- 1Division of Life Science, State Key Laboratory of Molecular Neuroscience and Molecular Neuroscience Center, The Hong Kong University of Science and Technology, Hong Kong, China
- 2Hong Kong Center for Neurodegenerative Diseases, Hong Kong Science Park, Hong Kong, China
- 3Guangdong Provincial Key Laboratory of Brain Science, Disease and Drug Development, HKUST Shenzhen Research Institute, Shenzhen–Hong Kong Institute of Brain Science, Shenzhen, China
The high prevalence of Alzheimer’s disease (AD) among the elderly population and its lack of effective treatments make this disease a critical threat to human health. Recent epidemiological and genetics studies have revealed the polygenic nature of the disease, which is possibly explainable by a polygenic score model that considers multiple genetic risks. Here, we systemically review the rationale and methods used to construct polygenic score models for studying AD. We also discuss the associations of polygenic risk scores (PRSs) with clinical outcomes, brain imaging findings, and biochemical biomarkers from both the brain and peripheral system. Finally, we discuss the possibility of incorporating polygenic score models into research and clinical practice along with potential challenges.
Introduction
Alzheimer’s disease (AD), an aging-related neurodegenerative disease and the most common form of dementia, is a health threat to societies worldwide. AD has a complex etiology that is influenced by both genetic and environmental factors, which account for its variable risk among individuals. The presence of known coding mutations located in APP and PSEN genes that exhibit extremely high disease penetrance for early-onset AD can be determined by genetic analysis well before disease onset. Moreover, sporadic late-onset AD (LOAD), which accounts for most AD cases, is suggested to be highly heritable (approximately 60–80%) in the general population (Gatz et al., 2006). Therefore, studying individual genomes might identify individuals at high risk of developing AD, create a time window for intervention, and aid the development of intervention strategies.
However, genome-wide association studies (GWASs) of LOAD have only revealed a few dozen genetic risk loci with mild or moderate disease risk-modifying effects; individually, these cannot adequately explain an individual’s risk of having AD at the population level (Lambert et al., 2013; Jansen et al., 2019). The inconsistencies among epidemiological studies regarding the high heritability of LOAD as well as the lack of causal genetic factors that adequately explain disease risk imply that LOAD has a polygenic nature: its risk might be modulated by the aggregate effects of many hidden variants as well as environmental factors. Accordingly, given that polygenic risk analysis has recently become a key facet in cohort studies of LOAD, herein we systemically review the current approaches to polygenic risk analysis along with their applications in AD.
Key Elements of Polygenic Score Models
Polygenic score models consider the aggregate effects of multiple variants to evaluate genetic contributions to continuous or discrete traits—for instance, gene expression levels or disease status (Chatterjee et al., 2016). Hence, polygenic score models require knowledge about which variants modify the disease in question. Variants are normally selected by screening the summary statistics generated by GWASs with proper filtering of the association p-values. Various p-value thresholds can be applied (e.g., 0.0001, 0.01, or 0.5) to obtain the pools of variants that exhibit optimal performance for AD classification (Escott-Price et al., 2019b). Meanwhile, several methods have been applied to overcome the redundancy of genetic information (i.e., the effects of the variants on a given disease) due to high linkage disequilibrium among selected variants. For instance, linkage disequilibrium-based pruning, which removes variants in high linkage disequilibrium, or linkage disequilibrium-aware clumping, which simultaneously removes variants in high linkage disequilibrium while retaining variants with the smallest p-values, have been applied to select the most informative variants to construct a polygenic score model. In addition to p-value–based selection, other statistical learning methods such as lasso regression, which can select the most informative variants for AD classification by removing variants minimally associated with the disease, have been also incorporated into polygenic risk analysis for AD (Romero-Rosales et al., 2020; Zhou et al., 2020).
Once the variants for model construction have been determined, their genotype dosages are summarized into a single value that can represent an individual’s status (i.e., their relative risk of having AD). The easiest way to achieve this is to simply sum the number of risk alleles across all selected variants to generate an unweighted polygenic score (Tosto et al., 2017). Meanwhile, two types of weighting measures are commonly introduced into polygenic score models to account for the variable impacts of individual variants on disease risk and generate a more accurate polygenic score model. First, the effect size can be determined from an association test, meta-analysis, or log-transformed odds ratios, thus yielding a weighted polygenic risk score (PRS) model (Tosto et al., 2017). Second, log-transformed hazard ratios generated from association analysis for disease onset age can also be introduced to produce a polygenic hazard score (PHS), which indicates an individual’s instantaneous risk of developing a given disease (Tan et al., 2018).
Nevertheless, introducing statistical learning methods into polygenic risk analysis enables simultaneous variant selection and model construction. Such methods, including lasso regression and support vector machines, can directly learn from the raw genotype data and use the same framework to construct models to predict various outcomes (e.g., phenotypes, cognitive performance, and onset age). Moreover, they may perform better than PRS and PHS models given their ability to better capture both local and global genomic structures.
Overview of Polygenic Score Research for Alzheimer’s Disease
The number of published research articles associated with AD polygenic score models has dramatically increased over the last 15 years (Figure 1A). In 2005, one study reported an AD polygenic score model constructed from nine cholesterol-related single nucleotide polymorphisms (SNPs) including APOE-ϵ4 that exhibited superior performance for classifying AD compared to APOE-ϵ4 alone [area under the receiver operating characteristic curve (AUC) = 0.74 vs. 0.66 for the polygenic score model and APOE-ϵ4, respectively] (Papassotiropoulos et al., 2005). That study was also the first to demonstrate the applicability of polygenic score models to predict AD risk—even before AD GWASs demonstrated the polygenic nature of AD.
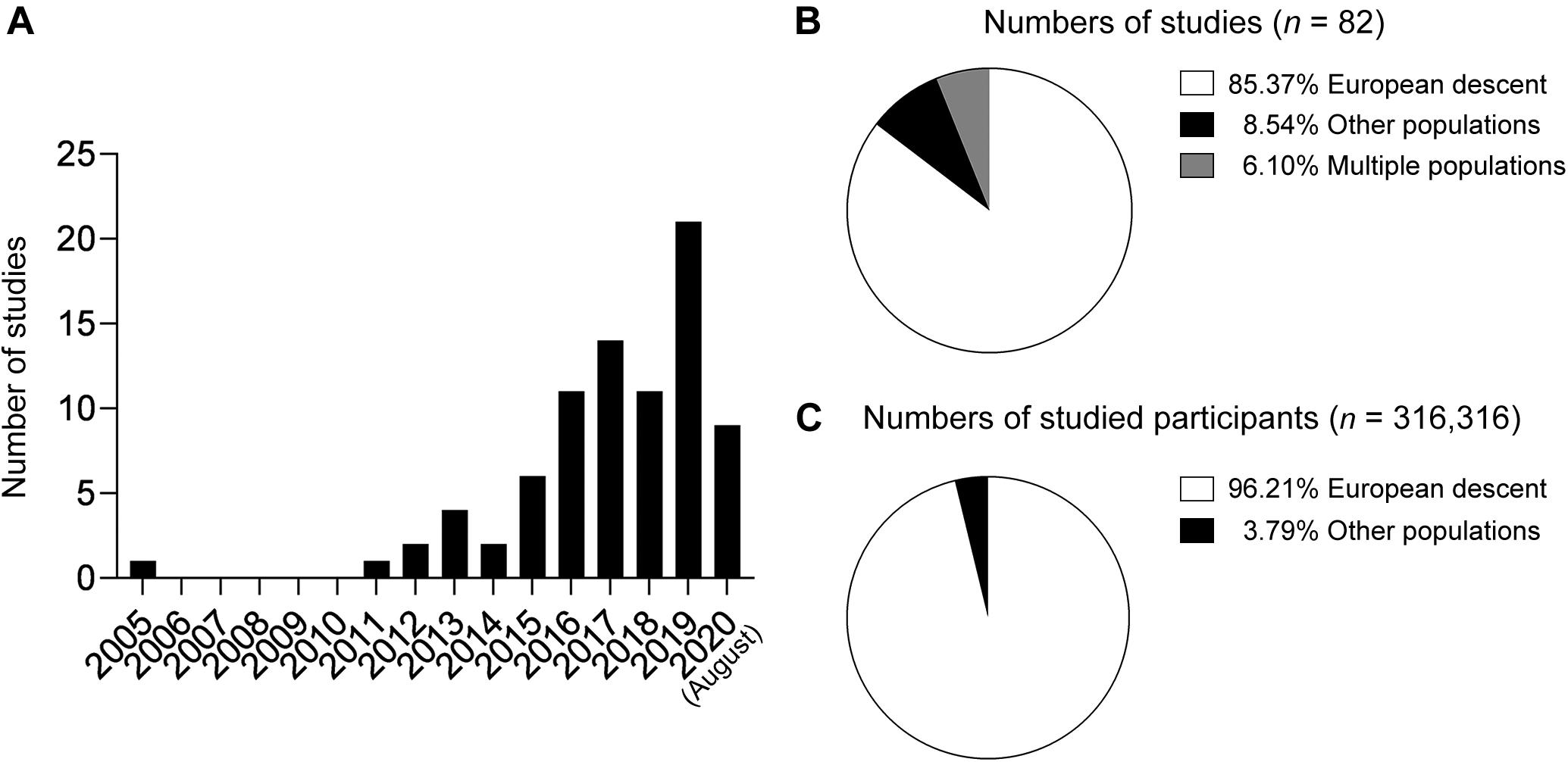
Figure 1. Summary of polygenic score research on Alzheimer’ disease. (A) Numbers of published papers by year. (B) Proportions of studies by population. (C) Proportions of study participants by ethnic group.
