- 1Research Institute of Pharmaceutical Sciences, Seoul National University, Seoul, South Korea
- 2College of Pharmacy, Seoul National University, Seoul, South Korea
Introduction: Alzheimer’s disease and other forms of dementia are disease that bring an increased global burden. However, the medicine developed to date remains limited. The purpose of this study is to predict drug repositioning candidates using a computational method that integrates gene expression profiles on Alzheimer’s disease and compound-induced changes in gene expression levels.
Methods: Gene expression data on Alzheimer’s disease were obtained from the Gene Expression Omnibus (GEO) and we conducted a meta-analysis of their gene expression levels. The reverse scores of compound-induced gene expressions were computed based on the reversal relationship between disease and drug gene expression profiles.
Results: Reversal genes and the candidate compounds were identified by the leave-one-out cross-validation procedure. Additionally, the half-maximal inhibitory concentration (IC50) values and the blood-brain barrier (BBB) permeability of candidate compounds were obtained from ChEMBL and PubChem, respectively.
Conclusion: New therapeutic target genes and drug candidates against Alzheimer’s disease were identified by means of drug repositioning.
Introduction
Alzheimer’s disease is the most common cause of dementia, a common neurodegenerative disease. Dementia is an escalating global burden disease, recently estimated to affect 57.4 million people worldwide as of 2019, increasing to 153.8 million by 2050 (GBD 2019 Dementia Forecasting Collaborators, 2022). Although the underlying mechanisms of Alzheimer’s disease remain not fully understood (Burns and Iliffe, 2009), currently Alzheimer’s disease is characterized by initial forgetfulness and cognitive decline that can affect behavior and the motor system finally (DeTure and Dickson, 2019). The presence of extracellular beta-amyloid deposition and intracellular neurofibrillary tangles consisting of hyperphosphorylated tau are the neuropathological hallmark features for an Alzheimer’s disease diagnosis (Long and Holtzman, 2019).
Drugs can be an important part of Alzheimer’s disease treatments. However, there is no treatment available to cure Alzheimer’s disease, only those that can treat the symptoms for a while or slow down their progression in some patients (Folch et al., 2018). The most commonly used medicines are cholinesterase inhibitors such as donepezil, galantamine, and rivastigmine, and an N-methyl-D-aspartate receptor antagonist, memantine (Scheltens et al., 2021). Despite the fact that aducanumab, a monoclonal antibody that reduces beta-amyloid deposits in the brain, was approved in an accelerated manner by the U.S. FDA in 2021 (Mullard, 2021), there is still some argument against its use (Whitehouse et al., 2022). The European Medicines Agency rejected this drug’s approval for the treatment of mild cognitive impairment due to Alzheimer’s disease and mild Alzheimer’s dementia (Mahase, 2021). Therefore, it remains necessary to discovery new drug targets and drugs against Alzheimer’s disease due to the ambiguity in efficient pathological targets or the adverse effects of previously used drugs.
Drug repositioning refers to the application of established drugs to new therapeutic indications (Langedijk et al., 2015), accelerating the development process due to the reductions in the time and costs involved in the progress of evaluating preclinical safety. Publicly available transcriptomic data generated from disease samples, as well as compounds, provide an opportunity for understanding the pathologies of diseases and the mechanisms of actions of drugs and for discovering new applications for existing drugs (Jarada et al., 2020). Recently, computational techniques using genome-wide gene expressions, especially chemical-induced or disease-specific differential gene expression levels, network analyses, or deep-learning approaches, have been applied for the identification of new therapeutic targets and drug repurposing (Lamb et al., 2006; Dudley et al., 2011; Chen et al., 2017; Advani and Kumar, 2021; Chyr et al., 2022).
Especially in relation to neurodegenerative diseases, which require long-term treatments in elderly patients, who frequently have comorbidities and take a multitude of medications, clinical trials are difficult. Consequently, the purpose of this study is to identify potential candidate drugs as a treatment for Alzheimer’s disease using a systematic computational drug repositioning method that combines disease-specific and drug-induced gene expression profiles.
Materials and methods
Collection of gene expression data on disease
Gene expression data related to Alzheimer’s disease were downloaded from the Gene Expression Omnibus (GEO) database established at the National Center for Biotechnology Information (NCBI) (Barrett and Edgar, 2006). The keywords were “Alzheimer’s disease” and the search results were limited to those published from 2017 to May of 2022, “Homo sapiens” as the organism, and “expression profiling by array” as the study type were used. These data, including the GEO accession number, summary, sample type, overall design, and platform were collected. The brain regions of the entorhinal cortex (EC), medial temporal gyrus (MTG) and temporal cortex (TC) were selected. The dataset containing healthy controls was included. If the Braak stages of the samples were available, the samples with Braak scores ≤3 from Alzheimer’s disease or the samples with Braak scores ≥3 from controls were removed from any further analysis (Patel et al., 2019). Gene expression data pertaining to brain tissues from patients with Alzheimer’s disease or controls were downloaded. The expression levels were transformed to logarithms and normalized. If multiple probes were assigned to one gene, the probe with the highest interquartile range was selected as the representative probe for that gene.
Meta-analysis of gene expression on disease
To obtain a robust result, 10% of non-expressed genes based on mean intensities across the studies and 30% of non-informative genes based on variance were removed. The standardized mean rank method in MetaQC (Kang et al., 2012) was initially applied to conduct a gene expression quality check of each GEO dataset. Quantitative quality control (QC) measures including internal QC, external QC, accuracy QC, and consistency QC of genes or pathways and the standardized mean rank summary score for each dataset were assessed. All P-values were adjusted by Bonferroni correction. Additionally, a principal component analysis (PCA) biplot was applied to the visualization and helped determine the inclusion or exclusion of studies in the meta-analysis. The MetaDE package (Wang et al., 2012) was used to identify meta-differentially expressed genes (DEGs) between Alzheimer’s disease and the control. The P-values and combined effect sizes in the meta-analysis were calculated with a fixed effect model (Choi et al., 2003). This meta-gene expression levels were used as disease signatures for further analysis.
