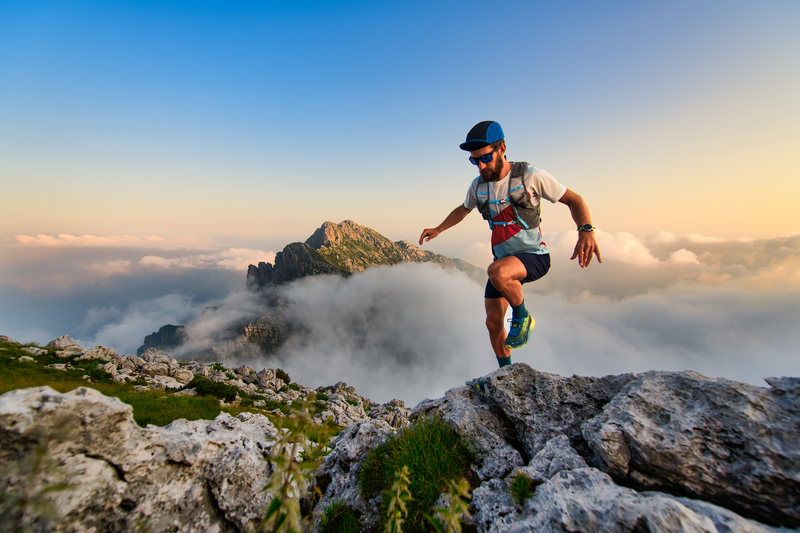
94% of researchers rate our articles as excellent or good
Learn more about the work of our research integrity team to safeguard the quality of each article we publish.
Find out more
ORIGINAL RESEARCH article
Front. Neurorobot. , 07 June 2010
Volume 4 - 2010 | https://doi.org/10.3389/fnbot.2010.00009
This article is part of the Research Topic Action and language integration in cognitive systems View all 10 articles
Several recent psychological investigations have demonstrated that planning an action biases visual processing. Symes et al. (2008) for example, reported faster target detection for a changing object amongst several non-changing objects following the planning of a target-congruent grasp. The current experimental work investigated how this effect might compare to, and indeed integrate with, effects of language cues. Firstly a cuing effect was established in its own right using the same change-detection scenes. Sentences cued object size (e.g., “Start looking for a change in the larger objects”), and these successfully enhanced detection of size-congruent targets. Having thereby established two effective sources of bias (i.e., action primes and language cues), the remaining three experiments explored their co-occurrence within the same task. Thus an action prime (participants planned a power or precision grasp) and a language cue (a sentence) preceded stimulus presentation. Based on the tenets of the biased competition model (Desimone and Duncan, 1995), various predictions were made concerning the integration of these different biases. All predictions were supported by the data, and these included reliably stronger effects of language, and concurrent biasing effects that were mutually suppressive and additive.
“Indeed, the general point is that attention greatly reduces the processing load for animal and robot. The catch, of course, is that reducing computing load is a Pyrrhic victory unless the moving focus of attention captures those aspects of behavior relevant for the current task…” (Arbib et al., 2008 , p. 1461).
The present paper examines how current behavioral targets that are defined explicitly through language, or implicitly through action intentions, might serve to bias object representations and ultimately selective attention. Moreover, the experimental work investigates how the biasing effects of these two different sources might integrate. The biased competition model (Desimone and Duncan, 1995 ) serves as the theoretical backdrop and is used to generate experimental predictions regarding the integration of language and action as sources of representational bias. The data reported later on support all of the predictions made by the model.
In the real world, action intentions or action plans typically refer to object-related goal states that can be broken down into various stages. For example, the specific intention to turn on a lamp may require planning to walk across the room and planning to reach toward and grasp its switch. At all of these stages, the relevant action plan depends to an extent on the goal object itself, whether it be its location (thus implicating walking direction), or its intrinsic properties (thus implicating grasp aperture, for instance). In the experimental world of the present studies however, we interchangeably use the terms action intentions or action plans to simply refer to a pre-activated motor system. Schütz-Bosbach et al. (2007) refer to similar states of preparedness between selecting and executing an action as “motor attention.” This pre-activation is not necessarily related to a target object (indeed in the change detection task used, an action is prepared but the target object itself is unknown!) All that is relevant therefore, is the prepared state of the motor system.
According to the biased competition model, all visual inputs compete for neuronal representation in multiple visual brain regions (Desimone and Duncan, 1995 ; Desimone, 1998 ; Duncan, 1998 ). Such competition occurs at all stages of visual processing, but most strongly at the level of the neuron’s receptive field (e.g., higher visual areas such as V4). Competition is automatic and ongoing, occurs with and without directed attention, and is characterized by suppressive interactions between stimuli. Enhanced amplitude and duration of responses to one object are associated with decreased responses to others. Evidence of these suppressive interactions comes in part from single-cell monkey studies that demonstrate smaller responses for pairs of stimuli falling within a neuron’s receptive field, than for those stimuli presented alone (Luck et al., 1997 ; and see Beck and Kastner, 2009 for a recent review). Importantly, this competition can be resolved by spatially directed attention. Attending to one stimulus in a pair biases competition between objects. Reynolds et al. (1999) reported that monkey neuronal responses were weighted in favor of the attended stimulus of the pair, such that response levels resembled those evoked by that stimulus when it was presented alone (i.e., the suppressive influence of the non-attended stimulus was counteracted). Related findings have been reported in humans using fMRI; here, language instructions explicitly told participants where to direct their attention (Kastner et al., 1998 ).
Contrast gain control has been proposed as one possible mechanism that can account for this attentional biasing (Reynolds et al., 2000 ). Contrast gain control increases the effective salience of the attended stimulus. Monkey V4 neurons for example, responded to an attended stimulus with increased sensitivity, as if its physical contrast had increased (Reynolds et al., 2000 ). Directly adjusting the physical luminance contrast of a stimulus produced equivalent responses (in the absence of attention, V4 neurons were preferentially driven by the higher contrast stimulus in a pair, Reynolds and Desimone, 2003 ). According to the contrast gain account, any effect that attention has on competition depends on where the stimulus falls on the contrast-response function; attention should not increase neuronal sensitivity, for instance, when a high contrast stimulus is at the saturation point on the contrast-response function (Reynolds et al., 2000 ).
As well as the biasing effects of spatially directed attention, the biased competition model also predicts that priming neurons responsive to current behavioral targets can bias competition. Actively searching for a red object, for instance, should bias competition in favor of red objects by preactivating “red” feature coding neurons. These magnified signals suppress the signals from neurons that are selective for other colors (Duncan, 1998 ). Some authors have recently proposed that biased competition could be the mechanism that underlies cases of enhanced visual processing following action planning (see Bekkering and Neggers, 2002 ; Hannus et al., 2005 ; Symes et al., 2008 , 2009 ). Indeed, there is a steadily growing body of behavioral research that suggests that planning an action of some sort (an action intention) affects a range of visual processes. These include selection (e.g., Bekkering and Neggers, 2002 ; Fischer and Hoellen, 2004 ; Hannus et al., 2005 ; Linnell et al., 2005 ); attentional capture (Welsh and Pratt, 2008 ), motion perception (Lindemann and Bekkering, 2009 ); detection through feature weighting (Craighero et al., 1999 ; Symes et al., 2008 , 2009 ); and detection through dimensional weighting (Fagioli et al., 2007 ; Wykowska et al., 2009 ). In such cases, action intentions may serve as the behaviorally relevant prime that preactivates neurons responsive to current behavioral targets.
One example of this that is of particular relevance to the current study comes from Symes et al. (2008) . The authors used a change detection paradigm as a tool for investigating visual processing following action-based priming. Change detection provides an effective means of measuring the locus of focused attention (Simons and Rensink, 2005 ), and the flicker paradigm used can be conceived of as a spatiotemporal version of the static extended displays in visual search experiments (Rensink, 2005 ). In the flicker paradigm, two pictures that are identical in all but one respect (i.e., the change) cycle back and forth, separated by a blank “flicker” that eradicates visual transients associated with the change. Changes can be surprisingly hard to detect (so-called “change blindness”), often taking several cycles. In one experiment (Symes et al., 2008 , Experiment 1b), participants searched for an unknown target amongst 12 graspable objects in a photographed array (the target was one object, such as an apple, being alternated with another size-matched object such as an orange). Prior to the onset of the scene, participants prepared and maintained a grasp plan (either a whole hand “power grasp,” or a thumb and forefinger “precision grasp”). Target detection time was faster when the intended grasp (e.g., power or precision) was compatible with the target (e.g., large or small). Thus planning an action biased object representations and ultimately selective attention of action-appropriate object features. The experiments reported later adopted this same methodology to investigate the interacting influences of action intentions (“action primes”) and language-directed attention (“language cues”).
