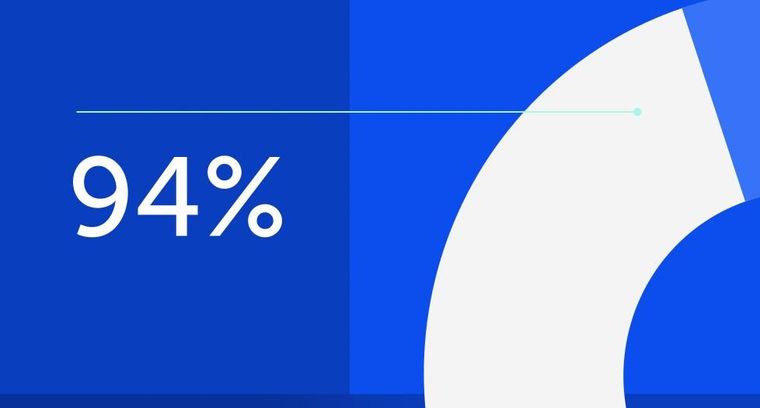
94% of researchers rate our articles as excellent or good
Learn more about the work of our research integrity team to safeguard the quality of each article we publish.
Find out more
SYSTEMATIC REVIEW article
Front. Neurol., 01 April 2025
Sec. Neurorehabilitation
Volume 16 - 2025 | https://doi.org/10.3389/fneur.2025.1544135
Background: As a major cause of disability worldwide, stroke affects about 80% of survivors with upper limb (UL) motor dysfunction, significantly impairing their quality of life. Virtual reality (VR) has been recognized as an innovative rehabilitation tool; however, the effectiveness of VR systems with different immersion modalities is still uncertain. This systematic review and network meta-analysis (NMA) aims to evaluate the comparative effectiveness of intervention measures, including non-immersive gaming consoles, immersive VR (IVR), non-immersive VR (NIVR), and conventional therapy (CT) on upper limb motor function in stroke rehabilitation.
Materials and methods: A systematic search of PubMed, Embase, Cochrane Library, and Scopus identified randomized controlled trials (RCTs) published up to 12 June 2024. UL motor recovery was assessed using the Fugl-Meyer Upper Extremity (FMUE) scale. The NMA was performed using the Bayesian approach with the BUGSnet package in R software to calculate the relative effectiveness of each intervention.
Results: 34 RCTs involving 1,704 participants were included. Among non-immersive gaming systems, Microsoft Kinect demonstrated the greatest effective in enhancing UL motor function, followed by Nintendo Wii, then NIVR and IVR head-mounted devices. CT showed the least effective. Specifically, Microsoft Kinect significantly improved FMUE scores (mean difference [MD] = 7.27, 95% confidence interval [CI]: 0.59 to 13.77, p < 0.05), followed by Nintendo Wii (MD = 4.53, 95% CI: 0.87 to 8.14, p < 0.05), and NIVR (MD = 3.57, 95% CI: 1.18 to 6.01, p < 0.05). In contrast, IVR head-mounted devices showed no statistically significant differences in outcomes, with MD of 4.16 (95% CI: −0.02 to 8.38).
Conclusion: Non-immersive gaming console of Microsoft Kinect is the most effective intervention for improving UL motor function in stroke survivors. In contrast, IVR head-mounted devices did not offer significant advantages over CT. These findings suggest that non-immersive gaming consoles of Microsoft Kinect could be a more cost-effective and accessible alternative for stroke rehabilitation.
Stroke represents a leading cause of global disability, impacting nearly 14 million individuals each year (1, 2). Although stroke-related mortality rates exhibit a downward trajectory, the prevalence of stroke survivors with long-term sequelae is increasing. This rise is primarily due to population growth and aging (3). As a result, the prevalence of persistent disabilities among adult populations is growing (4), which is increasing the demand for rehabilitation. Data from the Global Burden of Disease study indicate that over 2.4 billion people worldwide required rehabilitation services in 2019, with a significant proportion being stroke survivors with motor impairments (5). Among the various sequelae, approximately 80% of stroke survivors experience UL motor impairments. These impairments vary and often manifest as reduced hand grip strength, diminished finger dexterity, and limited elbow and shoulder mobility (6). Such deficits severely impact daily functioning, making it difficult for patients to perform tasks such as dressing, eating and maintaining personal hygiene. As a result, stroke survivors often experience a decline in autonomy and quality of life (7). Moreover, these impairments can increase dependence on others and lead to psychological distress and social isolation (8). Rehabilitation of UL motor function in stroke patients requires ongoing, dedicated efforts over an extended period. However, traditional rehabilitation approaches often encounter barriers like resource limitations and insufficient patient engagement (9), which lead to rehabilitation outcomes, emphasizing the need for innovative interventions. VR has emerged as a promising therapeutic modality to address these challenges. By providing a highly realistic three-dimensional environment, VR enables patients to engage in simulated activities. Through the integration of visual, auditory, and tactile elements, VR provides real-time feedback to patients (10, 11). Additionally, VR may also facilitate functional recovery by activating mechanisms of neuroplasticity (12).