Collection of gene expression data on compounds
Chemical compound-induced genome-wide transcriptional profiles were downloaded from the Library of Integrated Network-based Cellular Signatures (LINCS) (Koleti et al., 2018). Information regarding the cellular contexts, treatment time points, and treatment concentrations across multiple compounds was collected.
Computation of reverse gene expression scores
Reverse gene expression scores were computed using the method described by Chen et al. (2017), and the description in the methods section partly reproduces their wording. Reverse scores of compounds indicate a reversal correlation between the DEGs associated with disease and compounds. An enrichment reverse score of gene expression associated with disease was computed based on the DEG rank. Additionally, drug-associated genes were ranked based on their expression levels as regulated by each compound from LINCS. Therefore, if the possibility of reversing the gene expression associated with the disease is higher, the negative value of the reverse score becomes lower. The result is such that each compound can have more than one reverse gene expression score. If multiple scores were computed for the one compound, summarized reverse scores of gene expression were calculated by weighted linear regression considering the experimental conditions, including the cell lines, concentrations, and treatment time points of the compounds. The reference condition established here used a concentration of 10 μM and treatment time of 24 h (Chen et al., 2017).
Identification candidate compounds and reversed genes
Genes whose expression levels were reversed by a compound were determined by the leave-one-out cross-validation process to decrease over-fitting problems (Cheng et al., 2017). Upregulated genes were ranked toward the top, whereas downregulated genes were ranked toward the bottom. Each compound in turn was taken to be the test set, while the data for the remaining compounds served as the training set. Reversed genes by compound were then identified using the process described above. In all trials, false discovery rate-adjusted P-values of less than 0.05 were considered as indicative of reversal genes.
Cell cytotoxicity
Bioactivity IC50 data using human brain tumor cells were downloaded from ChEMBL (Gaulton et al., 2017). Information on the units, molecular weights, cells, and organisms was collected. Compounds in ChEMBL were manually mapped with those in LINCS based on the IUPAC International Chemical Identifier (InChI). If multiple IC50 values were available for one compound, the corresponding median value was calculated.
Prediction of permeability across the blood-brain barrier
The blood-brain barrier (BBB) permeability of the compounds in each case was predicted by the DeepPred-BBB model (Kumar et al., 2022) using their canonical simplified molecular input line entry system (SMILES). The SMILES strings were obtained from PubChem (Kim et al., 2021).
Results
Disease gene expression signatures
Gene expression data for Alzheimer’s disease were searched for and download from GEO. Forty-seven GEO Series Experiments (GSEs) were found (Figure 1). A number of datasets were excluded due to duplication (n = 4), a different disease (n = 8), a lack of brain temporal region tissue samples (n = 28), a lack of mRNA expression data (n = 1), and a lack of a control (n = 2). The four datasets of GSE109887, GSE118553, GSE132903, and GSE138260 were selected for the MetaQC analysis. The data were divided according to the region of brain tissue, EC, MTG, and TC, if the gene expression data were measured from different brain tissues. A detailed description of these datasets is presented in Supplementary Table 1. Supplementary Table 2 shows the QC measures and standardized mean rank scores. The PCA plot is shown in Supplementary Figure 1. Although the first two PCs explained approximately 98% of the total variance, the datasets were scattered in the plot. Therefore, all four datasets were included in the meta-analysis, which contains 249 Alzheimer’s disease and 204 control lesions. Among the disease signatures, 74 genes including ZNG621, ANTXR2, and DNALI1 showed increased expression levels in Alzheimer’s disease lesions compared to control lesions [log 2 (fold change) >1.0, adjusted P < 0.001], whereas 81 genes including SYNGR1, TUBB2A, SVOP, STMN2, and KLHL35 showed decreased expression levels in dementia lesions [log 2 (fold change) <−1.0 adjusted P < 0.001] (Supplementary Figure 2). Sixty-two DEGs filtered via log 2 (fold change) >1.1 or <−1.1 and adjusted P < 0.001 are listed in Supplementary Table 3.