Although they have almost exclusively been investigated in isolation, in everyday life top-down and bottom-up influences on competition are likely to interact (Beck and Kastner, 2009 ). Reynolds and Desimone (2003) report physiological data that captures an instance of top-down and bottom-up interaction, with the bottom-up bias coming from luminance contrast and the top-down bias coming from directed attention. Neuronal responses were recorded to a stimulus pair consisting of a “good” grating (the neurons responded well to its horizontal orientation) and a “poor” grating (the neurons responded poorly to its vertical orientation). When attending to either stimulus in the pair, attention and contrast were additive influences. Attending to the lower contrast poor stimulus afforded a slight reduction in the response to the pair (i.e., the poor stimulus gained some control over the neural response). Attending to the higher contrast poor stimulus afforded it almost complete control over the response to the pair (effectively eliminating the influence of the good, featurally preferred stimulus).
Based on the above considerations of biased competition, we make the following observations and broad predictions regarding the current behavioral study, which explores the interaction of two top-down influences on visual object representation – one explicit (language cues) and the other implicit (action intentions).
1) As was the case when using action primes (e.g., Symes et al., 2008 , 2009 ), cuing “large” or “small” objects through a single source – this time language instructions – should bias competition in favor of congruently sized objects (presumably by preactivating “large” or “small” feature coding neurons). We expected to find proxy evidence of this in faster detections on trials where the cue and target were size-congruent (i.e., trials with valid cues).
2) Since the language cues specified the targets of directed attention, they were expected to produce a strong biasing influence that is comparable to the influence of directed attention (e.g., Reynolds and Desimone, 2003 ). Relatedly, the biasing influence of action primes is expected to be weaker. This influence arises without directed attention (see Experiment 2a of Symes et al., 2008 ), and in this sense is more comparable to the bottom-up influence of contrast, which also arises without directed attention (e.g., Reynolds and Desimone, 2003 ).
3) In line with the suppressive interactions predicted by the biased competition model, we expected that when there were multiple concurrent weighting sources their effects on object representations would compete.
a. Firstly we expected to find biasing effects for each source that reflected their different relative strengths as described in prediction 2 above.
b. These concurrent effects should be smaller than when they are found independently (i.e., they are mutually suppressive).
c. However, when one weighting source is sufficiently stronger than another, it may even suppress the effect of the weaker source completely. Indeed, the findings of Symes et al. (2009) support this prediction – when bottom-up target saliency was high, action primes were ineffective (see also Wykowska et al., 2009 ).
4) Reynolds and Desimone’s (2003) data revealed that top-down and bottom-up biases produced similar cellular responses that were additive. It therefore follows that the biasing effects of two top-down signals (language and action) should also be additive. Thus we expected the best performance on trials when cue, prime, and target were all congruent.
All experiments were approved by the University of Plymouth’s Human Ethics Committee, and informed consent was obtained from all participants.
Using a flicker paradigm, the experiments reported below attempted to enhance change detection by weighting size-related features of the target in a top-down manner. This weighting was achieved using language cues and action primes. The language cues actually specified overt searching behaviors. Partially valid cues (Experiments 1, 3, and 4), which were valid for half of the time, instructed participants how to start their search (e.g., “Start looking for a change in the larger objects”). Participants were told that if they could not find the target easily, they should include non-cued objects in their search (i.e., they only had to start their search based on the cue). Completely valid cues however (Experiment 2), were always valid, and instructed participants how to conduct their search throughout (e.g., “Look for a change in the larger objects”). The association between the language cue and the object’s size was therefore explicit, whereas for the action prime it was implicit. Furthermore, the action primes did not specify any overt searching behavior. Partially valid (Experiments 2 and 4) or completely valid primes (Experiment 3), simply instructed participants which response device to hold (thereby establishing an action intention for a particular grasp). It is assumed that relative to one another, partially and completely valid cues or primes constituted different weighting strengths (low and high strengths respectively).
Action primes and language cues therefore provided quite different types of top-down bias, and how their effects might integrate (or not) when biasing competition between objects was at the heart of these investigations. Table 1 summarizes the methods for each experiment in terms of the different action primes and language cues used.
Some of the following methodological details have been adapted from Symes et al. (2008) . Change-blindness scenes consisted of an array of 12 grayscale photographs of fruit and vegetables (half were small objects congruent with a precision grasp, and half were large objects congruent with a power grasp). One random object in the scene changed back and forth into another object of a similar size (e.g., an apple changed into an orange), and this change coincided temporally with a visually disrupting screen flicker that provided the necessary conditions for change blindness. Participants were told that the identity of 1 of the 12 objects would change back and forth, and their basic task was to follow the screen instructions that appeared at the start of a trial, detect the change, indicate detection with a manual response, and then identify the change using the keyboard. Screen instructions typically included a language cue and instructions for planning a manual response (i.e., the action prime). The specific details of these instructions are described for each experiment. The stimuli described below however, were used in all four experiments (and Symes et al., 2008 ).
Change detection scenes arose from cyclically presenting a screen “flicker” (F) between an “original” (O) and “modified” (M) picture-pair in the order OFMFOFMF… This sequence cycled until a response was made, and a “change identification” picture was shown to establish that the correct change had been detected. Thus the stimulus set consisted of a flicker stimulus (a blank gray screen), and 60 “original,” “modified” and “change identification” grayscale pictures (1,024 × 768 pixels; 32.5 cm × 24.5 cm; visual angle (VA) ≈ 36.0° × 27.5°). As discernable from Figure 1 (top left panel), each original picture consisted of a 4 × 3 array of six large objects (e.g., an apple) and six small objects (e.g., a strawberry). These had been selected at random from a pool of 24 items of fruit and vegetables, again, half of which were large and half of which were small. The size of each individual object photograph was manipulated such that all small objects were of a similar size (mean VA ≈ 2.3° × 1.6°), and all large objects were of a similar size (mean VA ≈ 4.9° × 4.1°). These objects and their measurements are listed in the Appendix of Symes et al. (2008) .
Figure 1. Schematic illustration of the sequence and timings of the displays in all four experiments (adapted from Symes et al., 2008 ). In Experiment 1, the instruction consisted of only a language cue, whereas in the remaining experiments it consisted of a language cue and an action prime.
The order of objects in the array resulted from a random shuffle of the 12 selected objects, and their positions on the screen varied within a loosely defined grid (thereby creating perceptually distinct-looking scenes). An appropriately sized object (30 of each size were required) was selected at random from the 12 to be the changing object. An appropriately sized replacement object was selected at random from the pool (after object selection for the original picture, six large and six small objects remained in the pool). Thus in the modified picture, all objects remained the same as the original picture, except from a single changing object. This was removed and replaced by an object of a similar size (e.g., a strawberry was replaced with a cherry). Each original picture was also reproduced as a change identification picture, whereby each object in the array had an identification “F-number” (F1–F12) superimposed on it. These F-numbers corresponded to the 12 “F-keys” on a keyboard.
As mentioned earlier, Symes et al. (2008) demonstrated that partially valid action primes enhanced change detection for prime-congruent sized objects. This first experiment was simply designed to establish a similar effect of partially valid language cues using exactly the same stimuli. In terms of the flicker paradigm itself, enhanced detection was expected in principle (explicitly cuing a change with partially valid language cues enhanced detection in a study by Rensink et al., 1997 ).
