- 1Department of Surgical Sciences, University of Otago, Dunedin, New Zealand
- 2Department of Psychological Medicine, University of Otago, Dunedin, New Zealand
- 3Centre for Health, Activity, and Rehabilitation Research, University of Otago, Dunedin, New Zealand
Introduction: Internalizing disorders (IDs), e.g., major depressive disorder (MDD), posttraumatic stress disorder (PTSD), obsessive-compulsive disorder (OCD) are the most prevalent psychopathologies experienced worldwide. Current first-line therapies (i.e., pharmacotherapy and/or psychotherapy) offer high failure rates, limited accessibility, and substantial side-effects. Electroencephalography (EEG) guided closed-loop brain training, also known as EEG-neurofeedback (EEG-NFB), is believed to be a safe and effective alternative, however, there is much debate in the field regarding the existence of specificity [i.e., clinical effects specific to the modulation of the targeted EEG variable(s)]. This review was undertaken to determine if there is evidence for EEG-NFB specificity in the treatment of IDs.
Methods: We considered only randomized, double-blind, sham-controlled trials. Outcomes of interest included self/parent/teacher reports and clinician ratings of ID-related symptomatology.
Results: Of the four reports (total participant number = 152) meeting our eligibility criteria, three had point estimates suggesting small to moderate effect sizes favoring genuine therapy over sham, however, due to small sample sizes, all 95% confidence intervals (CIs) were wide and spanned the null. The fourth trial had yet to post results as of the submission date of this review. The limited overall number of eligible reports (and participants), large degree of inter-trial heterogeneity, and restricted span of ID populations with published/posted outcome data (i.e., PTSD and OCD) precluded a quantitative synthesis.
Discussion: The current literature suggests that EEG-NFB may induce specific effects in the treatment of some forms of IDs, however, the evidence is very limited. Ultimately, more randomized, double-blind, sham-controlled trials encompassing a wider array of ID populations are needed to determine the existence and, if present, degree of EEG-NFB specificity in the treatment of IDs.
Systematic Review Registration: [https://www.crd.york.ac.uk/prospero], identifier [CRD42020159702].
Introduction
Internalizing disorders (IDs), e.g., major depressive disorder (MDD), posttraumatic stress disorder (PTSD), and obsessive-compulsive disorder (OCD), are the most prevalent psychopathologies experienced worldwide (Demyttenaere et al., 2004; Kessler et al., 2005, 2007, 2009; Oakley-Browne et al., 2006) and can be broadly characterized by a proclivity to direct distress inwardly (Buchan et al., 2014; Carragher et al., 2015; Krueger and Eaton, 2015; Rhee et al., 2015; Kotov et al., 2017). Although effective for many, traditional frontline ID treatments (i.e., pharmacotherapy and/or psychotherapy) have significant short-comings including substantial long-term failure rates (Haller et al., 2015; James et al., 2015; Peters et al., 2016; Pinter et al., 2019), lack of access (Andrade et al., 2014; Schoenberg and David, 2014; Bandelow and Michaelis, 2015; Haller et al., 2015; Möller et al., 2016) and marked adverse side-effects (Tiller, 2013; Haller et al., 2015; Alvares et al., 2016; Möller et al., 2016; Pinter et al., 2019). Moreover, a decades long drought in the discovery of new compounds has prompted pharmaceutical companies abandon the neuropsychiatric space (Buzsáki and Watson, 2012) leading to appeals from around the world for innovative treatments (Flisher et al., 2007; Haller et al., 2015; Kris, 2018; Pinter et al., 2019).
With aberrations in the brain’s electrical activity well documented in IDs (Pizzagalli et al., 2002; Jokić-Begić and Begić, 2003; Alhaj et al., 2010; Iosifescu, 2011; Buzsáki and Watson, 2012; Wahbeh and Oken, 2013), closed-loop brain training of electrophysiological signals, also known as electroencephalography neurofeedback (EEG-NFB), has been touted as a possible solution. EEG-NFB is non-invasive form of biofeedback that teaches the brain to modify its function via a closed-loop brain-computer interface whereby an exogenous sensory stimulus (e.g., audible tone) is fed back to the participant in real-time following some pre-determined electrical activity recorded from the scalp (Collura, 2013; Marzbani et al., 2016; Sitaram et al., 2016; Arns et al., 2017; Orndorff-Plunkett et al., 2017). EEG-NFB is widely believed to work predominantly through a form of associative learning known as operant conditioning whereby the probability of some given (neural) behavior is modified via a temporally associated reinforcing stimulus (Enriquez-Geppert et al., 2017; Orndorff-Plunkett et al., 2017; Alkoby et al., 2018). Although the use of EEG-NFB for IDs in routine clinical psychiatric practice has yet to receive widespread support (Begemann et al., 2016; Arns et al., 2017; Omejc et al., 2019), there is substantial evidence that EEG-NFB might be efficacious (Schoenberg and David, 2014; Reiter et al., 2016; Van Der Kolk et al., 2016; Cheon et al., 2017; Noohi et al., 2017; Orndorff-Plunkett et al., 2017; Ros et al., 2017; Panisch and Hai, 2018; Askovic et al., 2019; Bell et al., 2019; Chiba et al., 2019; Wang et al., 2019; Tolin et al., 2020; Hou et al., 2021).
That said, skeptics assert that EEG-NFB’s efficacy derives exclusively from non-specific factors (e.g., expectations, demand characteristics, and context) based primarily on a collection of randomized, sham/placebo-controlled trials for attention deficit hyperactivity disorder (ADHD) which demonstrated comparable clinical improvements in both experimental and sham groups (Thibault et al., 2016, 2018; Schönenberg et al., 2017a,b; Ghaziri and Thibault, 2019; Arnold et al., 2021). EEG-NFB proponents’ most salient objection to this conclusion is that evidence of differential targeted EEG-learning (i.e., greater improvement in the trained electrophysiological variable(s) in genuine vs. sham EEG-NFB groups), considered by many to be essential for a valid evaluation of EEG-NFB’s specificity (Sherlin et al., 2011; Kerson, 2013; Arns et al., 2014; Holtmann et al., 2014; Zuberer et al., 2015; Szewczyk et al., 2018; Witte et al., 2018), was noticeably absent in the trials presented as evidence for wholly non-specific effects (Pigott et al., 2018; Trullinger et al., 2019). Remediation of this apparent shortcoming can be complicated, however, due to a lack of established guidelines for determining successful EEG-learning (Weber et al., 2020).
The aim of our review was to comprehensively evaluate all available randomized, double-blind, sham/placebo-controlled trials in an ID population for evidence of EEG-NFB specificity via differences in clinical outcomes (i.e., symptom rating scales) between genuine and sham EEG-NFB groups.
Materials and Methods
Registration and Protocol
This review was prospectively registered on the International Prospective Register of Systematic Reviews (PROSPERO) under the registration number: CRD42020159702. The protocol for this systematic review and meta-analysis has been published previously (Perez et al., 2021) and uploaded in PDF format to PROSPERO.
Eligibility Criteria
We considered all EEG-NFB reports involving humans with at least one ID diagnosis per the Diagnostic and Statistical Manual of Mental Disorders [DSM; (American Psychiatric Association [APA], 2013)] or the International Classification of Diseases [ICD; (World Health Organization [WHO], 2018)] with no exclusion by language, locality, ethnicity, age, or sex. Regarding outcome assessments, all reports were required to include data from at least one clinical rating scale assessing one or more core symptoms of the disorder(s) under investigation. To minimize bias and control for non-specific effects, we included only randomized, double-blind (participants and outcome assessors), sham/placebo-controlled (i.e., feedback contingent on either a random signal, the activity from a different person’s brain, or a signal from the participant’s own brain derived from a region unrelated to the condition under study) reports. Concomitant interventions were permitted provided they were identical for both active and sham groups.