Large-scale AD GWASs in populations of European descent bolstered AD polygenic score research in recent years by providing comprehensive information about the effects of individual variants on AD risk at a genome-wide scale. Those studies’ summary statistics, which contain the effect sizes of individual variants, can be directly applied as weighting factors to construct a PRS model. In fact, several AD polygenic risk studies were based on the summary statistics generated by the IGAP Consortium published in 2013 (Lambert et al., 2013) and investigated AD polygenic score models in populations of European descent (Marden et al., 2014, 2016; Escott-Price et al., 2015, 2017a,b, 2019a,b; Habes et al., 2016; Harrison et al., 2016; Louwersheimer et al., 2016; Lupton et al., 2016; Mormino et al., 2016; Walter et al., 2016; Darst et al., 2017; Desikan et al., 2017; Foley et al., 2017; Gibson et al., 2017; Hayes et al., 2017, 2020; Marioni et al., 2017; Morgan et al., 2017; Tan et al., 2017, 2019; Xiao et al., 2017; Axelrud et al., 2018, 2019; Cruchaga et al., 2018; Del-Aguila et al., 2018; Ge et al., 2018; Kauppi et al., 2018, 2020; Patel et al., 2018; Stephan et al., 2018; Tasaki et al., 2018; Andrews et al., 2019; Chaudhury et al., 2019; Elman et al., 2019; Guerreiro et al., 2019; Korologou-Linden et al., 2019a,b; Kremen et al., 2019; Lancaster et al., 2019; Leonenko et al., 2019a,b; Logue et al., 2019; Wang et al., 2019; Yu et al., 2019; Ajnakina et al., 2020; Han et al., 2020; Matloff et al., 2020; Reus et al., 2020; Yesavage et al., 2020). Meanwhile, a few other studies focusing on populations of non-European descent also applied the IGAP data to select variants for genotyping analysis (Marden et al., 2014; Tosto et al., 2017; Axelrud et al., 2018, 2019; Li et al., 2020). Notably, the study populations of most AD polygenic risk studies (Figure 1B) and studied individuals (Figure 1C) were of European descent.
The availability of GWAS results from AD genetics studies has enabled the selection of variants for model construction. Studies using the same IGAP summary statistics can generate models with different numbers of variants (from 6 to 1.1 million sites) by selecting different p-value thresholds (Ajnakina et al., 2020; Han et al., 2020; Reus et al., 2020). Meanwhile, the sample sizes used for polygenic score models also vary among studies: from less than 80 to more than 20,000 participants (Desikan et al., 2017; Chandler et al., 2019). Regarding model construction, PLINK and PRSice are the most widely used tools to select variants and construct polygenic score models. Other statistical analysis methods, such as linear support vector machine (Filipovych et al., 2012), lasso regression (Romero-Rosales et al., 2020; Zhou et al., 2020), multilocus genotype patterns analysis (Barral et al., 2012), and decision tree (Yokoyama et al., 2015; Porter et al., 2018c), have also been adopted to construct polygenic score models for AD.
Of note, polygenic score models have been implemented to investigate the effects of genetic variants on various aspects of AD pathogenesis and progression. Most studies focus on clinical outcomes, specifically the classification of patients with AD (Papassotiropoulos et al., 2005; Sabuncu et al., 2012; Marden et al., 2014; Adams et al., 2015; Escott-Price et al., 2015, 2017b, 2019b; Yokoyama et al., 2015; Lupton et al., 2016; Tosto et al., 2017; Xiao et al., 2017; Cruchaga et al., 2018; Patel et al., 2018; Chaudhury et al., 2019; Leonenko et al., 2019a,b; Zhang et al., 2019; Altmann et al., 2020; Andrews et al., 2020; Zhou et al., 2020). Some other studies investigated the possible associations between AD polygenic score models and the risk of conversion to AD or mild cognitive impairment (MCI) (Filipovych et al., 2012; Rodríguez-Rodríguez et al., 2013; Verhaaren et al., 2013; Carrasquillo et al., 2015; Desikan et al., 2017; Tosto et al., 2017; Kauppi et al., 2018; Chaudhury et al., 2019; Elman et al., 2019; Ajnakina et al., 2020; Altmann et al., 2020; Andrews et al., 2020), cognitive function (Louwersheimer et al., 2016; Del-Aguila et al., 2018; Ge et al., 2018; Kauppi et al., 2018, 2020; Porter et al., 2018a,c,b; Stephan et al., 2018; Tan et al., 2018, 2019; Tasaki et al., 2018; Korologou-Linden et al., 2019a; Han et al., 2020; Zhou et al., 2020), and memory function (Barral et al., 2012; Verhaaren et al., 2013; Marden et al., 2014, 2016; Adams et al., 2015; Carrasquillo et al., 2015; Mormino et al., 2016; Hayes et al., 2017; Marioni et al., 2017; Axelrud et al., 2018; Ge et al., 2018; Porter et al., 2018a,b,c; Tan et al., 2018, 2019; Altmann et al., 2020). Notably, given that the brain’s structure and functions are closely associated with cognitive ability, several studies have also investigated the use of polygenic score models to predict brain status including changes in brain structure (Sabuncu et al., 2012; Habes et al., 2016; Harrison et al., 2016; Nho et al., 2016; Desikan et al., 2017; Foley et al., 2017; Hayes et al., 2017, 2020; Xiao et al., 2017; Ge et al., 2018; Kauppi et al., 2018; Li et al., 2018; Tasaki et al., 2018; Chandler et al., 2019, 2020; Tan et al., 2019; Wang et al., 2019; Altmann et al., 2020; Matloff et al., 2020; Zhou et al., 2020) and function (Xiao et al., 2017; Axelrud et al., 2019; Chandler et al., 2019, 2020). Moreover, some studies investigated biochemical changes indicative of brain status, such as AD pathological hallmarks including amyloid-beta (Aβ) load and tau tangles (Mormino et al., 2016; Darst et al., 2017; Desikan et al., 2017; Laiterä et al., 2017; Ge et al., 2018; Porter et al., 2018a,b,c; Tan et al., 2018, 2019; Tasaki et al., 2018; Leonenko et al., 2019a; Yu et al., 2019; Altmann et al., 2020), enzyme activity in brain samples (Martiskainen et al., 2015; Laiterä et al., 2017), and levels of proteins (e.g., the “ATN” biomarker panel, which comprises Aβ, tau, and neurofilament light polypeptide) or metabolites (Papassotiropoulos et al., 2005; Sabuncu et al., 2012; Martiskainen et al., 2015; Louwersheimer et al., 2016; Mormino et al., 2016; Darst et al., 2017; Morgan et al., 2017; Cruchaga et al., 2018; Porter et al., 2018a; Tasaki et al., 2018; Korologou-Linden et al., 2019b; Tan et al., 2019; Altmann et al., 2020; Hayes et al., 2020; Li et al., 2020; Reus et al., 2020; Zhou et al., 2020). Some studies also used polygenic score models to evaluate the extent to which certain diseases or pathways modulate AD risk (Papassotiropoulos et al., 2005; Moskvina et al., 2013; Mukherjee et al., 2015; Walter et al., 2016; Gibson et al., 2017; Hayes et al., 2017; Demichele-Sweet et al., 2018; Creese et al., 2019; Elman et al., 2019; Guerreiro et al., 2019; Kremen et al., 2019; Lancaster et al., 2019; Andrews et al., 2020; Yesavage et al., 2020). Collectively, those studies suggest that genetic factors have crucial roles in modifying AD risk and highlight the potential utility of polygenic score models in AD research and routine clinical practice. In the following section, we summarize the key findings of each of those aspects.