Preceding stimulus onset a partially valid language cue appeared on the screen (e.g., “Start looking for a change in the larger objects”). Participants were told to follow the text instructions, and that the identity of 1 of the 12 objects would change back and forth. Their basic task was to press the spacebar as soon they detected which object was changing. The first two predictions set out in the Section “Introduction” are relevant for this experiment, and are summarized below:
1) We expected proxy evidence of language cues biasing competition between objects, with faster detections on trials where cue and target were size-congruent.
2) Since language cues told participants where to look, they should have a strong biasing influence like that of directed attention. Relatedly, the biasing effect of action primes (that arises without directed attention) was expected to be weaker. A cross-experimental comparison of effect sizes tested this prediction.
Twenty volunteers between 39 and 18 years of age [mean (M) = 21.8 years] were paid for their participation in a single session that lasted approximately 20 min. Of these, 17 were females (1 left-handed) and 3 were males (all right-handed). All self-reported normal or corrected-to-normal vision and normal motor control, and all were naïve as to the purpose of the study.
Experimental sessions took place in a dimly lit room at a single computer workstation. Situated centrally at the back of the table was a RM Innovator desktop computer that supported a 16-inch RM color monitor (with a screen resolution of 1,024 × 768 pixels and a refresh frequency of 85 Hz). In front of the computer was a keyboard and mouse. The viewing distance was approximately 50 cm, and the hand-to-screen distance was approximately 30 cm. See Section “General Method” for details of the change detection stimuli used.
Four conditions arose from the orthogonal variation of two within-subjects variables, each with two levels: Language Cue (large or small – specifically, “Start looking for a change in the larger/smaller objects”) and Target Size (large or small). At the beginning of the experiment, participants were talked through some written instructions that explained the task. A short practice session of four trials was followed by 120 experimental trials. These consisted of two blocks of 60 trials (4 conditions × 15 replications), with each of the 60 change detection scenes being shown in a random order within each block.
Each trial followed three broad phases: search-and-response preparation, change detection and change identification. Preceding stimulus onset, the language cue appeared center screen and the participant rested the fingertips of both hands on the spacebar of the keyboard (search-and-response preparation phase). The change detection scene then appeared, and as it cycled the participant scrutinized the 12 objects for a change. Upon noticing the change, the participant immediately pressed the spacebar (change detection phase). This response caused the change identification picture to appear, and the participant pressed an F-key on the keyboard corresponding to the F-number of the object they thought they had seen change (change identification phase). Otherwise, it timed-out after 10 s. The sequence and timings for these three phases are illustrated in Figure 1 .
Response times (RTs) and errors were recorded to a data file for off-line analysis, and the possible source of error related to an F-key response that timed-out or did not correspond to the changing object’s F-number.
Errors and RTs more than two standard deviations (SDs) from each participant’s condition means were excluded from this analysis and the analyses of all the other experiments reported. 1.8% of trials were removed as change identification errors (i.e., when an F-key identified the wrong object). No further analysis of errors was undertaken; the change identification error data revealed that on the vast majority of trials the correct object had been identified. 4.6% of the remaining trials were removed as outliers, reducing the maximum detection time from 21,065 to 13,113 ms (M = 4,848 ms; SD = 3,028).
The condition means of the remaining data were computed for each participant and subjected to a repeated measures analysis of variance (ANOVA) with the within-subjects factors of Language Cue (large or small) and Target Size (large or small). An interaction between Language Cue and Target Size was observed, F(1, 19) = 120.11, p < 0.001, that revealed the predicted biasing effect (see prediction 1 above). Mean detection times were faster for large targets following a large (3,622 ms) rather than small (6,131 ms) language cue, and faster for small targets following a small (3,497 ms) rather than large (6,155 ms) language cue.
In order to evaluate whether the biasing effect of language cues was significantly larger than that of action primes obtained in Experiment 1b of Symes et al. (2008) (see prediction 2 above), cropped correct RT data for each experiment were split by participant and cue/prime–target congruent and incongruent trials. From this a mean effect size for each participant in each experiment was calculated (mean effect size = mean incongruent RTs − mean congruent RTs). These data were compared in a one-tailed independent samples t test. This analysis revealed that the mean effect size associated with language cues was indeed significantly larger (current experiment: language cues = 2,579 ms, Experiment 1b: action primes = 372 ms), t(40) = 8.874, p < 0.001.
In order to see whether the biasing effect of language cues behaved consistently across different portions of the RT distribution, the Vincentization procedure (Ratcliff, 1979 ) was used to derive the mean RTs for a new ANOVA (Greenhouse–Geisser corrections for sphericity violations have been used where G is shown). Mean RTs were calculated for five equal bins of rank ordered raw data according to each experimental condition. A statistically significant full interaction, F(1.249, 23.734) = 8.894, p = 0.004G, derived from the resulting 2 × 2 × 5 ANOVA (Language Cue – large or small; Target Size – large or small, and Bin – first to fifth). Unpacking this interaction in separate ANOVAS for each bin revealed that all bins had produced significant cue–target compatibility effects (p < 0.05). In accounting for the significant full interaction however, it is notable that the effect sizes were smaller in the first and last bins (Bin 1 = 1,805 ms, Bin 5 = 1,151 ms) than in those in-between (Bins 2, 3, and 4 = 2,645, 2,767, and 2,892 ms, respectively).
Overall, these results supported the two predictions generated by the biased competition model, and suggested that the partially valid language cues had successfully biased object representations and ultimately selective attention. This was the case when detection times were averaged across the whole distribution, and when they were divided into individual bins.
The remaining three experiments presented both cues and primes in a variety of validity combinations (return to Table 1 for an overview) to investigate how such different sources of intentional weighting might work together to bias object representations.
In this second experiment, language cues were completely valid and action primes were only partially valid. On any given trial prior to stimulus presentation, a language cue instructed participants how to search for the target (e.g., “Look for a change in the larger objects”), and a separate instruction told participants which grasp-simulating response device to hold and prepare to squeeze (this planned action was the action prime). Participants were told that the identity of 1 of the 12 objects would change back and forth, and their basic task was to search according to the language cue, and to execute their planned grasp as soon as they detected which object was changing.
Prediction 3 set out in the Section “Introduction” is relevant for this experiment, and is summarized below:
3) With two concurrent top-down weighting sources, we expected that their effects on object representations would compete.
a. We therefore expected to find biasing effects for each source that reflected their different relative strengths (i.e., language cue effects are bigger).
b. These effects should be smaller than when found independently (i.e., they are mutually suppressive).
c. However, when one weighting source is sufficiently stronger than another, it may completely suppress the effect of the weaker source.
Given that the already stronger bias of language cues was maximized in this experiment (i.e., they were completely valid cues), it was expected to dominate competition (prediction 3c).
Twenty-one volunteers between 52 and 18 years of age (M = 24.0 years) were paid for their participation in a single session that lasted approximately 20 min. Of these, 15 were right-handed females and 6 were males (1 left-handed). All self-reported normal or corrected-to-normal vision and normal motor control, and all were naïve as to the purpose of the study.
As Experiment 1. In addition, the keyboard was moved closer to the screen (by 15 cm) to make room for the new response apparatus, which was affixed centrally (from left to right) and set in by 10.5 cm from the table’s leading edge. When holding this apparatus, the hand-to-screen distance was approximately 30 cm. The apparatus was fixed to the table top in a vertical position and consisted of two physically connected devices – a cylindrical “power device” (l = 10 cm; diameter = 3 cm), and a square “precision device” (l = 1.25 cm, w = 1.25 cm, h = 1.25 cm). A power grasp was required to hold the power device and a precision grasp was required to hold the precision device. In order to avoid establishing any semantic associations between the devices and their size or required grasp (i.e., ensuring the association between the action prime and the object’s size was implicit), the power device was neutrally referred to by the experimenter (and the on-screen instructions) as the “Black device” (it was colored black) and the precision device as the “White device” (it was colored white). Execution of a particular grasp depressed a micro switch embedded in that device, and this response was registered with millisecond accuracy by the computer (micro switches were connected via an input/output box to the parallel interface of the computer).
Four conditions arose from the orthogonal variation of two within-subjects variables, each with two levels: Action Prime (power or precision) and Language-cued Target Size (large or small). At the beginning of the experiment, participants were talked through some written instructions that explained the task. A short practice session of four trials was followed by 120 experimental trials. These consisted of two blocks of 60 trials (4 conditions × 15 replications), with each of the 60 change detection scenes being shown in a random order within each block.
