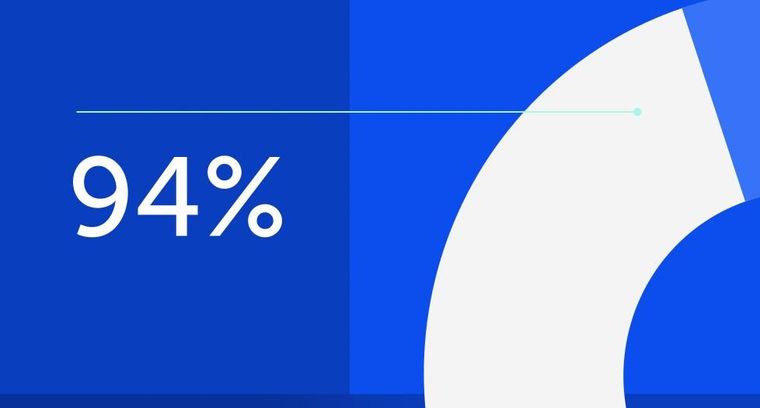
94% of researchers rate our articles as excellent or good
Learn more about the work of our research integrity team to safeguard the quality of each article we publish.
Find out more
ORIGINAL RESEARCH article
Front. Neurosci., 28 July 2022
Sec. Neuroprosthetics
Volume 16 - 2022 | https://doi.org/10.3389/fnins.2022.899772
Persistent pain is a key symptom in people living with knee osteoarthritis (KOA). Infra-slow Neurofeedback (ISF-NF) training is a recent development focusing on modulating cortical slow-wave activity to improve pain outcomes. A parallel, two-armed double-blinded, randomized sham-controlled, feasibility clinical trial aimed to determine the feasibility and safety of a novel electroencephalography-based infraslow fluctuation neurofeedback (EEG ISF-NF) training in people with KOA and determine the variability of clinical outcomes and EEG changes following NF training. Eligible participants attended nine 30-min ISF-NF training sessions involving three cortical regions linked to pain. Feasibility measures were monitored during the trial period. Pain and functional outcomes were measured at baseline, post-intervention, and follow-up after 2 weeks. Resting-state EEG was recorded at baseline and immediate post-intervention. Participants were middle-aged (61.7 ± 7.6 years), New Zealand European (90.5%), and mostly females (62%) with an average knee pain duration of 4 ± 3.4 years. The study achieved a retention rate of 91%, with 20/22 participants completing all the sessions. Participants rated high levels of acceptance and “moderate to high levels of perceived effectiveness of the training.” No serious adverse events were reported during the trial. Mean difference (95% CI) for clinical pain and function measures are as follows for pain severity [active: 0.89 ± 1.7 (−0.27 to 2.0); sham: 0.98 ± 1.1 (0.22–1.7)], pain interference [active: 0.75 ± 2.3 (−0.82 to 2.3); Sham: 0.89 ± 2.1 (−0.60 to 2.4)], pain unpleasantness [active: 2.6 ± 3.7 (0.17–5.1); sham: 2.8 ± 3 (0.62–5.0)] and physical function [active: 6.2 ± 13 (−2.6 to 15); sham: 1.6 ± 12 (−6.8 to 10)]. EEG sources demonstrated frequency-specific neuronal activity, functional connectivity, and ISF ratio changes following NF training. The findings of the study indicated that the ISF-NF training is a feasible, safe, and acceptable intervention for pain management in people with KOA, with high levels of perceived effectiveness. The study also reports the variability in clinical, brain activity, and connectivity changes following training.
Persistent pain is a significant presenting complaint in knee osteoarthritis (KOA), leading to activity limitation and participation restrictions (Vongsirinavarat et al., 2020). Available non-pharmacological and pharmacological interventions for reducing pain and improving function (Yusuf, 2016; Ferreira et al., 2019) only achieve modest improvements in pain outcomes and associated disability (Beswick et al., 2012; Kittelson et al., 2014; Gregori et al., 2018). Neuroimaging studies demonstrate altered cortical activities at the somatosensory cortex (SSC) (Gingold et al., 1991; Price, 2000; Craig, 2002; Bushnell et al., 2013), dorsal anterior cingulate cortex (dACC), (Rainville et al., 1997; Price, 2000; Vogt and Sikes, 2000; Rainville, 2002; Fallon et al., 2015; Vanneste et al., 2017), and the pregenual anterior cingulate cortex (pgACC) in persistent pain conditions including KOA (Price, 2000; Fields, 2004; Sofat et al., 2011; Bushnell et al., 2013; Kwon et al., 2014; Ossipov et al., 2014; Fingleton et al., 2015; Vanneste et al., 2017; Peters et al., 2019; Soni et al., 2019; Apkarian, 2020). A heuristic pathophysiological model suggests that pain is the consequence of an imbalance between the pain-evoking and descending pain inhibitory brain regions in patients with chronic pain treated by spinal cord stimulation (De Ridder and Vanneste, 2016, 2021; Vanneste and De Ridder, 2021).
Electroencephalography-based neurofeedback (EEG-NF) is a non-invasive neuromodulatory technique in which cortical electrical activity is measured and fed back in real-time to the individual to facilitate self-regulation of the cortical activity to influence specific behavior or clinical outcomes (Ros et al., 2014; Sitaram et al., 2017). EEG-NF protocols are designed either to upregulate or downregulate the cortical potentials of various regions of interest (ROI) linked to symptom or disease states (Rance et al., 2011; Gorini et al., 2015; Yang L. et al., 2015; Marzbani et al., 2016; Enriquez-Geppert et al., 2017; Shoji et al., 2017; Sitaram et al., 2017; Prinsloo et al., 2018; Reiner et al., 2018; Saj et al., 2018; Roy et al., 2020). The functional magnetic resonance imaging (fMRI) Blood Oxygenation Level Dependent (BOLD) signal fluctuates in the infraslow frequency (ISF) band (0.01–0.1 Hz) and correlates with EEG infraslow fluctuations (Pan et al., 2013; Hiltunen et al., 2014; Thompson et al., 2014; Keinänen et al., 2018; Gutierrez-Barragan et al., 2019). Increasing evidence implicates the role of a novel ISF in modulating dynamic brain connections (Ploner et al., 2017) and physiological and pathological brain functions including in chronic pain (Vanhatalo et al., 2004; Lőrincz et al., 2009; Schroeder and Lakatos, 2009; Hughes et al., 2011; Ko et al., 2011; Rodin et al., 2014). EEG-NF training targeting the ISF band (0.0–0.1 Hz) can produce clinical benefits (Leong et al., 2018; Balt et al., 2020). The ISF band correlates with higher frequency oscillations and is phase-locked with the faster frequency spectrum greater than 1 Hz extending to 20 Hz (Vanhatalo et al., 2005; Ploner et al., 2017). Preclinical and empirical research shows that neuropathic pain is associated with altered ISF within the dorsal horn, extending to the SSC (Gerke et al., 2003; Iwata et al., 2011). Additionally, evidence from neuroimaging studies demonstrates increased ISF activity within the pain-evoking brain regions, including dACC and SSC, and decreased ISF activity within the antinociceptive network, including pgACC (Majeed et al., 2011; Kucyi et al., 2013; Kucyi and Davis, 2015; Alshelh et al., 2016; Alshelh, 2018; Zhou et al., 2018; Zhang B. et al., 2019; Zhang Y. et al., 2019; Di Pietro et al., 2020). Also, greater infra-Slow oscillation power in the contralateral orbitofrontal cortex, insula, thalamus, secondary SSC, and the ipsilateral anterior insula was identified in individuals with CRPS compared with controls (Di Pietro et al., 2020). EEG-NF training of the ISF band can modulate the resting-state brain networks, and this has been demonstrated in both clinical and preclinical studies (Monto et al., 2008; Dong et al., 2012; Mairena et al., 2012; Smith et al., 2014). Currently, there is no evidence of the efficacy of ISF-NF training in people with pain. Moreover, there is no evidence on the safety, feasibility, and acceptability of the ISF-NF training as an intervention for persistent pain (Mathew et al., 2020). Therefore, the objectives of the study were: (1) to assess the feasibility, safety, and acceptability of ISF-NF training in individuals with KOA; (2) to descriptively report the variability of change in the clinical and experimental pain outcomes in people with KOA following NF training; (3) to descriptively explore the changes in EEG current source density (CSD) at the targeted cortical areas (SSC, dACC, and pgACC), functional connectivity between the three cortical areas (SSC, dACC, and pgACC), and the CSD ratios between the ROIs (SSC, dACC, and pgACC) following ISF-NF training.
A two-arm, parallel-group, double-blinded, randomized sham-controlled feasibility trial was conducted in Dunedin, New Zealand. The trial is reported according to the Consolidated Standards of Reporting Trials (CONSORT) extension for randomized pilot and feasibility trials (Eldridge et al., 2016). The description of the study intervention was structured following the Template for Intervention Description and Replication (TIDieR) guide (Hoffmann et al., 2014). The trial was registered with the Australian New Zealand Clinical Trials Registry (ACTRN12620000273987), and the study protocol was published in a peer-reviewed journal (Mathew et al., 2020). This study was approved by the Health and Disability Ethics Committee (HDEC), New Zealand (19CEN182), and the cultural consultation was obtained from the Ngāi Tahu Research Consultation Committee (5733_21392).
Study participants were recruited from the Dunedin community using convenience sampling. Periodic advertising was carried out through Newspapers, Facebook (Sponsored), an e-mail invitation to the University of Otago (UO), displaying study flyers at the Public Hospital, School of Physiotherapy clinic, and other UO departments. Interested volunteers contacted the first author via telephone or email and underwent screening using the Qualtrics online survey platform (Qualtrics, Provo, UT, United States, 2013) (Cantor and Thorpe, 2018). Eligible participants were further contacted via phone, and appointments were fixed for the confirmatory baseline assessment session. Eligible participants provided written consent and completed a baseline assessment. Calendar invitations were sent to all the participants confirming their appointments for their training sessions and post-intervention assessment session. Automated reminder emails or text messages were sent prior to assessment and training session days.
Adults aged 44–75 years, with a clinical diagnosis of KOA with pain severity of at least ≥ four on an 11-point numerical rating scale for a minimum duration of 3 months were eligible to participate in the study (Fingleton et al., 2015; Bartley et al., 2016).