Information Sources
Studies eligible for review were identified in a literature search from earliest dates within multiple databases (Table 1). Of note, we decided to remove database limit options from our protocol in order to broaden the scope of our searches. Databases were last searched on 23 November 2021. Considering the known importance of including unpublished data in systematic reviews (Trespidi et al., 2011), we also searched various clinical trial registries including ClinicalTrials.gov1, the World Health Organization’s International Clinical Trials Registry Platform (ICTRP)2, and the Australia New Zealand Clinical Trials Registry (ANZCTR)3 to identify completed, unpublished trials. Notably, the ICTRP indexes trial registrations from 17 registries around the world. All registries were last accessed on 23 November 2021. Additionally, reference lists of included articles and relevant systematic reviews were manually screened to identify additional studies.
Search Strategy
Literature search strategies were developed using medical subject heading (MeSH) and text words related to IDs and neurofeedback. The search strategies were developed by author TMP with guidance from the University of Otago’s Health Sciences librarian. A detailed account of the search strategy for each database and registry can be found in Supplementary Material.
Selection Process
TMP collated the list of possible records for inclusion and exported them from each database to EndNote (version X9.2) where duplicates were located using EndNote’s duplicate identification strategy (i.e., identifying references in a library of the same reference type with matching Author, Year, and Title fields) and then removed manually. Two independent reviewers (TMP and JM) screened titles and abstracts for eligibility. In cases of disagreement, consensus on articles to assess for eligibility was reached by discussion between TMP and JM. When disagreements couldn’t be resolved, a third team member (DBA) was enlisted to make the final decision. TMP and JM then independently assessed full-text reports appearing to meet the inclusion criteria or when there was any uncertainty. Again, in cases of disagreement, consensus on inclusion/exclusion was reached via discussion and, when needed, DBA was consulted to make the final decision. When necessary to resolve questions regarding eligibility, TMP sought additional information from study authors via a maximum of three electronic (i.e., email and/or ResearchGate) requests. Notably, two non-English language reports [(Biriukova et al., 2005; Eskandari et al., 2014)] were translated during our full-text eligibility assessments. One report [(Biriukova et al., 2005)] was entered into Google Translate by our team and the subsequent output was validated by the study’s last author. The other (Eskandari et al., 2014) was translated by a native speaker here at the University of Otago. Reasons for excluding trials were recorded. Neither TMP nor JM were blinded to the journal titles, trial authors, or institutions.
Data Collection Process
Data was extracted by TMP and independently verified by JM. In cases of disagreement, consensus was reached via discussion. For all three included reports, trial authors were successfully contacted via email for clarification of trial details and/or to obtain missing data.
Data Items
Our primary outcome of interest was clinician ratings or self/parent/teacher reports of ID-related symptomatology. In trials incorporating multiple domains (e.g., clinician ratings and self/parent/teacher reports), a single scale was selected based on a hierarchy (i.e., clinician > self > parent > teacher). When multiple rating scales within a given domain were assessed, the validated scale querying the most core/central feature(s) of the condition under study (as determined by our content expert PG) was selected. In cases where multiple values for a single scale (i.e., total vs. sub-scale scores) were assessed, the total scores were used. Notably, as it has been postulated that longer-term outcomes may help to clarify the issue of specificity (Van Doren et al., 2019), in trials with multiple post-treatment data collection time-points, scores obtained furthest from treatment termination were given preference. To date, standard EEG-NFB protocols have not been established for the treatment of IDs (Banerjee and Argáez, 2017), therefore, no protocols were excluded.
Study Risk of Bias Assessment
The risk of bias for each eligible report was assessed using the Cochrane Risk of Bias tool version 2 (RoB 2.0) for randomized trials which covers 5 domains (domain 1: risk of bias arising from the randomization process; domain 2: risk of bias due to deviations from the intended interventions; domain 3: risk of bias due to missing outcome data; domain 4: risk of bias in measurement of the outcome; domain 5: risk of bias in the selection of the reported result) (Sterne et al., 2019). Two reviewers (TMP and JM) independently applied the tool to each trial and recorded supporting information/justifications for risk of bias judgments (low, some concerns, high) in each domain. Further, an overall summary risk of bias judgment (low, some concerns, high) was made for each report determined by the highest risk of bias level across all 5 domains. All decisions were guided by the published criteria for judging the risk of bias (Higgins et al., 2021). If there was insufficient detail reported in the study, the original study investigators were contacted via email for more information. Disagreements were resolved in discussion and, in cases where consensus was not reached, a third team member (DBA) was enlisted to make the final decision.
Effect Measures
Because the included trials utilized different measurement scales to assess clinical outcomes, standardized mean differences (SMDs) and 95% confidence intervals (CIs) were calculated using Hedges’ adjusted g in RevMan (version 5.4.1) and presented in a forest plot. In our forest plot, an SMD of 0 is represented by a black vertical line with negative (left-sided) and positive (right-sided) values favoring sham and active groups, respectively. Each trial’s SMD point estimate and 95% CI are represented by a green vertical dashes and bilateral black horizontal lines, respectively. The SMD expresses the size of the intervention effect relative to the variability observed under the assumption that between-study standard deviation variations reflect differences in measurement scales rather than variability in the study populations or reliability in outcome measures (Deeks et al., 2021). SMDs of 0.2, 0.5, and 0.8 are generally interpreted to reflect small, medium, and large effects sizes, respectively (Cohen, 1988).
Synthesis Methods
The limited overall number of eligible reports (and participants), large degree of inter-trial heterogeneity, and restricted span of ID populations with published/posted outcome data (i.e., PTSD and OCD) precluded a robust quantitative synthesis investigating the potential for EEG-NFB specificity in the treatment of IDs.
Reporting Bias Assessment
To assess for reporting biases (e.g., selective non-publication and selective non-reporting of results), two independent reviewers (TMP and JM) searched various registries for unpublished trials as well as to compare published trial report outcomes to outcomes specified in their registrations to help guide ratings for domain 5 (i.e., risk of bias in the selection of the reported result) of the RoB 2.0. When registrations were unavailable, we compared the congruency of the section “Materials and Methods” and section “Results” for each trial. Disagreements were resolved in discussion and, when necessary, DBA was consulted to make the final decision.
Certainty Assessment
Two independent reviewers (TMP and JM) assessed the certainty in the body of the evidence as it related to the trials that contributed data to the meta-analysis using the Grading of Recommendations, Assessment, Development and Evaluations (GRADE). A certainty ranking of high (there is a lot of confidence that the true effect lies close to that of the estimated effect), moderate (the true effect is probably close to the estimated effect), low (the true effect might be markedly different from the estimated effect.), or very low (the true effect is likely to be substantially different from the estimated effect) was assigned by the software based on the reviewers assignments across a number of domains including study design (i.e., randomized trial or observational study), risk of bias (not serious, serious, or very serious), inconsistency (not serious, serious, or very serious), indirectness (not serious, serious, or very serious), imprecision (not serious, serious, or very serious). We used the methods and recommendation described in the GRADE handbook (Schünemann and Oxman, 2013). Disagreements were resolved in discussion and, when necessary, DBA was consulted to make the final decision. A GRADE profile (v2) was generated using GRADEpro GDT software (Evidence Prime, 2020). Where necessary, we provided explanations for our assignments using footnotes to aid the reader’s understanding of the results.