Polygenic Score Models for Predicting Alzheimer’s Disease Risk
The primary goal of a polygenic score model is to classify individuals according to disease risk (AD in this case). Numerous studies conducted in recent decades have established various polygenic score models and report their ability to adequately distinguish patients with AD from cognitively normal individuals. Reported AD prediction accuracy ranges from an AUC of 0.57 (Tosto et al., 2017) to 0.84 (Escott-Price et al., 2017a). Notably, Yokoyama et al. (2015) generated a PRS using a decision tree model and report an AUC of 0.88 for the prediction of AD (vs. 0.69 for APOE genotype) in their discovery cohort (n = 192). However, this model failed to surpass the accuracy of using APOE genotype to predict AD in their replication cohort (AUC = 0.62 vs. 0.63 for the PRS and APOE genotype, respectively; n = 276). In contrast, several other studies demonstrate that PRS models exhibit superior performance to APOE genotype for predicting AD or associated cognitive states as indicated by significant associations between AD and PRSs that do not include APOE genotype (Sabuncu et al., 2012; Xiao et al., 2015; Leonenko et al., 2019a,b; Zhang et al., 2019) or PRS results after controlling for APOE genotype (Tosto et al., 2017; Escott-Price et al., 2019b). Specifically, in one study recently published by Escott-Price et al. (2019b), the application of a PRS to homozygous APOE-ϵ3 carriers achieved an AUC of 0.831 for the prediction of AD with a comparable AUC of 0.834 after excluding the variants in the APOE region in homozygous APOE-ϵ3 carriers. Thus, polygenic effects might account for the non-APOE–dependent genetic mechanisms of AD pathogenesis. Meanwhile, a whole-exome sequencing study conducted by Patel et al. (2018) revealed the applicability of polygenic score models using exonic variants to predict AD, yielding an AUC of 0.830 for AD prediction with the inclusion of APOE genotype, age, sex, and 19 GWAS-identified SNPs, further implying the polygenic contribution of the exonic regions to the modulation of AD risk.
In addition to disease risk, a few studies investigated the possible contribution of polygenic risk to the modulation of the likelihood of AD conversion, specifically conversion from MCI to AD (Rodríguez-Rodríguez et al., 2013; Tan et al., 2017; Kauppi et al., 2018; Chaudhury et al., 2019) or conversion from cognitive normality to MCI or AD (Carrasquillo et al., 2015; Tan et al., 2017; Logue et al., 2019; Altmann et al., 2020), or the time to develop AD (Verhaaren et al., 2013; Desikan et al., 2017; Tosto et al., 2017; Ajnakina et al., 2020; Andrews et al., 2020). Of note, Tan et al. (2017) studied 1,081 asymptomatic elderly adults and report a PHS model based on 31 SNPs selected from IGAP and ADGC phase 1 data that can accurately predict the risk of conversion from cognitive normality to AD (hazard ratio = 2.36), from MCI to AD (hazard ratio = 1.17), and from cognitive normality or MCI to AD (hazard ratio = 1.31). Furthermore, Kauppi et al. (2018) integrated the PHS with cognitive score and brain atrophy status, resulting in relatively high accuracy for predicting conversion from MCI to AD (AUC = 0.84).
Notably, Carrasquillo et al. (2015) suggest that only APOE-inclusive PRSs are correlated with the likelihood of developing MCI or AD in a longitudinally assessed cohort. Moreover, Rodríguez-Rodríguez et al. (2013) also report that conversion from MCI to AD cannot be successfully predicted by PRSs after controlling for age, sex, and APOE genotype. However, the models in both studies included fewer than 10 non-APOE variants. Meanwhile, by integrating more variants into the analysis, Altmann et al. (2020) observed significant associations between AD polygenic risk and clinical conversion from non-demented to demented status as well as Clinical Dementia Rating Scale Sum of Boxes (CDR-SB) score after excluding the effect of the APOE locus. Therefore, the polygenic risk effects from non-APOE loci probably contribute to the likelihood of AD development and progression.
Polygenic Score Models for Predicting Memory and Cognitive Functions
Besides disease states, polygenic risk is also correlated with individual memory function (Barral et al., 2012; Verhaaren et al., 2013; Marden et al., 2014, 2016; Adams et al., 2015; Carrasquillo et al., 2015; Mormino et al., 2016; Hayes et al., 2017; Marioni et al., 2017; Axelrud et al., 2018; Ge et al., 2018; Porter et al., 2018a,b,c; Tan et al., 2018, 2019; Altmann et al., 2020). Specifically, a multilocus mapping analysis conducted by Barral et al. (2012) demonstrates an association between episodic memory and specific genetic patterns from GWAS-identified variants; a few other studies also suggest possible associations between polygenic risk and episodic memory function. Specifically, a PRS study conducted by Marden et al. (2014) suggests that AD polygenic risk might modulate both baseline memory and its rate of decline in people of non-Hispanic European descent (n = 7,172) or African descent (n = 1,081). Again, there is some controversy about the effects of non-APOE polygenic risks on memory function. For instance, Carrasquillo et al. (2015) suggests that only APOE-inclusive PRSs are correlated with worsening memory function, while Verhaaren et al. (2013) and Porter et al. (2018b) report a significant association between non-APOE polygenic risk and memory function. Moreover, Ge et al. (2018) report a significant correlation between high AD polygenic risk and the rate of memory decline after controlling for APOE-ϵ4 genotype. Hence, the polygenic risk effects from non-APOE loci likely also influence memory function.
Polygenic scores can also indicate individual cognitive functions. Several studies report associations between polygenic risk and cognitive functions (Louwersheimer et al., 2016; Del-Aguila et al., 2018; Ge et al., 2018; Kauppi et al., 2018, 2020; Porter et al., 2018a,b,c; Stephan et al., 2018; Tan et al., 2018, 2019; Tasaki et al., 2018; Korologou-Linden et al., 2019a; Han et al., 2020; Zhou et al., 2020). For instance, Korologou-Linden et al. (2019a) report an association between PRS and lower total, verbal, and performance intelligence quotients in childhood and adolescence, and Kauppi et al. (2020) suggest that AD polygenic risk is indicative of individual differences in the rate of cognitive decline in normal aging. Meanwhile, Xiao et al. (2017) and Li et al. (2018) did not identify a significant association between AD polygenic risk and cognitive function in cognitively normal individuals.
Polygenic Score Models for Predicting Brain Status
The associations of polygenic scores with memory and cognitive function imply possible alterations of brain structure and functions. Several studies have examined the associations between AD polygenic risk and MRI findings (Sabuncu et al., 2012; Habes et al., 2016; Harrison et al., 2016; Mormino et al., 2016; Nho et al., 2016; Desikan et al., 2017; Foley et al., 2017; Hayes et al., 2017, 2020; Xiao et al., 2017; Ge et al., 2018; Kauppi et al., 2018; Li et al., 2018; Chandler et al., 2019; Tan et al., 2019; Wang et al., 2019; Altmann et al., 2020; Matloff et al., 2020; Zhou et al., 2020), fMRI findings (Xiao et al., 2017; Axelrud et al., 2019; Chandler et al., 2020), and PET imaging findings (Mormino et al., 2016; Darst et al., 2017; Ge et al., 2018; Porter et al., 2018a,b,c; Tan et al., 2018, 2019; Leonenko et al., 2019a; Altmann et al., 2020).
Notably, some studies have examined the associations between AD polygenic risk and the volumetric changes of various brain regions such as the retrosplenial and posterior cingulate cortices (Sabuncu et al., 2012), frontal cortex (Chandler et al., 2019), entorhinal cortex (Harrison et al., 2016; Desikan et al., 2017; Hayes et al., 2020), amygdala (Lupton et al., 2016; Zhou et al., 2020), and hippocampus (Harrison et al., 2016; Lupton et al., 2016; Desikan et al., 2017; Foley et al., 2017; Xiao et al., 2017; Axelrud et al., 2018; Ge et al., 2018; Lancaster et al., 2019; Altmann et al., 2020; Hayes et al., 2020; Matloff et al., 2020; Zhou et al., 2020).
Interestingly, some studies have focused on individuals of varying ages including young adolescents (Li et al., 2018; Chandler et al., 2019) and elderly people (Lupton et al., 2016; Nho et al., 2016; Darst et al., 2017; Desikan et al., 2017; Tan et al., 2019; Hayes et al., 2020). Specifically, Li et al. (2018) and Chandler et al. (2019) report significant associations of AD polygenic risk with gray matter cerebral blood flow and gray matter volume, respectively, in young individuals, indicating a potential long-term effect of polygenic risk on brain function well before AD onset.
In addition to structural changes, AD polygenic risk might be associated with brain Aβ load (Mormino et al., 2016; Darst et al., 2017; Porter et al., 2018a,c; Tan et al., 2018, 2019; Leonenko et al., 2019a; Altmann et al., 2020) as measured by PET imaging. Moreover, several studies discuss the possible effects of polygenic risk on brain functional changes including hippocampal activation (Xiao et al., 2017; Chandler et al., 2020) and connectivity between specific brain regions (Axelrud et al., 2019), providing additional evidence for the effects of AD polygenic risk on brain function. Meanwhile, Aβ measured by PET imaging has been introduced to stratify AD patients prior to PRS evaluation (Porter et al., 2018b).