The trial procedure was similar to Experiment 1 (refer back to Figure 1 ); with three broad phases of search-and-response preparation, change detection and change identification. Preceding stimulus onset this time, the completely valid language cue (e.g., “Look for a change in the larger objects”) appeared above text instructions for the partially valid action prime (which warned participants to prepare a response using either the “Black device” or the “White device”). The participant reached to the instructed device, and held it lightly in their dominant hand (using the device-appropriate hand shape). When the participant detected a change, s/he executed the grasp by squeezing the device. The participant then identified the change as before, by pressing the appropriate F-key.
Response times and errors were recorded to a data file for off-line analysis, and there were two possible sources of error: violations of the response instruction (participants used the wrong device), and change identification errors (an F-key response that timed-out or did not correspond to the changing object’s F-number).
1.87% of trials were removed as errors (0.56% response errors, 1.35% change identification errors, 0.04% both errors on same trial). No further analysis of errors was undertaken; response and change identification error data revealed that on the vast majority of trials the response instructions had been adhered to and the correct object had been identified. 3.92% of the remaining trials were removed as outliers, reducing the maximum detection time from 18,943 to 7,986 ms (M = 2,583 ms; SD = 1,152 ms).
Mean cropped experimental RTs for each participant were compared for the current experiment and Experiment 1b of Symes et al., (2008) in order to establish whether completely valid language cues enhanced overall detection times (see prediction 3a above). The single methodological difference between the two experiments was the presence of a language cue in the current experiment. If this language cue enhanced detection, we should expect faster overall detection times for the current experiment. Indeed, mean experimental RTs were 2,102 ms faster (current experiment grand mean = 2,584 ms, Experiment 1b grand mean = 4,686 ms), and a one-tailed independent samples t test confirmed that this difference was statistically significant, t(41) = 10.156, p < 0.001. [In this instance there is no comparison case to test prediction 3b (whether the effect is smaller than when completely valid cues are the only weighting source)].
The condition means of the cropped data were computed for each participant and subjected to a repeated measures ANOVA with the within-subjects factors of Action Prime (power or precision) and Language-cued Target Size (large or small). The crucial interaction between Action Prime and Language-cued Target Size failed to even approach statistical significance, F(1, 20) = 0.11, p > 0.5. Thus it seems that any biasing effect of action primes was completely suppressed by the dominant completely valid language cues (thereby supporting prediction 3c above).
In order to establish that this (null) effect of action primes was smaller than the biasing effect of action primes in Experiment 1b of Symes et al. (2008) (according to prediction 3b above it should be smaller because it was in competition with another source of bias – a language cue, whereas in Experiment 1b it was not), cropped correct response data for each experiment was split by participant and prime–target congruent and incongruent trials. From this a mean effect size for each participant in each experiment was calculated, and these data were compared in a one-tailed independent samples t test. This analysis revealed that the mean effect size of prime–target compatibility was indeed significantly smaller when it was a shared rather than only source of bias (current experiment: shared source = 4 ms, Experiment 1b: single source = 372 ms), t(41) = 3.360, p = 0.001.
Furthermore, as expected from prediction 3a, the stronger biasing source of Language Cue produced the larger biasing effect of the two (language cue effect = 2,012 ms; action prime effect = 4 ms).
In order to see whether the null effect of action primes in this experiment was consistent across different portions of the RT distribution, distributional analyses were performed (see Experiment 1 for procedural details). A statistically significant full interaction, F(1.232, 24.645) = 4.454, p = 0.038G, derived from the resulting 2 × 2 × 5 ANOVA (Action Prime – power or precision; Language-cued Target Size – large or small, and Bin – first to fifth). Unpacking this interaction in separate ANOVAS for each bin revealed that no significant interactions between Action Prime and Language-cued Target Size were observed under Bins 1, 3, and 4 (p > 0.05). Under Bins 2 and 5 however, some interesting patterns emerged. Under the relatively fast RTs of Bin 2, an interaction resembling a reversed compatibility effect was found, F(1, 20) = 4.271, p = 0.052. Here, mean detection times were actually slower for large-cued targets following a power (1,975 ms) rather than precision (1,796 ms) action prime, and marginally slower for small-cued targets following a small (1,886 ms) rather than large (1,894 ms) action prime. Contrastingly, under the relatively slow RTs of Bin 5, an interaction resembling a compatibility effect was found, F(1, 20) = 3.438, p = 0.079. Here, mean detection times were faster for large-cued targets following a power (5,109 ms) rather than precision (5,299 ms) action prime, and faster for small-cued targets following a small (4,584 ms) rather than large (5,149 ms) action prime. It is plausible that this pattern reflects those longest detection-time cases in which the language cue has lost its potency (i.e., it has not helped participants find the change quickly). In this situation, the language cue weightings no longer dominate suppressive interactions, and hence the action prime is able to exert its influence.
Overall, these results supported the predictions generated by the biased competition model, and suggest that the completely valid language cue had enhanced detection (and dominated competition such that any effect of action primes was completely suppressed in most bins).
This third experiment continued to combine language cues and action primes to investigate how such different sources of intentional weighting might work together to bias object representations. In a reversal of the conditions of the previous experiment, now action primes were completely valid and language cues were only partially valid. On any given trial prior to stimulus presentation, a language cue instructed participants how to commence their search for the target (e.g., “Start looking for a change in the larger objects”), and a separate instruction told participants which response device to hold and prepare to squeeze on target detection (i.e., the action prime).
Prediction 3 set out in the Section “Introduction” is again relevant for this experiment, and is summarized below:
3) With two concurrent top-down weighting sources, we expected that their effects on object representations would compete.
a. We therefore expected to find biasing effects for each source that reflected their different relative strengths (i.e., language cue effects are bigger).
b. These effects should be smaller than when found independently (i.e., they are mutually suppressive).
c. However, when one weighting source is sufficiently stronger than another, it may completely suppress the effect of the weaker source.
Even though action primes were completely valid here, they were not expected to dominate competition in the same way that completely valid language cues did in the previous experiment. This is because they are an inherently weaker source of bias (as formally established in the cross-experimental analysis of Experiment 1). Thus prediction 3c does not apply here.
Twenty-one volunteers between 51 and 18 years of age (M = 21.1 years) were paid for their participation in a single session that lasted approximately 20 min. Of these, 19 were right-handed females and 2 right-handed males. All self-reported normal or corrected-to-normal vision and normal motor control, and all were naïve as to the purpose of the study.
As Experiment 2.
As Experiment 2, differing only in that Action Primes were completely valid, and Language Cues were partially valid. The four conditions that arose from the orthogonal variation of two within-subjects variables, each with two levels were: Language Cue (large or small) and Action-primed Target Size (large or small).
3.29% of trials were removed as errors (0.44% response errors, 2.90% change identification errors, 0.04% both errors on same trial). No further analysis of errors was undertaken. 5.50% of the remaining trials were removed as outliers, reducing the maximum detection time from 27,686 to 13,200 ms (M = 4,087 ms; SD = 2,213).
Mean cropped experimental RTs for each participant were compared for the current experiment and Experiment 1, in order to establish whether completely valid action primes enhanced overall detection times (see prediction 3a above). The only methodological difference between the two experiments was the presence of an action prime in the current experiment (this, along with its associated grasp responses). If this action prime enhanced detection, we should expect faster overall detection times for the current experiment. Indeed, mean experimental RTs were 784 ms faster (current experiment grand mean = 4,082 ms, Experiment 1 grand mean = 4,866 ms), and a one-tailed independent samples t test confirmed that this difference was statistically significant, t(39) = 2.901, p < 0.005. [In this instance there is no comparison case to test prediction 3b (whether the effect is smaller than when completely valid primes are the only weighting source)].
The condition means of the cropped data were computed for each participant and subjected to a repeated measures ANOVA with the within-subjects factors of Language Cue (large or small) and Action-primed Target Size (large or small).
