01376-7
Seeley, W. W., Crawford, R. K., Zhou, J., Miller, B. L., and Greicius, M. D. (2009). Neurodegenerative diseases target large-scale human brain networks. Neuron 62, 42–52. doi: 10.1016/j.neuron.2009.03.024
Seidlitz, J., Váša, F., Shinn, M., Romero-Garcia, R., Whitaker, K., Whitaker, K. J., et al. (2018). Morphometric similarity networks detect microscale cortical organization and predict inter-individual cognitive variation. Neuron 97, 1–17. doi: 10.1016/j.neuron.2017.11.039
Wang, F., Lian, C., Wu, Z., Zhang, H., Li, T., Meng, Y., et al. (2019). Developmental topography of cortical thickness during infancy. Proc. Nat. Acad. Sci. U. S. A. 116, 15855–15860. doi: 10.1073/pnas.1821523116
Xia, J., Wang, F., Benkarim, O. M., Sanroma, G., Piella, G., González Ballester, M. A., et al. (2019). Fetal cortical surface atlas parcellation based on growth patterns. Hum. Brain Mapp. 40, 3881–3899. doi: 10.1002/hbm.24637
Yao Z. Zhang Y. Lin L. Zhou Y. Xu C. Jiang T. the Alzheimer's Disease Neuroimaging Initiative (2010). Abnormal cortical networks in mild cognitive impairment and Alzheimer's disease. PLoS Comput. Biol. 6, e1001006. doi: 10.1371/journal.pcbi.1001006
Keywords: MRI, cortical morphology, structural covariance network, human brain, early development
Citation: Duan D and Wen D (2023) MRI-based structural covariance network in early human brain development. Front. Neurosci. 17:1302069. doi: 10.3389/fnins.2023.1302069
Received: 26 September 2023; Accepted: 16 October 2023;
Published: 01 November 2023.
Edited by:
Feng Liu, Tianjin Medical University General Hospital, ChinaReviewed by:
Xuyun Wen, Nanjing University of Aeronautics and Astronautics, ChinaCopyright © 2023 Duan and Wen. This is an open-access article distributed under the terms of the Creative Commons Attribution License (CC BY). The use, distribution or reproduction in other forums is permitted, provided the original author(s) and the copyright owner(s) are credited and that the original publication in this journal is cited, in accordance with accepted academic practice. No use, distribution or reproduction is permitted which does not comply with these terms.
*Correspondence: Dong Wen, d2VuY2FuZ2RvbmdAMTYzLmNvbQ==
Disclaimer: All claims expressed in this article are solely those of the authors and do not necessarily represent those of their affiliated organizations, or those of the publisher, the editors and the reviewers. Any product that may be evaluated in this article or claim that may be made by its manufacturer is not guaranteed or endorsed by the publisher.
Research integrity at Frontiers
Learn more about the work of our research integrity team to safeguard the quality of each article we publish.