Results
Study Selection
Our search uncovered 8,887 records in databases, registries, and relevant reference lists (Figure 1). After duplicates were removed, we screened 6,405 records, from which we reviewed 17 full-text documents. Ultimately, four reports met our eligibility criteria.
Following full-text assessments, we excluded 13 studies from our review (Watson and Herder, 1980; Rice et al., 1993; Biriukova et al., 2005; Iranian Registry of Clinical Trials, 2008; Choi et al., 2011; Eskandari et al., 2014; Sadjadi and Hashemian, 2014; Hashemian and Sadjadi, 2015; Mennella et al., 2017; Noohi et al., 2017; Viereck et al., 2017; Esmaeeli, 2021; Hou et al., 2021) for various reasons (Figure 1) which included a lack of randomization (Watson and Herder, 1980), double-blinding ((Rice et al., 1993; Biriukova et al., 2005; Iranian Registry of Clinical Trials, 2008; Hou et al., 2021), sham EEG-NFB controls (Choi et al., 2011), ID diagnosis (Mennella et al., 2017) and (Viereck et al., 2017), or missing details regarding the control group and/or blinding (Eskandari et al., 2014; Sadjadi and Hashemian, 2014; Hashemian and Sadjadi, 2015; Noohi et al., 2017; Esmaeeli, 2021). With respect to these latter five trials excluded due to missing information, one failed to provide the trialist’s contact information (Esmaeeli, 2021), whereas, for the other four, we made multiple attempts to contact the authors via email and/or ResearchGate, however, they failed to respond to our requests.
Study Characteristics
An detailed overview of the eligible trials (ClinicalTrials.gov, 2000a; Nederlands Trial Register, 2004; Kopřivová et al., 2013; Nicholson et al., 2020) is presented in Table 2. As can be seen, there is significant clinical (e.g., population) and methodological (e.g., clinical outcome scale) heterogeneity across trials.
Risk of Bias in Studies
We used the RoB 2.0 to assess risk of bias for the eligible trials with posted/published outcome data (Figure 2). The overall risk of bias was deemed moderate (i.e., “some concerns”) for two of the trials (Kopřivová et al., 2013; Nicholson et al., 2020) and high for the third (ClinicalTrials.gov, 2000a) suggesting some methodological heterogeneity across trials. Concerns included a lack of pre-registered protocols and/or analysis plans with which to compare the final reports and outcomes.
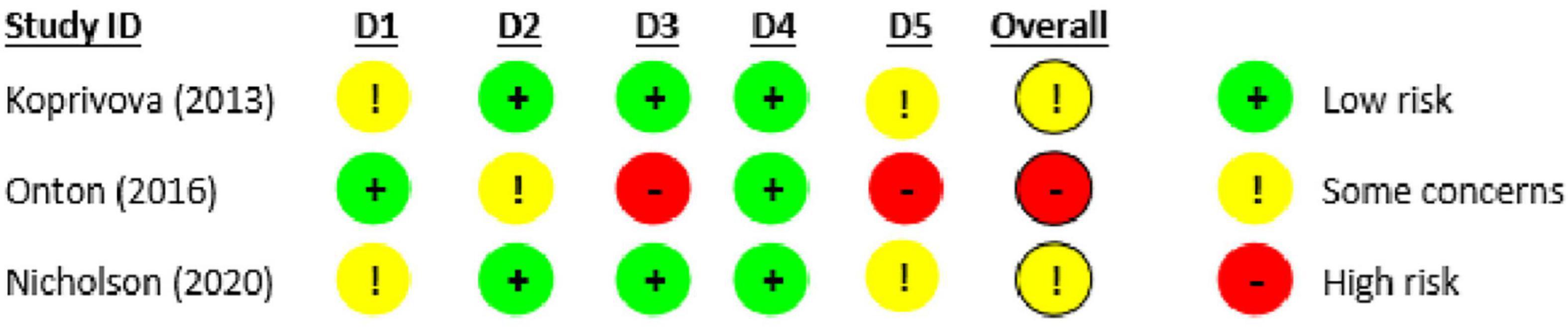
Figure 2. Risk of bias in eligible studies with published/posted outcome data using version 2 of the Cochrane risk-of-bias tool for randomized trials (RoB 2.0).
Results of Individual Studies
Figure 3 displays summary statistics [CFB mean and SD, sample size (Total)] for the sham and genuine EEG-NFB groups along with the SMD and 95% CI of the continuous outcome (reduction in internalizing symptoms) for each study. Of note, one eligible trial [i.e., (Nederlands Trial Register, 2004)] is absent due to a failure to post/publish results. Unfortunately, the lead trialist was unwilling to share unpublished data. For all trials with final results, trialists were successfully contacted via email for additional data not provided in the original report. Baseline outcome measures were reported to be equivalent between genuine and sham groups in the two published studies (Kopřivová et al., 2013; Nicholson et al., 2020) and were not reported in the unpublished trial (ClinicalTrials.gov, 2000a). Across trials, point estimates consistently demonstrated small to moderate effect sizes favoring genuine over sham EEG-NFB, however, in all cases 95% CIs were relatively wide and spanned the null line. Specifically, SMDs [95% CIs] were 0.42 [−0.52, 1.37] (Kopřivová et al., 2013), 0.46 [−0.11, 1.03] (ClinicalTrials.gov, 2000a), and 0.33 [−0.33, 0.99] (Nicholson et al., 2020). The two published trials [i.e., (Nicholson et al., 2020) and (Kopřivová et al., 2013)] did assess for differential targeted EEG-learning with group point estimates suggesting a trend toward greater improvements (i.e., reductions) in the trained EEG variable(s) in the genuine EEG-NFB groups relative to sham, however, correlation analyses between changes in the targeted EEG variable(s) and clinical outcome measures were not performed.

Figure 3. Forest plot showing standardized mean differences in change-from-baseline scores between sham and genuine EEG-neurofeedback using a Hedges’ (adjusted) g.
Reporting Biases
Overall, we feel the risk of selective outcome reporting was low as all trials’ methods and results sections were congruent and well-accepted scales were generally incorporated for the conditions under study, however, there remains a risk of selective analysis reporting due to a lack of pre-specified analysis plans per trial registry records or published protocols. Additionally, we have concerns surrounding publication bias as we were unable to include the outcome data from one of the completed trials meeting our eligibility criteria [i.e., (Nederlands Trial Register, 2004)] because they have yet to be made publicly available despite being “terminated [in 2017] because of disappointing mid trial results” (personal communication with the lead trialist, 7 July 2020).
Certainty of Evidence
As seen in the GRADE profile (Table 3), although the certainty of the evidence is initially rated “high” due to the incorporation of the three RCTs with published/posted outcomes, it was subsequently downgraded three steps to “very low” due to (1) “serious” concerns surrounding the cumulative risk of bias, (2) “serious” concerns regarding the imprecision (i.e., wide 95% CIs), and (3) “strongly suspected” publication bias (i.e., no published/posted outcomes from the fourth eligible trial).