Polygenic Score Models for Predicting Biochemical Changes in the Brain and Peripheral System
Corroborating PET imaging findings, AD polygenic risk is also associated with the levels of several hallmark proteins of AD in postmortem brain tissues. For instance, AD PRSs are reported to be significantly correlated with Aβ and tau tangle levels (Tasaki et al., 2018), although some studies did not identify such a correlation between AD polygenic risk and Aβ levels (Laiterä et al., 2017; Yu et al., 2019). Notably, AD polygenic risk might be correlated with the activity of brain γ-secretase (but not β-secretase) (Martiskainen et al., 2015; Laiterä et al., 2017) as well as levels of VGF, IGFBP5, and STX1A in brain tissues as measured by proteomic analysis (Tasaki et al., 2018).
As the ATN biomarkers in cerebrospinal fluid (CSF) are correlated with the brain pathology in AD, several studies also suggest possible correlations between PRSs and CSF biomarkers including Aβ (Sabuncu et al., 2012; Martiskainen et al., 2015; Darst et al., 2017; Cruchaga et al., 2018; Hayes et al., 2020; Li et al., 2020) and tau or p-tau (Louwersheimer et al., 2016; Darst et al., 2017; Cruchaga et al., 2018; Porter et al., 2018a; Tan et al., 2018; Altmann et al., 2020; Li et al., 2020; Reus et al., 2020). However, Louwersheimer et al. (2016) and Mormino et al. (2016) did not observe a correlation between AD polygenic risk and CSF Aβ levels. Meanwhile, Reus et al. (2020) examined the associations between polygenic risk and 412 CSF proteins and protein fragments, and found that 48.8% of the candidate proteins were associated with at least one of the 14 constructed scores, implying a possible global alteration of the CSF proteome that is possibly associated with polygenic risk.
Notably, a recent study also implies the involvement of the peripheral immune system in AD pathogenesis (Zhou et al., 2018), while other studies demonstrate associations between AD polygenic risk and plasma proteins (Morgan et al., 2017; Korologou-Linden et al., 2019b; Zhou et al., 2020) or metabolites (Papassotiropoulos et al., 2005; Korologou-Linden et al., 2019b). Specifically, by applying the proximity extension assay to plasma proteomic analysis, we investigated 280 proteins and revealed potential protein candidates (i.e., osteopontin and neurocan core protein) along with a protein network associated with AD polygenic risk—again implying global changes in plasma profiles that might be modulated by polygenic risk (Zhou et al., 2020).
Polygenic Score Models for Examining the Involvement of Other Diseases in Alzheimer’s Disease Pathogenesis
The complex etiology of AD is reflected by the identification of various modifiable risk factors such as cardiovascular risk factors, hypertension, and immune factors. Polygenic score models suggest that AD genetic risks are associated with cholesterol levels (Papassotiropoulos et al., 2005), depression (Gibson et al., 2017), schizophrenia (Demichele-Sweet et al., 2018; Creese et al., 2019), frontotemporal lobar degeneration, amyotrophic lateral sclerosis (Adams et al., 2015), insulin sensitivity (Walter et al., 2016), microglial dysfunction (Lancaster et al., 2019), and mitochondrial dysfunction (Andrews et al., 2020). Meanwhile, the polygenic risks for cardiovascular risk factors, frontotemporal lobar degeneration, and amyotrophic lateral sclerosis are implicated in the pathogenesis of MCI (Adams et al., 2015; Elman et al., 2019). Thus, these findings collectively suggest the underlying mechanisms of AD comorbidities and indicate possible pathways for intervention.
Applications and Potential Issues
Given the high prevalence of AD, early risk prediction might facilitate early intervention and greatly mitigate the future growth of the AD patient population. Specifically, polygenic risk factors rooted in individual genomes can be used as biomarkers for the early assessment of relative risk at a population scale. To illustrate the utility of such a strategy, delaying disease onset by 5 years would reduce the predicted AD population among people aged 70 years or above by 41% in the United States. in 2050 (Zissimopoulos et al., 2015). Furthermore, inspiring work by Solomon et al. (2018) further suggests that lifestyle interventions might override the risk effects of APOE-ϵ4, implying a possible means of delaying AD onset once an individual is informed of their relative risk of developing AD. Moreover, a recent study revealed that prior knowledge of genetic risk would also be critical for drug discovery, as drugs targeting proteins encoded in genetic risk loci would be more likely to be successful in phase II and III clinical trials (King et al., 2019). Notably, a polygenic score study of coronary heart disease risk showed that compared to people with lower genetic risk, those with higher genetic risk exhibited a greater decrease in absolute disease risk after receiving statin therapy (Mega et al., 2015). Therefore, conducting population-scale genetic screening for AD might simultaneously support the development of intervention strategies and enable the stratification of individuals according to their risk of AD based on their genetic patterns. More specifically, a hierarchal screening strategy for AD risk evaluation combining genetic, circulatory factors, and brain imaging techniques can be implemented at a populational scale to facilitate disease risk screening and clinical research on personalized interventions in a genotype-aware manner (Figure 2).
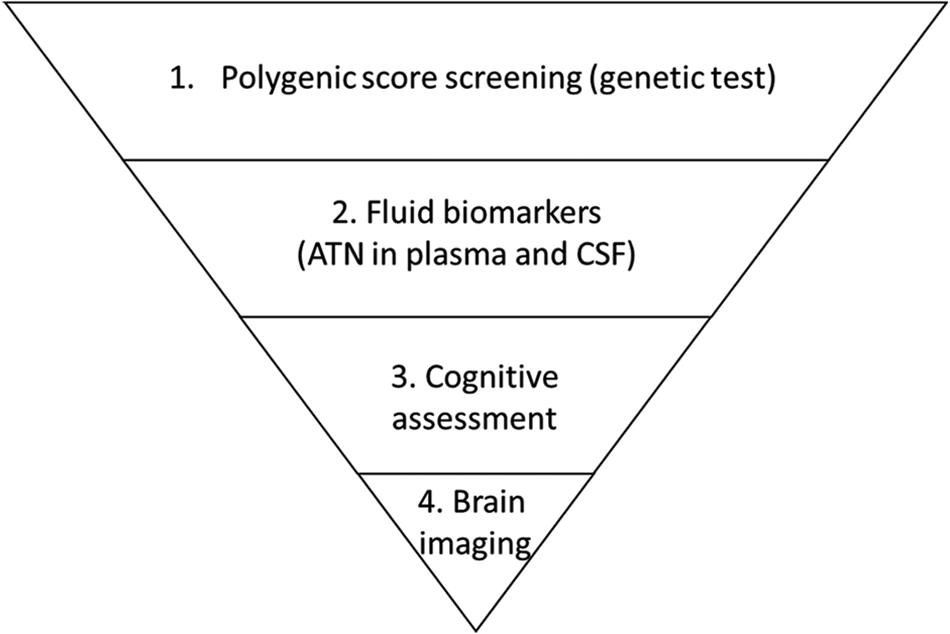
Figure 2. Proposed hierarchal strategy for Alzheimer’s disease risk screening. Individuals enrolled in a screening task are first examined according to genetic risk as indicated by polygenic risk analysis. Individuals who have relatively high risk and report symptoms are referred for biomarker examination to evaluate amyloid-beta, tau (and p-tau), and neurofilament light polypeptide levels (i.e., the “ATN” panel) in blood or cerebrospinal fluid (CSF). Those who exhibit altered levels of biomarkers are further referred to clinicians for cognitive assessment followed by brain imaging including magnetic resonance imaging and positron emission tomography.
Nevertheless, there are potential issues that could hinder the development and implementation of polygenic scoring in routine clinical practice. First, policies protecting patient privacy must be carefully considered, because the results of one person’s genetic test might not only indicate their own risks of certain diseases but also those of their close relatives (Clayton et al., 2019). Second, the possible consequences of informing certain individuals about their estimated genetic risks for certain diseases must be carefully considered, as this could have positive and/or negative outcomes. Fortunately, after receiving brain amyloid imaging, cognitively normal people with elevated amyloid loads tend to make more changes to their lifestyle and future plans than those who do not have elevated amyloid loads (Largent et al., 2020). In addition, in one recent study, providing genetic test results illustrating the 3-year risk of developing AD to patients with MCI did not increase the risk of anxiety or depression (Christensen et al., 2020). Meanwhile, different diagnostic criteria across study cohorts might introduce bias into genetics studies and the subsequent construction of polygenic score models, although this can be reduced or eliminated by further incorporating other biomarkers to refine clinical diagnosis (Escott-Price et al., 2017a). Furthermore, the application of polygenic score models can help refine the results of genetic analyses based on control cohorts (i.e., controls in whom the disease of interest has not been investigated in detail) by ruling out individuals at risk of developing diseases (Escott-Price et al., 2019a). Moreover, polygenic score models may be used to define an individual’s risk of having a specific neurodegenerative disease, as studies have demonstrated that such models (or the genotyping of specific variants) can predict the risk of Parkinson’s disease (Nalls et al., 2016), Huntington’s disease (Kremer et al., 1994), amyotrophic lateral sclerosis (Saez-Atienzar et al., 2021), and multiple sclerosis (The International Multiple Sclerosis Genetics Consortium (IMSGC), 2010). In addition, polygenic score models may help estimate the effects of aging on disease risk. Finally, conducting polygenic risk analysis requires the availability of population-specific genetic risk information at the single-variant level. We previously showed that a polygenic score model based on the Chinese population performs poorly when applied to an AD cohort of European descent (Zhou et al., 2020). The poor performance of that polygenic score model can be explained by the differences in the genomic structures between populations of East-Asian and European descent. Given that there are limited AD GWASs on populations of non-European descent (Zhou et al., 2018; Kunkle et al., 2020), it is critical to comprehensively analyze AD genetic risk in such populations to facilitate the development of polygenic score models and their associated applications in populations worldwide.