In the field of post-stroke UL functional recovery, VR technologies encompass a variety of applications. These primarily include IVR, such as head-mounted devices, NIVR, and non-immersive gaming consoles (e.g., Nintendo Wii and Microsoft Kinect) (13, 14). While IVR provides high immersion levels, which may enhance motor recovery (15, 16), its widespread adoption is limited by challenges such as high costs and patient discomfort (17). NIVR, in contrast, is more accessible but has shown variability in its effectiveness due to differences in technological features and interactivity (18, 19).
Many existing studies focus on individual VR modalities, leaving a gap in direct comparisons of different VR technologies for UL rehabilitation (20, 21). A 2022 NMA (22) suggested the potential benefits of IVR for UL motor recovery. However, it did not incorporate recent advances in VR technologies or newly published RCTs. Moreover, the study did not consider the practical limitations of IVR, which have prompted a growing interest in NIVR systems for scalable stroke rehabilitation. Based on these considerations, we hypothesize that non-immersive gaming consoles, such as Microsoft Kinect, may offer a superior effect due to their accessibility, affordability, and ease of integration into clinical and home-based rehabilitation. Therefore, we will test this through a systematic review and NMA to provide a comprehensive comparison of VR interventions with varying levels of immersion. By doing so, it offers updated evidence to guide clinical decision-making and enhance rehabilitation strategies for stroke survivors.
This systematic review and NMA was conducted in accordance with the guidelines provided by the Preferred Reporting Items for Systematic Reviews and Meta-Analyses statement (PRISMA-NMA) (23). The study was registered with PROSPERO (CRD42024610482), which can be found at https://www.crd.york.ac.uk/PROSPERO/view/CRD42024610482.
A systematic search was performed across PubMed, Embase, the Cochrane Library, and Scopus from their inception up to 12 June 2024. The search strategies were tailored for each database, combining controlled vocabulary terms and title/abstract keywords related to virtual reality, upper limb, stroke, and randomized controlled trials. The comprehensive search strategies are detailed in Supplementary Table S1. The literature review was restricted to peer-reviewed journal articles reported human studies in English.
Studies were eligible for our NMA if they met all following criteria:
1. Study design: RCTs.
2. Patient characteristics: adult individuals experiencing their initial ischemic or hemorrhagic stroke, with diagnosis established according to well-defined or internationally recognized diagnostic criteria, and with no restrictions on race or gender.
3. Intervention: VR-based rehabilitation, encompassing IVR (such as head-mounted devices), NIVR, and non-immersive gaming consoles (such as Microsoft Kinect or Nintendo Wii).
4. Comparison: CT, including conventional rehabilitation, occupational therapy, physical therapy, usual care alone, and UL conventional. The frequency and duration should correspond to those of the intervention group.
5. Outcome: FMUE was used to assess upper extremity function.
Studies were excluded based on the following criteria:
1. VR was integrated with robot-assisted therapy or neuromodulation in the intervention.
2. The total treatment dosages administered to the VR group and the CT group were not equivalent.
3. Studies with an intervention frequency lower than twice weekly and lacking clear specification of individual intervention durations.
4. Studies with unclear outcome indicators that could not be accurately extracted, or those with missing data.
5. Articles with only abstracts, reviews, case reports and study protocols.
6. In instances of duplicate published studies, the research presenting the most comprehensive data was chosen for inclusion.