Discussion
To our knowledge, this is the first systematic review exploring the potential for EEG-NFB specificity (i.e., specific effects) in ID populations. Of the four eligible reports identified, three (2 PTSD and 1 OCD) trials provided outcome data with point estimates suggesting EEG-NFB specificity, however, effect sizes were modest with wide 95% CIs spanning the null. The fourth trial, which recruited people with MDD, has yet to publish its results although, according to the lead trialist, the results were “disappointing.” Notably, it could be argued that the protocols undertaken in the eligible trials may have mitigated between-group differences and the overall effect size. More specifically, it has been reported that individualized training is typical in clinical settings and may lead to augmented effects and better outcomes (Hammond, 2010; Omejc et al., 2019; Weber et al., 2020), however, only one of the trials utilized an individualized training paradigm insofar as the targeted frequency or frequency band was based on individual deviations from a normative sample. Furthermore, it has been postulated that non-specific (i.e., placebo) effects are transient, therefore longer term follow-ups serve to better elucidate specificity (Van Doren et al., 2019) yet only one of the eligible trials incorporated any follow-up (i.e., 3 months). Although highly speculative, against this general backdrop of standardized protocols and lack of extended follow ups, theoretically the effect sizes may have been downwardly biased.
Some limitations of the current review should be noted. Firstly, although we had no exclusions by language, we may have unintentionally overlooked some non-English language trials meeting our criteria considering the fact that coverage bias (i.e., systematic exclusion of journals from certain countries and/or in certain languages) has been reported in some of the databases that were utilized (Pilkington et al., 2005). That said, the exclusion of non-English language publications from quantitative reviews of clinical interventions has been shown to have little-to-no effect on results and conclusions (Nussbaumer-Streit et al., 2020). Secondly, although two of the trials did assess for differential group EEG-learning (i.e., reduction in the power of the targeted frequency band) with point estimates in both suggesting greater improvements in the genuine group relative to sham, neither correlated this learning with clinical outcomes. Thirdly, outcome data were only available for PTSD and OCD populations, thereby excluding information on the most prevalent IDs (i.e., anxiety and depressive disorders) and limiting the generalizability of our findings. Lastly, due to the small number and extreme heterogeneity of eligible trials, a proper meta-analysis could not be undertaken. A major potential contributor to these latter two limitations was that many candidate trials failed to provide sufficient details necessitating requests for additional information and, in many cases, exclusion of their data due to non-responsive trialists. Reporting issues in RCTs are widespread (Moher et al., 2012) which have prompted skeptics and proponents alike to come together to publish a consensus paper on the reporting and design of neurofeedback studies (Ros et al., 2020). It is our hope that these guidelines will be widely adopted in the field and incorporated into future publications. Additionally, the lack of eligible reports, small participant numbers, and lack of replication may be explained, in part, by the significant investment in resources (i.e., time, human, and monetary) required to undertake EEG-NFB trials of this nature although this too is highly speculative.
Neuropsychiatric disorders are among the most common causes of morbidity and mortality (Kessler et al., 2009) with rates markedly increasing world-wide in recent years (Duffy et al., 2019; Keyes et al., 2019; Twenge et al., 2019; Haidt and Allen, 2020; Pfeifer and Allen, 2021). Among them, IDs, which are characterized by distress experienced inwardly (Cosgrove et al., 2011; Buchan et al., 2014), are the most prevalent. Recently, a government inquiry here in Aotearoa New Zealand has shed light on the shortcomings of traditional front-line treatments (i.e., pharmacotherapy and/or psychotherapy) and urged wider implementation of alternative approaches to the treatment of mental health problems (Kris, 2018). Likewise, scientists abroad have called for more research into “novel interventions that may be based on altering plasticity or returning circuitry rather than neurotransmitter pharmacology” (Insel and Wang, 2010). EEG-NFB is a non-invasive treatment that appears to be generally safe and efficacious when directed by a qualified practitioner (Hammond and Kirk, 2008), however, despite over 50 years since its inception, questions regarding the nature of those effects abound. Specifically, there is much controversy surrounding the existence of EEG-NFB specificity [i.e., specific effects deriving from modulation of the EEG variable(s) of interest]. The current systematic review suggests that genuine EEG-NFB may induce specific effects in at least some ID populations (i.e., PTSD and OCD). That said, the evidence is very limited, thereby warranting more randomized, double-blind, sham-controlled studies to verify the existence and, if present, degree of specificity across the spectrum of IDs. Encouragingly, we are aware of a number of registered randomized, double-blind, sham-controlled EEG-NFB trials in ID populations [i.e., (ClinicalTrials.gov, 2000b; Australian New Zealand Clinical Trials Registry, 2005a,b)] which, when incorporated into a future meta-analysis, may help bring clarity to this intriguing and important topic.
Data Availability Statement
The original contributions presented in the study are included in the article/Supplementary Material, further inquiries can be directed to the corresponding author.
Author Contributions
TP is the guarantor and drafter of the manuscript and developed and implemented the search strategies. PG provided expertise on mental health disorders. JM assisted with the screening, data extraction, and assessments. All authors contributed to the development of the selection criteria, the risk of bias assessment strategy and data extraction criteria with guidance from the Health Sciences Librarian and read, provided feedback, and approved the final manuscript.
Funding
This systematic review is part of a Ph.D. thesis supported by the Department of Surgical Sciences, University of Otago, Dunedin, New Zealand.
Conflict of Interest
The authors declare that the research was conducted in the absence of any commercial or financial relationships that could be construed as a potential conflict of interest.
The reviewer PN declared a past co-authorship with several of the authors DA and DD to the handling editor.
Publisher’s Note
All claims expressed in this article are solely those of the authors and do not necessarily represent those of their affiliated organizations, or those of the publisher, the editors and the reviewers. Any product that may be evaluated in this article, or claim that may be made by its manufacturer, is not guaranteed or endorsed by the publisher.
Acknowledgments
First, we would like to acknowledge the University of Otago Health Sciences Librarian, Christy Ballard, for her help with designing the search strategies. Second, we would like to thank Mark Trullinger, who graciously agreed to proofread and provide feedback on the manuscript. Lastly, we are grateful to all the trialists who responded to our requests for additional information.
Supplementary Material
The Supplementary Material for this article can be found online at: https://www.frontiersin.org/articles/10.3389/fnins.2022.821136/full#supplementary-material
Abbreviations
ALI, anxiety level index; CAPS, Clinician Administered PTSD Scale; CFB, change-from-baseline; EEG-NFB, electroencephalography neurofeedback; GRADE, grading of recommendationsassessmentdevelopment and evaluations; IC, independent component; ICTRP, World Health Organization’s International Clinical Trials Registry Platform; ANZCTR, Australia New Zealand Clinical Trials Registry; IDs, internalizing disorders; MDD, major depressive disorder; OCD, obsessive-compulsive disorder; PI, post-intervention; PROSPERO, international prospective register of systematic reviews; PTSD, post-traumatic stress disorder; RoB 2.0, Cochrane Risk of Bias tool version 2; SMD, standardized mean difference; Y-BOCS, Yale-Brown Obsessive Compulsive Scale.
Footnotes
References
Alhaj, H., Wisniewski, G., and McAllister-Williams, R. H. (2010). The use of the EEG in measuring therapeutic drug action: focus on depression and antidepressants. J. Psychopharmacol. 25, 1175–1191.