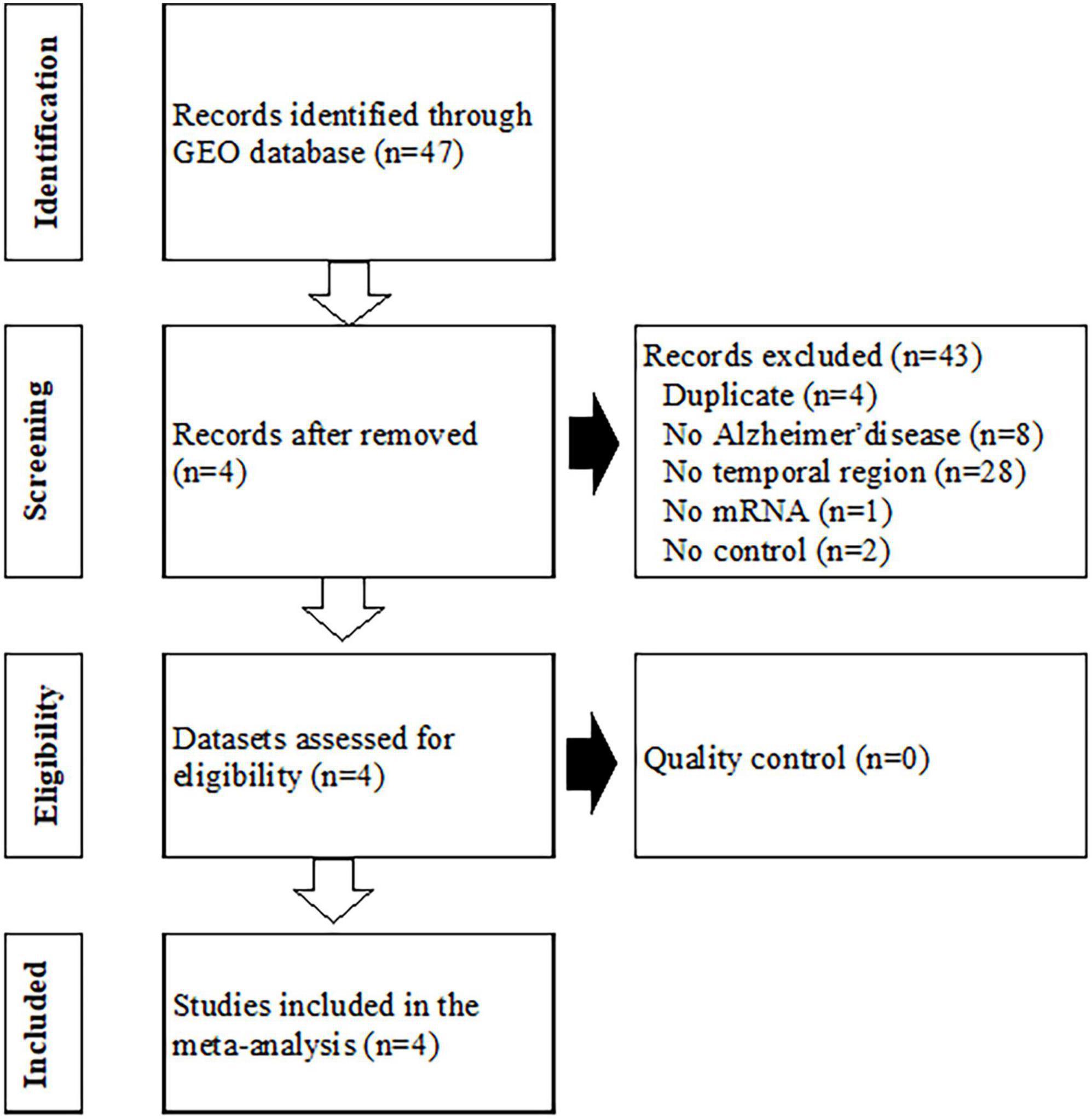
Figure 1. Flowchart of the process used to select gene expression datasets for the meta-analysis of Alzheimer’s disease. GEO, Gene Expression Omnibus.
Drug gene expression signatures
Reverse scores as drug signatures were computed according to changes in the expression levels of the 978 landmark genes from the LINCS data. These scores were computed based on 5,302 compound treatments in NPC human neural precursor cells. Summarized reverse scores were computed by weighting the concentrations and time points of the compounds and various cell lines. The minimal summarized reverse score was −1.014 and maximal summarized reverse scores was 1.093, respectively.
Inhibitory concentrations in human brain tumor cells
The IC50 values for 875 compounds in human brain tumor cells, such as astrocytoma, glioblastoma, and neuroblastoma, were obtained from ChEMBL. The cell lines used for the IC50 values are listed in Supplementary Table 4. The IC50 values varied widely from 0.005 nM to 1320000 μM. Among the compounds, 174 compounds have computed reverse scores. Summarized reversal scores of the 174 compounds were variously distributed according to their median IC50 values (Figure 2).
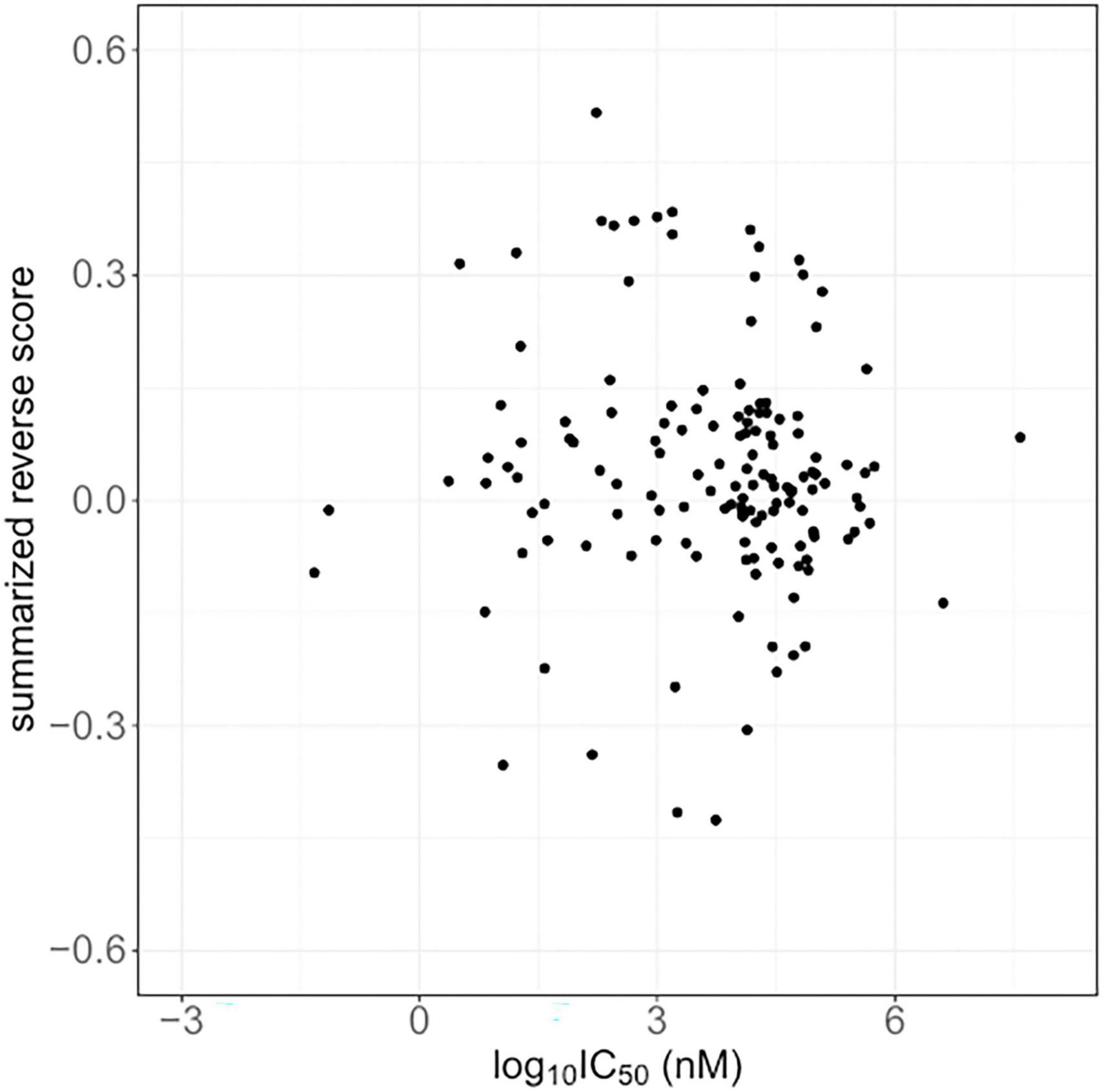
Figure 2. The summarized reverse gene expression scores and half-maximal inhibitory concentrations (IC50) from ChemBL.