Author Contributions
XZ, AF, and NI outlined and wrote the review. XZ and YL conducted the literature review and data organization. All authors contributed to the article and approved the submitted version.
Funding
This work was supported in part by the Research Grants Council of Hong Kong [the Theme-Based Research Scheme (T13-607/12R) and the Collaborative Research Fund (C6027-19GF)], the National Key R&D Program of China (2017YFE0190000 and 2018YFE0203600), the Areas of Excellence Scheme of the University Grants Committee (AoE/M-604/16), the Innovation and Technology Commission (ITCPD/17-9), the Guangdong Provincial Key S&T Program (2018B030336001), and the Shenzhen Knowledge Innovation Program (JCYJ20180507183642005 and JCYJ20170413173717055).
Conflict of Interest
The authors declare that the research was conducted in the absence of any commercial or financial relationships that could be construed as a potential conflict of interest.
The handling editor declared a past collaboration with one of the authors NI.
Acknowledgments
We would like to thank Kin Y. Mok for constructive suggestions on the manuscript.
References
Adams, H. H. H., De Bruijn, R. F. A. G., Hofman, A., Uitterlinden, A. G., Van Duijn, C. M., Vernooij, M. W., et al. (2015). Genetic risk of neurodegenerative diseases is associated with mild cognitive impairment and conversion to dementia. Alzheimer’s Dement. 11, 1277–1285. doi: 10.1016/j.jalz.2014.12.008
Ajnakina, O., Cadar, D., and Steptoe, A. (2020). Interplay between socioeconomic markers and polygenic predisposition on timing of dementia diagnosis. J. Am. Geriatr. Soc. 68, 1529–1536. doi: 10.1111/jgs.16406
Altmann, A., Scelsi, M. A., Shoai, M., de Silva, E., Aksman, L. M., Cash, D. M., et al. (2020). A comprehensive analysis of methods for assessing polygenic burden on Alzheimer’s disease pathology and risk beyond APOE. Brain Commun. 2:fcz047. doi: 10.1093/braincomms/fcz047
Andrews, S. J., Fulton-Howard, B., Patterson, C., McFall, G. P., Gross, A., Michaelis, E. K., et al. (2020). Mitonuclear interactions influence Alzheimer’s disease risk. Neurobiol. Aging 87, 138.e7–138.e14. doi: 10.1016/j.neurobiolaging.2019.09.007
Andrews, S. J., McFall, G. P., Booth, A., Dixon, R. A., and Anstey, K. J. (2019). Association of Alzheimer’s disease genetic risk loci with cognitive performance and decline: a systematic review. J. Alzheimer’s Dis. 69, 1109–1136. doi: 10.3233/JAD-190342
Axelrud, L. K., Santoro, M. L., Pine, D. S., Talarico, F., Gadelha, A., Manfro, G. G., et al. (2018). Polygenic risk score for Alzheimer’s disease: Implications for memory performance and hippocampal volumes in early life. Am. J. Psychiatry 175, 555–563. doi: 10.1176/appi.ajp.2017.17050529
Axelrud, L. K., Sato, J. R., Santoro, M. L., Talarico, F., Pine, D. S., Rohde, L. A., et al. (2019). Genetic risk for Alzheimer’s disease and functional brain connectivity in children and adolescents. Neurobiol. Aging 82, 10–17. doi: 10.1016/j.neurobiolaging.2019.06.011
Barral, S., Bird, T., Goate, A., Farlow, M. R., Diaz-Arrastia, R., Bennett, D. A., et al. (2012). Genotype patterns at PICALM, CR1, BIN1, CLU, and APOE genes are associated with episodic memory. Neurology 78, 1464–1471. doi: 10.1212/WNL.0b013e3182553c48
Carrasquillo, M. M., Crook, J. E., Pedraza, O., Thomas, C. S., Pankratz, V. S., Allen, M., et al. (2015). Late-onset Alzheimer’s risk variants in memory decline, incident mild cognitive impairment, and Alzheimer’s disease. Neurobiol. Aging 36, 60–67. doi: 10.1016/j.neurobiolaging.2014.07.042
Chandler, H. L., Hodgetts, C. J., Caseras, X., Murphy, K., and Lancaster, T. M. (2020). Polygenic risk for Alzheimer’s disease shapes hippocampal scene-selectivity. Neuropsychopharmacology 45, 1171–1178. doi: 10.1038/s41386-019-0595-1
Chandler, H. L., Wise, R. G., Murphy, K., Tansey, K. E., Linden, D. E. J., and Lancaster, T. M. (2019). Polygenic impact of common genetic risk loci for Alzheimer’s disease on cerebral blood flow in young individuals. Sci. Rep. 9:467. doi: 10.1038/s41598-018-36820-3
Chatterjee, N., Shi, J., and García-Closas, M. (2016). Developing and evaluating polygenic risk prediction models for stratified disease prevention. Nat. Rev. Genet. 17, 392–406. doi: 10.1038/nrg.2016.27
Chaudhury, S., Brookes, K. J., Patel, T., Fallows, A., Guetta-Baranes, T., Turton, J. C., et al. (2019). Alzheimer’s disease polygenic risk score as a predictor of conversion from mild-cognitive impairment. Transl. Psychiatry 9:154. doi: 10.1038/s41398-019-0485-7
Christensen, K. D., Karlawish, J., Roberts, J. S., Uhlmann, W. R., Harkins, K., Wood, E. M., et al. (2020). Disclosing genetic risk for Alzheimer’s dementia to individuals with mild cognitive impairment. Alzheimer’s Dement. Transl. Res. Clin. Interv. 6:e12002. doi: 10.1002/trc2.12002
Clayton, E. W., Evans, B. J., Hazel, J. W., and Rothstein, M. A. (2019). The law of genetic privacy: applications, implications, and limitations. J. Law Biosci. 6, 1–36. doi: 10.1093/jlb/lsz007
Creese, B., Vassos, E., Bergh, S., Athanasiu, L., Johar, I., Rongve, A., et al. (2019). Examining the association between genetic liability for schizophrenia and psychotic symptoms in Alzheimer’s disease. Transl. Psychiatry 9:273. doi: 10.1038/s41398-019-0592-5
Cruchaga, C., Del-Aguila, J. L., Saef, B., Black, K., Fernandez, M. V., Budde, J., et al. (2018). Polygenic risk score of sporadic late-onset Alzheimer’s disease reveals a shared architecture with the familial and early-onset forms. Alzheimer’s Dement. 14, 205–214. doi: 10.1016/j.jalz.2017.08.013
Darst, B. F., Koscik, R. L., Racine, A. M., Oh, J. M., Krause, R. A., Carlsson, C. M., et al. (2017). Pathway-specific polygenic risk scores as predictors of amyloid-β deposition and cognitive function in a sample at increased risk for Alzheimer’s disease. J. Alzheimer’s Dis. 55, 473–484. doi: 10.3233/JAD-160195
Del-Aguila, J. L., Fernández, M. V., Schindler, S., Ibanez, L., Deming, Y., Ma, S., et al. (2018). Assessment of the genetic architecture of Alzheimer’s disease risk in rate of memory decline. J. Alzheimer’s Dis. 62, 745–756. doi: 10.3233/JAD-170834
Demichele-Sweet, M. A. A., Weamer, E. A., Klei, L., Vrana, D. T., Hollingshead, D. J., Seltman, H. J., et al. (2018). Genetic risk for schizophrenia and psychosis in Alzheimer disease. Mol. Psychiatry 23, 963–972. doi: 10.1038/mp.2017.81
Desikan, R. S., Fan, C. C., Wang, Y., Schork, A. J., Cabral, H. J., Cupples, L. A., et al. (2017). Genetic assessment of age-associated Alzheimer disease risk: development and validation of a polygenic hazard score. PLoS Med. 14:e1002258. doi: 10.1371/journal.pmed.1002258
Elman, J. A., Panizzon, M. S., Logue, M. W., Gillespie, N. A., Neale, M. C., Reynolds, C. A., et al. (2019). Genetic risk for coronary heart disease alters the influence of Alzheimer’s genetic risk on mild cognitive impairment. Neurobiol. Aging 84, 237.e5–237.e12. doi: 10.1016/j.neurobiolaging.2019.06.001
Escott-Price, V., Baker, E., Shoai, M., Leonenko, G., Myers, A. J., Huentelman, M., et al. (2019a). Genetic analysis suggests high misassignment rates in clinical Alzheimer’s cases and controls. Neurobiol. Aging 77, 178–182. doi: 10.1016/j.neurobiolaging.2018.12.002