An unexpected main effect of Language Cue, F(1, 20) = 7.041, p < 0.05, reflected faster mean detection times following “large” (3,972 ms) rather than “small” (4,191 ms) cues. The crucial interaction between Language Cue and Action-primed Target Size was also observed, F(1, 20) = 78.609, p < 0.001, revealing the predicted compatibility effect (see prediction 3a above). Mean detection times were faster for large action-primed targets following a large (2,964 ms) rather than small (5,197 ms) language cue, and faster for small action-primed targets following a small (3,186 ms) rather than large (4,980 ms) language cue.
In order to establish whether this biasing effect of language cues was smaller than the biasing effect of language cues in Experiment 1 (according to prediction 3b above it should be smaller because it was in competition with another source of bias – an action prime, whereas in Experiment 1 it was not), cropped correct response data for each experiment was split by participant and prime–target congruent and incongruent trials. From this a mean effect size for each participant in each experiment was calculated, and these data were compared in a one-tailed independent samples t test. This analysis revealed that the mean effect size of cue–target compatibility was indeed significantly smaller when it was a shared rather than only source of bias (current experiment: shared source = 2,013 ms, Experiment 1: single source = 2,579 ms), t(39) = 1.723, p < 0.05.
Furthermore, as expected from prediction 3a, the stronger biasing source of Language Cue produced the larger biasing effect of the two (language cue effect = 2,013 ms; action prime effect = 784 ms).
In order to see whether the significant biasing effect of language cues in this experiment was consistent across different portions of the RT distribution, distributional analyses were performed (see Experiment 1 for procedural details). A statistically significant full interaction, F(1.260, 25.196) = 12.302, p < 0.001G, derived from the resulting 2 × 2 × 5 ANOVA (Language Cue – large or small; Action-primed Target Size – large or small; and Bin – first to fifth). Unpacking this interaction in separate ANOVAS for each bin revealed that the first four bins had all produced highly significant cue–target compatibility effects (p < 0.001). The fifth bin revealed a similar, if diminished, pattern of compatibility (p = 0.103). Overall then, the effect of language cues seemed highly consistent across bins.
Overall, these results again supported the predictions generated by the biased competition model. The presence of a completely valid action prime enhanced detection, but it did not dominate competition (being an inherently weaker source of bias). Thus language cues exerted a consistent effect across the RT distribution, and as predicted, this was a smaller effect than the one generated in Experiment 1 (where languages cues were the only source of bias).
This last experiment combined partially valid language cues with partially valid action primes. The third and fourth predictions set out in the Section “Introduction” are relevant for this experiment, and are summarized below:
3) With two concurrent top-down weighting sources, we expected that their effects on object representations would compete.
a. We therefore expected to find biasing effects for each source that reflected their different relative strengths (i.e., language cue effects are bigger).
b. These effects should be smaller than when found independently (i.e., they are mutually suppressive).
c. However, when one weighting source is sufficiently stronger than another, it may completely suppress the effect of the weaker source.
4) Consistent with other sources of additive bias (Reynolds and Desimone, 2003 ), we expected that the effects of language cues and action primes would be additive. Thus on trials when cue, prime and target were all congruent, we expected the best performance.
Language cues were expected to continue to produce a larger effect than action primes. However, because they were only partially valid they were not necessarily expected to dominate competition. Thus prediction 3c does not apply here (although as it turns out, it does help to explain a later unforeseen result that appeared to arise from the additional influence of a bottom-up source of bias).
Twenty volunteers between 51 and 18 years of age (M = 22.4 years) were paid for their participation in a single session that lasted approximately 20 min. All were right-handed, with 18 females and 2 males. All self-reported normal or corrected-to-normal vision and normal motor control, and all were naïve as to the purpose of the study.
As Experiments 2 and 3.
As Experiments 2 and 3, except that Action Primes and Language Cues were both partially valid. To accommodate this design, the experiment was twice as long, with 240 trials consisting of four blocks of 60 trials, with each of the 60 change detection scenes being shown in a random order within each block (overall, 8 conditions × 30 replications). Eight conditions arose from the orthogonal variation of three within-subjects variables, each with two levels: a) Language Cue (1: large or 2: small), b) Action Prime (1: power or 2: precision), and c) Target Size (1: large or 2: small). These were as follows: 1: a1, b1, c1; 2: a1, b1, c2; 3: a1, b2, c1; 4: a1, b2, c2; 5: a2, b1, c1; 6: a2, b1, c2; 7: a2, b2, c1; 8: a2, b2, c2.
3.6% of trials were removed as errors (0.38% response errors, 3.25% change identification errors, 0.02% both errors on same trial). No further analysis of errors was undertaken. 4.45% of the remaining trials were removed as outliers, reducing the maximum detection time from 56,635 to 16,975 ms (M = 4,203 ms; SD = 2,305).
A coarse-grained analysis was performed as a first look at this more complex data set. Mean RTs were computed from the remaining data for each participant in each of four conditions of target congruence: valid cue + valid prime (e.g., both were target-congruent); valid cue + not valid prime; not valid cue + valid prime; not valid cue + not valid prime. These means were subjected to a repeated measures ANOVA with the within-subjects factors of Cue–Target congruency (congruent or incongruent) and Prime–Target congruency (congruent or incongruent).
Isolating the effects of language cues and action primes. In line with prediction 3a above, separate biasing effects were found for each source as expected, and the stronger biasing source of Language Cue produced the larger effect of the two (by a factor of 15). These biasing effects were reflected by main target-congruency effects of Language Cue, F(1, 19) = 75.582, p < 0.001; and of Action Prime, F(1, 19) = 5.207, p < 0.05. Mean change detections were faster for cue-congruent targets (3,229 ms) than for cue-incongruent targets (5,131 ms); and they were faster for prime-congruent targets (4,122 ms) than for prime-incongruent targets (4,239 ms).
According to prediction 3b, each effect should be smaller here, than when it was found alone. In order to establish whether this was the case for language cues, cropped correct response data for this experiment and Experiment 1 (where language cues were the only source of bias) was split by participant and cue–target congruent and incongruent trials. From this a mean effect size for each participant in each experiment was calculated, and these data were compared in a one-tailed independent samples t test. This analysis revealed that the mean effect size of language cues was indeed significantly smaller when it was a shared rather than only source of bias (current experiment: shared source = 1,901 ms, Experiment 1: single source = 2,579 ms), t(38) = 2.100, p < 0.05. Similarly for action primes, the mean effect size was also significantly smaller when it was a shared rather than only source of bias (current experiment: shared source = 131 ms, Experiment 1b of Symes et al., 2008 : single source = 372 ms), t(40) = 2.105, p < 0.05.
Additive effects of language cues and action primes. Finally, according to prediction 4 above, the biasing effects of language cue and action prime should be additive rather than interactive. The direction of means across the four conditions fully supported an additive model, with detections being driven by valid language cues whilst nevertheless benefiting from concurrently valid action primes (see “All targets” column of Table 2 ). The ANOVA output also supported an additive model, given that there was no significant interaction between Language Cue and Action Prime, F(1, 19) = 1.074, p > 0.10.
In keeping with the condition-specific analyses performed for previous experiments, in this finer-grained analysis the condition means of the cropped data were computed for each participant and subjected to a repeated measures ANOVA with the within-subjects factors of Language Cue (large or small); Action Prime (power or precision) and Target Size (large or small).
A main effect of target size. A main effect of Target Size, F(1, 19) = 5.440, p < 0.05, revealed faster mean change detections for small (4,112 ms) rather than large (4,247 ms) targets. This is a somewhat counter-intuitive finding, since one might expect larger objects to be more salient. Indeed, in testing predictions from the biased competition model, Proulx and Egeth (2008) reported evidence from a singleton paradigm suggesting that similar to increased luminance contrast, increased size contrast also biased competition. Nevertheless, it does seem that smaller objects were genuinely more salient than larger ones in the specific context of the change detection scenes used here. Indeed, using the same scenes, Symes et al. (2008) found a robust and reliable advantage for small targets across several experiments – including an eye-tracking experiment that revealed preferential fixating of smaller objects (see Symes et al., 2008 , for a possible explanation for this). The crucial question here then, is why these apparently salient smaller objects exerted an influence on detection times in this current experiment (and repeatedly in Symes et al., 2008 ), and yet they did not do so in the preceding three experiments?