Reversed gene identification and compound predictions
The method of leave-one-out cross-validation was used to select reversely expressed genes by these compounds. Seventeen genes showed significantly reversed expressions (Table 1). TNFRSF21, WDR7, DFFA, IQGAP1, WFS1, RAI14, and ANXA7 were downregulated upon treatment with compounds. STMN1, SMARCC1, ALDOA, CLTC, PSMG1, SUZ12, DAG1, PGRMC1, and HMGCR, and MYO10 were upregulated following compound treatment Additionally, the compounds against Alzheimer’s disease were identified and their BBB permeability levels were predicted. The predicted BBB non-permeable compounds are listed in Supplementary Table 5, while the predicted BBB permeable compounds are shown in Figure 3. The protein kinase inhibitors including dasatinib, semaxanib, palbociclib, bosutinib, erlotinib, axitinib, tozasertib, saracatinib, lestaurtinib, staurosporine, and midostaurin, calcium channel blockers including nimodipine and nifedipine, histone deacetylase inhibitors including vorinostat, proteasome inhibitors including mg-132, and natural polyphenols including curcumin and pterostilbene were identified. Additionally, antineoplatic agents such as obatoclax, olomoucine, amsacrine, temozolomide, and tamoxifen were identified.
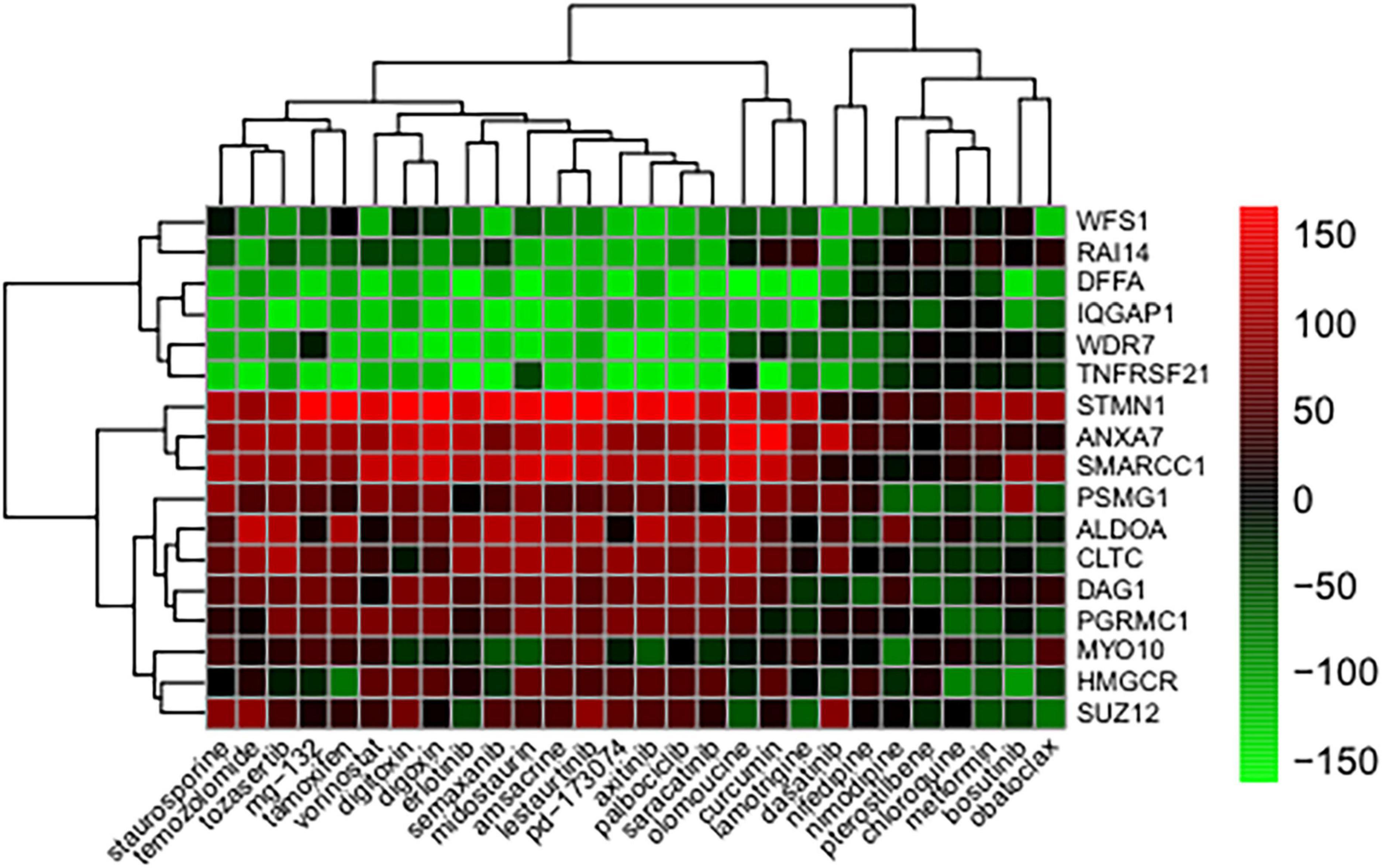
Figure 3. Genes whose expression was reversed in response to treatments with compound. The heatmap indicates the relative position of a gene in ranked compound expression data. Position are normalized and compound columns are ordered according to reversal scores. The distance matrix was calculated by the Euclidean method and the dendrogram was drawn by the complete linkage method. Low and high ranks suggest that the gene expression is down and upregulated, respectively, by the corresponding compound. Red and green colors indicate up and downregulation, respectively, after compound treatment.