Two independent reviewers evaluated studies for inclusion through a two-step process: initially screening titles and abstracts, followed by a comprehensive full-text assessment based on predetermined inclusion and exclusion criteria to determine final eligibility. Any disagreements were resolved through consultation with a third reviewer or by referencing to the original study. Data extraction was conducted using a standardized form to collect essential information, including the first author, publication year, study location, intervention and comparison group content and dosage, sample size, age, male proportion, and assessment time points for evaluating VR effects. Furthermore, elements critical for risk of bias assessment were recorded. In studies featuring multiple groups, only the CT group with a matched treatment dosage matching the VR group was included for comparison. For studies presenting both Per-protocol and Intention-to-Treat (ITT) analyses, ITT data were prioritized for extraction (24).
Version 2 of the Cochrane risk-of-bias tool for randomized trials (RoB-2) was employed to evaluate the quality of included studies (25). Two independent reviewers assessed six domains: bias in the randomization process, bias due to deviations from intended interventions, bias from missing outcome data, bias in outcome measurement, bias in selection of reported results, and overall bias. The studies were categorized as low risk of bias (all domains low risk), some concerns (concerns in at least one domain but no high risk), or high risk of bias (high risk in at least one domain). Discrepancies between reviewers were resolved through discussion to reach a consensus.
This study employed a Bayesian NMA to evaluate continuous outcome measures associated with UL function recovery in stroke patients. All statistical analyses were conducted using R (version 4.4.1; R Foundation for Statistical Computing, Vienna, Austria) with the “BUGSnet” package (26). We employed Bayesian NMA to model the posterior probability distribution, providing insights into the relative effects of treatments, quantifying uncertainty around parameter estimates and the ranking of the treatments within the network. The analysis employed a Markov Chain Monte Carlo (MCMC) with Gibbs sampling for robust parameter estimation (27), utilizing a Bayesian framework with 1,000 adaptations, 10,000 burn-ins, and 50,000 iterations. Continuous outcome data were presented as mean differences (MD) with corresponding 95% credible intervals; p < 0.05 suggests a statistically significant difference in outcome measures (28). Transitivity in this NMA was addressed by including studies with comparable patient populations, intervention designs, and outcome measures. Model selection and goodness-of-fit were evaluated through deviance information criteria (DIC), with lower values indicating superior model fit (29). Model adequacy was further evaluated by comparing the residual deviance with the number of unconstrained data points, where a close alignment suggested an adequate fit (30). Convergence was assessed using the Gelman–Rubin–Brooks diagnostic, with a potential scale reduction factor (PSRF) value below 1.05 considered indicative of acceptable convergence (31).
Furthermore, surface under the cumulative ranking (SUCRA) values were employed to rank the various interventions, with higher SUCRA values signifying more effective interventions (32). SUCRA values approach one when a treatment consistently ranks first, and approach zero when it consistently ranks last (33). Following this analysis, we developed the league table and heat plot, providing comprehensive information on the relative effectiveness and associated uncertainty for all possible intervention pairs.
The search strategy initially identified 4,631 studies from the databases. After eliminating duplicates, 4,049 studies remained for title and abstract screening. Subsequently, 387 articles underwent full-text assessment based on predefined inclusion and exclusion criteria. Ultimately, 34 RCTs met the eligibility criteria and were included in this systematic review and NMA (34–67). The PRISMA flow diagram is depicted in Figure 1.
Figure 1. PRISMA flow diagram illustrating the process for searching and selecting eligible studies included in the network meta-analysis.
The current analysis encompasses 34 RCTs were included, all of which were two-arm studies, involving a combined total of 1,704 participants. Table 1 provides a summary of the characteristics of these studies. Across included studies, the frequency, duration, and total dose of interventions were comparable between VR and CT groups. The intervention duration ranged from 2 to 16 weeks, with the total VR intervention dose spanning 7 to 56 h. All studies utilized the Fugl-Meyer assessment to evaluate UL function. Each study employed two measurement time points: baseline and post-intervention. Follow-up evaluations were conducted in some studies to assess long-term effects. However, the follow-up time varied, with the longest being 12 weeks post-intervention (45, 55) and the shortest being 3 weeks post-intervention (41). One study (40) conducted two follow-ups: at 4 weeks after the intervention and at 12 weeks post-intervention.
The risk-of-bias assessment in the RCTs (Figure 2) demonstrated a low risk of bias across the analyzed trials.