What each of the preceding three experiments shared in common were relatively stronger sources of top-down bias than were present in this experiment and those of Symes et al. (2008) . In Experiment 1, the top-down bias came from partially valid language cues (which were at their most influential, being the only source of bias). In Experiment 2, sources of bias were completely valid language cues with partially valid action primes, and in Experiment 3, completely valid action primes with partially valid language cues. Relative to these three experiments, top-down sources of bias in the current experiment were at their weakest (two partially valid sources). Similarly, top-down sources of bias in Symes et al. (2008) were relatively weak too, always being partially valid action primes. With these cases of relatively weak top-down biases, we argue that another source of bias (i.e., a bottom-up bias of small objects) was able to successfully compete for neuronal representation. In the previous three experiments, this relatively weak bottom-up bias had presumably been unable to exert an influence in the context of stronger concurrent top-down biases that dominated competition (see prediction 3c above).
Isolating the effects of language cues and action primes. As was the case with the earlier course-grained analysis, the effects of language cues and action primes supported prediction 3a. Target Size interacted separately with both sources of top-down bias. Language Cue by Target Size, F(1, 19) = 75.341, p < 0.001, revealed that mean detection times were faster for large targets following a large (3,238 ms) rather than small (5,256 ms) cue, and faster for small targets following a small (3,220 ms) rather than large (5,005 ms) cue. As already reported above, this effect was significantly smaller as a shared rather than only source of bias (current experiment: shared source = 1,901 ms, Experiment 1: single source = 2,579 ms), t(38) = 2.100, p < 0.05.
Action Prime by Target Size, F(1, 19) = 5.108, p < 0.05, revealed that mean detection times were faster for large targets following a large (4,167 ms) rather than small (4,327 ms) prime, and faster for small targets following a small (4,076 ms) rather than large (4,149 ms) prime. As already reported above, this effect was also significantly smaller when it was a shared rather than only source of bias (current experiment: shared source = 131 ms, Experiment 1b of Symes et al., 2008 : single source = 372 ms), t(40) = 2.105, p < 0.05.
Additive effects of language cues and action primes. Finally, according to prediction 4 above, the effects of language cue and action prime should be additive rather than interactive. The direction of means across the eight conditions supported an additive model, with detections again being driven by valid language cues whilst nevertheless benefiting from concurrently valid action primes (see “Small and Large targets” columns of Table 2 ).
The ANOVA output revealed a three-way interaction between Language Cue, Action Prime and Target Size that was statistically significant at the 10% level, F(1, 19) = 3.840, p = 0.065. In examining this interaction, the biasing effect of action primes appeared stronger under one level of Language Cue – namely “large” cues. Thus with large language cues the effect size was 200 ms, with mean detection times that were faster for large targets following a large (3,088 ms) rather than small (3,388 ms) action prime, and faster for small targets following a small (4,954 ms) rather than large (5,055 ms) prime. However, with small language cues the effect size was only 33 ms, with mean detection times that were similar for large targets following large and small action primes (5,246 and 5,266 ms respectively), and similar for small targets following large and small action primes (3,243 and 3,198 ms respectively).
Interestingly, this finding makes good sense from the perspective of biased competition, and it does not contradict an additive model. We break down our explanation into two related parts:
1) Recall the main effect of Target Size reported earlier – salient small targets were detected faster overall. It was suggested that this bottom-up bias was able to exert a small influence in this experiment because the concurrent sources of top-down bias were relatively weak. This biasing effect of stimulus salience also appeared to be an additive effect. Indeed, the language cue biasing effect was 269 ms larger when cues were congruent rather than incongruent with this visually preferred small stimulus. Similarly, the prime–target effect was 87 ms larger when primes were congruent rather than incongruent with this visually preferred small stimulus.
2) When the effects of stimulus salience, language cues and action primes concurrently contribute to biasing competition between objects for neuronal representation, certain combinations may result in the effects of one source being heavily suppressed – even to the point where it no longer has a biasing influence of its own (see prediction 3c above). Indeed, previous findings from Experiment 2 (and see also Symes et al., 2009 ) indicated that dominant biasing signals completely suppressed the weaker effect of action primes. This appears to have been the case here too. In particular, when the biasing influence of salient small objects co-occurred with the biasing influence of a specific language cue (“Small”), their combined influence dominated, such that action primes could no longer exert any real influence. Indeed, the three-way interaction reported above revealed exactly this pattern – the biasing effect of action primes barely arose under “Small” language cues (in fact as the distributional analysis below reveals, it was not significant in any bin).
Distributional analyses. In order to see whether the weaker biasing effect of action primes was consistent across different portions of the RT distribution, distributional analyses were performed (see Experiment 1 for procedural details). Separate ANOVAS for each bin were performed under each level of Language Cue. Under “Small” language cues, no significant interactions between Action Prime and Language-cued Target Size were observed in any bins (p > 0.10). Under “Large” language cues, signs of an action prime biasing effect began in the first bin, and reached statistical significance in the second and third bins only (p < 0.05). By contrast, the more robust biasing effect of language cues was statistically significant (p < 0.001) in each bin under each level of Action Prime (except for the fifth bin under precision primes, p = 0.063).
Overall, these results comprehensively supported the predictions generated by the biased competition model. Firstly, each source of top-down bias produced its own biasing effect, with language cues producing the larger effect (prediction 3a). Through the suppressive interactions of biased competition, each of these effects was significantly smaller than when found alone (prediction 3b). Furthermore, the two biasing effects of language cues and action primes seemed to be additive (prediction 4). This was transparently the case in the initial course-grained analysis, and it was also the case following a more careful examination in the finer-grained analysis. Here, it was found that a third source of bias (visually salient small objects) had exerted its own bottom-up influence. The combined influence of this bottom-up bias (which also seemed to be an additive effect) and “Small” language cues dominated competition, such that action primes could no longer exert much of an influence (prediction 3c).
Relatively little is known about how multiple sources of bias might interact. It is known that language cues presented with objects can influence the kinematics of actions directed to those objects (e.g., Gentilucci et al., 2000 ; Gentilucci, 2003 ; Lindemann et al., 2006 ). Superimposing the word “large” on an object, for instance, results in increased maximum grip aperture (Glover and Dixon, 2002 ). Relatedly, sentence comprehension appears to evoke motor representations – Glenberg and Kaschak (2002) reported that sentence judgments were faster when the required action response (e.g., moving the hand away from or toward the body) matched the actions implied by the sentence. These insights fit well with broader accounts of embodied cognition that suggest that various sources of activation (whether semantic, visual, motoric) may trigger perceptuo-motor simulations (e.g., Barsalou, 2008 , 2009 ).
The biased competition model is an influential theory of attention proposing that objects compete for neuronal representation via mutually suppressive interactions (Desimone and Duncan, 1995 ). Various top-down and bottom-up factors can bias competition, and some authors have recently suggested that one such top-down factor might be action intentions (e.g., Bekkering and Neggers, 2002 ). The current study explored the effects of two sources of top-down bias on visual object representation – one explicit (language cues) and the other implicit (action intentions).
Using a change-detection flicker paradigm, participants searched for an unknown identity-changing target amongst 12 graspable objects in a photographed array (half were small objects like cherries, half were larger objects like apples). Prior to the onset of the scene, participants received a language cue that advised them to search for the change in “larger” or “smaller” objects, and an action prime that established an action intention to make a power or precision grip (grips that were congruent with large and small objects respectively). Language cues and action primes were either relatively weak sources of bias (partially valid) or stronger sources of bias (completely valid). Experiment 1b of Symes et al. (2008) has previously established enhanced detections following partially valid action primes, and in the current study, four experiments tested a further variety of cue/prime/validity combinations (Experiment 1: partially valid language cues; Experiment 2: completely valid language cues + partially valid action primes; Experiment 3: partially valid language cues + completely valid action primes; Experiment 4: partially valid language cues + partially valid action primes). The predictions derived from the biased competition model, and their related results, are summarized below (and effect sizes across all experiments are displayed graphically in Figure 2 ).