Discussion
The burden associated with Alzheimer’s disease continues to increase, and there is consequently an urgent need to seek innovative solutions to resolve this issue. In this study, we used a computational method that integrates disease-specific gene expression profiles and drug-induced gene expression profiles to predict drug candidates for use in Alzheimer’s disease treatments.
We identified seventeen therapeutic targets and twenty-eight repositioning candidates against Alzheimer’s disease. Several DEGs were found after a meta-gene expression analysis of Alzheimer’s disease in our study. It has been reported that amyloid precursor protein binds with tumor necrosis factor receptor superfamily member 21 (TNFRSF21) to induce neural inflammation in Alzheimer’s disease (Zhang et al., 2021). Induced wolframin endoplasmic reticulum transmembrane glycoprotein (WFS1) deficiency has been found to increase tau toxicity, which may play important roles in the development and progression of Alzheimer’s disease (Li et al., 2020). Progesterone receptor membrane component 1 (PGRMC1) increases the neuronal toxicity of amyloid beta-peptides by binding to the amyloid beta oligomer in Alzheimer’s disease (Qin et al., 2015). 3-Hydroxy-3-methylglutaryl-CoA reductase (HMGCR) was found to be a genetic modifier of the risk of Alzheimer’s disease (Leduc et al., 2015). Stathmin 1 (STMN1) as a cytosolic phosphoprotein that regulated microtubules dynamics, impaired axonal transport, and cause human neurodegenerative diseases (Duncan et al., 2013). Astrocytic dystrophin-associated complex components including dystroglycan 1 (DAG1) is known to be associated with temporal tau pathology (Simon et al., 2018).
Several repositionable candidate drugs, especially anticancer agents, against Alzheimer’s disease were identified in our study. Autophagy is the key mechanism by which to remove cellular abnormal protein aggregates, and the dysfunction of this process contributes to the pathogenesis of Alzheimer’s disease (Zhang et al., 2021). Most anticancer agents are able to induce autophagy or apoptosis, which may contribute to the neurodegenerative process (Eshraghi et al., 2022). Accumulating evidence has suggested that Alzheimer’s disease and cancer share some familiar biological pathways (Advani et al., 2020). Preclinical and clinical evaluations of several kinase inhibitors are ongoing in relation to Alzheimer’s disease (Fagiani et al., 2020; Prins et al., 2021). A pilot clinical trial of a senolytic combination therapy of dasatinib plus quercetin in early-stage Alzheimer’s disease was conducted (Gonzales et al., 2022). Additionally, vorinostat, a histone deacetylase inhibitor has also been proposed for use in the treatment of Alzheimer’s disease, phase I clinical trials were conducted (Eshraghi et al., 2022). However, it is important to consider that anticancer drugs can destroy subcellular components and disable fundamental biological processes. For instance, some anti-neoplastic agents can cause DNA damage, which is a critical pathological cause of Alzheimer’s disease (Lin et al., 2020). Therefore, we compared the reverse scores with the IC50 values of the compounds in our study. The reversal potency was not correlated to the IC50 values in Alzheimer’s disease, despite the higher variations in the reverse scores and IC50 values. It was previously reported that reverse scores were not correlated with IC50 values in cases involving microtubule inhibitors (Chen et al., 2017). The most commonly identified compound candidates in our results had microtubule-associated activity, and microtubule destabilization is known to be related to Alzheimer’s disease (Fernandez-Valenzuela et al., 2020). From this point of view, further investigations are required.
An antidiabetic agent, metformin (De Santi et al., 2019) exhibit pro-autophagy properties, which imply that these may be useful as a treatment for Alzheimer’s disease. Digoxin was reported to be beneficial in treating cognitive impairment in a mammalian model (Erdogan et al., 2022). The clinical efficacy of lamotrigine and changes in the dosages of concomitantly used psychotropic drugs were studied in relation to Alzheimer’s disease in conjunction with behavioral and psychological symptoms of dementia as a preliminary clinical trial (Suzuki and Gen, 2015). Calcium channel blockers have been reported to decrease significantly the rate of progression to dementia, which may minimize the formation of amyloid beta (Lovell et al., 2015), and nilvadipine was studied as a treatment in patients with mild to moderate Alzheimer’s disease (Lawlor et al., 2018).
Several natural products have been used in patients with Alzheimer’s disease. Curcumin has been shown to maintain the normal structure and function of cerebral vessels, mitochondria, and synapses effectively and to reduce the risk of Alzheimer’s disease (Chen et al., 2018). Numerous investigations in cellular and animal models have associated resveratrol and pterostilbene with protection against Alzheimer’s disease (Lange and Li, 2018).