Alkoby, O., Abu-Rmileh, A., Shriki, O., and Todder, D. (2018). Can we predict who will respond to neurofeedback? A review of the inefficacy problem and existing predictors for successful EEG neurofeedback learning. Neuroscience 378, 155–164. doi: 10.1016/j.neuroscience.2016.12.050
Alvares, G. A., Quintana, D. S., Hickie, I. B., and Guastella, A. J. (2016). Autonomic nervous system dysfunction in psychiatric disorders and the impact of psychotropic medications: a systematic review and meta-analysis. J. Psychiatry Neurosci. 41, 89–104. doi: 10.1503/jpn.140217
American Psychiatric Association [APA] (2013). American Psychiatric Association DSMTF. Diagnostic and Statistical Manual of Mental Disorders : DSM-5, 5th Edn. Washington, D.C: American Psychiatric Publishing.
Andrade, L. H., Alonso, J., Mneimneh, Z., Wells, J. E., Al-Hamzawi, A., Borges, G., et al. (2014). Barriers to mental health treatment: results from the WHO World Mental Health surveys. Psychol. Med. 44, 1303–1317. doi: 10.1017/S0033291713001943
Arnold, L. E., Arns, M., Barterian, J., Bergman, R., Black, S., Conners, C. K., et al. (2021). Double-blind placebo-controlled randomized clinical trial of neurofeedback for attention-Deficit/Hyperactivity Disorder With 13 Month Follow-up. J. Am. Acad. Child Adolesc. Psychiatry 60, 841–855. doi: 10.1016/j.jaac.2020.07.906
Arns, M., Batail, J. M., Bioulac, S., Congedo, M., Daudet, C., Drapier, D., et al. (2017). Neurofeedback: one of today’s techniques in psychiatry? Encéphale 43, 135–145. doi: 10.1016/j.encep.2016.11.003
Arns, M., Heinrich, H., and Strehl, U. (2014). Evaluation of neurofeedback in ADHD: the long and winding road. Biol. Psychol. 95, 108–115.
Askovic, M., Watters, A. J., Coello, M., Aroche, J., Harris, A. W. F., and Kropotov, J. (2019). Evaluation of neurofeedback for posttraumatic stress disorder related to refugee experiences using self-report and cognitive ERP measures. Clin. EEG Neurosci. 51, 79–86. doi: 10.1177/1550059419849170
Australian New Zealand Clinical Trials Registry (2005a). A Controlled Study to Assess the Therapeutic Effects of Neurofeedback Training in Depression. [Internet]. Identifier ACTRN12619001487101. Available online at: http://www.anzctr.org.au/Trial/Registration/TrialReview.aspx?id=375899&showOriginal=true&isReview=true (accessed October 29, 2019).
Australian New Zealand Clinical Trials Registry (2005b). Resting State Networks and Infraslow Neurofeedback in Anxiety and Depression: A Randomised, Double-Blind, Sham-Controlled Trial. [Internet]. Identifier ACTRN12619001428156. Available online at: http://www.anzctr.org.au/Trial/Registration/TrialReview.aspx?id=378406&isReview=true (accessed October 15, 2019).
Bandelow, B., and Michaelis, S. (2015). Epidemiology of anxiety disorders in the 21st century. Dialogues Clin. Neurosci. 17:327. doi: 10.31887/DCNS.2015.17.3/bbandelow
Banerjee, S., and Argáez, C. (2017). Neurofeedback and Biofeedback for Mood and Anxiety Disorders: A Review of Clinical Effectiveness and Guidelines. Ottawa, ON: Canadian Agency for Drugs and Technologies in Health.
Begemann, M. J., Florisse, E. J., van Lutterveld, R., Kooyman, M., and Sommer, I. E. (2016). Efficacy of EEG neurofeedback in psychiatry: a comprehensive overview and meta-analysis. Transl. Brain Rhythmicity. 1, 19–29.
Bell, A. N., Moss, D., and Kallmeyer, R. J. (2019). Healing the neurophysiological roots of trauma: a controlled study examining loreta z-score neurofeedback and HRV biofeedback for chronic PTSD. NeuroRegulation 6, 54–70.
Biriukova, E. N., Timofeev, I. N., and Mosolov, S. N. (2005). P.3.041 Clinical and neurophysiologicalefficacy of neurofeedback in the combined therapy of anxiety disorders resistant to psychopharmacotherapy. Eur. Neuropsychopharmacol. 15, S162–S163.
Buchan, H., Sunderland, M., Carragher, N., Batterham, P., and Slade, T. (2014). Investigating age-related differences in responses to screening items for internalising disorders in three national surveys. J. Affect. Disord. 15, 229–236. doi: 10.1016/j.jad.2013.09.015
Buzsáki, G., and Watson, B. O. (2012). Brain rhythms and neural syntax: implications for efficient coding of cognitive content and neuropsychiatric disease. Dialogues Clin. Neurosci. 14, 345–367. doi: 10.31887/DCNS.2012.14.4/gbuzsaki
Carragher, N., Krueger, R., Eaton, N., and Slade, T. (2015). Disorders without borders: current and future directions in the meta-structure of mental disorders. Soc. Psychiatry Psychiatr. Epidemiol. 50, 339–350. doi: 10.1007/s00127-014-1004-z
Cheon, E. J., Choi, J. H., Lee, G. W., Koo, B. H., Seo, W. S., Kim, H. G., et al. (2017). P.2.f.009 - Neurofeedback treatment on depressive symptoms and functional recovery and brain-derived neurotrophic factor in treatment-resistant major depression. Eur. Neuropsychopharmacol. 27:S851. doi: 10.3346/jkms.2019.34.e287
Chiba, T., Kanazawa, T., Koizumi, A., Ide, K., Taschereau-Dumouchel, V., Boku, S., et al. (2019). Current status of neurofeedback for post-traumatic stress disorder: a systematic review and the possibility of decoded neurofeedback.(Report). Front. Hum. Neurosci. 13:233. doi: 10.3389/fnhum.2019.00233
Choi, S. W., Chi, S. E., Chung, S. Y., Kim, J. W., Ahn, C. Y., and Kim, H. T. (2011). Is alpha wave neurofeedback effective with randomized clinical trials in depression? A pilot study. Neuropsychobiology 63, 43–51. doi: 10.1159/000322290
ClinicalTrials.gov (2000a). EEG Biofeedback Therapy as an Adjunct Treatment for PTSD [Internet]. Identifier NCT01591408. Available online at: https://clinicaltrials.gov/ct2/show/NCT01591408 (accessed May 4, 2012).
ClinicalTrials.gov (2000b). Efficacy of Electroencephalography (EEG) Neurofeedback (NF) for the Treatment of Anxiety Disorder. [Internet]. Identifier NCT04562324. Available online at: https://clinicaltrials.gov/ct2/show/NCT04562324 (accessed September 24, 2020).
Cohen, J. (1988). Statistical Power Analysis for the Behavioral Sciences. Hillsdale, NJ: Lawrence Erlbaum Associates, Publishers. Conner, BE (1988). The Box in the Barn. Columbus: Highlights for Children Inc.
Cosgrove, V. E., Rhee, S. H., Gelhorn, H. L., Boeldt, D., Corley, R. C., Ehringer, M. A., et al. (2011). Structure and etiology of co-occurring internalizing and externalizing disorders in adolescents. J. Abnorm. Child Psychol. 39, 109–123. doi: 10.1007/s10802-010-9444-8
Deeks, J. J., Higgins, J. P. T., and Altman, D. G. (2021). “Analysing data and undertaking meta-analyses,” in Cochrane Handbook for Systematic Reviews of Interventions version 62 [Internet], eds J.P. T. Higgins, J. Thomas, J. Chandler, M. Cumpston, T. Li, M. J. Page, et al. (London: Cochrane).