Figure 2. A summary graph of mean effect sizes (mean incongruent RTs − mean congruent RTs) across all experiments. PV, partially valid cue/prime; CV, completely valid cue/prime; * refers to Symes et al. (2008 , Experiment 1b).
1) We expected proxy evidence of the single top-down source of language cues biasing competition, with faster detections on trials where cue and target were size-congruent (i.e., valid trials).
• Experiment 1 (partially valid language cues) found faster detections on trials where cue and target were size-congruent.
2) Since language cues tell participants where to look, they should have a strong biasing influence (like directed attention does). Relatedly, the biasing effect of action primes (that arises without directed attention) is expected to be weaker.
• A cross-experimental comparison of the effects of partially valid action primes (Experiment 1b of Symes et al., 2008 ) and the effects of partially valid language cues (Experiment 1) revealed that language cues had a significantly larger biasing effect than action primes.
3) With two concurrent top-down weighting sources, we expected that their effects on object representations would compete.
a. We therefore expected to find biasing effects for each source that reflected their relative strengths (i.e., stronger language cue effects).
• Experiments 2–4: Language cues had a larger effect than action primes.
b. These effects should be smaller than when found independently (i.e., than when there is only weighting source).
• Experiments 2–4: The biasing effects of partially valid cues and primes were significantly smaller than when found alone. [There were no available experimental comparison cases for completely valid cues and primes].
c. However, when one weighting source is sufficiently stronger than another, it may completely suppress the effect of the weaker source.
• Experiments 1–3: The additive effects of language cues and action primes completely suppressed a weaker bottom-up effect of small object saliency.
• Experiment 2: Completely valid language cues completely suppressed a weaker effect of action primes.
• Experiment 4: The additive effects of small language cues and small object saliency completely suppressed a weaker effect of action primes.
4) Consistent with other sources of additive bias (Reynolds and Desimone, 2003 ), we expected that the effects of language cues and action primes would be additive.
• Experiment 4: Both course-grained and finer-grained analyses supported an additive model (see Table 2 ).
As the above summary makes clear, the various tenets of the biased competition model accounted for all degrees of biasing influence derived from action intentions, including when they produced no effect. While some authors have similarly proposed that biased competition may be the mechanism that underlies cases of enhanced visual processing following action intentions (e.g., Bekkering and Neggers, 2002 ; Hannus et al., 2005 ; Symes et al., 2008 , 2009 ), other authors have proposed alternative models. Most recently, Wykowska et al. (2009) have suggested combining an intentional weighting mechanism (e.g., Hommel et al., 2001 ) with the guided search model (e.g., Wolfe, 1994 ) and the dimensional weighting account (e.g., Müller et al., 1995 ). In explaining the absence of an action-related biasing effect when selection could be based on bottom-up saliency signals alone (cf. similar results of Symes et al., 2009 ), Wykowska et al. (2009 , p. 1767) suggested that,
“Only if a task-relevance bias occurs, will the action-related weighting also influence perceptual processing. In such a case, bottom-up processing will be modulated by the common weight combining task-relevance and action relevance.”
Given the results of the current study, we suggest that the mechanism of biased competition is a sufficient and simpler means of explaining a null-effect of action when stimulus salience is high. We argue this is the case for feature weighting, which our data apply to, although it may also apply to dimension weighting (indeed, Wykowska et al., 2009 suggest that the mechanism underlying dimension weighting may be the same one hypothesized to account for other top-down effects on visual selection). Under our preferred account of this mechanism, there is no common weight that inputs to a master map of activation; rather, ongoing suppressive interactions between objects take place across the various brain regions that represent visual information, (sensory, motor, cortical, and subcortical), Beck and Kastner (2009) . Sometimes a particularly strong weighting signal will dominate competition between objects and completely suppress the effects of other weaker signals (e.g., action signals). Nevertheless, the nature of biased competition is such that any weighting signal, whether top-down or bottom-up, action-based or language-based, competes to influence perceptual processing (indeed, top-down and bottom-up signals seem to produce very similar neuronal responses, Reynolds and Desimone, 2003 ; Reynolds and Chelazzi, 2004 ). Thus action-based sources of bias are not assumed to be “special cases” that only have a modulatory influence that is conditional on higher-order goals (such as a task-relevance bias). To qualify this further, in line with the findings of Reynolds and Desimone (2003) the current results suggested that the various biasing effects were additive, and action-related effects in Experiment 4 for example, were not dependant on task-relevance. Instead they occurred with and without a task-relevance bias (i.e., alongside valid and non-valid language cues).
Itti (2007 , p. 93) captures the essence of the demands that the visual world places on animals (and robots), when he writes;
“Visual processing of complex natural environments requires animals to combine, in a highly dynamic and adaptive manner, sensory signals that originate from the environment (bottom-up) with behavioral goals and priorities dictated by the task at hand (top-down).”
In examining the influences of differently weighted bottom-up and top-down signals, the current series of behavioral experiments revealed a sensitive hierarchy of predicted attentional effects. Such findings serve a “proof-of-principle” role for scientists interested in modeling an embodied neuro-robotic system:
Firstly, the behavioral data suggest that selective perceptual enhancement may be initiated by manual action plans, such as grasping. Although it is perhaps surprising that simply intending to perform an action (even when it is not directed to a known target) might have such diverse influences on an embodied system, complementary neurological evidence does exist. Electrical stimulation of premotor sites within monkey frontal eye fields for example, initiated a bias in the strength of visual signals in corresponding sites of extrastriate visual cortex (Moore and Armstrong, 2003 ). Recent advances in fMRI methods too, shed further light on the role of different brain areas such as pre-frontal cortex, involved in modulating visual signals (Grill-Spector and Sayres, 2008 ).
Secondly, the modulatory influence of action planning appeared to integrate with other sources of bias (such as language) through biased competition – a neural mechanism that is sufficiently well-defined for modeling. Indeed, various neural implementations of biased competition have already simulated a wide range of attentional effects that accommodate both top-down and bottom-up influences (e.g., Sun and Fisher, 2003 ; Lanyon and Denham, 2004a ,b ; Deco and Rolls, 2005 ; see also Spratling, 2008a for a review). While sharing similarities with other influential models of visual processing, the physiologically plausible neural architecture of the biased competition model does recommend it (Spratling, 2008a ,b ). Indeed, it may be more parsimonious than the influential class of saliency map models (e.g., Wolfe, 1994 ; Itti and Koch, 2001 ) in three key areas – it does not require a single map for competition to ultimately be resolved, since ongoing competition occurs across a distributed network of interacting brain regions; it does not assume separate preattentive and attentive stages of perceptual processing; and it does not require separate neural pathways for processing saliency and featural information (see Spratling, 2008b for a discussion of these differences). The current behavioral findings therefore recommend future implementations of the biased competition model in robots, and that these consider including action intentions as a form of top-down bias that reflects the behavioral goals of the robot.
The authors declare that the research was conducted in the absence of any commercial or financial relationships that could be construed as a potential conflict of interest.
This work was supported by a grant from the Economic and Social Research Council (RES-000-23-1497).
Arbib, M., Metta, G., and van der Smagt, P. (2008). “Neurorobotics: from vision to action,” in Springer Handbook of Robotics, eds B. Siciliano and O. Khatib (Berlin/Heidelberg: Springer), 1453–1480.
Barsalou, L. W. (2009). Simulation, situated conceptualization, and prediction. Philos. Trans. R. Soc. Lond., B, Biol. Sci. 364, 1281–1289.
Beck, D. M., and Kastner, S. (2009). Top-down and bottom-up mechanisms in biasing competition in the human brain. Vision Res. 49, 1154–1165.
Bekkering, H., and Neggers, S. F. (2002). Visual search is modulated by action intentions. Psychol. Sci. 13, 370–374.
Craighero, L., Fadiga, L., Rizzolatti, G., and Umiltà, C. (1999). Action for perception: a motor–visual attentional effect. J. Exp. Psychol. Hum. Percept. Perform. 25, 1673–1692.
Deco, G., and Rolls, E. T. (2005). Neurodynamics of biased-competition and cooperation for attention: a model with spiking neurons. J. Neurophysiol. 94, 295–313.