On the other hand, with regard to repurposing for the treatment of Alzheimer’s disease, accessing the degree of penetration through the BBB requires significant consideration (Benn and Dawson, 2020). Therefore, we also predicted BBB permeability using deep learning and machine learning algorithms in our study.
Highly expressed TNFRSF21 gene was significantly correlated with the dasatinib sensitivity or resistance (Huang et al., 2007). Nifedipine was found to activate 3-hydroxy-3-methyl-glutaryl-coenzyme A (HMG CoA) reductase, suggesting elevated production of cholesterol and phospholipids (Lin et al., 2019). DFFA gene associated with sensitivity or resistance of curcumin in tumor cells (Sertel et al., 2012) and treatment with curcumin was able to significantly increase the levels of CLTC mRNA in curcumin treated cells compared with control (Luo et al., 2018). The studies of association between candidate compounds and genes were still not enough for Alzheimer’s disease. The pathophysiologic mechanisms of Alzheimer’s disease are complex and are not well known. Pharmacological action and therapeutic outcomes cannot be determined by the simple approach of reverse gene expression profiling and the further evaluations need to replicate our results. Therefore, our findings likely indicate further investigations of treatments of Alzheimer’s disease. In summary, repositioning drug candidates and target genes in Alzheimer’s disease were identified using a computational method that combined disease-specific gene expressions with drug-induced gene expressions.
Data availability statement
The datasets generated and/or analyzed for this study can be found are available from the corresponding author on reasonable request.
Author contributions
I-WK analyzed the data and prepared the manuscript. HJ corrected and revised the manuscript. I-WK and JO contributed to the conception and design of the study. All authors were engaged in commenting on the manuscript, and read and approved the final manuscript.
Funding
This study was supported by the National Research Foundation of Korea grant funded by the Korea government (MSIT) (No. 2021R1A2C1006046).
Acknowledgments
We appreciate the previous work in Chen’s laboratory that developed the RGES method and provided analysis code.
Conflict of interest
The authors declare that the research was conducted in the absence of any commercial or financial relationships that could be construed as a potential conflict of interest.
Publisher’s note
All claims expressed in this article are solely those of the authors and do not necessarily represent those of their affiliated organizations, or those of the publisher, the editors and the reviewers. Any product that may be evaluated in this article, or claim that may be made by its manufacturer, is not guaranteed or endorsed by the publisher.
Supplementary material
The Supplementary Material for this article can be found online at: https://www.frontiersin.org/articles/10.3389/fnins.2022.989174/full#supplementary-material
References
Advani, D., and Kumar, P. (2021). Therapeutic targeting of repurposed anticancer drugs in Alzheimer’s disease: Using the multiomics approach. ACS Omega 6, 13870–13887. doi: 10.1021/acsomega.1c01526
Advani, D., Gupta, R., Tripathi, R., Sharma, S., Ambasta, R. K., and Kumar, P. (2020). Protective role of anticancer drugs in neurodegenerative disorders: A drug repurposing approach. Neurochem. Int. 140:104841. doi: 10.1016/j.neuint.2020.104841
Barrett, T., and Edgar, R. (2006). Mining microarray data at NCBI’s Gene expression omnibus (GEO)*. Methods Mol. Biol. 338, 175–190. doi: 10.1385/1-59745-097-9:175
Benn, C. L., and Dawson, L. A. (2020). Clinically precedented protein kinases: Rationale for their use in neurodegenerative disease. Front. Aging Neurosci. 12:242. doi: 10.3389/fnagi.2020.00242
Chen, B., Ma, L., Paik, H., Sirota, M., Wei, W., Chua, M. S., et al. (2017). Reversal of cancer gene expression correlates with drug efficacy and reveals therapeutic targets. Nat. Commun. 8:16022. doi: 10.1038/ncomms16022
Chen, M., Du, Z. Y., Zheng, X., Li, D. L., Zhou, R. P., and Zhang, K. (2018). Use of curcumin in diagnosis, prevention, and treatment of Alzheimer’s disease. Neural Regen. Res. 13, 742–752. doi: 10.4103/1673-5374.230303
Cheng, H., Garrick, D. J., and Fernando, R. L. (2017). Efficient strategies for leave-one-out cross validation for genomic best linear unbiased prediction. J. Anim. Sci. Biotechnol. 8:38. doi: 10.1186/s40104-017-0164-6
Choi, J. K., Yu, U., Kim, S., and Yoo, O. J. (2003). Combining multiple microarray studies and modeling interstudy variation. Bioinformatics 19:i84–i90. doi: 10.1093/bioinformatics/btg1010
Chyr, J., Gong, H., and Zhou, X. (2022). DOTA: Deep learning optimal transport approach to advance drug repositioning for Alzheimer’s disease. Biomolecules 12:196. doi: 10.3390/biom12020196
De Santi, M., Baldelli, G., Diotallevi, A., Galluzzi, L., Schiavano, G. F., and Brandi, G. (2019). Metformin prevents cell tumorigenesis through autophagy-related cell death. Sci. Rep. 9:66. doi: 10.1038/s41598-018-37247-6