The network diagram is displayed in Figure 3. Six studies (39, 41–44, 55) compared head-mounted devices to CT, while 16 studies compared NIVR systems to CT (38, 49–53, 56, 59–67). A total of 12 studies utilized non-immersive gaming consoles; four (34, 37, 45, 46) compared Microsoft Kinect to CT, and eight (35, 36, 40, 47, 48, 54, 57, 58) compared Nintendo Wii to CT. Among these interventions, CT had the largest sample size (759 participants), followed by NIVR (613 participants), Nintendo Wii (151 participants), head-mounted devices (114 participants), and Microsoft Kinect (67 participants).
Figure 3. Network of randomized controlled trials (RCTs) comparing different virtual reality modalities for upper limb recovery after stroke. The size of each circle represents the number of participants in the trial, while the thickness of the connecting lines corresponds to the number of participants directly comparing the two treatments. The numbers indicate the number of trials contributing to each treatment comparison. CT, Conventional therapy.
In terms of model fit, both fixed-effects and random-effects models were examined. As illustrated in Supplementary Figure S1, the random-effects model demonstrated a superior fit, with lower DIC values and visually minimized leverage outliers, showed a better fit for the data than the fixed-effects model. Consequently, the random-effects model was selected for the final analysis. A Gelman–Rubin–Brooks plot was utilized to evaluate the convergence, which revealed that the simulations were valid, as the PSRF was <1.05 (Supplementary Figure S2, Gelman convergence plot).
Secondly, as consistency is a key assumption in NMA, we evaluated the model’s inconsistency by fitting a random-effects inconsistency model and comparing it with our random-effects consistency model. Supplementary Figure S3 illustrates the consistency and inconsistency models for FMUE. The consistency model demonstrated a marginally lower DIC value, suggesting a superior fit.
A treatment rank probability analysis was conducted to compare the posterior probabilities of each treatment, determining their relative rankings for FMUE outcomes. Additionally, we generated SUCRA plots to visually illustrate the percentage probability of rankings. For FMUE, examining the SUCRA plot (Figure 4A) and treatment rank plot (Figure 4B), reveal that non-immersive gaming consoles of Microsoft Kinect (SUCRA value 0.847) was associated with the highest probability of improving UL function in stroke patients, thereby ranking as the most effective treatment. This was followed by non-immersive gaming console of Nintendo Wii (SUCRA value 0.614), Immersive VR: head-mounted device (SUCRA value 0.557), and Non-immersive VR (SUCRA value 0.469). CT was identified as the least effective treatment, indicating minimal impact on improving FMUE.
Figure 4. Results of the network meta-analysis of different modalities for upper limb recovery after stroke. (A) SUCRA plot for FMUE. (B) Rankogram plot of interventions for FMUE. SUCRA, surface under the cumulative ranking, represents the probability of ranking for each treatment, as shown in the graphs; FMUE, The Fugl-Meyer Upper Extremity; CT, Conventional therapy.
A league plot was generated to provide a comprehensive summary of the NMA results, comparing the effectiveness of various interventions in improving the FMUE score. The symbols (**) indicate statistically significant differences between treatments and comparators at a 95% confidence level, with p < 0.05. As shown in Figure 5, non-immersive gaming consoles, including the Microsoft Kinect and Nintendo Wii, significantly improved UL function compared to CT. Specifically, the non-immersive gaming consoles of Microsoft Kinect yielded MD of 7.27 (95% CI: 0.59 to 13.77), indicating a significant benefit (p < 0.05) over CT. While the non-immersive gaming console Nintendo Wii showed a significant improvement (p < 0.05) with MD of 4.53 (95% CI: 0.87 to 8.14). In addition, NIVR was also found to have a moderate benefit, with an MD of 3.57 (95% CI: 1.18 to 6.01) and p < 0.05. In contrast, IVR head-mounted devices showed no statistically significant differences in outcomes, with MD of 4.16 (95% CI: −0.02 to 8.38).
Figure 5. League table for all treatment in the network for FMUE. The symbol ** in the figure indicates significant differences between the treatments (p < 0.05). FMUE, The Fugl-Meyer Upper Extremity; CT, Conventional therapy.