Participants were excluded if they had one of the following situations or conditions: underwent surgery or other invasive procedures in the last 6 months and any surgical procedures scheduled within 8 weeks after screening; undertaken any steroid injections to the knee joint in the past 3 months or on oral steroids in the previous month; current intake of centrally acting medications or intention of taking new medications in the next 8 weeks; neurological conditions or diseases; soft tissue injuries of the knee in the last 3 months; cognitive impairments; difficulty or inability to read or understand English or provide informed consent; and pregnancy or 6 months post-labor. A paper-based Mini-Mental State Examination (MMSE) was carried out for screening volunteers with cognitive impairments. The maximum MMSE is scored out of 30 points, and volunteers scoring a total score of 24 or below were excluded from the study.
Participants were randomized into either active ISF-NF or sham ISF-NF groups (in a ratio of 1:1) using an open-access block randomization program by the department research administrator not involved in the assessments, allocation, or interventions. The allocations were concealed until after the initial assessment was completed. The NF provider (first author) opened the sealed envelope before the first NF training session.
Participants and the outcome assessor were blinded to the group allocation. The group was disclosed to the participant after completing the follow-up questionnaire after 2 weeks after the post-intervention assessment session. Every week, all the participants were asked at the end of the third training session, “Which training condition do you think you received?” to determine the blinding integrity (Leong et al., 2018).
The recruitment rate was determined by the number of participants attending the screening assessment. The randomization rate was assessed by the ratio of the number of participants randomized into the trial from amongst those eligible. The retention rate was determined by the number of sessions attended by the participant as per the initial appointment schedule. The drop-out rate was measured as the number of participants who dropped out from each group and expressed as a percentage of the total number of participants enrolled in the study.
Acceptability is a “multi-faceted construct that reflects the extent to which people receiving a healthcare intervention consider it appropriate, based on anticipated or experienced cognitive and emotional responses to the intervention” (Sekhon et al., 2017). All the participants reported the training acceptability on a Likert scale with “0” corresponding “not at all acceptable” and “7” corresponding to “very acceptable” on their post-intervention assessment.
The subjective likelihood that the ISF-NF training will have a persuasive impact on the participant (Suka et al., 2018). Participants reported their perceived levels of effectiveness on a Likert scale with “0-not at all effective and 7-very effective” on their post-intervention assessment.
An adverse effect is described as any harmful sign or symptom resulting from the treatment, which could be related to the ISF-NF training. All the participants were instructed to complete a Discontinuation-Emergent Sign and Symptom (DESS) inventory to record worsening or improving side effects compared to the status before every training session. DESS has been used in previous NF studies to record adverse events associated with NF training (Rogel et al., 2015). DESS is a checklist of 43 symptoms, consisting of emotional, behavioral, cognitive, and physical conditions that can be considered possible side effects from NF training. At each training session, participants were asked the following question: “Since the last visit, have you experienced any changes in the following symptoms? (Please check only one response for each symptom).” The scale was originally developed and used to capture symptoms associated with discontinuation or interruption of Selective Serotonin Reuptake Inhibitors (SSRI) treatment (Bhat and Kennedy, 2017). Participants rated each symptom as a “new symptom,” “old symptom but worse,” “old symptom but improved,” “old symptom but unchanged,” and “symptom not present.” We have reported any adverse effect if the participant has reported any symptom as a “new symptom” at least once during the trial, which they think is related to the NF training.
Pain, function, and psycho-social constructs were collected using validated questionnaires. The multi-dimensional constructs were chosen based on the biopsychosocial model of pain literature. Based on the recommendations by Initiative on Methods, Measurement, and Pain Assessment in Clinical Trials (IMMPACT) consensus II, Pain (both numerical and categorical tools), physical and emotional functioning measurement tools were included (Edwards et al., 2016). A summary of the outcome measures is given in Supplementary Table 1. Details including psychometric properties and the method of implementation of each tool are explained in the protocol (Mathew et al., 2020).
Quantitative Sensory Testing (QST) procedures including pressure pain threshold (PPT), mechanical temporal summation (MTS), conditioned pain modulation (CPM), vibration perception threshold, cold hyperalgesia, tactile acuity, and body schema integrity (Rolke et al., 2006; Georgopoulos et al., 2019) are detailed in the published protocol (Mathew et al., 2020) and are also summarized in (Supplemental Digital Content).
Resting-state EEG was obtained in a quiet room while the participant was sitting upright in a comfortable chair by an independent researcher. EEG was collected using the Mitsar EEG system sampled at 500 Hz with WinEEG software at baseline (T0) and immediately post-intervention (T1) (Gorecka and Makiewicz, 2019). The EEG was sampled with 21 electrodes placed in the standard 10–20 International placement, and impedances were checked to remain below 5 kΩ (Khazi et al., 2012). Data was collected for ∼10 min with the participant’s eyes closed. The participants’ alertness was observed by monitoring both the slowing of the alpha rhythm and the appearance of spindles in the EEG stream to prevent possible enhancement of the theta power due to drowsiness during recording (Britton et al., 2016).
Two weeks following the final training session, all participants were contacted by a phone call or email to complete the online survey on pain (pain intensity, pain bothersomeness, pain unpleasantness) and adverse events using the DESS tool.
Eligible participants attended nine sessions (30 min each; three sessions/week) of NF training at the School of Physiotherapy, UO. The intervention was provided by a researcher (JM) who was not involved in the outcome assessment and randomization process. Two NF experts trained the researcher who provided the intervention in the trial. Before the trial, the researcher conducted NF training sessions on 10 healthy people to get familiarized with standardized training protocols (Mathew et al., 2020). The experimental methodology and reporting of NF followed the Consensus on the Reporting and Experimental Design of clinical and cognitive-behavioral NeuroFeedback studies checklist (CRED-nf checklist) (Ros et al., 2020). Since this was a feasibility trial, most of the items on the CRED-nf list were not applicable.
During each session, participants were asked to sit on a chair with their back supported and stay relaxed for 10 min, allowing the trainer to prepare the participant for the NF training. Both active ISF-NF and sham ISF-NF were administered using a 21-channel DC-coupled amplifier produced by BrainMaster Technologies, Inc. The amplifier was connected to a high-end laptop (G752VS, Intel® Core™ i7-6700HQ CPU @ 2.60GHZ) produced by the ASUSTek computer INC. The Comby EEG lead cap with sensors (Ag/AgCl) with appropriate size was fixed to the individual’s head, with reference electrodes placed at the mastoids. A sparse amount of EEG gel was applied to each electrode using a syringe with caution to prevent bridging between adjacent electrodes. The tip of the syringe was used to move the hair beneath each electrode, and a mild abrasion of the scalp was performed. This was to ensure proper electrode placement on the scalp and to acquire quality EEG recording.
The impedance of the active electrodes was monitored and kept below 5 kΩ (Leong et al., 2018). The optimal impedance was acquired by manual adjustment of the electrode initially, and an additional amount of gel was applied, if necessary, but always sparsely. The trainer ensured no more than 5 ml of the gel had been utilized for each training preparation. Any amount of gel escaping from the electrode was removed using a cotton swab before the commencement of the training session. The NF training was performed in a large, closed room with appropriate ventilation. All efforts were made to maintain the room temperature with a central temperature control system. A silent cooler was used when necessary. This was to control (not eliminate) the influence of sweat with EEG recording. The room lighting and influence of external sound were controlled during each training session. Clear instructions were given to the participant before starting the NF training. Participants were emphasized to minimize eyeball movement, head and neck movements, swallowing, and clenching of teeth to minimize motion artifact in the EEG. Each NF session consisted of 30 min of training. The following instruction was given to all the participants before every NF session; “please close your eyes, keep your eyes and tongue nice and still. Listen and concentrate on the sound being played for the coming 30 min. Please let me know if you experience any problem or discomfort; I am right behind you controlling the training system.”
A novel ISF-NF balance training protocol was developed to enhance a balance between the three cortical areas (SSC, dACC, and pgACC) (De Ridder and Vanneste, 2020). The idea of ISF-NF balance training protocol was based on the previous studies that observed an imbalance between the dACC and SSC and pgACC cortical areas (De Ridder and Vanneste, 2020; Vanneste and De Ridder, 2021). The ISF-NF training protocol (Figure 1) involved simultaneously downregulating the electrical activities of SSC (sensory-discriminative function) and dACC (motivational/affective function), and upregulating the pgACC (descending nociceptive inhibitory function) (Rainville et al., 1997; Vogt and Sikes, 2000; Rainville, 2002; Kulkarni et al., 2007; Baliki et al., 2008, 2014; Kong et al., 2010; Tagliazucchi et al., 2010; Howard et al., 2012; Bushnell et al., 2013; Kucyi et al., 2013; Kwon et al., 2014; Ossipov et al., 2014; Fallon et al., 2015; Vanneste et al., 2017; Cottam et al., 2018; Furman et al., 2018; Yam et al., 2018; De Ridder and Vanneste, 2020; Spisak et al., 2020). The SSC was made up of Brodmann areas 1, 2, 3, and 5, as defined by the Montreal Neurological Institute (MNI) coordinate database (Fuchs et al., 2002; Lancaster et al., 2007). The dACC and pgACC were designer ROIs and are defined with the help of the NeuroSynth meta-analytic database.1
Figure 1. The Regions of interests and neurofeedback training directions with respective coordinates (XYZ). This figure was created using brain images produced from eLORETA software and with the help of Microsoft PowerPoint application.
Each participant received auditory feedbacks delivered by BrainMaster Technologies Software for an approximate of 60–80% in real-time when the participant’s brain activity met the desired infraslow (0.0–0.1 Hz) threshold at the targeted ROIs.
The software calculated the ratio in real-time in the ISF band, and the feedback was given when the ratio was > 1 based on satisfying the following equation:
As the dACC and SSC combine two current densities and the pgACC only one, the current density of the pgACC was doubled (Vanneste and De Ridder, 2021). Efforts were made to keep the reward threshold between 60 and 80%. In other words, 60–80% of the time, a sound will be played (reward) when the participant’s brain activity meets the infraslow magnitude (threshold). This was chosen based on the insights from our previous study and the authors’ clinical experience (Leong et al., 2018). Reaching a predetermined threshold brain activity (activities) is a response in relation to the received feedback and participant’s engagement with the training (Enriquez-Geppert et al., 2017; Sitaram et al., 2017). The software delivered the auditory feedback within 30 ms when the activity threshold is met (upregulation of pgACC and downregulation of SSC and dACC). However, further improvement in the response would be dependent on how the participant responds to the reinforcement (Sitaram et al., 2017).