DeTure, M. A., and Dickson, D. W. (2019). The neuropathological diagnosis of Alzheimer’s disease. Mol. Neurodegener. 14:32. doi: 10.1186/s13024-019-0333-5
Dudley, J. T., Deshpande, T., and Butte, A. J. (2011). Exploiting drug-disease relationships for computational drug repositioning. Brief Bioinform. 12, 303–311. doi: 10.1093/bib/bbr013
Duncan, J. E., Lytle, N. K., Zuniga, A., and Goldstein, L. S. (2013). The microtubule regulatory protein stathmin is required to maintain the integrity of axonal microtubules in drosophila. PLoS One 8:e68324. doi: 10.1371/journal.pone.0068324
Erdogan, M. A., Kirazlar, M., Yigitturk, G., and Erbas, O. (2022). Digoxin exhibits neuroprotective properties in a rat model of dementia. Neurochem. Res. 47, 1290–1298. doi: 10.1007/s11064-022-03528-w
Eshraghi, M., Ahmadi, M., Afshar, S., Lorzadeh, S., Adlimoghaddam, A., Rezvani Jalal, N., et al. (2022). Enhancing autophagy in Alzheimer’s disease through drug repositioning. Pharmacol. Ther. 237:108171. doi: 10.1016/j.pharmthera.2022.108171
Fagiani, F., Lanni, C., Racchi, M., and Govoni, S. (2020). Targeting dementias through cancer kinases inhibition. Alzheimers Dement 6:e12044. doi: 10.1002/trc2.12044
Fernandez-Valenzuela, J. J., Sanchez-Varo, R., Munoz-Castro, C., De Castro, V., Sanchez-Mejias, E., Navarro, V., et al. (2020). Enhancing microtubule stabilization rescues cognitive deficits and ameliorates pathological phenotype in an amyloidogenic Alzheimer’s disease model. Sci. Rep. 10:14776. doi: 10.1038/s41598-020-71767-4
Folch, J., Ettcheto, M., Petrov, D., Abad, S., Pedros, I., Marin, M., et al. (2018). Review of the advances in treatment for Alzheimer disease: Strategies for combating beta-amyloid protein. Neurologia 33, 47–58. doi: 10.1016/j.nrl.2015.03.012
Gaulton, A., Hersey, A., Nowotka, M., Bento, A. P., Chambers, J., Mendez, D., et al. (2017). The ChEMBL database in 2017. Nucleic Acids Res. 45:D945–D954. doi: 10.1093/nar/gkw1074
GBD 2019 Dementia Forecasting Collaborators (2022). Estimation of the global prevalence of dementia in 2019 and forecasted prevalence in 2050: An analysis for the global burden of disease Study 2019. Lancet Public Health 7:e105–e125. doi: 10.1016/S2468-2667(21)00249-8
Gonzales, M. M., Garbarino, V. R., Marques Zilli, E., Petersen, R. C., Kirkland, J. L., Tchkonia, T., et al. (2022). Senolytic therapy to modulate the progression of Alzheimer’s disease (SToMP-AD): A pilot clinical trial. J. Prev. Alzheimers Dis. 9, 22–29. doi: 10.14283/jpad.2021.62
Huang, F., Reeves, K., Han, X., Fairchild, C., Platero, S., Wong, T. W., et al. (2007). Identification of candidate molecular markers predicting sensitivity in solid tumors to dasatinib: Rationale for patient selection. Cancer Res. 67, 2226–2238. doi: 10.1158/0008-5472.CAN-06-3633
Jarada, T. N., Rokne, J. G., and Alhajj, R. (2020). A review of computational drug repositioning: Strategies, approaches, opportunities, challenges, and directions. J. Cheminform. 12:46. doi: 10.1186/s13321-020-00450-7
Kang, D. D., Sibille, E., Kaminski, N., and Tseng, G. C. (2012). MetaQC: Objective quality control and inclusion/exclusion criteria for genomic meta-analysis. Nucleic Acids Res. 40:e15. doi: 10.1093/nar/gkr1071
Kim, S., Chen, J., Cheng, T., Gindulyte, A., He, J., He, S., et al. (2021). PubChem in 2021: New data content and improved web interfaces. Nucleic Acids Res. 49:D1388–D1395. doi: 10.1093/nar/gkaa971
Koleti, A., Terryn, R., Stathias, V., Chung, C., Cooper, D. J., Turner, J. P., et al. (2018). Data Portal for the Library of Integrated Network-based Cellular Signatures (LINCS) program: Integrated access to diverse large-scale cellular perturbation response data. Nucleic Acids Res. 46:D558–D566. doi: 10.1093/nar/gkx1063
Kumar, R., Sharma, A., Alexiou, A., Bilgrami, A. L., Kamal, M. A., and Ashraf, G. M. (2022). DeePred-BBB: A blood brain barrier permeability prediction model with improved accuracy. Front. Neurosci. 16:858126. doi: 10.3389/fnins.2022.858126
Lamb, J., Crawford, E. D., Peck, D., Modell, J. W., Blat, I. C., Wrobel, M. J., et al. (2006). The connectivity map: Using gene-expression signatures to connect small molecules, genes, and disease. Science 313, 1929–1935. doi: 10.1126/science.1132939
Lange, K. W., and Li, S. (2018). Resveratrol, pterostilbene, and dementia. Biofactors 44, 83–90. doi: 10.1002/biof.1396
Langedijk, J., Mantel-Teeuwisse, A. K., Slijkerman, D. S., and Schutjens, M. H. (2015). Drug repositioning and repurposing: Terminology and definitions in literature. Drug Discov. Today 20, 1027–1034. doi: 10.1016/j.drudis.2015.05.001
Lawlor, B., Segurado, R., Kennelly, S., Olde Rikkert, M. G. M., Howard, R., Pasquier, F., et al. (2018). Nilvadipine in mild to moderate Alzheimer disease: A randomised controlled trial. PLoS Med. 15:e1002660. doi: 10.1371/journal.pmed.1002660
Leduc, V., De Beaumont, L., Theroux, L., Dea, D., Aisen, P., Petersen, R. C., et al. (2015). HMGCR is a genetic modifier for risk, age of onset and MCI conversion to Alzheimer’s disease in a three cohorts study. Mol. Psychiatry 20, 867–873. doi: 10.1038/mp.2014.81