Escott-Price, V., Myers, A. J., Huentelman, M., and Hardy, J. (2017a). Polygenic risk score analysis of pathologically confirmed Alzheimer disease. Ann. Neurol. 82, 311–314. doi: 10.1002/ana.24999
Escott-Price, V., Myers, A., Huentelman, M., Shoai, M., and Hardy, J. (2019b). Polygenic risk score analysis of Alzheimer’s disease in cases without APOE4 or APOE2 alleles. J. Prev. Alzheimer’s Dis. 6, 16–19. doi: 10.14283/jpad.2018.46
Escott-Price, V., Shoai, M., Pither, R., Williams, J., and Hardy, J. (2017b). Polygenic score prediction captures nearly all common genetic risk for Alzheimer’s disease. Neurobiol. Aging 49:214.e7. doi: 10.1016/j.neurobiolaging.2016.07.018
Escott-Price, V., Sims, R., Bannister, C., Harold, D., Vronskaya, M., Majounie, E., et al. (2015). Common polygenic variation enhances risk prediction for Alzheimer’s disease. Brain 138, 3673–3684. doi: 10.1093/brain/awv268
Filipovych, R., Gaonkar, B., and Davatzikos, C. (2012). “A composite multivariate polygenic and neuroimaging score for prediction of conversion to Alzheimer’s disease,” in Proceedings of the 2012 2nd International Workshop on Pattern Recognition in NeuroImaging, PRNI 2012 (London), 105–108. doi: 10.1109/PRNI.2012.9
Foley, S. F., Tansey, K. E., Caseras, X., Lancaster, T., Bracht, T., Parker, G., et al. (2017). Multimodal brain imaging reveals structural differences in Alzheimer’s disease polygenic risk carriers: a study in healthy young adults. Biol. Psychiatry 81, 154–161. doi: 10.1016/j.biopsych.2016.02.033
Gatz, M., Reynolds, C. A., Fratiglioni, L., Johansson, B., Mortimer, J. A., Berg, S., et al. (2006). Role of genes and environments for explaining Alzheimer disease. Arch. Gen. Psychiatry 63, 168–174. doi: 10.1001/archpsyc.63.2.168
Ge, T., Sabuncu, M. R., Smoller, J. W., Sperling, R. A., and Mormino, E. C. (2018). Dissociable influences of APOE «4 and polygenic risk of AD dementia on amyloid and cognition. Neurology 90, E1605–E1612. doi: 10.1212/WNL.0000000000005415
Gibson, J., Russ, T. C., Adams, M. J., Clarke, T. K., Howard, D. M., Hall, L. S., et al. (2017). Assessing the presence of shared genetic architecture between Alzheimer’s disease and major depressive disorder using genome-wide association data. Transl. Psychiatry 7, e1094–e1098. doi: 10.1038/tp.2017.49
Guerreiro, R., Escott-Price, V., Hernandez, D. G., Kun-Rodrigues, C., Ross, O. A., Orme, T., et al. (2019). Heritability and genetic variance of dementia with Lewy bodies. Neurobiol. Dis. 127, 492–501. doi: 10.1016/j.nbd.2019.04.004
Habes, M., Janowitz, D., Erus, G., Toledo, J. B., Resnick, S. M., Doshi, J., et al. (2016). Advanced brain aging: relationship with epidemiologic and genetic risk factors, and overlap with Alzheimer disease atrophy patterns. Transl. Psychiatry 6:e775. doi: 10.1038/tp.2016.39
Han, S. H., Roberts, J. S., Mutchler, J. E., and Burr, J. A. (2020). Volunteering, polygenic risk for Alzheimer’s disease, and cognitive functioning among older adults. Soc. Sci. Med. 253:112970. doi: 10.1016/j.socscimed.2020.112970
Harrison, T. M., Mahmood, Z., Lau, E. P., Karacozoff, A. M., Burggren, A. C., Small, G. W., et al. (2016). An Alzheimer’s disease genetic risk score predicts longitudinal thinning of hippocampal complex subregions in healthy older adults. eNeuro 3, 795–804. doi: 10.1523/ENEURO.0098-16.2016
Hayes, J. P., Logue, M. W., Sadeh, N., Spielberg, J. M., Verfaellie, M., Hayes, S. M., et al. (2017). Mild traumatic brain injury is associated with reduced cortical thickness in those at risk for Alzheimer’s disease. Brain 140, 813–825. doi: 10.1093/brain/aww344
Hayes, J. P., Moody, J. N., Roca, J. G., and Hayes, S. M. (2020). Body mass index is associated with smaller medial temporal lobe volume in those at risk for Alzheimer’s disease. NeuroImage Clin. 25:102156. doi: 10.1016/j.nicl.2019.102156
Jansen, I. E., Savage, J. E., Watanabe, K., Bryois, J., Williams, D. M., Steinberg, S., et al. (2019). Genome-wide meta-analysis identifies new loci and functional pathways influencing Alzheimer’s disease risk. Nat. Genet. 51, 404–413. doi: 10.1038/s41588-018-0311-9
Kauppi, K., Fan, C. C., McEvoy, L. K., Holland, D., Tan, C. H., Chen, C. H., et al. (2018). Combining polygenic hazard score with volumetric MRI and cognitive measures improves prediction of progression from mild cognitive impairment to Alzheimer’s disease. Front. Neurosci. 12:260. doi: 10.3389/fnins.2018.00260
Kauppi, K., Rönnlund, M., Nordin Adolfsson, A., Pudas, S., and Adolfsson, R. (2020). Effects of polygenic risk for Alzheimer’s disease on rate of cognitive decline in normal aging. Transl. Psychiatry 10:250. doi: 10.1038/s41398-020-00934-y
King, E. A., Wade Davis, J., and Degner, J. F. (2019). Are drug targets with genetic support twice as likely to be approved? Revised estimates of the impact of genetic support for drug mechanisms on the probability of drug approval. PLoS Genet. 15:e1008489. doi: 10.1371/journal.pgen.1008489
Korologou-Linden, R., Anderson, E. L., Jones, H. J., Davey Smith, G., Howe, L. D., and Stergiakouli, E. (2019a). Polygenic risk scores for Alzheimer’s disease, and academic achievement, cognitive and behavioural measures in children from the general population. Int. J. Epidemiol. 48, 1972–1980. doi: 10.1093/ije/dyz080
Korologou-Linden, R., O’Keeffe, L., Howe, L. D., Davey-Smith, G., Jones, H. J., Anderson, E. L., et al. (2019b). Polygenic risk score for Alzheimer’s disease and trajectories of cardiometabolic risk factors in children. Wellcome Open Res. 4:125. doi: 10.12688/wellcomeopenres.15359.1
Kremen, W. S., Panizzon, M. S., Elman, J. A., Granholm, E. L., Andreassen, O. A., Dale, A. M., et al. (2019). Pupillary dilation responses as a midlife indicator of risk for Alzheimer’s disease: association with Alzheimer’s disease polygenic risk. Neurobiol. Aging 83, 114–121. doi: 10.1016/j.neurobiolaging.2019.09.001
Kremer, B., Goldberg, P., Andrew, S. E., Theilmann, J., Telenius, H., Zeisler, J., et al. (1994). A worldwide study of the Huntington’s disease mutation: the sensitivity and specificity of measuring CAG repeats. N. Engl. J. Med. 330, 1401–1406. doi: 10.1056/nejm199405193302001
Kunkle, B. W., Schmidt, M., Klein, H. U., Naj, A. C., Hamilton-Nelson, K. L., Larson, E. B., et al. (2020). Novel Alzheimer disease risk loci and pathways in African American individuals using the African genome resources panel a meta-analysis. JAMA Neurol. 78, 102–113. doi: 10.1001/jamaneurol.2020.3536
Laiterä, T., Paananen, J., Helisalmi, S., Sarajärvi, T., Huovinen, J., Laitinen, M., et al. (2017). Effects of Alzheimer’s disease-associated risk loci on amyloid-β accumulation in the brain of idiopathic normal pressure hydrocephalus patients. J. Alzheimer’s Dis. 55, 995–1003. doi: 10.3233/JAD-160554
Lambert, J. C., Ibrahim-Verbaas, C. A., Harold, D., Naj, A. C., Sims, R., Bellenguez, C., et al. (2013). Meta-analysis of 74,046 individuals identifies 11 new susceptibility loci for Alzheimer’s disease. Nat. Genet. 45, 1452–1458. doi: 10.1038/ng.2802