Conditions for the sham ISF-NF group was the same as the active ISF-NF group, except the participants received sound feedback according to someone else’s pre-recorded session. To ensure this, we have trained healthy participants with an active ISF-NF program for nine sessions. We captured the feedback sound using Audacity software, a free and open-source digital audio editor and recording application (Maheshkumar et al., 2016). Participants in the sham ISF-NF were prepared as same as the ISF-NF group, and they received these pre-recorded feedback sounds. The Audacity software uses the computer’s sound card as an audio to digital (A/D) converter and eliminates the additional requirement of an external microprocessor (Maheshkumar et al., 2016). The pre-recorded signals were selected randomly by the chit method from a set of nine files.
Using WinEEG advanced software, raw EEG data were sampled at 500 Hz for 19 channels, filtered from DC to 50 HZ, plotted, and exported in American Standard Code for Information Interchange (ASCII) format (Golomolzina et al., 2014).
The exported files were then batch imported into the EEGLAB (MATLAB R2020a). Each file was resampled to 128 Hz, bandpass filtered from 0.01 to 0.1 Hz and the first 4 s of data were truncated. Subsequently, Average Fourier cross-spectral matrices (Lin and Cai, 2002) were computed for the infraslow band (0.01–0.1 Hz). The transformation matrix was exported for import into the Independent Component Neurofeedback (ICoN) software (Delorme and Makeig, 2004). The ROIs used in the present study were SSC left (S1Lt) [MNI coordinates (MNIxyz) = 18, −40, 68], SSC right (S1Rt) (MNIxyz = −18, −40, 68), pgACC (MNIxyz = 0, 36, 2), and dACC (MNIxyz = 0, 16, 32) (Figure 1).
Each EEG file was carefully inspected in ICoN for eye blinks, muscle artifacts, perspiration, and body movements, and the artifacts were manually rejected from the file. ICoN performs fast blind source separation (BSS) on multiple EEG time-series using second-order statistics (SOS). The ICoN allows source estimation, data filtering (e.g., artifact rejection), and source localization of separated sources through the LORETA-Key software (Pascual-Marqui, 2002; Congedo et al., 2008). The software is widely used in EEG studies for BSS and artifact rejection (Bersagliere et al., 2018; Leong et al., 2018; Vanneste and De Ridder, 2021). The raw EEG data were randomized by the research administrator and the pre-processing and cleaning of the EEG data was blinded. The blinding was concealed until the EEG cleaning was completed.
Exact low-resolution brain electromagnetic tomography (eLORETA) software (Jatoi et al., 2014; Aoki et al., 2015) was used to perform a voxel-by-voxel analysis (comprising 6,239 voxels) for the ISF frequency band of the CSD distribution. The log-transformed current density was averaged across all voxels belonging to the S1Lt, S1Rt, dACC, and pgACC for the ISF frequency band to identify differences in brain electrical activity between the groups. Means, standard deviations, and mean differences for the CSD at the targeted ROIs in the ISF band were calculated.
We also computed the ISF band ratios between the ROIs as below and descriptively compared between the groups.
Similar eLORETA techniques were applied to compute the FC between the ROIs (Pascual-Marqui, 2002). FC is a statistical measure of coherence, i.e., co-varying activity or phase synchronization between two areas in the brain (Vanneste et al., 2017; De Ridder and Vanneste, 2020). The lagged phase synchronization between S1Lt, S1Rt, pgACC, and dACC reflects the communication between those areas (Lancaster et al., 2007; Mathew et al., 2020). Measures of linear dependence (coherence) between the multivariate time series are defined. Functional connectivity contrast maps were calculated through multiple ROI-by-ROI comparisons in eLORETA. The significance threshold was based on a permutation test with 5,000 permutations. The log-transformed electric current density of the FC strength was derived for each connection between the ROI and presented descriptively. The source localization FC analysis is widely used in neuromodulation and neurophysiological studies (Pascual-Marqui, 2007, 2017; Duke Han et al., 2013; Yang X. et al., 2015; Fallon et al., 2016; Pascual-Marqui et al., 2018; Aedo-Jury et al., 2020; Guo et al., 2020; Modares-Haghighi et al., 2021).
All the graphs and calculations were performed using GraphPad Prism software version 9.1.0 for Windows (GraphPad Software, San Diego, CA, United States).
As this was a feasibility trial, a formal sample size estimation was not conducted, and feasibility outcomes were reported based on the feasibility trial recommendations (Tickle-Degnen, 2013; Orsmond and Cohn, 2015). Hypothesis testing to compare study groups was not performed; instead, data were reported descriptively in aggregate and group allocation. Means and standard deviations are reported for continuous normal distribution data. Medians and percentile range are reported for non-normal data. Counts and percentages are reported for categorical data. Both 95 and 75% confidence intervals (CI) for the mean differences were derived and reported for the pain and function measures. Based on the intention-to-treat analysis principle, a “last observation carried forward” methodology was used to impute the missing data for one participant for the follow-up assessment session (Moher et al., 2012; Twisk et al., 2020).
Twenty-two participants with KOA underwent baseline assessment and were randomly assigned to receive either active ISF-NF (n = 11) or sham ISF-NF (n = 11). Participants were middle-aged (61.7 ± 7.6 years), New Zealand European (90.5%), and mostly females (62%) with an average knee pain duration of 4.0 ± 3.4 years. The demographic and clinical characteristics of the participants at baseline are summarized in Table 1. Baseline measures of physical activity, quality of life, health status, psycho-social, and sleep measures are presented as Supplementary Table 2.
One person dropped out from the study during the training session and his data is not demonstrated in the results. Sport/recreational item was missing from the baseline and post-intervention assessment from one participant.
The recruitment period was 5 months. A total number of 113 people indicated their interest to take part in the study. Of 113, 58 people could not be reached either via email or phone. Fifty-five participants completed online screening, out of which 20 did not meet the inclusion criteria, and 13 declined to participate in the study. Twenty-two participants attended baseline assessment sessions and were randomized into either active or sham groups. A detailed CONSORT flow diagram and trial timeline are given in Figures 2A,B. We were able to recruit an average of 4 participants per month for 5 months of the recruitment period. The details of recruitment and data collection are summarized in Table 2.
Table 2 summarizes the number of participants recruited through various advertisement strategies employed in this trial. The overall data collection period was from February to December 2020.
None of the participants indicated any objection or raised concerns regarding the randomization process. All the participants who underwent baseline assessment sessions (n = 22) were randomized to receive either active ISF-NF training (n = 11) or sham ISF-NF training (n = 11).
The study achieved a 95.4% retention rate at the end of the trial, with 21/22 participants completing all the nine NF training sessions (S1–S9). However, 85.7% rescheduled their training sessions at least once during the NF training period due to various personal reasons.
Due to the COVID-19 lockdown in New Zealand (Susan Strongman et al., 2021), one participant in the sham group was forced to withdraw from the study after the seventh training session. Also, one participant from the active group failed to respond to the follow-up email and/or phone call.
Both active and sham group participants reported ISF-NF training as an acceptable intervention with high levels of perceived levels of effectiveness (Table 3).
Participants in both the groups had reported higher levels of motivation, mood, and engagement with the training sessions (Table 3 and Figures 3A–C).
Figure 3. Overall motivation, mood, and engagement with neurofeedback training sessions. (A–C) Violine plot of participants motivation and mood recorded before every neurofeedback session and level engagement scores recorded after the sessions.
Eight participants from the active group correctly identified their allocation group at the end of the training sessions. The results are presented in Table 2. Out of eight, six reported improvements in pain and function as their reason for guessing the group allocation. Two of them were being hopeful and believed that they were in the active group. Only three participants from the sham group correctly identified their allocation group reported due to no improvement in their pain. Participants who failed to identify their allocation group correctly reported various reasons, including being hopeful, positive feeling, and pain improvement.
No serious adverse effects were reported during the trial period. Also, none of the reported symptoms was worsened during the training period (Figure 4). Two sham group participants reported improvement (old symptom but improved) with their “nervousness or anxiety” symptoms after the 4th training session. Three participants from both groups reported improved sleeping after the 3rd and 4th training sessions. One sham group participant reported having improvement with “agitation” symptoms after the 3rd training session. One participant from active ISF-NF and two participants from sham ISF-NF reported improved “fatigue and tiredness” after the 3rd training session. The participants in both groups reported no other symptoms.
Figure 4. Distribution of the reported adverse effects by both groups on the Discontinuation-Emergent Sign and Symptom inventory.
Participants in both groups have shown changes in pain severity, pain interference, and unpleasantness (Table 4). Individual participant data for all outcome measures are plotted in Figures 5A–H. Descriptive trend analyses show that the active group (vs. Sham group) showed greater improvement in pain unpleasantness, pain interference, physical function, and physical activity measures, and positive changes in the QST measures (Tables 4–6). These observations were made based on the descriptive data and graphical trend. No statistical analysis was conducted, as this was a feasibility trial and not powered to perform any statistical analysis.
Figure 5. (A–H) Individual participant data for pain severity, pain unpleasantness, pain interference, and physical function scores. *Higher the score, the higher the physical function.
Another observation includes the improvements in pain severity during immediate and follow-up assessments when compared to baseline. The sham group tends to taper off on pain severity after the training period, whereas the active training group effect is either maintained or further improved over time (Figures 6A–C). Also, participants in the active ISF-NF group have shown a consistent reduction in their pain interference score during their NF sessions. In contrast, sham ISF-NF has not demonstrated a reduction in the pain interference scores.
Figure 6. (A–C) Pain severity, pain unpleasantness, and pain interference (baseline: T0; weekly: WK1-WK3; post-intervention: T1 and the follow-up: T2) measures during the training period.
The log transformed CSD values were averaged across all voxels belonging to the dACC, pgACC, S1Rt, and S1Lt for the ISF band (Figures 7A–C).
Figure 7. (A,B) Shows the CSD (mean and standard deviation) of ISF band in different ROIs utilized in this trial for both groups. (C) The mean difference (T1-T0) of CSD of ISF band at the ROIs for both the groups.
The balance between the areas of interest (pgACC, dACC, and SSC) for the ISF band was calculated and illustrated in Figures 8A,B.
Figure 8. ISF ratio changes. (A,B) Shows the ISF-NF ratio changes before and after NF training for the active and sham groups for left and right cortical regions.
The strength of the ISF band FC between the four ROIs for both the groups are represented in Figures 9A,B.
Figure 9. (A,B) Illustrates the functional connectivity changes (means and standard deviations) for the ISF band frequency between the ROIs for both active and sham group.