To obtain the visualization of the MD differences with a 95% CI between interventions, forest plots were performed for FMUE (Figure 6). Both plots show approximately the same results, indicating that non-immersive gaming consoles of Microsoft Kinect interventions significantly improved FMUE, followed by the non-immersive gaming console Nintendo Wii intervention, and then NIVR.
Figure 6. Forest plot for all treatments compared to conventional therapy as reference for FMUE. FMUE, The Fugl-Meyer Upper Extremity; CT, Conventional therapy.
This systematic review and NMA examined 34 RCTs encompassing 1,704 stroke survivors to assess VR interventions with varying immersion levels on UL motor function using the FMUE scale. The analysis indicates that non-immersive gaming consoles of Microsoft Kinect, demonstrated the most substantial improvement in UL function, followed by the Nintendo Wii and other NIVR systems. Conversely, IVR systems did not demonstrate significant superiority over CT.
The findings are supported by a rigorous methodological approach. All included studies ensured comparable intervention frequency and duration between VR and CT groups, minimizing potential confounding factors. This analysis included only high-quality RCTs, with risk-of-bias assessments confirming low risk across all domains. The FMUE scale, consistently used as the primary outcome measure, provided a reliable, stroke-specific evaluation of UL recovery (68). Additionally, the Bayesian NMA facilitated the simultaneous comparison and ranking of multiple interventions, enhancing the robustness of the conclusions.
Our findings demonstrate the significant benefits of non-immersive gaming consoles of Microsoft Kinect in UL rehabilitation. Microsoft Kinect’s precise motion-sensing technology enhances interactivity, fostering greater patient engagement and motivation—crucial factors in motor relearning (69, 70). Moreover, its personalized training content and accessibility render it suitable for both clinical and home-based applications (71).
While the Nintendo Wii also provides interactivity, its limited motion capture accuracy and less advanced game design reduce its effectiveness in stroke rehabilitation (72, 73). Additionally, Microsoft Kinect has been shown to improve activities of daily living and cognitive engagement, further corroborating its therapeutic potential (74). Conversely, IVR systems, despite their immersive experiences, encounter substantial obstacles to widespread implementation due to hardware complexity, high costs, and patient discomfort, including motion sickness (75). These factors may impede training duration and adherence among elderly or physically frail patients (76).
Variations in UL rehabilitation outcomes may be attributed to differences in motion capture technology and task design. Microsoft Kinect (34, 37, 45, 46) systems employ camera-based motion tracking, enabling natural UL movements, while the Wii relies on handheld controllers, which potentially restrict distal upper extremity engagement (77).
Our findings are consistent with those of Soleimani et al. (78), who similarly demonstrated the significantly improve in UL motor recovery post-stroke through VR interventions. However, Soleimani’s meta-analysis was constrained by pairwise comparisons, limiting the evaluation of different VR modalities. In contrast, our study employed the NMA framework, enabling for direct comparisons across multiple VR modalities and offering a more comprehensive ranking of interventions.
To the best of our knowledge, only one other NMA has assessed the efficacy of various VR interventions for UL motor function, involving 20 RCTs with 813 participants (22). However, our analysis identified non-immersive gaming consoles of Microsoft Kinect, as the most effective, while the previous NMA favored immersive VR. These differences may be attributed to advancements in NIVR technology and our analysis of a larger, more recent dataset.
Several limitations should be considered when interpreting our findings. Firstly, the inability to differentiate between acute, subacute, and chronic stroke phases constrains the applicability of our results to specific rehabilitation stages. Secondly, the lack of long-term data limits our understanding of the sustainability of VR interventions beyond the post-intervention period. Thirdly, the absence of direct head-to-head comparisons among different VR systems restricts the robustness and comprehensiveness of the conclusions, as indirect comparisons may introduce additional uncertainties. However, we included studies with highly similar designs and population characteristics to ensure the validity and consistency of the indirect comparisons. Additionally, we employed a consistency model to minimize potential biases arising from the absence of direct comparisons. In light of these limitations, the findings of our network analysis should be interpreted with caution. We recommend that subsequent studies validate our findings through direct comparisons.