Li, L., Venkataraman, L., Chen, S., and Fu, H. (2020). Function of WFS1 and WFS2 in the central nervous system: Implications for wolfram syndrome and Alzheimer’s disease. Neurosci. Biobehav. Rev. 118, 775–783. doi: 10.1016/j.neubiorev.2020.09.011
Lin, X., Kapoor, A., Gu, Y., Chow, M. J., Peng, J., Zhao, K., et al. (2020). Contributions of DNA damage to Alzheimer’s disease. Int. J. Mol. Sci. 21:1666. doi: 10.3390/ijms21051666
Lin, Y. C., Wu, M. S., Lin, Y. F., Chen, C. R., Chen, C. Y., Chen, C. J., et al. (2019). Nifedipine modulates renal lipogenesis via the AMPK-SREBP transcriptional pathway. Int. J. Mol. Sci. 20:1570. doi: 10.3390/ijms20071570
Long, J. M., and Holtzman, D. M. (2019). Alzheimer disease: An update on pathobiology and treatment strategies. Cell 179, 312–339. doi: 10.1016/j.cell.2019.09.001
Lovell, M. A., Abner, E., Kryscio, R., Xu, L., Fister, S. X., and Lynn, B. C. (2015). Calcium channel blockers, progression to dementia, and effects on amyloid beta peptide production. Oxid. Med. Cell Longev. 2015:787805. doi: 10.1155/2015/787805
Luo, Z., Li, D., Luo, X., Li, L., Gu, S., Yu, L., et al. (2018). Curcumin may serve an anticancer role in human osteosarcoma cell line U-2 OS by targeting ITPR1. Oncol. Lett. 15, 5593–5601. doi: 10.3892/ol.2018.8032
Mahase, E. (2021). Aducanumab: European agency rejects Alzheimer’s drug over efficacy and safety concerns. BMJ 375:n3127. doi: 10.1136/bmj.n3127
Mullard, A. (2021). Controversial Alzheimer’s drug approval could affect other diseases. Nature 595, 162–163. doi: 10.1038/d41586-021-01763-9
Patel, H., Dobson, R. J. B., and Newhouse, S. J. (2019). A meta-analysis of Alzheimer’s disease brain transcriptomic data. J. Alzheimers Dis. 68, 1635–1656. doi: 10.3233/JAD-181085
Prins, N. D., Harrison, J. E., Chu, H. M., Blackburn, K., Alam, J. J., Scheltens, P., et al. (2021). A phase 2 double-blind placebo-controlled 24-week treatment clinical study of the p38 alpha kinase inhibitor neflamapimod in mild Alzheimer’s disease. Alzheimers Res. Ther. 13:106. doi: 10.1186/s13195-021-00843-2
Qin, Y., Chen, Z., Han, X., Wu, H., Yu, Y., Wu, J., et al. (2015). Progesterone attenuates Abeta(25-35)-induced neuronal toxicity via JNK inactivation and progesterone receptor membrane component 1-dependent inhibition of mitochondrial apoptotic pathway. J. Steroid Biochem. Mol. Biol. 154, 302–311. doi: 10.1016/j.jsbmb.2015.01.002
Scheltens, P., De Strooper, B., Kivipelto, M., Holstege, H., Chetelat, G., Teunissen, C. E., et al. (2021). Alzheimer’s disease. Lancet 397, 1577–1590. doi: 10.1016/S0140-6736(20)32205-4
Sertel, S., Eichhorn, T., Bauer, J., Hock, K., Plinkert, P. K., and Efferth, T. (2012). Pharmacogenomic determination of genes associated with sensitivity or resistance of tumor cells to curcumin and curcumin derivatives. J. Nutr. Biochem. 23, 875–884. doi: 10.1016/j.jnutbio.2011.04.012
Simon, M. J., Wang, M. X., Murchison, C. F., Roese, N. E., Boespflug, E. L., Woltjer, R. L., et al. (2018). Transcriptional network analysis of human astrocytic endfoot genes reveals region-specific associations with dementia status and tau pathology. Sci. Rep. 8:12389. doi: 10.1038/s41598-018-30779-x
Suzuki, H., and Gen, K. (2015). Clinical efficacy of lamotrigine and changes in the dosages of concomitantly used psychotropic drugs in Alzheimer’s disease with behavioural and psychological symptoms of dementia: A preliminary open-label trial. Psychogeriatrics 15, 32–37. doi: 10.1111/psyg.12085
Wang, X., Kang, D. D., Shen, K., Song, C., Lu, S., Chang, L. C., et al. (2012). An R package suite for microarray meta-analysis in quality control, differentially expressed gene analysis and pathway enrichment detection. Bioinformatics 28, 2534–2536. doi: 10.1093/bioinformatics/bts485
Whitehouse, P., Gandy, S., Saini, V., George, D. R., Larson, E. B., Alexander, G. C., et al. (2022). Making the Case for Accelerated Withdrawal of Aducanumab. J. Alzheimers Dis. 87, 1003–1007. doi: 10.3233/JAD-220262
Keywords: drug repositioning, gene expression, Alzheimer’s disease, meta-analysis, in silico
Citation: Jang HY, Oh JM and Kim I-W (2022) Drug repurposing using meta-analysis of gene expression in Alzheimer’s disease. Front. Neurosci. 16:989174. doi: 10.3389/fnins.2022.989174
Received: 08 July 2022; Accepted: 19 October 2022;
Published: 10 November 2022.
Edited by:
Raymond Scott Turner, Georgetown University, United StatesReviewed by:
Kwangsik Timothy Nho, Indiana University Bloomington, United StatesWeihua Yu, Chongqing Medical University, China
Copyright © 2022 Jang, Oh and Kim. This is an open-access article distributed under the terms of the Creative Commons Attribution License (CC BY). The use, distribution or reproduction in other forums is permitted, provided the original author(s) and the copyright owner(s) are credited and that the original publication in this journal is cited, in accordance with accepted academic practice. No use, distribution or reproduction is permitted which does not comply with these terms.
*Correspondence: In-Wha Kim, aXdraW0yQGhhbm1haWwubmV0