To our knowledge, this study is the first to assess the feasibility, safety, and acceptability of ISF-NF training in a musculoskeletal pain population using a randomized, double-blinded, sham-controlled design. The trial results suggest that a future fully powered clinical trial to evaluate the effectiveness of ISF-NF in KOA is feasible, safe, and acceptable, with participants rating high levels of perceived effectiveness. The overall willingness to participate in the trial was high. The results demonstrate a 100% treatment fidelity with all the NF training components, including dosage, duration, and frequency of intervention was delivered and completed as per the study protocol (Mowbray et al., 2003; Kubiak et al., 2014). The study also maintained a 95.4% retention rate, with participants in both groups had reported higher motivation and engagement levels with the NF training sessions. Both the planned primary and secondary outcome measures were feasible and acceptable. Participants in both active and sham groups reported no serious adverse events.
As planned, we recruited the desired number of participants in 5 months of the recruitment period (Mathew et al., 2020). While the COVID-19 pandemic and associated lockdown periods impacted the study recruitment strategies, this extended the overall study recruitment and data collection period. Most of the study participants were recruited through newspaper advertisement (82%), and the rest of them were recruited through social media (9%) and university email circulation (9%). Local community newspaper advertisement is considered the most strategic recruitment method for future trials for the defined age limits.
The majority of the participants were New Zealand European and middle-aged females, consistent with the previous NZ-based studies on KOA (Abbott et al., 2017; Awatere, 2018; Wilson and Abbott, 2019). However, recruitment of a more heterogeneous ethnic sample, including Māori and Pacifica populations with equal sex representation, is warranted in future research. Participants in the active group had an average pain duration of 5 years, and the sham group with nearly 3 years. However, the average pain reported by both the groups in the last 3 months was comparable at baseline (6/10), and both groups displayed a similar number of co-morbidities.
Acceptability and perceived levels of effectiveness are two important domains in the design, evaluation, and implementation of health care interventions (Sekhon et al., 2017; Suka et al., 2017). Our results show that participants in both groups rated high levels of acceptance. Low acceptability and negative treatment perception could influence treatment adherence and outcomes (Milosevic et al., 2015).
Participants in both groups also reported moderate to high levels of perceived effectiveness of the training. The domain is understudied in health care interventions, and it is important to conduct standalone research or incorporate it to add further value to future clinical trials. In the current study, we have used only a single-item measure to rate the level of the acceptability and perceived levels of effectiveness with NF training. In the future, studies need to consider incorporating a qualitative methodology approach to investigate participants’ perception of the NF training to understand the depth of the perspective component associated with the intervention.
Participants did not report difficulties or concerns with the assessments, and training procedures, and follow-up. Although all participants completed their training sessions, some of the training sessions were rescheduled within the same week. The main reason for rescheduling is participants’ unavailability to undergo training. Another challenge was that participants were not comfortable returning to work/home with dried electrode gel. Although participants were offered the option to wash their hair at the study location, none did so due to other commitments/personal reasons following the training session. Future research could consider incorporating dry electrodes for training (Yu et al., 2016; Pei et al., 2018).
Evidence from previous studies has reported various side effects associated with NF training (Lubar and Shouse, 1976; Hammond and Kirk, 2008; Todder et al., 2010; Lansbergen et al., 2011; Dongen-Boomsma, 2014; Rogel et al., 2015). It appears that different NF protocols are associated with a varying set of side effects and cannot be generalized. However, all these studies used different NF training protocols, and none of them was designed to modulate the ISF band. To our knowledge, this is the first clinical trial performed a structured methodology using a full DESS scale to document the adverse effects associated with ISF-NF protocol training. In the absence of any serious adverse events, participants in both groups have reported improvement with their pre-existing symptoms during NF training. The results support our previous study on ISF-NF training in food addiction, which also reported no serious/persisting side effects associated with ISF-NF training (Leong et al., 2018).
The level of motivation and mood may impact the participants’ engagement with the NF training (Kleih-Dahms et al., 2011; Ros et al., 2020). Recently NF studies have established the importance of motivational value as an essential addition in predicting the success of NF training (Diaz Hernandez et al., 2018; Perez-Elvira et al., 2021). Our study adds to the growing literature regarding the predictors and factors influencing NF training outcomes (Weber et al., 2020). In our study, participants in both groups were highly motivated and engaged in undergoing the NF training. Our findings support the notion that the individual’s daily mood and motivation will significantly impact the training outcome. It would be of value if the future trial could investigate the relationship between the everyday mood and motivation with the NF training EEG measures. Also, the training time of the day is an important factor determining the participant’s engagement with NF training. Participants who opted for afternoon training sessions displayed higher slowing of the alpha rhythm and enhancement of the theta power indicative of drowsiness during the training time (Noreika et al., 2020). It is important to monitor the participant’s alertness during the training time and need to provide verbal commands if necessary to improve the alertness and engagement with the training sessions. Participants should be fully relaxed and involved with the NF training to learn the patterns of “reward” and maintain the percent of success during the training. However, this needs to be considered an objective to predict the NF outcome in future clinical trials.
We found that most of the active ISF-NF participants could predict their group allocation correctly. A potential reason could be due to reduction of their symptoms. On the other hand, most sham group participants indicated that they were in the active group, as they believed and were hopeful of being in it. This could potentially contribute to the non-specific effect observed in the sham group and needs further investigation. Future trials must incorporate methodologies to investigate any relationship between the subjective pain measures observed in the sham group with their associated motivational or belief neural network (Taylor et al., 2004; Wiech et al., 2008; Zhu et al., 2012; Sterpenich et al., 2014). The failure to identify the group allocation also implies the integrity of the sham methodology used in this trial. However, this does not entirely omit the therapeutic element (Brim and Miller, 2013; Pigott et al., 2018). Also, future trials must evaluate the blinding integrity of the assessor to eliminate the potential bias in the QST measures.
This study demonstrated changes in the clinical outcomes in both active and sham ISF-NF groups. It is noteworthy that the active ISF-NF group showed greater improvements in pain unpleasantness and pain interference scores. This study also observed a group difference in the time course effect on the symptoms during training, post-training (immediate and follow-up) assessments. The time course effect of NF is discussed in previous literature (Rance et al., 2018), and the same trend has been observed in previous NF clinical trials (Van Doren et al., 2019; Arnold et al., 2020). Considering the persistence and continuing improvement in symptoms after NF treatment highlights the importance of including regular longer follow-up assessment sessions in future trials to ensure that the time point of greatest effect is sampled (Rance et al., 2018).
Considering the nature of the study design, we haven’t performed any statistical analysis for the EEG changes. However, the descriptive EEG data will help design and perform sample size calculations for future studies investigating the efficacy of ISF-NF training inducing neural changes in the chronic pain population. Future trials also need to consider a full band activity (>0.1 Hz) and connectivity EEG analysis to explore the effects of ISF-NF and its relationship with change in clinical pain outcomes. The results also demonstrated a positive trend in the balance between the ascending (SSC and dACC) and descending (pgACC) pain pathways in the active group. Moreover, the findings support the concept of the brain imbalance model of chronic pain (Vanneste et al., 2018; De Ridder et al., 2021; Vanneste and De Ridder, 2021), and thus, the imbalance can potentially be improved using ISF-NF intervention. However, these results need to be tested on a larger population to determine the changes in ratio/balance between the pain pathways and their association with the difference in the clinical and experimental pain outcomes following NF training.
Our findings should be interpreted with consideration of the study’s limitations. We recruited a small sample of KOA individuals with a homogeneous sex and race distribution, which restricts the generalizability of the study findings. The feasibility of long-term follow-up assessment and a higher number of NF training sessions was not studied in this trial. Despite the limitations, this study provides an important foundation for designing and implementing future clinical trials investigating ISF-NF protocols. Our study outcomes regarding the feasibility, safety, and acceptability provide validation for such future studies to replicate and explore the effectiveness of ISF-NF for chronic musculoskeletal pain conditions. We recommend a fully powered RCT to investigate the ISF-NF balance training protocol’s effectiveness with a justifiable sample size calculated based on the reported means and standard deviations for detecting differences between groups of at least medium effect size (Cohen’s d = 0.5) with 80% statistical power.
In conclusion, this feasibility clinical trial suggests that a fully powered clinical trial is feasible and safe to be conducted in people with KOA. Moreover, people with KOA reported high levels of acceptability and perceived high levels of effectiveness of training and higher levels of motivation and engagement with the NF training. The study provides variability in the clinical and EEG measures, thus informing the future studies in ISF-NF for the estimation of sample size for a fully powered clinical trial.
The datasets generated during and/or analyzed during the current study are available from the corresponding author on reasonable request.
The studies involving human participants were reviewed and approved by Health and Disability Ethics Committee (HDEC), New Zealand (19CEN182), and the cultural consultation was obtained from the Ngāi Tahu Research Consultation Committee (5733_21392). The patients/participants provided their written informed consent to participate in this study.
DD, DA, RM, and JM provided the cortical areas to be targeted, their coordinates, and the desired training protocol. MS developed the ISF-NF programme based on the provided regions and coordinates. JM conducted the participant recruitment, data collection, EEG data processing, analysis, and wrote the first draft. All authors contributed to the interpretation of the results, manuscript revision and reviewed the final version making the necessary changes, approved the submitted version, and agreed to be accountable for the content of the work.
This study was supported by the Centre for Health, Activity, and Rehabilitation Research (CHARR) yearly graduate research fund.
We acknowledge the International Society for Neuroregulation and Research (ISNR) and the International QEEG Certification Board for supporting the JM in the form of monetary awards and scholarships in support of this study. We would like to thank Hazel Potterton, Tyson Michael Perez, and Matt Hall for their active support and help throughout this study. Also, we thank all the study participants for their time and support to complete this study during the COVID-19 pandemic in 2020.
MS is the owner of Neurofeedback Therapy Services of New York, New York.
The remaining author declares that the research was conducted in the absence of any commercial or financial relationships that could be construed as a potential conflict of interest.
All claims expressed in this article are solely those of the authors and do not necessarily represent those of their affiliated organizations, or those of the publisher, the editors and the reviewers. Any product that may be evaluated in this article, or claim that may be made by its manufacturer, is not guaranteed or endorsed by the publisher.