Demyttenaere, K., Bruffaerts, R., Posada-Villa, J., Gasquet, I., Kovess, V., Lepine, J. P., et al. (2004). Prevalence, severity, and unmet need for treatment of mental disorders in the World Health Organization World Mental Health Surveys. JAMA 291, 2581–2590. doi: 10.1001/jama.291.21.2581
Duffy, M. E., Twenge, J. M., and Joiner, T. E. (2019). Trends in mood and anxiety symptoms and suicide-related outcomes among U.S. Undergraduates, 2007–2018: evidence from two national surveys. J. Adolesc. Health 65, 590–598. doi: 10.1016/j.jadohealth.2019.04.033
Enriquez-Geppert, S., Huster, R. J., and Herrmann, C. S. (2017). EEG-neurofeedback as a tool to modulate cognition and behavior: a review tutorial.(Report)(Author abstract). Front. Hum. Neurosci. 11:51. doi: 10.3389/fnhum.2017.00051
Eskandari, Z., Taremian, F., Nazari, M. A., Bakhtiari, M., Momtazi, S., and Rezae, M. (2014). Effectiveness of neurofeedback treatment to decrease severity symptoms in major depression disorder. J. Zanjan Univers. Med. Sci. Health Serv. 22, 86–95.
Esmaeeli, S. (2021). The effectiveness of neuro feedback training on the working-memory of people with obsessive-compulsive disorder symptoms. Eur. J. Mol. Clin. Med. 8, 1795–1803.
Evidence Prime, I. (2020). GRADEpro GDT: GRADEpro Guideline Development Tool [Software]. Hamilton, ON: McMaster University.
Flisher, A., Lund, C., Patel, V., Saxena, S., Thornicroft, G., and Tomlinson, M. (2007). Scale up services for mental disorders: a call for action. Lancet 370, 1241–1252. doi: 10.1016/S0140-6736(07)61242-2
Ghaziri, J., and Thibault, R. T. (2019). “19 - Neurofeedback: an inside perspective,” in Casting Light on the Dark Side of Brain Imaging, eds A. Raz and R. T. Thibault (New York, NY: Academic Press), 113–116.
Haidt, J., and Allen, N. (2020). Scrutinizing the Effects of Digital Technology on Mental Health. Berlin: Nature Publishing Group.
Haller, S. P. W., Cohen Kadosh, K., Scerif, G., and Lau, J. Y. F. (2015). Social anxiety disorder in adolescence: how developmental cognitive neuroscience findings may shape understanding and interventions for psychopathology. Dev. Cogn. Neurosci. 13, 11–20. doi: 10.1016/j.dcn.2015.02.002
Hammond, D. (2010). The need for individualization in neurofeedback: heterogeneity in QEEG patterns associated with diagnoses and symptoms. Appl. Psychophysiol. Biofeedback 35, 31–36. doi: 10.1007/s10484-009-9106-1
Hammond, D. C., and Kirk, L. (2008). First, do no harm: adverse effects and the need for practice standards in neurofeedback. J. Neurother. 12, 79–88.
Hashemian, P., and Sadjadi, S. A. (2015). Evaluation of neurofeedback therapy in adolescents with major depressive disorder who take fluoxetine. Afr. J. Psychiatry 18, 180–182.
Higgins, J. P. T., Savovic, J., Page, M. J., Elbers, R. G., and Sterne, J. A. C. (2021). “Assessing risk of bias in a randomized trial,” in Cochrane Handbook for Systematic Reviews of Interventions Version 62 [Internet], eds J.P. T. Higgins, J. Thomas, J. Chandler, M. Cumpston, T. Li, M. J. Page, et al. (London: Cochrane).
Holtmann, M., Sonuga-Barke, E., Cortese, S., and Brandeis, D. (2014). Neurofeedback for ADHD: a review of current evidence. Child Adolesc. Psychiatr. Clin. North Am. 23, 789–806. doi: 10.1016/j.chc.2014.05.006
Hou, Y., Zhang, S., Li, N., Huang, Z., Wang, L., and Wang, Y. (2021). Neurofeedback training improves anxiety trait and depressive symptom in GAD. Brain Behav. 11:e02024. doi: 10.1002/brb3.2024
Iosifescu, D. (2011). Electroencephalography-derived biomarkers of antidepressant response. Harvard Rev. Psychiatry 19, 144–154. doi: 10.3109/10673229.2011.586549
Iranian Registry of Clinical Trials (2008). The Effect of Neurofeedback with Pharmacotherapy on Cognitive Ability and Executive Function in Patients with Obsessive – Compulsive Disorder [Internet]. Identifier IRCT2015030321313N1. Available online at: https://en.irct.ir/trial/18706 (accessed March 11, 2015).
James, A. C., James, G., Cowdrey, F. A., Soler, A., and Choke, A. (2015). Cognitive behavioural therapy for anxiety disorders in children and adolescents. Cochrane Database Syst. Rev. 2015:CD004690.
Jokić-Begić, N., and Begić, D. (2003). Quantitative electroencephalogram (qEEG) in combat veterans with post-traumatic stress disorder (PTSD). Nordic J. Psychiatry 57, 351–355. doi: 10.1080/08039480310002688
Kerson, C. A. (2013). Proposed Multisite Double-Blind Randomized Clinical Trial of Neurofeedback for ADHD: need, Rationale, and Strategy. J. Attention Disord. 17, 420–436. doi: 10.1177/1087054713482580
Kessler, R. C., Aguilar-Gaxiola, S., Alonso, J., Chatterji, S., Lee, S., Ormel, J., et al. (2009). The global burden of mental disorders: an update from the WHO World Mental Health (WMH) surveys. Epidemiol. Psichiatr. Soc. 18, 23–33. doi: 10.1017/s1121189x00001421
Kessler, R. C., Angermeyer, M., Anthony, J. C., De Graaf, R., Demyttenaere, K., Gasquet, I., et al. (2007). Lifetime prevalence and age-of-onset distributions of mental disorders in the World Health Organization’s World Mental Health Survey Initiative. World psychiatry 6:168.
Kessler, R. C., Berglund, P., Demler, O., Jin, R., Merikangas, K. R., and Walters, E. E. (2005). Lifetime prevalence and age-of-onset distributions of DSM-IV disorders in the National Comorbidity Survey Replication. Arch. Gen. Psychiatry 62:593. doi: 10.1001/archpsyc.62.6.593
Keyes, K. M., Dahsan, G., Patrick, M. O. M., Hamilton, A., and Schulenberg, J. (2019). Recent increases in depressive symptoms among US adolescents: trends from 1991 to 2018. Soc. Psychiatry Psychiatr. Epidemiol. 54, 987–996. doi: 10.1007/s00127-019-01697-8
Kopřivová, J., Congedo, M., Raszka, M., Praško, J., Brunovskı, M., and Horáèek, J. (2013). Prediction of treatment response and the effect of independent component neurofeedback in obsessive-compulsive disorder: a randomized, sham-controlled, double-blind study. Neuropsychobiology 67, 210–223. doi: 10.1159/000347087
Kotov, R., Krueger, R. F., Watson, D., Achenbach, T. M., Althoff, R. R., Bagby, R. M., et al. (2017). The Hierarchical Taxonomy of Psychopathology (HiTOP): a dimensional alternative to traditional nosologies. J. Abnorm. Psychol. 126, 454–477. doi: 10.1037/abn0000258
Kris, N. (2018). ‘Once in a Generation’: The Crucial Passages from the Mental Health Inquiry. Wellington: Government Inquiry into Mental Health and Addiction.