Lancaster, T. M., Hill, M. J., Sims, R., and Williams, J. (2019). Microglia – mediated immunity partly contributes to the genetic association between Alzheimer’s disease and hippocampal volume. Brain Behav. Immun. 79, 267–273. doi: 10.1016/j.bbi.2019.02.011
Largent, E. A., Harkins, K., Van Dyck, C. H., Hachey, S., Sankar, P., and Karlawish, J. (2020). Cognitively unimpaired adults’ reactions to disclosure of amyloid PET scan results. PLoS One 15:e0229137. doi: 10.1371/journal.pone.0229137
Leonenko, G., Shoai, M., Bellou, E., Sims, R., Williams, J., Hardy, J., et al. (2019a). Genetic risk for Alzheimer disease is distinct from genetic risk for amyloid deposition. Ann. Neurol. 86, 427–435. doi: 10.1002/ana.25530
Leonenko, G., Sims, R., Shoai, M., Frizzati, A., Bossù, P., Spalletta, G., et al. (2019b). Polygenic risk and hazard scores for Alzheimer’s disease prediction. Ann. Clin. Transl. Neurol. 6, 456–465. doi: 10.1002/acn3.716
Li, J., Zhang, X., Li, A., Liu, S., Qin, W., Yu, C., et al. (2018). Polygenic risk for Alzheimer’s disease influences precuneal volume in two independent general populations. Neurobiol. Aging 64, 116–122. doi: 10.1016/j.neurobiolaging.2017.12.022
Li, W. W., Wang, Z., Fan, D. Y., Shen, Y. Y., Chen, D. W., Li, H. Y., et al. (2020). Association of polygenic risk score with age at onset and cerebrospinal fluid biomarkers of Alzheimer’s disease in a Chinese Cohort. Neurosci. Bull. 36, 696–704. doi: 10.1007/s12264-020-00469-8
Logue, M. W., Panizzon, M. S., Elman, J. A., Gillespie, N. A., Hatton, S. N., Gustavson, D. E., et al. (2019). Use of an Alzheimer’s disease polygenic risk score to identify mild cognitive impairment in adults in their 50s. Mol. Psychiatry 24, 421–430. doi: 10.1038/s41380-018-0030-8
Louwersheimer, E., Wolfsgruber, S., Espinosa, A., Lacour, A., Heilmann-Heimbach, S., Alegret, M., et al. (2016). Alzheimer’s disease risk variants modulate endophenotypes in mild cognitive impairment. Alzheimer’s Dement. 12, 872–881. doi: 10.1016/j.jalz.2016.01.006
Lupton, M. K., Strike, L., Hansell, N. K., Wen, W., Mather, K. A., Armstrong, N. J., et al. (2016). The effect of increased genetic risk for Alzheimer’s disease on hippocampal and amygdala volume. Neurobiol. Aging 40, 68–77. doi: 10.1016/j.neurobiolaging.2015.12.023
Marden, J. R., Mayeda, E. R., Walter, S., Vivot, A., Tchetgen, E. J. T., Kawachi, I., et al. (2016). Using an Alzheimer disease polygenic risk score to predict memory decline in black and white Americans over 14 years of follow-up. Alzheimer Dis. Assoc. Disord. 30, 195–202. doi: 10.1097/WAD.0000000000000137
Marden, J. R., Walter, S., Tchetgen Tchetgen, E. J., Kawachi, I., and Glymour, M. M. (2014). Validation of a polygenic risk score for dementia in black and white individuals. Brain Behav. 4, 687–697. doi: 10.1002/brb3.248
Marioni, R. E., Campbell, A., Hagenaars, S. P., Nagy, R., Amador, C., Hayward, C., et al. (2017). Genetic stratification to identify risk groups for Alzheimer’s disease. J. Alzheimer’s Dis. 57, 275–283. doi: 10.3233/JAD-161070
Martiskainen, H., Helisalmi, S., Viswanathan, J., Kurki, M., Hall, A., Herukka, S. K., et al. (2015). Effects of Alzheimer’s disease-associated risk loci on cerebrospinal fluid biomarkers and disease progression: a polygenic risk score approach. J. Alzheimer’s Dis. 43, 565–573. doi: 10.3233/JAD-140777
Matloff, W. J., Zhao, L., Ning, K., Conti, D. V., and Toga, A. W. (2020). Interaction effect of alcohol consumption and Alzheimer disease polygenic risk score on the brain cortical thickness of cognitively normal subjects. Alcohol 85, 1–12. doi: 10.1016/j.alcohol.2019.11.002
Mega, J. L., Stitziel, N. O., Smith, J. G., Chasman, D. I., Caulfield, M. J., Devlin, J. J., et al. (2015). Genetic risk, coronary heart disease events, and the clinical benefit of statin therapy: an analysis of primary and secondary prevention trials. Lancet 385, 2264–2271. doi: 10.1016/S0140-6736(14)61730-X
Morgan, A. R., Touchard, S., O’Hagan, C., Sims, R., Majounie, E., Escott-Price, V., et al. (2017). The correlation between inflammatory biomarkers and polygenic risk score in Alzheimer’s disease. J. Alzheimer’s Dis. 56, 25–36. doi: 10.3233/JAD-160889
Mormino, E. C., Sperling, R. A., Holmes, A. J., Buckner, R. L., De Jager, P. L., Smoller, J. W., et al. (2016). Polygenic risk of Alzheimer disease is associated with early- and late-life processes. Neurology 87, 481–488. doi: 10.1212/WNL.0000000000002922
Moskvina, V., Harold, D., Russo, G. C., Vedernikov, A., Sharma, M., Saad, M., et al. (2013). Analysis of genome-wide association studies of Alzheimer disease and of Parkinson disease to determine if these 2 diseases share a common genetic risk. JAMA Neurol. 70, 1268–1276. doi: 10.1001/jamaneurol.2013.448
Mukherjee, S., Walter, S., Kauwe, J. S. K., Saykin, A. J., Bennett, D. A., Larson, E. B., et al. (2015). Genetically predicted body mass index and Alzheimer’s disease-related phenotypes in three large samples: Mendelian randomization analyses. Alzheimer’s Dement. 11, 1439–1451. doi: 10.1016/j.jalz.2015.05.015
Nalls, M. A., Keller, M. F., Hernandez, D. G., Chen, L., Stone, D. J., and Singleton, A. B. (2016). Baseline genetic associations in the Parkinson’s progression markers initiative (PPMI). Mov. Disord. 31, 79–85. doi: 10.1002/mds.26374
Nho, K., Saykin, A. J., and Nelson, P. T. (2016). Hippocampal sclerosis of aging, a common Alzheimer’s disease “Mimic”: risk genotypes are associated with brain atrophy outside the temporal lobe. J. Alzheimer’s Dis. 52, 373–383. doi: 10.3233/JAD-160077
Papassotiropoulos, A., Wollmer, M. A., Tsolaki, M., Brunner, F., Molyva, D., Lütjohann, D., et al. (2005). A cluster of cholesterol-related genes confers susceptibility for Alzheimer’s disease. J. Clin. Psychiatry 66, 940–947. doi: 10.4088/jcp.v66n0720
Patel, T., Brookes, K. J., Turton, J., Chaudhury, S., Guetta-Baranes, T., Guerreiro, R., et al. (2018). Whole-exome sequencing of the BDR cohort: evidence to support the role of the PILRA gene in Alzheimer’s disease. Neuropathol. Appl. Neurobiol. 44, 506–521. doi: 10.1111/nan.12452
Porter, T., Burnham, S. C., Milicic, L., Savage, G., Maruff, P., Lim, Y. Y., et al. (2018a). Utility of an Alzheimer’s disease risk-weighted polygenic risk score for predicting rates of cognitive decline in preclinical Alzheimer’s disease: a prospective longitudinal study. J. Alzheimer’s Dis. 66, 1193–1211. doi: 10.3233/JAD-180713
Porter, T., Burnham, S. C., Savage, G., Lim, Y. Y., Maruff, P., Milicic, L., et al. (2018b). A polygenic risk score derived from episodic memory weighted genetic variants is associated with cognitive decline in preclinical Alzheimer’s disease. Front. Aging Neurosci. 10:423. doi: 10.3389/fnagi.2018.00423
Porter, T., Villemagne, V. L., Savage, G., Milicic, L., Ying Lim, Y., Maruff, P., et al. (2018c). Cognitive gene risk profile for the prediction of cognitive decline in presymptomatic Alzheimer’s disease. Pers. Med. Psychiatry 7–8, 14–20. doi: 10.1016/j.pmip.2018.03.001
Reus, L. M., Stringer, S., Posthuma, D., Teunissen, C. E., Scheltens, P., Pijnenburg, Y. A. L., et al. (2020). Degree of genetic liability for Alzheimer’s disease associated with specific proteomic profiles in cerebrospinal fluid. Neurobiol. Aging 93, 144.e1–144.e15. doi: 10.1016/j.neurobiolaging.2020.03.012