The Supplementary Material for this article can be found online at: https://www.frontiersin.org/articles/10.3389/fnins.2022.899772/full#supplementary-material
Abbott, J. H., Usiskin, I. M., Wilson, R., Hansen, P., and Losina, E. (2017). The quality-of-life burden of knee osteoarthritis in new zealand adults: a model-based evaluation. PLoS One 12:e0185676. doi: 10.1371/journal.pone.0185676
Aedo-Jury, F., Schwalm, M., Hamzehpour, L., and Stroh, A. (2020). Brain states govern the spatio-temporal dynamics of resting-state functional connectivity. Elife 9:e53186. doi: 10.7554/eLife.53186.sa2
Alshelh, Z. (2018). Infra-Slow Oscillations In Chronic Orofacial Neuropathic Pain And The Effects Of Palmitoylethanolamide Ph. D, Thesis. University of Sydney Faculty of Medicine and Health Department of Anatomy and Histology Laboratory of Neural Imaging.
Alshelh, Z., Di Pietro, F., Youssef, A. M., Reeves, J. M., Macey, P. M., Vickers, E. R., et al. (2016). Chronic neuropathic pain: it’s about the rhythm. J. Neurosci. 36, 1008–1018. doi: 10.1523/JNEUROSCI.2768-15.2016
Aoki, Y., Ishii, R., Pascual-Marqui, R. D., Canuet, L., Ikeda, S., Hata, M., et al. (2015). Detection of EEG-resting state independent networks by eLORETA-ICA method. Front Hum Neurosci 9:31. doi: 10.3389/fnhum.2015.00031
Apkarian, A. V. (2020). Making Chronic Pain Visible: Risks, Mechanisms, Consequences,” in Make Life Visible. Singapore: Springer, 127–131. doi: 10.1007/978-981-13-7908-6_12
Arnold, L. E., Arns, M., Barterian, J., Bergman, R., Black, S., Conners, C. K., et al. (2020). Double-blind placebo-controlled randomized clinical trial of neurofeedback for attention-deficit/hyperactivity disorder with 13-month follow-up. J. Am. Acad. Child Adoles. Psychiatry. 60, 811–812. doi: 10.1016/j.jaac.2020.07.906
Awatere, S. A. (2018). I Like To Be Treated Like A Person, A Little Smile Never Costs A Thing” : Weaving Kaumâtua Experiences Of Living With Osteoarthritis In Hawkes’s Bay, Aotearoa New Zealand Into A Collaborative Osteoarthritis-Management Toolkit : A Thesis Presented For The Requirements For The Degree of Doctor of Philosophy, Public Health, Massey University, Palmerston North, New Zealand Ph. D, Thesis.
Baliki, M. N., Geha, P. Y., Jabakhanji, R., Harden, N., Schnitzer, T. J., and Apkarian, A. V. (2008). A preliminary fMRI study of analgesic treatment in chronic back pain and knee osteoarthritis. Mol. Pain 4:47. doi: 10.1186/1744-8069-4-47
Baliki, M. N., Mansour, A. R., Baria, A. T., and Apkarian, A. V. (2014). Functional reorganization of the default mode network across chronic pain conditions. PLoS One 9:e106133. doi: 10.1371/journal.pone.0106133
Balt, K., Du Toit, P., Smith, M. L., and Van Rensburg, C. J. (2020). The effect of infraslow frequency neurofeedback on autonomic nervous system function in adults with anxiety and related diseases. NeuroRegulation 7, 64–64. doi: 10.15540/nr.7.2.64
Bartley, E. J., King, C. D., Sibille, K. T., Cruz-Almeida, Y., Riley Iii, J. L., Glover, T. L., et al. (2016). Enhanced pain sensitivity among individuals with symptomatic knee osteoarthritis: potential sex differences in central sensitization. Arthr. Care Res. 68, 472–480. doi: 10.1002/acr.22712
Bersagliere, A., Pascual-Marqui, R. D., Tarokh, L., and Achermann, P. (2018). Mapping slow waves by EEG topography and source localization: effects of sleep deprivation. Brain Topogr 31, 257–269. doi: 10.1007/s10548-017-0595-6
Beswick, A. D., Wylde, V., Gooberman-Hill, R., Blom, A., and Dieppe, P. (2012). What proportion of patients report long-term pain after total hip or knee replacement for osteoarthritis? A systematic review of prospective studies in unselected patients. BMJ Open 2, 1–18. doi: 10.1136/bmjopen-2011-000435
Bhat, V., and Kennedy, S. H. (2017). Recognition and management of antidepressant discontinuation syndrome. J. Psychiatry Neurosci. 42, E7–E8. doi: 10.1503/jpn.170022
Brim, R. L., and Miller, F. G. (2013). The potential benefit of the placebo effect in sham-controlled trials: implications for risk-benefit assessments and informed consent. J. Med. Ethics 39, 703–707. doi: 10.1136/medethics-2012-101045
Britton, J. W., Frey, L. C., Hopp, J., Korb, P., Koubeissi, M., Lievens, W., et al. (2016). An Introductory Text And Atlas Of Normal And Abnormal Findings In Adults, Children, And Infants. Chicago: American Epilepsy Society.
Bushnell, M. C., Ceko, M., and Low, L. A. (2013). Cognitive and emotional control of pain and its disruption in chronic pain. Nat. Rev. Neurosci. 14, 502–511. doi: 10.1038/nrn3516
Cantor, M. N., and Thorpe, L. (2018). Integrating data on social determinants of health into electronic health records. Health Affairs 37, 585–590. doi: 10.1377/hlthaff.2017.1252
Congedo, M., Gouy-Pailler, C., and Jutten, C. (2008). On the blind source separation of human electroencephalogram by approximate joint diagonalization of second order statistics. Clin. Neurophysiol. 119, 2677–2686. doi: 10.1016/j.clinph.2008.09.007
Cottam, W. J., Iwabuchi, S. J., Drabek, M. M., Reckziegel, D., and Auer, D. P. (2018). Altered connectivity of the right anterior insula drives the pain connectome changes in chronic knee osteoarthritis. Pain 159, 929–938. doi: 10.1097/j.pain.0000000000001209
Craig, A. D. (2002). How do you feel? Interoception: the sense of the physiological condition of the body. Nat. Rev. Neurosci. 3, 655–666. doi: 10.1038/nrn894
De Ridder, D., Adhia, D., and Vanneste, S. (2021). The anatomy of pain and suffering in the brain and its clinical implications. Neurosci. Biobehav. Rev. 130, 125–146. doi: 10.1016/j.neubiorev.2021.08.013
De Ridder, D., and Vanneste, S. (2016). Burst and tonic spinal cord stimulation: different and common brain mechanisms. Neuromodulation 19, 47–59. doi: 10.1111/ner.12368
De Ridder, D., and Vanneste, S. (2020). The Bayesian Brain in Imbalance: Medial, Lateral and Descending Pathways In Tinnitus And Pain: A Perspective,” in Progress in Brain Research. Elsevier. doi: 10.1016/bs.pbr.2020.07.012
De Ridder, D., and Vanneste, S. (2021). The Bayesian brain in imbalance: medial, lateral and descending pathways in tinnitus and pain: a perspective. Prog. Brain Res. 262, 309–334.
Delorme, A., and Makeig, S. (2004). EEGLAB: an open source toolbox for analysis of single-trial EEG dynamics including independent component analysis. J. Neurosci. Methods 134, 9–21. doi: 10.1016/j.jneumeth.2003.10.009
Di Pietro, F., Lee, B., and Henderson, L. A. (2020). Altered resting activity patterns and connectivity in individuals with complex regional pain syndrome. Hum. Brain Mapp. 41, 3781–3793. doi: 10.1002/hbm.25087
Diaz Hernandez, L., Rieger, K., and Koenig, T. (2018). Low motivational incongruence predicts successful EEG resting-state neurofeedback performance in healthy adults. Neuroscience 378, 146–154. doi: 10.1016/j.neuroscience.2016.12.005
Dong, Z. Y., Liu, D. Q., Wang, J., Qing, Z., Zang, Z. X., Yan, C. G., et al. (2012). Low-frequency fluctuation in continuous real-time feedback of finger force: a new paradigm for sustained attention. Neurosci. Bull. 28, 456–467. doi: 10.1007/s12264-012-1254-2
Dongen-Boomsma, M. V. (2014). Need, Quest & Evidence. Resting-State Oscillations, Neurofeedback, and Working Memory Training in ADHD. (Doctoral dissertation). Available online at: https://repository.ubn.ru.nl/handle/2066/125153
Duke Han, S., Buchman, A. S., Arfanakis, K., Fleischman, D. A., and Bennett, D. A. (2013). Functional connectivity networks associated with chronic musculoskeletal pain in old age. Int. J. Geriatr. Psychiatry 28, 858–867. doi: 10.1002/gps.3898
Edwards, R. R., Dworkin, R. H., Turk, D. C., Angst, M. S., Dionne, R., Freeman, R., et al. (2016). Patient phenotyping in clinical trials of chronic pain treatments: IMMPACT recommendations. Pain 157, 1851–1871. doi: 10.1097/j.pain.0000000000000602
Eldridge, S. M., Chan, C. L., Campbell, M. J., Bond, C. M., Hopewell, S., Thabane, L., et al. (2016). CONSORT 2010 statement: extension to randomised pilot and feasibility trials. BMJ 355:i5239. doi: 10.1136/bmj.i5239
Enriquez-Geppert, S., Huster, R. J., and Herrmann, C. S. (2017). EEG-neurofeedback as a tool to modulate cognition and behavior: a review tutorial. Front. Hum. Neurosci. 11:51. doi: 10.3389/fnhum.2017.00051
Fallon, N., Chiu, Y., Nurmikko, T., and Stancak, A. (2016). Functional connectivity with the default mode network is altered in fibromyalgia patients. PLoS One 11:e0159198. doi: 10.1371/journal.pone.0159198
Fallon, N., Li, X., and Stancak, A. (2015). Pain catastrophising affects cortical responses to viewing pain in others. PLoS One 10:e0133504. doi: 10.1371/journal.pone.0133504
Ferreira, R. M., Torres, R. T., Duarte, J. A., and Goncalves, R. S. (2019). Non-pharmacological and non-surgical interventions for knee osteoarthritis: a systematic review and meta-analysis. Acta Reumatol. Port 44, 173–217.