Krueger, R. F., and Eaton, N. R. (2015). Transdiagnostic factors of mental disorders. World Psychiatry 14, 27–29.
Marzbani, H., Marateb, H. R., and Mansourian, M. (2016). Neurofeedback: a comprehensive review on system design, methodology and clinical applications. Basic Clin. Neurosci. 7:143. doi: 10.15412/J.BCN.03070208
Mennella, R., Patron, E., and Palomba, D. (2017). Frontal alpha asymmetry neurofeedback for the reduction of negative affect and anxiety. Behav. Res. Ther. 92, 32–40. doi: 10.1016/j.brat.2017.02.002
Moher, D., Hopewell, S., Schulz, K. F., Montori, V., Gøtzsche, P. C., Devereaux, P., et al. (2012). CONSORT 2010 explanation and elaboration: updated guidelines for reporting parallel group randomised trials. Int. J. Surgery. 10, 28–55. doi: 10.1016/j.ijsu.2011.10.001
Möller, H.-J., Bandelow, B., Volz, H.-P., Barnikol, U. B., Seifritz, E., and Kasper, S. (2016). The relevance of ‘mixed anxiety and depression’ as a diagnostic category in clinical practice. Eur. Arch. Psychiatry Clin. Neurosci. 266, 725–736. doi: 10.1007/s00406-016-0684-7
Nederlands Trial Register (2004). A Randomized Controlled Trial into the Efficacy of Neurofeedback for Treatment of Major Depressive Disorder [Internet]. Identifier NL4649. Available online at: https://www.trialregister.nl/trial/4649 (accessed September 17, 2014).
Nicholson, A. A., Ros, T., Densmore, M., Frewen, P. A., Neufeld, R. W. J., Théberge, J., et al. (2020). A randomized, controlled trial of alpha-rhythm EEG neurofeedback in posttraumatic stress disorder: a preliminary investigation showing evidence of decreased PTSD symptoms and restored default mode and salience network connectivity using fMRI. NeuroImage 28:102490. doi: 10.1016/j.nicl.2020.102490
Noohi, S., Miraghaie, A. M., and Arabi, A. (2017). Effectiveness of neuro-feedback treatment with alpha/theta method on PTSD symptoms and their executing function. Biomed. Res. 28, 2019–2027.
Nussbaumer-Streit, B., Klerings, I., Dobrescu, A. I., Persad, E., Stevens, A., Garritty, C., et al. (2020). Excluding non-English publications from evidence-syntheses did not change conclusions: a meta-epidemiological study. J. Clin. Epidemiol. 118, 42–54. doi: 10.1016/j.jclinepi.2019.10.011
Oakley-Browne, M., Wells, J. E., and Scott, K. M. (2006). Te Rau Hinengaro the New Zealand Mental Health Survey. Wellington, NZ: Ministry of Health.
Omejc, N., Rojc, B., Battaglini, P. P., and Marusic, U. (2019). Review of the therapeutic neurofeedback method using electroencephalography: EEG Neurofeedback. Bosnian J. Basic Med. Sci. 19:213. doi: 10.17305/bjbms.2018.3785
Orndorff-Plunkett, F., Singh, F., Aragón, O., and Pineda, J. (2017). Assessing the effectiveness of neurofeedback training in the context of clinical and social neuroscience. Brain Sci. 7:95. doi: 10.3390/brainsci7080095
Panisch, L. S., and Hai, A. H. (2018). The effectiveness of using neurofeedback in the treatment of post-traumatic stress disorder: a systematic review. Trauma Violence Abuse 21, 541–550. doi: 10.1177/1524838018781103
Perez, T. M., Glue, P., Adhia, D. B., Mathew, J., and DeRidder, D. (2021). Is there evidence for EEG-neurofeedback specificity in the treatment of internalizing disorders? A protocol for a systematic review and meta-analysis. NeuroRegulation 8:22. doi: 10.15540/nr.8.1.22
Peters, S. K., Dunlop, K., and Downar, J. (2016). Cortico-striatal-thalamic loop circuits of the salience network: a central pathway in psychiatric disease and treatment. Front. Syst. Neurosci. 10:104. doi: 10.3389/fnsys.2016.00104
Pfeifer, J. H., and Allen, N. B. (2021). Puberty initiates cascading relationships between neurodevelopmental, social, and internalizing processes across adolescence. Biol. Psychiatry 89, 99–108. doi: 10.1016/j.biopsych.2020.09.002
Pigott, H. E., Cannon, R., and Trullinger, M. (2018). The fallacy of sham-controlled neurofeedback trials: a reply to thibault and colleagues (2018). J. Attention Disord. 25, 448–457. doi: 10.1177/1087054718790802
Pilkington, K., Boshnakova, A., Clarke, M., and Richardson, J. (2005). “No language restrictions” in database searches: what does this really mean? J. Altern. Complement Med. 11, 205–207. doi: 10.1089/acm.2005.11.205
Pinter, A., Szatmari, S. Jr., Horvath, T., Penzlin, A. I., Barlinn, K., Siepmann, M., et al. (2019). Cardiac dysautonomia in depression - heart rate variability biofeedback as a potential add-on therapy. Neuropsychiatr. Dis. Treatment 15, 1287–1310. doi: 10.2147/NDT.S200360
Pizzagalli, D. A., Nitschke, J. B., Oakes, T. R., Hendrick, A. M., Horras, K. A., Larson, C. L., et al. (2002). Brain electrical tomography in depression: the importance of symptom severity, anxiety, and melancholic features. Biol. Psychiatry 52, 73–85. doi: 10.1016/s0006-3223(02)01313-6
Reiter, B. K., Andersen, B. S., and Carlsson, B. J. (2016). Neurofeedback treatment and posttraumatic stress disorder: effectiveness of neurofeedback on posttraumatic stress disorder and the optimal choice of protocol. J. Nervous Ment. Dis. 204, 69–77. doi: 10.1097/NMD.0000000000000418
Rhee, S. H., Lahey, B. B., and Waldman, I. D. (2015). Comorbidity among dimensions of childhood psychopathology: converging evidence from behavior genetics. Child. Dev. Perspect. 9, 26–31. doi: 10.1111/cdep.12102
Rice, K. M., Blanchard, E. B., and Purcell, M. (1993). Biofeedback treatments of generalized anxiety disorder: preliminary results. Biofeedback Self Regul. 18, 93–105. doi: 10.1007/BF01848110
Ros, T., Enriquez-Geppert, S., Zotev, V., Young, K. D., Wood, G., Whitfield-Gabrieli, S., et al. (2020). Consensus on the Reporting and Experimental Design of Clinical and Cognitive-Behavioural Neurofeedback Studies (CRED-nf Checklist). Oxford: Oxford University Press.
Ros, T., Frewen, P., Théberge, J., Michela, A., Kluetsch, R., Mueller, A., et al. (2017). Neurofeedback tunes scale-free dynamics in spontaneous brain activity. Cereb. Cortex 27, 4911–4922. doi: 10.1093/cercor/bhw285
Sadjadi, S. A., and Hashemian, P. (2014). Effectiveness of neurofeedback therapy in children with separation anxiety disorder. Afr. J. Psychiatry 17:10000149.