Desimone, R. (1998). Visual attention mediated by biased competition in extrastriate visual cortex. Philos. Trans. R. Soc. Lond., B, Biol. Sci. 353, 1245–1255.
Desimone, R., and Duncan, J. (1995). Neural mechanisms of selective visual attention. Annu. Rev. Neurosci. 18, 193–222.
Duncan, J. (1998). Converging levels of analysis in the cognitive neuroscience of visual attention. Philos. Trans. R. Soc. Lond., B, Biol. Sci. 353, 1307–1317.
Fagioli, S., Hommel, B., and Schubotz, R. I. (2007). Intentional control of attention: action planning primes action-related stimulus dimensions. Psychol. Res. 71, 22–29.
Fischer, M. H., and Hoellen, N. (2004). Space-based and object-based attention depend on motor intention. J. Gen. Psychol. 131, 365–377.
Gentilucci, M., Benuzzi, F., Bertolani, L., Daprati, E., and Gangitano, M. (2000). Language and motor control. Exp. Brain Res. 133, 468–490.
Glenberg, A. M., and Kaschak, M. P. (2002). Grounding language in action. Psychon. Bull. Rev. 9, 558–565.
Glover, S., and Dixon, P. (2002). Semantics affects the planning but not the control of grasping. Exp. Brain Res. 146, 383–387.
Grill-Spector, K., and Sayres, R. (2008). Object recognition: Insights from advances in fMRI methods. Curr. Dir. Psychol. Sci. 17, 73–79.
Hannus, A., Cornelissen, F. W., Lindemann, O., and Bekkering, H. (2005). Selection-for-action in visual search. Acta Psychol. 118, 171–191.
Hommel, B., Müsseler, J., Aschersleben, G., and Prinz, W. (2001). The theory of event coding (TEC). Behav. Brain Sci. 24, 849–937.
Itti, L. (2007). “Computational cognitive neuroscience and its applications,” in Frontiers of Engineering: Reports on Leading-Edge Engineering from the 2007 Symposium (Washington, DC: The National Academies Press), 87–98.
Itti, L., and Koch, C. (2001). Computational modeling of visual attention. Nat. Rev. Neurosci. 2, 194–203.
Kastner, S., De Weerd, P., Desimone, R., and Ungerleider, L. G. (1998). Mechanisms of directed attention in the human extrastriate cortex as revealed by functional MRI. Science 282, 108–111.
Lanyon, L. J., and Denham, S. L. (2004a). A model of active visual search with object-based attention guiding scan paths. Neural Netw. 17, 873–897.
Lanyon, L. J., and Denham, S. L. (2004b). A biased competition computational model of spatial and object-based attention mediating active visual search. Neurocomputing 58–60, 655–662.
Lindemann, O., and Bekkering, H. (2009). Object manipulation and motion perception: evidence of an influence of action planning on visual processing. J. Exp. Psychol. Hum. Percept. Perform. 35, 1062–1071.
Lindemann, O., Stenneken, P., van Schie, H. T., and Bekkering, H. (2006). Semantic activation in action planning. J. Exp. Psychol. Hum. Percept. Perform. 32, 633–643.
Linnell, K. J. and Humphreys, G. W., McIntyre, D. B., Laitinen, S., and Wing, A. M. (2005). Action modulates object-based selection. Vision Res. 45, 2268–2286.
Luck, S. J., Chelazzi, L., Hillyard, S. A., and Desimone, R. (1997). Neural mechanisms of spatial selective attention in areas V1, V2, and V4 of macaque visual cortex. J. Neurophysiol. 77, 24–42.
Moore, T., and Armstrong, K. M. (2003). Selective gating of visual signals by microstimulation of frontal cortex. Nature. 421, 370–373.
Müller, H. J., Heller, D., and Ziegler, J. (1995). Visual search for singleton feature targets within and across feature dimensions. Percept. Psychophys. 57, 1–17.
Proulx, M. J., and Egeth, H. E. (2008). Biased-competition and visual search: the role of luminance and size contrast. Psychol. Res. 72, 106–113.
Ratcliff, R. (1979). Group reaction time distribution and an analysis of distribution statistics. Psychol. Bull. 86, 446–461.
Rensink, R. A. (2005). “Change blindness,” in Neurobiology of Attention, eds L. Itti, G. Rees, and J. K. Tsotsos (San Diego, CA: Elsevier), 76–81.
Rensink, R. A., O’Regan, J. K., and Clark, J. J. (1997). To see or not to see: the need for attention to perceive changes in scenes. Psychol. Sci. 8, 368–373.
Reynolds J. H., and Chelazzi, L. (2004). Attentional modulation of visual processing. Annu. Rev. Neurosci. 27, 611–647.
Reynolds, J. H., Chelazzi, L., and Desimone, R. (1999). Competitive mechanisms subserve attention in macaque areas V2 and V4. J. Neurosci. 19, 1736–1753.
Reynolds J. H., and Desimone, R. (2003). Interacting roles of attention and visual salience in V4. Neuron 37, 853–863.
Reynolds, J. H., Pasternak, T., and Desimone, R. (2000). Attention increases sensitivity of V4 neurons. Neuron 26, 703–714.
Schütz-Bosbach, S., Haggard, P., Fadiga, L., and Craighero, L. (2007). “Motor cognition: TMS studies of action generation,” in Oxford Handbook of Transcranial Stimulation, eds E. Wassermann, C. Epstein, U. Ziemann, V. Walsh, T. Paus, and S. Lisanby (Oxford, UK: Oxford University Press).
Simons, D. J., and Rensink, R. A. (2005). Change blindness: past, present, and future. Trends Cogn. Sci. 9, 16–20.
Spratling, M. W. (2008a). Reconciling predictive coding and biased competition models of cortical function. Front. Comput. Neurosci. 2, 1–8. doi: 10.3389/neuro.10.004.2008.
Spratling, M. W. (2008b). Predictive coding as a model of biased competition in visual attention. Vision Res. 48, 1391–1408.
Sun, Y., and Fisher, R. (2003). Object-based attention for computer vision. Artif. Intell. 146, 77–123.
Symes, E., Ottoboni, G., Tucker, M., Ellis, R., and Tessari, A. (2009). When motor attention improves selective attention: the dissociating role of saliency. Q. J. Exp. Psychol. doi: 10.1080/17470210903380806.
Symes, E., Tucker, M., Ellis, R., Vainio, L., and Ottoboni, G. (2008). Grasp preparation improves change-detection for congruent objects. J. Exp. Psychol. Hum. Percept. Perform. 34, 854–871.
Welsh, T., and Pratt, J. (2008). Actions modulate attention capture. Q. J. Exp. Psychol. 61, 968–976.
Wolfe, J. M. (1994). Guided search 2.0: a revised model of visual search. Psychon. Bull. Rev. 1, 202–238.
Keywords: biased competition, top-down and bottom-up interaction, action intentions, language cues, change detection
Citation: Symes E, Tucker M and Ottoboni G (2010) Integrating action and language through biased competition. Front. Neurorobot. 4:9. doi: 10.3389/fnbot.2010.00009
Received: 23 December 2009;
Paper pending published: 13 March 2010;
Accepted: 21 May 2010;
Published online:07 June 2010
Edited by:
Angelo Cangelosi, University of Plymouth, UKReviewed by:
Claudia Gianelli, Università di Bologna, ItalyCopyright: © 2010 Symes, Tucker and Ottoboni. This is an open-access article subject to an exclusive license agreement between the authors and the Frontiers Research Foundation, which permits unrestricted use, distribution, and reproduction in any medium, provided the original authors and source are credited.
*Correspondence: Ed Symes, School of Psychology, University of Plymouth, Drake Circus, Plymouth, Devon PL4 8AA, UK. e-mail:ZXN5bWVzQHBseW1vdXRoLmFjLnVr
Disclaimer: All claims expressed in this article are solely those of the authors and do not necessarily represent those of their affiliated organizations, or those of the publisher, the editors and the reviewers. Any product that may be evaluated in this article or claim that may be made by its manufacturer is not guaranteed or endorsed by the publisher.
Research integrity at Frontiers
Learn more about the work of our research integrity team to safeguard the quality of each article we publish.