Rodríguez-Rodríguez, E., Sánchez-Juan, P., Vázquez-Higuera, J. L., Mateo, I., Pozueta, A., Berciano, J., et al. (2013). Genetic risk score predicting accelerated progression from mild cognitive impairment to Alzheimer’s disease. J. Neural Transm. 120, 807–812. doi: 10.1007/s00702-012-0920-x
Romero-Rosales, B. L., Tamez-Pena, J. G., Nicolini, H., Moreno-Treviño, M. G., and Trevino, V. (2020). Improving predictive models for Alzheimer’s disease using GWAS data by incorporating misclassified samples modeling. PLoS One 15:e0232103. doi: 10.1371/journal.pone.0232103
Sabuncu, M. R., Buckner, R. L., Smoller, J. W., Lee, P. H., Fischl, B., and Sperling, R. A. (2012). The association between a polygenic Alzheimer score and cortical thickness in clinically normal subjects. Cereb. Cortex 22, 2653–2661. doi: 10.1093/cercor/bhr348
Saez-Atienzar, S., Bandres-Ciga, S., Langston, R. G., Kim, J. J., Choi, S. W., Reynolds, R. H., et al. (2021). Genetic analysis of amyotrophic lateral sclerosis identifies contributing pathways and cell types. Sci. Adv. 7:eabd9036. doi: 10.1126/sciadv.abd9036
Solomon, A., Turunen, H., Ngandu, T., Peltonen, M., Levälahti, E., Helisalmi, S., et al. (2018). Effect of the apolipoprotein e genotype on cognitive change during a multidomain lifestyle intervention a subgroup analysis of a randomized clinical trial. JAMA Neurol. 75, 462–470. doi: 10.1001/jamaneurol.2017.4365
Stephan, Y., Sutin, A. R., Luchetti, M., Caille, P., and Terracciano, A. (2018). Polygenic score for Alzheimer disease and cognition: the mediating role of personality. J. Psychiatr. Res. 107, 110–113. doi: 10.1016/j.jpsychires.2018.10.015
Tan, C. H., Bonham, L. W., Fan, C. C., Mormino, E. C., Sugrue, L. P., Broce, I. J., et al. (2019). Polygenic hazard score, amyloid deposition and Alzheimer’s neurodegeneration. Brain 142, 460–470. doi: 10.1093/brain/awy327
Tan, C. H., Fan, C. C., Mormino, E. C., Sugrue, L. P., Broce, I. J., Hess, C. P., et al. (2018). Polygenic hazard score: an enrichment marker for Alzheimer’s associated amyloid and tau deposition. Acta Neuropathol. 135, 85–93. doi: 10.1007/s00401-017-1789-4
Tan, C. H., Hyman, B. T., Tan, J. J. X., Hess, C. P., Dillon, W. P., Schellenberg, G. D., et al. (2017). Polygenic hazard scores in preclinical Alzheimer disease. Ann. Neurol. 82, 484–488. doi: 10.1002/ana.25029
Tasaki, S., Gaiteri, C., Mostafavi, S., De Jager, P. L., and Bennett, D. A. (2018). The molecular and neuropathological consequences of genetic risk for Alzheimer’s dementia. Front. Neurosci. 12:699. doi: 10.3389/fnins.2018.00699
The International Multiple Sclerosis Genetics Consortium (IMSGC) (2010). Evidence for polygenic susceptibility to multiple sclerosis-the shape of things to come. Am. J. Hum. Genet. 86, 621–625. doi: 10.1016/j.ajhg.2010.02.027
Tosto, G., Bird, T. D., Tsuang, D., Bennett, D. A., Boeve, B. F., Cruchaga, C., et al. (2017). Polygenic risk scores in familial Alzheimer disease. Neurology 88, 1180–1186. doi: 10.1212/WNL.0000000000003734
Verhaaren, B. F. J., Vernooij, M. W., Koudstaal, P. J., Uitterlinden, A. G., Van Duijn, C. M., Hofman, A., et al. (2013). Alzheimer’s disease genes and cognition in the nondemented general population. Biol. Psychiatry 73, 429–434. doi: 10.1016/j.biopsych.2012.04.009
Walter, S., Marden, J. R., Kubzansky, L. D., Mayeda, E. R., Crane, P. K., Chang, S. C., et al. (2016). Diabetic phenotypes and late-life dementia risk: a mechanism-specific mendelian randomization study. Alzheimer Dis. Assoc. Disord. 30, 15–20. doi: 10.1097/WAD.0000000000000128
Wang, T., Han, Z., Yang, Y., Tian, R., Zhou, W., Ren, P., et al. (2019). Polygenic risk score for Alzheimer’s disease is associated with Ch4 volume in normal subjects. Front. Genet. 10:519. doi: 10.3389/fgene.2019.00519
Xiao, E., Chen, Q., Goldman, A. L., Tan, H. Y., Healy, K., Zoltick, B., et al. (2017). Late-onset Alzheimer’s disease polygenic risk profile score predicts hippocampal function. Biol. Psychiatry Cogn. Neurosci. Neuroimaging 2, 673–679. doi: 10.1016/j.bpsc.2017.08.004
Xiao, Q., Liu, Z. J., Tao, S., Sun, Y. M., Jiang, D., Li, H. L., et al. (2015). Risk prediction for sporadic Alzheimer’s disease using genetic risk score in the Han Chinese population. Oncotarget 6, 36955–36964. doi: 10.18632/oncotarget.6271
Yesavage, J. A., Noda, A., Heath, A., McNerney, M. W., Domingue, B. W., Hernandez, Y., et al. (2020). Sleep-wake disorders in Alzheimer’s disease: further genetic analyses in relation to objective sleep measures. Int. Psychogeriatr. 32, 807–813. doi: 10.1017/S1041610219001777
Yokoyama, J. S., Bonham, L. W., Sears, R. L., Klein, E., Karydas, A., Kramer, J. H., et al. (2015). Decision tree analysis of genetic risk for clinically heterogeneous Alzheimer’s disease. BMC Neurol. 15:47. doi: 10.1186/s12883-015-0304-6
Yu, L., Petyuk, V. A., Tasaki, S., Boyle, P. A., Gaiteri, C., Schneider, J. A., et al. (2019). Association of cortical β-amyloid protein in the absence of insoluble deposits with Alzheimer disease. JAMA Neurol. 76, 818–826. doi: 10.1001/jamaneurol.2019.0834
Zhang, C., Hu, R., Zhang, G., Zhe, Y., Hu, B., He, J., et al. (2019). A weighted genetic risk score based on four APOE-independent Alzheimer’s disease risk loci may supplement APOE E4 for better disease prediction. J. Mol. Neurosci. 69, 433–443. doi: 10.1007/s12031-019-01372-2
Zhou, X., Chen, Y., Ip, F. C. F., Lai, N. C. H., Li, Y. Y. T., Jiang, Y., et al. (2020). Genetic and polygenic risk score analysis for Alzheimer’s disease in the Chinese population. Alzheimer’s Dement. 12:e12074. doi: 10.1002/dad2.12074
Zhou, X., Chen, Y., Mok, K. Y., Zhao, Q., Chen, K., Chen, Y., et al. (2018). Identification of genetic risk factors in the Chinese population implicates a role of immune system in Alzheimer’s disease pathogenesis. Proc. Natl. Acad. Sci. U.S.A. 115, 1697–1706. doi: 10.1073/pnas.1715554115
Keywords: Alzheimer’s disease, polygenic score, APOE, genetics, polygenic risk score, polygenic hazard score, risk prediction
Citation: Zhou X, Li YYT, Fu AKY and Ip NY (2021) Polygenic Score Models for Alzheimer’s Disease: From Research to Clinical Applications. Front. Neurosci. 15:650220. doi: 10.3389/fnins.2021.650220
Received: 06 January 2021; Accepted: 09 March 2021;
Published: 29 March 2021.
Edited by:
Jie Tu, Chinese Academy of Sciences (CAS), ChinaReviewed by:
Sam Gandy, Icahn School of Medicine at Mount Sinai, United StatesChongzhao Ran, Harvard Medical School, United States
Copyright © 2021 Zhou, Li, Fu and Ip. This is an open-access article distributed under the terms of the Creative Commons Attribution License (CC BY). The use, distribution or reproduction in other forums is permitted, provided the original author(s) and the copyright owner(s) are credited and that the original publication in this journal is cited, in accordance with accepted academic practice. No use, distribution or reproduction is permitted which does not comply with these terms.
*Correspondence: Nancy Y. Ip, boip@ust.hk