Fields, H. (2004). State-dependent opioid control of pain. Nat. Rev. Neurosci. 5, 565–575. doi: 10.1038/nrn1431
Fingleton, C., Smart, K., Moloney, N., Fullen, B. M., and Doody, C. (2015). Pain sensitization in people with knee osteoarthritis: a systematic review and meta-analysis. Osteoarthritis and Cartilage 23, 1043–1056. doi: 10.1016/j.joca.2015.02.163
Fuchs, M., Kastner, J., Wagner, M., Hawes, S., and Ebersole, J. S. (2002). A standardized boundary element method volume conductor model. Clin. Neurophysiol. 113, 702–712. doi: 10.1016/S1388-2457(02)00030-5
Furman, A. J., Meeker, T. J., Rietschel, J. C., Yoo, S., Muthulingam, J., Prokhorenko, M., et al. (2018). Cerebral peak alpha frequency predicts individual differences in pain sensitivity. Neuroimage 167, 203–210. doi: 10.1016/j.neuroimage.2017.11.042
Georgopoulos, V., Akin-Akinyosoye, K., Zhang, W., Mcwilliams, D. F., Hendrick, P., and Walsh, D. A. (2019). Quantitative sensory testing and predicting outcomes for musculoskeletal pain, disability, and negative affect: a systematic review and meta-analysis. Pain 160, 1920–1932. doi: 10.1097/j.pain.0000000000001590
Gerke, M., Duggan, A., Xu, L., and Siddall, P. (2003). Thalamic neuronal activity in rats with mechanical allodynia following contusive spinal cord injury. Neuroscience 117, 715–722. doi: 10.1016/S0306-4522(02)00961-2
Gingold, S. I., Greenspan, J. D., and Apkarian, A. V. (1991). Anatomic evidence of nociceptive inputs to primary somatosensory cortex - relationship between spinothalamic terminals and thalamocortical cells in squirrel-monkeys. J. Comparat. Neurol. 308, 467–490. doi: 10.1002/cne.903080312
Golomolzina, D. R., Gorodnichev, M. A., Levin, E. A., Savostyanov, A. N., Yablokova, E. P., Tsai, A. C., et al. (2014). Advanced electroencephalogram processing: automatic clustering of EEG components. Int. J. E Health Med. Commun. 5, 49–69. doi: 10.4018/ijehmc.2014040103
Gorecka, J., and Makiewicz, P. (2019). The dependence of electrode impedance on the number of performed EEG examinations. Sensors 19:2608. doi: 10.3390/s19112608
Gorini, A., Marzorati, C., Casiraghi, M., Spaggiari, L., and Pravettoni, G. (2015). A neurofeedback-based intervention to reduce post-operative pain in lung cancer patients: study protocol for a randomized controlled trial. JMIR Res. Protoc. 4:e52. doi: 10.2196/resprot.4251
Gregori, D., Giacovelli, G., Minto, C., Barbetta, B., Gualtieri, F., Azzolina, D., et al. (2018). Association of pharmacological treatments with long-term pain control in patients with knee osteoarthritis: a systematic review and meta-analysis. JAMA 320, 2564–2579. doi: 10.1001/jama.2018.19319
Guo, X., Zhang, Q., Singh, A., Wang, J., and Chen, Z. S. (2020). Granger causality analysis of rat cortical functional connectivity in pain. J. Neural. Eng. 17:016050. doi: 10.1088/1741-2552/ab6cba
Gutierrez-Barragan, D., Basson, M. A., Panzeri, S., and Gozzi, A. (2019). Infraslow state fluctuations govern spontaneous fMRI network dynamics. Curr. Biol. 29, 2295–2306.e2295. doi: 10.1016/j.cub.2019.06.017
Hammond, D. C., and Kirk, L. (2008). First, do no harm: adverse effects and the need for practice standards in neurofeedback. J. Neurother. 12, 79–88. doi: 10.1080/10874200802219947
Hiltunen, T., Kantola, J., Abou Elseoud, A., Lepola, P., Suominen, K., Starck, T., et al. (2014). Infra-slow EEG fluctuations are correlated with resting-state network dynamics in fMRI. J. Neurosci. 34, 356–362. doi: 10.1523/JNEUROSCI.0276-13.2014
Hoffmann, T. C., Glasziou, P. P., Boutron, I., Milne, R., Perera, R., Moher, D., et al. (2014). Better reporting of interventions: template for intervention description and replication (TIDieR) checklist and guide. BMJ 348:g1687. doi: 10.1136/bmj.g1687
Howard, M. A., Sanders, D., Krause, K., O’muircheartaigh, J., Fotopoulou, A., Zelaya, F., et al. (2012). Alterations in resting-state regional cerebral blood flow demonstrate ongoing pain in osteoarthritis: an arterial spin-labeled magnetic resonance imaging study. Arthr. Rheum 64, 3936–3946. doi: 10.1002/art.37685
Hughes, S. W., Lorincz, M. L., Parri, H. R., and Crunelli, V. (2011). Infraslow (<0.1 Hz) oscillations in thalamic relay nuclei basic mechanisms and significance to health and disease states. Prog. Brain Res. 193, 145–162. doi: 10.1016/B978-0-444-53839-0.00010-7
Iwata, M., Leblanc, B. W., Kadasi, L. M., Zerah, M. L., Cosgrove, R. G., and Saab, C. Y. (2011). High-frequency stimulation in the ventral posterolateral thalamus reverses electrophysiologic changes and hyperalgesia in a rat model of peripheral neuropathic pain. Pain 152, 2505–2513. doi: 10.1016/j.pain.2011.07.011
Jatoi, M. A., Kamel, N., Malik, A. S., and Faye, I. (2014). EEG based brain source localization comparison of sLORETA and eLORETA. Austr. Phys. Eng. Sci. Med. 37, 713–721. doi: 10.1007/s13246-014-0308-3
Keinänen, T., Rytky, S., Korhonen, V., Huotari, N., Nikkinen, J., Tervonen, O., et al. (2018). Fluctuations of the EEG-fMRI correlation reflect intrinsic strength of functional connectivity in default mode network. J. Neurosci. Res. 96, 1689–1698. doi: 10.1002/jnr.24257
Khazi, M., Kumar, A., and Vidya, M. (2012). Analysis of EEG using 10: 20 electrode system. Int. J. Innov. Res. Sci. Eng. Technol. 1, 185–191.
Kittelson, A. J., George, S. Z., Maluf, K. S., and Stevens-Lapsley, J. E. (2014). Future directions in painful knee osteoarthritis: harnessing complexity in a heterogeneous population. Phys. Ther. 94, 422–432. doi: 10.2522/ptj.20130256
Kleih-Dahms, S. C., Botrel, L., and Kübler, A. (2021). The influence of motivation and emotion on sensorimotor rhythm?based brain-computer interface performance. Psychophysiology 58:e13832. doi: 10.1111/psyp.13832
Ko, A. L., Darvas, F., Poliakov, A., Ojemann, J., and Sorensen, L. B. (2011). Quasi-periodic fluctuations in default mode network electrophysiology. J. Neurosci. 31, 11728–11732. doi: 10.1523/JNEUROSCI.5730-10.2011
Kong, J., Loggia, M. L., Zyloney, C., Tu, P., Laviolette, P., and Gollub, R. L. (2010). Exploring the brain in pain: activations, deactivations and their relation. PAIN 148, 257–267. doi: 10.1016/j.pain.2009.11.008
Kubiak, S. P., Fedock, G., Tillander, E., Kim, W. J., and Bybee, D. (2014). Assessing the feasibility and fidelity of an intervention for women with violent offenses. Eval. Prog. Plann 42, 1–10. doi: 10.1016/j.evalprogplan.2013.08.001
Kucyi, A., and Davis, K. D. (2015). The dynamic pain connectome. Trends Neurosci. 38, 86–95. doi: 10.1016/j.tins.2014.11.006
Kucyi, A., Salomons, T. V., and Davis, K. D. (2013). Mind wandering away from pain dynamically engages antinociceptive and default mode brain networks. Proc. Natl. Acad. Sci. U.S.A. 110, 18692–18697. doi: 10.1073/pnas.1312902110
Kulkarni, B., Bentley, D. E., Elliott, R., Julyan, P. J., Boger, E., Watson, A., et al. (2007). Arthritic pain is processed in brain areas concerned with emotions and fear. Arthr. Rheum 56, 1345–1354. doi: 10.1002/art.22460
Kwon, M., Altin, M., Duenas, H., and Alev, L. (2014). The role of descending inhibitory pathways on chronic pain modulation and clinical implications. Pain Pract 14, 656–667. doi: 10.1111/papr.12145
Lancaster, J. L., Tordesillas-Gutiérrez, D., Martinez, M., Salinas, F., Evans, A., Zilles, K., et al. (2007). Bias between MNI and talairach coordinates analyzed using the ICBM-152 brain template. Hum. Brain Mapp. 28, 1194–1205. doi: 10.1002/hbm.20345
Lansbergen, M. M., Van Dongen-Boomsma, M., Buitelaar, J. K., and Slaats-Willemse, D. (2011). ADHD and EEG-neurofeedback: a double-blind randomized placebo-controlled feasibility study. J. Neural. Transm (Vienna) 118, 275–284. doi: 10.1007/s00702-010-0524-2
Leong, S. L., Vanneste, S., Lim, J., Smith, M., Manning, P., and De Ridder, D. (2018). A randomised, double-blind, placebo-controlled parallel trial of closed-loop infraslow brain training in food addiction. Sci. Rep. 8:11659. doi: 10.1038/s41598-018-30181-7
Lin, Q., and Cai, Y. (2002). Fractional fourier transform for partially coherent gaussian-schell model beams. Opt. Lett. 27, 1672–1674. doi: 10.1364/OL.27.001672