Schoenberg, P., and David, A. (2014). Biofeedback for psychiatric disorders: a systematic review. Appl. Psychophysiol. Biofeedback 39, 109–135. doi: 10.1007/s10484-014-9246-9
Schönenberg, M., Wiedemann, E., Schneidt, A., Scheeff, J., Logemann, A., Keune, P. M., et al. (2017b). Neurofeedback, sham neurofeedback, and cognitive-behavioural group therapy in adults with attention-deficit hyperactivity disorder: a triple-blind, randomised, controlled trial. Lancet Psychiatry 4, 673–684. doi: 10.1016/S2215-0366(17)30291-2
Schönenberg, M., Wiedemann, E., Schneidt, A., Scheeff, J., Logemann, A., Keune, P. M., et al. (2017a). Confusion regarding operant conditioning of the EEG Authors’ reply. Lancet Psychiatry 4, 897–898. doi: 10.1016/S2215-0366(17)30437-6
Schünemann, H. B. J., and Oxman, A. (eds) (2013). GRADE Handbook for Grading Quality of Evidence and Strength of Recommendation. Hamilton, ON: McMaster University.
Sherlin, L. H., Arns, M., Lubar, J., Heinrich, H., Kerson, C., Strehl, U., et al. (2011). Neurofeedback and basic learning theory: implications for research and practice. J. Neurother. 15, 292–304. doi: 10.1080/10874208.2011.623089
Sitaram, R., Ros, T., Stoeckel, L., Haller, S., Scharnowski, F., Lewis-Peacock, J., et al. (2016). Closed-loop brain training: the science of neurofeedback. Nat. Rev. Neurosci. 18:86. doi: 10.1038/nrn.2016.164
Sterne, J. A. C., Savovic, J., Page, M. J., Elbers, R. G., Blencowe, N. S., Boutron, I., et al. (2019). RoB 2: a revised tool for assessing risk of bias in randomised trials. BMJ 366:l4898. doi: 10.1136/bmj.l4898
Szewczyk, R. Ł, Ratomska, M., and Jaśkiewicz, M. (eds) (2018). “The neglected problem of the neurofeedback learning (In)ability,” in Biomedical Engineering and Neuroscience, eds W. Hunek and S. Paszkiel (Cham: Springer International Publishing).
Thibault, R. T., Lifshitz, M., and Raz, A. (2016). The self-regulating brain and neurofeedback: experimental science and clinical promise. Cortex 74, 247–261. doi: 10.1016/j.cortex.2015.10.024
Thibault, R. T., Veissière, S., Olson, J. A., and Raz, A. (2018). Treating ADHD With suggestion: neurofeedback and placebo therapeutics. J. Attention Disord. 22, 707–711. doi: 10.1177/1087054718770012
Tolin, D. F., Davies, C. D., Moskow, D. M., and Hofmann, S. G. (2020). “Biofeedback and neurofeedback for anxiety disorders: a quantitative and qualitative systematic review,” in Anxiety Disorders: Rethinking and Understanding Recent Discoveries, ed. Y.-K. Kim (Singapore: Springer Singapore), 265–289. doi: 10.1007/978-981-32-9705-0_16
Trespidi, C., Barbui, C., and Cipriani, A. (2011). Why it is important to include unpublished data in systematic reviews. Epidemiol. Psychiatr. Sci. 20, 133–135. doi: 10.1017/s2045796011000217
Trullinger, M., Novian, A., Russell-Chapin, L., and Pradhan, D. (2019). Perspectives on type III statistical errors: exaggerating the effects of placebo in neurofeedback. NeuroRegulation 6:38.
Twenge, J. M., Cooper, A. B., Joiner, T. E., Duffy, M. E., and Binau, S. G. (2019). Age, period, and cohort trends in mood disorder indicators and suicide-related outcomes in a nationally representative dataset, 2005–2017. J. Abnorm. Psychol. 128:185. doi: 10.1037/abn0000410
Van Der Kolk, B. A., Hodgdon, H., Gapen, M., Musicaro, R., Suvak, M. K., Hamlin, E., et al. (2016). A randomized controlled study of neurofeedback for chronic PTSD. PLoS One 11:e0166752. doi: 10.1371/journal.pone.0166752
Van Doren, J., Arns, M., Heinrich, H., Vollebregt, M. A., Strehl, U. K., and Loo, S. (2019). Sustained effects of neurofeedback in ADHD: a systematic review and meta-analysis. Eur. Child Adolesc. Psychiatry 28, 293–305. doi: 10.1007/s00787-018-1121-4
Viereck, B., Strehl, U., and Kotchoubey, B. (2017). Is A/T neuro feedback training (NFT) a successful treatment method for women with moderate to severe trait anxiety: a clinical trial and methodological considerations. NeuroRegulation 4, 140–142.
Wahbeh, H., and Oken, B. (2013). Peak high-frequency HRV and peak alpha frequency higher in PTSD. Appl. Psychophysiol. Biofeedback 38, 57–69. doi: 10.1007/s10484-012-9208-z
Wang, S.-Y., Lin, I. M., Fan, S.-Y., Tsai, Y.-C., Yen, C.-F., Yeh, Y.-C., et al. (2019). The effects of alpha asymmetry and high-beta down-training neurofeedback for patients with the major depressive disorder and anxiety symptoms. J. Affect. Disord. 257, 287–296. doi: 10.1016/j.jad.2019.07.026
Watson, C. G., and Herder, J. (1980). Effectiveness of alpha biofeedback therapy: negative results. J. Clin. Psychol. 36, 508–513. doi: 10.1002/jclp.6120360221
Weber, L. A., Ethofer, T., and Ehlis, A.-C. (2020). Predictors of neurofeedback training outcome: a systematic review. NeuroImage Clin. 27:102301. doi: 10.1016/j.nicl.2020.102301
Witte, M., Kober, S. E., and Wood, G. (2018). Noisy but not placebo: defining metrics for effects of neurofeedback. Brain 141:e40.
World Health Organization [WHO] (2018). ICD-11 for Mortality and Morbidity Statistics, 2018 Version. Geneva: WHO.
Zuberer, A., Brandeis, D., and Drechsler, R. (2015). Are treatment effects of neurofeedback training in children with ADHD related to the successful regulation of brain activity? A review on the learning of regulation of brain activity and a contribution to the discussion on specificity. Front. Hum. Neurosci. 9:135. doi: 10.3389/fnhum.2015.00135
Keywords: EEG, neurofeedback, systematic review, internalizing disorders, emotional disorders, OCD, PTSD, major depressive disorder (MDD)
Citation: Perez TM, Mathew J, Glue P, Adhia DB and De Ridder D (2022) Is There Evidence for the Specificity of Closed-Loop Brain Training in the Treatment of Internalizing Disorders? A Systematic Review. Front. Neurosci. 16:821136. doi: 10.3389/fnins.2022.821136
Received: 23 November 2021; Accepted: 10 February 2022;
Published: 10 March 2022.
Edited by:
Reinhold Scherer, University of Essex, United KingdomReviewed by:
Tomas Ros, Université de Genève, SwitzerlandPatrick K. A. Neff, Swiss Federal Institute of Technology Lausanne, Switzerland
Copyright © 2022 Perez, Mathew, Glue, Adhia and De Ridder. This is an open-access article distributed under the terms of the Creative Commons Attribution License (CC BY). The use, distribution or reproduction in other forums is permitted, provided the original author(s) and the copyright owner(s) are credited and that the original publication in this journal is cited, in accordance with accepted academic practice. No use, distribution or reproduction is permitted which does not comply with these terms.
*Correspondence: Tyson Michael Perez, dHlzb24ucGVyZXpAcG9zdGdyYWQub3RhZ28uYWMubno=