Lőrincz, M. L., Geall, F., Bao, Y., Crunelli, V., and Hughes, S. W. (2009). ATP-dependent infra-slow (< 0.1 Hz) oscillations in thalamic networks. PLoS One 4:e4447. doi: 10.1371/journal.pone.0004447
Lubar, J. F., and Shouse, M. N. (1976). EEG and behavioral changes in a hyperkinetic child concurrent with training of the sensorimotor rhythm (SMR): a preliminary report. Biofeedback Self Regul. 1, 293–306. doi: 10.1007/BF01001170
Maheshkumar, K., Dilara, K., Maruthy, K. N., and Sundareswaren, L. (2016). Validation of PC-based sound card with biopac for digitalization of ECG recording in short-term HRV analysis. N. Am. J. Med. Sci. 8, 307–311. doi: 10.4103/1947-2714.187150
Mairena, M. A., Di Martino, A., Dominguez-Martin, C., Gomez-Guerrero, L., Gioia, G., Petkova, E., et al. (2012). Low frequency oscillations of response time explain parent ratings of inattention and hyperactivity/impulsivity. Eur. Child Adolesc. Psychiatry 21, 101–109. doi: 10.1007/s00787-011-0237-6
Majeed, W., Magnuson, M., Hasenkamp, W., Schwarb, H., Schumacher, E. H., Barsalou, L., et al. (2011). Spatiotemporal dynamics of low frequency BOLD fluctuations in rats and humans. Neuroimage 54, 1140–1150. doi: 10.1016/j.neuroimage.2010.08.030
Marzbani, H., Marateb, H. R., and Mansourian, M. (2016). Neurofeedback: a comprehensive review on system design, methodology and clinical applications. Basic Clin. Neurosci. 7, 143–158. doi: 10.15412/J.BCN.03070208
Mathew, J., Adhia, D., Smith, M., De Ridder, D., and Mani, R. (2020). Protocol for a pilot randomized sham-controlled clinical trial evaluating the feasibility, safety, and acceptability of infraslow electroencephalography neurofeedback training on experimental and clinical pain outcomes in people with chronic painful knee. NeuroRegulation 7, 30–44. doi: 10.15540/nr.7.1.30
Milosevic, I., Levy, H. C., Alcolado, G. M., and Radomsky, A. S. (2015). The treatment acceptability/adherence scale: moving beyond the assessment of treatment effectiveness. Cogn. Behav. Ther. 44, 456–469. doi: 10.1080/16506073.2015.1053407
Modares-Haghighi, P., Boostani, R., Nami, M., and Sanei, S. (2021). Quantification of pain severity using EEG-based functional connectivity. Biomed. Signal Proc. Control 69:102840. doi: 10.1016/j.bspc.2021.102840
Moher, D., Hopewell, S., Schulz, K. F., Montori, V., Gotzsche, P. C., Devereaux, P. J., et al. (2012). CONSORT 2010 explanation and elaboration: updated guidelines for reporting parallel group randomised trials. Int. J. Surg. 10, 28–55. doi: 10.1016/j.ijsu.2011.10.001
Monto, S., Palva, S., Voipio, J., and Palva, J. M. (2008). Very slow EEG fluctuations predict the dynamics of stimulus detection and oscillation amplitudes in humans. J. Neurosci. 28, 8268–8272. doi: 10.1523/JNEUROSCI.1910-08.2008
Mowbray, C., Holter, M., Teague, G., and Bybee, D. (2003). Evaluation methods to establish best practices: fidelity criteria. Am. J. Evaluation 24, 315–340. doi: 10.1177/109821400302400303
Noreika, V., Kamke, M. R., Canales-Johnson, A., Chennu, S., Bekinschtein, T. A., and Mattingley, J. B. (2020). Alertness fluctuations when performing a task modulate cortical evoked responses to transcranial magnetic stimulation. Neuroimage 223:117305. doi: 10.1016/j.neuroimage.2020.117305
Orsmond, G. I., and Cohn, E. S. (2015). The distinctive features of a feasibility study: objectives and guiding questions. OTJR (Thorofare N J) 35, 169–177. doi: 10.1177/1539449215578649
Ossipov, M. H., Morimura, K., and Porreca, F. (2014). Descending pain modulation and chronification of pain. Curr. Opin. Support Palliat Care 8, 143–151. doi: 10.1097/SPC.0000000000000055
Pan, W.-J., Thompson, G. J., Magnuson, M. E., Jaeger, D., and Keilholz, S. (2013). Infraslow LFP correlates to resting-state fMRI BOLD signals. NeuroImage 74, 288–297. doi: 10.1016/j.neuroimage.2013.02.035
Pascual-Marqui, R. D. (2002). Standardized low-resolution brain electromagnetic tomography (sLORETA): technical details. Methods Find. Exp. Clin. Pharmacol. 24, 5–12.
Pascual-Marqui, R. D. (2007). Discrete, 3D distributed, linear imaging methods of electric neuronal activity. Part 1: exact, zero error localization. arXiv [Preprint]. doi: 10.48550/arXiv.0710.3341
Pascual-Marqui, R. D., Faber, P., Kinoshita, T., Kochi, K., Milz, P., Nishida, K., et al. (2018). Comparing EEG/MEG neuroimaging methods based on localization error, false positive activity, and false positive connectivity. BioRxiv [preprint]. * BioRxiv, 269753, doi: 10.1101/269753
Pei, G., Wu, J., Chen, D., Guo, G., Liu, S., Hong, M., et al. (2018). Effects of an integrated neurofeedback system with dry electrodes: EEG acquisition and cognition assessment. Sensors 18, 1–18. doi: 10.3390/s18103396
Perez-Elvira, R., Oltra-Cucarella, J., Carrobles, J. A., Molto, J., Florez, M., Parra, S., et al. (2021). Enhancing the effects of neurofeedback training: the motivational value of the reinforcers. Brain Sci. 11:457. doi: 10.3390/brainsci11040457
Peters, C. M., Munoz-Islas, E., Ramirez-Rosas, M. B., and Jimenez-Andrade, J. M. (2019). Mechanisms underlying non-malignant skeletal pain. Curr. Opin. Physiol. 11, 103–108. doi: 10.1016/j.cophys.2019.10.003
Pigott, H. E., Cannon, R., and Trullinger, M. (2018). The fallacy of sham-controlled neurofeedback trials: a reply to thibault and colleagues (2018). J. Attent. Dis. 25, 448–457. doi: 10.1177/1087054718790802
Ploner, M., Sorg, C., and Gross, J. (2017). Brain rhythms of pain. Trends Cogn. Sci. 21, 100–110. doi: 10.1016/j.tics.2016.12.001
Price, D. D. (2000). Psychological and neural mechanisms of the affective dimension of pain. Science 288, 1769–1772. doi: 10.1126/science.288.5472.1769
Prinsloo, S., Novy, D., Driver, L., Lyle, R., Ramondetta, L., Eng, C., et al. (2018). The long-term impact of neurofeedback on symptom burden and interference in patients with chronic chemotherapy-induced neuropathy: analysis of a randomized controlled trial. J. Pain Sympt. Manage. 55, 1276–1285. doi: 10.1016/j.jpainsymman.2018.01.010
Rainville, P. (2002). Brain mechanisms of pain affect and pain modulation. Curr. Opin. Neurobiol. 12, 195–204. doi: 10.1016/S0959-4388(02)00313-6
Rainville, P., Duncan, G. H., Price, D. D., Carrier, B., and Bushnell, M. C. (1997). Pain affect encoded in human anterior cingulate but not somatosensory cortex. Science 277, 968–971. doi: 10.1126/science.277.5328.968
Rance, M., Walsh, C., Sukhodolsky, D. G., Pittman, B., Qiu, M., Kichuk, S. A., et al. (2018). Time course of clinical change following neurofeedback. NeuroImage 181, 807–813. doi: 10.1016/j.neuroimage.2018.05.001
Rance, M., Yilmaz, P., Foell, J., Bekrater-Bodmann, R., Diers, M., Trojan, J., et al. (2011). Neurofeedback and pain: a viable tool to treat phantom limb pain (PLP)? Eur. J. Pain Suppl. 5:258. doi: 10.1016/S1754-3207(11)70892-6
Reiner, M., Lev, D. D., and Rosen, A. (2018). Theta neurofeedback effects on motor memory consolidation and performance accuracy: an apparent paradox? Neuroscience 378, 198–210. doi: 10.1016/j.neuroscience.2017.07.022
Rodin, E., Constantino, T., and Bigelow, J. (2014). Interictal infraslow activity in patients with epilepsy. Clin. Neurophysiol. 125, 919–929. doi: 10.1016/j.clinph.2013.10.014
Rogel, A., Guez, J., Getter, N., Keha, E., Cohen, T., Amor, T., et al. (2015). Transient adverse side effects during neurofeedback training: a randomized, sham-controlled, double blind study. Appl. Psychophysiol. Biofeedback 40, 209–218. doi: 10.1007/s10484-015-9289-6
Rolke, R., Baron, R., Maier, C., Tolle, T. R., Treede, D. R., Beyer, A., et al. (2006). Quantitative sensory testing in the german research network on neuropathic pain (DFNS): standardized protocol and reference values. Pain 123, 231–243. doi: 10.1016/j.pain.2006.01.041
Ros, T., Enriquez-Geppert, S., Zotev, V., Young, K. D., Wood, G., Whitfield-Gabrieli, S., et al. (2020). Consensus on the Reporting And Experimental Design Of Clinical And Cognitive-Behavioural Neurofeedback Studies (CRED-nf Checklist). Oxford: Oxford University Press.
Ros, T., Lanius, R. A., and Vuilleumier, P. (2014). Tuning pathological brain oscillations with neurofeedback: a systems neuroscience framework. Front. Hum. Neurosci. 8:1008. doi: 10.3389/fnhum.2014.01008
Roy, R., De La Vega, R., Jensen, M. P., and Miro, J. (2020). Neurofeedback for pain management: a systematic review. Front. Neurosci. 14:671. doi: 10.3389/fnins.2020.00671
Saj, A., Ros, T., Michela, A., and Vuilleumier, P. (2018). Effect of a single early EEG neurofeedback training on remediation of spatial neglect in the acute phase. Ann. Phys. Rehabil. Med. 61, 111–112. doi: 10.1016/j.rehab.2017.11.001
Schroeder, C. E., and Lakatos, P. (2009). Low-frequency neuronal oscillations as instruments of sensory selection. Trends Neurosci. 32, 9–18. doi: 10.1016/j.tins.2008.09.012
Sekhon, M., Cartwright, M., and Francis, J. J. (2017). Acceptability of healthcare interventions: an overview of reviews and development of a theoretical framework. BMC Health Serv. Res. 17:88. doi: 10.1186/s12913-017-2031-8
Shoji, Y., Patti, C. R., and Cvetkovic, D. (2017). Electroencephalographic neurofeedback to up-regulate frontal theta rhythms: preliminary results. Ann. Int. Conf. IEEE. Eng. Med. Biol. Soc. 2017, 1425–1428. doi: 10.1109/EMBC.2017.8037101
Sitaram, R., Ros, T., Stoeckel, L., Haller, S., Scharnowski, F., Lewis-Peacock, J., et al. (2017). Closed-loop brain training: the science of neurofeedback. Nat. Rev. Neurosci. 18, 86–100. doi: 10.1038/nrn.2016.164
Smith, M. L., Collura, T. F., Ferrera, J., and De Vries, J. (2014). Infra-slow fluctuation training in clinical practice: a technical history. NeuroRegulation 1, 187–187. doi: 10.15540/nr.1.2.187
Sofat, N., Ejindu, V., and Kiely, P. (2011). What makes osteoarthritis painful? The evidence for local and central pain processing. Rheumatology 50, 2157–2165. doi: 10.1093/rheumatology/ker283
Soni, A., Wanigasekera, V., Mezue, M., Cooper, C., Javaid, M. K., Price, A. J., et al. (2019). Central sensitization in knee osteoarthritis: relating presurgical brainstem neuroimaging and pain DETECT-based patient stratification to arthroplasty outcome. Arthr. Rheumatol. 71, 550–560. doi: 10.1002/art.40749
Spisak, T., Kincses, B., Schlitt, F., Zunhammer, M., Schmidt-Wilcke, T., Kincses, Z. T., et al. (2020). Pain-free resting-state functional brain connectivity predicts individual pain sensitivity. Nat. Commun. 11:187. doi: 10.1038/s41467-019-13785-z
Sterpenich, V., Schwartz, S., Maquet, P., and Desseilles, M. (2014). Ability to maintain internal arousal and motivation modulates brain responses to emotions. PLoS One 9:e112999. doi: 10.1371/journal.pone.0112999
Suka, M., Yamauchi, T., and Yanagisawa, H. (2017). Perceived effectiveness rating scales applied to insomnia help-seeking messages for middle-aged Japanese people: a validity and reliability study. Environ. Health Prevent. Med. 22:69. doi: 10.1186/s12199-017-0676-x
Suka, M., Yamauchi, T., and Yanagisawa, H. (2018). Comparing responses to differently framed and formatted persuasive messages to encourage help-seeking for depression in Japanese adults: a cross-sectional study with 2-month follow-up. BMJ Open 8:e020823. doi: 10.1136/bmjopen-2017-020823
Susan Strongman, M. H., Michelle, C., and Rose, D. (2021). Timeline: the year of covid-19 in new zealand. Radio New Zealand 28:2021.
Tagliazucchi, E., Balenzuela, P., Fraiman, D., and Chialvo, D. R. (2010). Brain resting state is disrupted in chronic back pain patients. Neurosci. Lett. 485, 26–31. doi: 10.1016/j.neulet.2010.08.053
Taylor, S. F., Welsh, R. C., Wager, T. D., Phan, K. L., Fitzgerald, K. D., and Gehring, W. J. (2004). A functional neuroimaging study of motivation and executive function. Neuroimage 21, 1045–1054. doi: 10.1016/j.neuroimage.2003.10.032
Thompson, G. J., Pan, W.-J., Billings, J. C. W., Grooms, J. K., Shakil, S., Jaeger, D., et al. (2014). Phase-amplitude coupling and infraslow (<1 Hz) frequencies in the rat brain: relationship to resting state fMRI. Front. Integrat. Neurosci. 8:41. doi: 10.3389/fnint.2014.00041
Tickle-Degnen, L. (2013). Nuts and bolts of conducting feasibility studies. Am. J. Occup. Ther. 67, 171–176. doi: 10.5014/ajot.2013.006270
Todder, D., Levine, J., Dwolatzky, T., and Kaplan, Z. (2010). Case report: impaired memory and disorientation induced by delta band down-training over the temporal brain regions by neurofeedback treatment. J. Neurother. 14, 153–155. doi: 10.1080/10874201003766785
Twisk, J. W., Rijnhart, J. J., Hoekstra, T., Schuster, N. A., Ter Wee, M. M., and Heymans, M. W. (2020). Intention-to-treat analysis when only a baseline value is available. Contemp. Clin. Trials Commun. 20:100684. doi: 10.1016/j.conctc.2020.100684
Van Doren, J., Arns, M., Heinrich, H., Vollebregt, M. A., and Strehl, U. (2019). Sustained effects of neurofeedback in ADHD: a systematic review and meta-analysis. Eur. Child Adolesc. Psychiatry 28, 293–305. doi: 10.1007/s00787-018-1121-4
Vanhatalo, S., Palva, J. M., Holmes, M., Miller, J., Voipio, J., and Kaila, K. (2004). Infraslow oscillations modulate excitability and interictal epileptic activity in the human cortex during sleep. Proc. Natl. Acad. Sci. 101, 5053–5057. doi: 10.1073/pnas.0305375101
Vanhatalo, S., Voipio, J., and Kaila, K. (2005). Full-band EEG (FbEEG): an emerging standard in electroencephalography. Clin. Neurophysiol. 116, 1–8. doi: 10.1016/j.clinph.2004.09.015
Vanneste, S., and De Ridder, D. (2021). Chronic pain as a brain imbalance between pain input and pain suppression. Brain Commun. 3:fcab014. doi: 10.1093/braincomms/fcab014
Vanneste, S., Ost, J., Van Havenbergh, T., and De Ridder, D. (2017). Resting state electrical brain activity and connectivity in fibromyalgia. PLoS One 12:e0178516. doi: 10.1371/journal.pone.0178516
Vanneste, S., Song, J. J., and De Ridder, D. (2018). Thalamocortical dysrhythmia detected by machine learning. Nat. Commun. 9:1103. doi: 10.1038/s41467-018-02820-0
Vogt, B. A., and Sikes, R. W. (2000). The medial pain system, cingulate cortex, and parallel processing of nociceptive information. Prog. Brain Res. 122, 223–235. doi: 10.1016/S0079-6123(08)62141-X
Vongsirinavarat, M., Nilmart, P., Somprasong, S., and Apinonkul, B. (2020). Identification of knee osteoarthritis disability phenotypes regarding activity limitation: a cluster analysis. BMC Musculoskelet Dis. 21:237. doi: 10.1186/s12891-020-03260-y
Weber, L. A., Ethofer, T., and Ehlis, A. C. (2020). Predictors of neurofeedback training outcome: a systematic review. Neuroimage Clin. 27:102301. doi: 10.1016/j.nicl.2020.102301
Wiech, K., Farias, M., Kahane, G., Shackel, N., Tiede, W., and Tracey, I. (2008). An fMRI study measuring analgesia enhanced by religion as a belief system. PAIN 139, 467–476. doi: 10.1016/j.pain.2008.07.030
Wilson, R., and Abbott, J. H. (2019). The projected burden of knee osteoarthritis in new zealand: healthcare expenditure and total joint replacement provision. N. Z. Med. J. 132, 53–65.
Yam, M. F., Loh, Y. C., Tan, C. S., Khadijah Adam, S., Abdul Manan, N., and Basir, R. (2018). General pathways of pain sensation and the major neurotransmitters involved in pain regulation. Int. J. Mol. Sci. 19:2164. doi: 10.3390/ijms19082164
Yang, L., Nan, W., Qu, X., Wan, F., Mak, P. I., Mak, P. U., et al. (2015). Beta/theta ratio neurofeedback training effects on the spectral topography of EEG. Ann. Int. Conf. IEEE Eng. Med. Biol. Soc. 2015, 4741–4744.
Yang, X., Sun, J., Chen, T. L., Jian, F., Ye, N. S., Long, H., et al. (2015). Amplitude of low-frequency fluctuation of BOLD signal and resting-state functional connectivity analysis of brains in patients with orthodontic pain. J. Med. Imaging Health Inform. 5, 1548–1552. doi: 10.1166/jmihi.2015.1559
Yu, Y. H., Chen, S. H., Chang, C. L., Lin, C. T., Hairston, W. D., and Mrozek, R. A. (2016). New flexible silicone-based eeg dry sensor material compositions exhibiting improvements in lifespan, conductivity, and reliability. Sensors 16:1826. doi: 10.3390/s16111826
Yusuf, E. (2016). Pharmacologic and non-pharmacologic treatment of osteoarthritis. Curr. Treat. Opti. Rheumatol. 2, 111–125. doi: 10.1007/s40674-016-0042-y
Zhang, B., Jung, M., Tu, Y., Gollub, R., Lang, C., Ortiz, A., et al. (2019). Identifying brain regions associated with the neuropathology of chronic low back pain: a resting-state amplitude of low-frequency fluctuation study. Br. J. Anaesth 123, e303–e311. doi: 10.1016/j.bja.2019.02.021
Zhang, Y., Mao, Z., Pan, L., Ling, Z., Liu, X., Zhang, J., et al. (2019). Frequency-specific alterations in cortical rhythms and functional connectivity in trigeminal neuralgia. Brain Imag. Behav. 13, 1497–1509. doi: 10.1007/s11682-019-00105-8
Zhou, F., Gu, L., Hong, S., Liu, J., Jiang, J., Huang, M., et al. (2018). Altered low-frequency oscillation amplitude of resting state-fMRI in patients with discogenic low-back and leg pain. J. Pain Res. 11, 165–176. doi: 10.2147/JPR.S151562
Keywords: infraslow oscillation, knee osteoarthritis, neurofeedback, pain neuromodulation, safety, feasibility
Citation: Mathew J, Adhia DB, Smith ML, De Ridder D and Mani R (2022) Source localized infraslow neurofeedback training in people with chronic painful knee osteoarthritis: A randomized, double-blind, sham-controlled feasibility clinical trial. Front. Neurosci. 16:899772. doi: 10.3389/fnins.2022.899772
Received: 19 March 2022; Accepted: 01 July 2022;
Published: 28 July 2022.
Edited by:
Reinhold Scherer, University of Essex, United KingdomReviewed by:
Cynthia Kerson, Saybrook University, United StatesCopyright © 2022 Mathew, Adhia, Smith, De Ridder and Mani. This is an open-access article distributed under the terms of the Creative Commons Attribution License (CC BY). The use, distribution or reproduction in other forums is permitted, provided the original author(s) and the copyright owner(s) are credited and that the original publication in this journal is cited, in accordance with accepted academic practice. No use, distribution or reproduction is permitted which does not comply with these terms.
*Correspondence: Jerin Mathew, amVyaW4ubWF0aGV3QG90YWdvLmFjLm56
†Present Address: Jerin Mathew, Department of Anatomy, School of Biomedical Sciences, University of Otago, Dunedin, New Zealand
Disclaimer: All claims expressed in this article are solely those of the authors and do not necessarily represent those of their affiliated organizations, or those of the publisher, the editors and the reviewers. Any product that may be evaluated in this article or claim that may be made by its manufacturer is not guaranteed or endorsed by the publisher.
Research integrity at Frontiers
Learn more about the work of our research integrity team to safeguard the quality of each article we publish.