This study underscores the cost-effectiveness and accessibility of non-immersive gaming consoles of Microsoft Kinect interventions for stroke rehabilitation. These platforms offer an interactive environment that promotes patient engagement and adherence—key factors for sustained recovery. Future research should focus on assessing long-term outcomes and conducting comprehensive cost-effectiveness analyses. In future research, more refined classification methods should be employed, or studies should focus on individual stages of stroke recovery to enhance the applicability of the results to specific rehabilitation phases. Additionally, future studies should prioritize the evaluation of long-term outcomes and conduct comprehensive cost-effectiveness analyses to gain a better understanding of the sustainability of VR interventions during the post-intervention period.
This NMA highlights the significant benefits of non-immersive gaming consoles of Microsoft Kinect, in enhancing UL motor function among stroke survivors. The findings support the integration of non-immersive gaming consoles of Microsoft Kinect interventions into clinical practice. However, our analysis also reveals that CT provides the least improvement in upper limb motor function, underscoring the need for more engaging and interactive rehabilitation tools. This comparison between the most effective (Microsoft Kinect) and least effective interventions clearly demonstrates the potential of gaming consoles in stroke rehabilitation.
The original contributions presented in the study are included in the article/Supplementary material, further inquiries can be directed to the corresponding authors.
JZ: Conceptualization, Data curation, Formal analysis, Visualization, Writing – original draft. ML: Conceptualization, Data curation, Formal analysis, Writing – original draft. JunY: Formal analysis, Resources, Software, Writing – original draft. JinY: Data curation, Software, Writing – original draft. YX: Data curation, Investigation, Writing – original draft. JieY: Methodology, Software, Validation, Visualization, Writing – original draft. EC: Funding acquisition, Supervision, Writing – review & editing.
The author(s) declare that financial support was received for the research and/or publication of this article. The study was supported by a grant from “Research on the Integrated Nursing Intervention Model of Traditional Chinese and Western Medicine for Early Recovery Patients with Ischemic Stroke Based on KTA” (No. 202101AZ070001-221) and “Evidence-based Nursing Practice for Motor Dysfunction in Patients with Qi Deficiency and Blood Stasis Type of Ischemic Stroke” (No. 202201AH070163) in Yunnan Province, China. No funding bodies had any role in study design, data collection and analysis, decision to publish, or preparation of the manuscript.
The authors declare that the research was conducted in the absence of any commercial or financial relationships that could be construed as a potential conflict of interest.
The author(s) declare that no Gen AI was used in the creation of this manuscript.
All claims expressed in this article are solely those of the authors and do not necessarily represent those of their affiliated organizations, or those of the publisher, the editors and the reviewers. Any product that may be evaluated in this article, or claim that may be made by its manufacturer, is not guaranteed or endorsed by the publisher.
The Supplementary material for this article can be found online at: https://www.frontiersin.org/articles/10.3389/fneur.2025.1544135/full#supplementary-material
SUPPLEMENTARY FIGURE S1 | Leverage plots and DIC for fixed and random effects models for FMUE.
SUPPLEMENTARY FIGURE S2 | Gelman convergence plot.
SUPPLEMENTARY FIGURE S3 | Leverage plots and DIC for consistency and inconsistency model FMUE.
1. Feigin, VL, Norrving, B, and Mensah, GA. Global burden of stroke. Circ Res. (2017) 120:439–48. doi: 10.1161/CIRCRESAHA.116.308413
2. Saini, V, Guada, L, and Yavagal, DR. Global epidemiology of stroke and access to acute ischemic stroke interventions. Neurology. (2021) 97:S6–S16. doi: 10.1212/WNL.0000000000012781
3. Wu, S, Wu, B, Liu, M, Chen, Z, Wang, W, Anderson, CS, et al. Stroke in China: advances and challenges in epidemiology, prevention, and management. Lancet Neurol. (2019) 18:394–405. doi: 10.1016/S1474-4422(18)30500-3
4. Collaborators, GBDSCI . Global, regional, and national burden of spinal cord injury, 1990-2019: a systematic analysis for the global burden of disease study 2019. Lancet Neurol. (2023) 22:1026–47. doi: 10.1016/S1474-4422(23)00287-9
5. Cieza, A, Causey, K, Kamenov, K, Hanson, SW, Chatterji, S, and Vos, T. Global estimates of the need for rehabilitation based on the global burden of disease study 2019: a systematic analysis for the global burden of disease study 2019. Lancet. (2021) 396:2006–17. doi: 10.1016/S0140-6736(20)32340-0
6. Hijikata, N, Kawakami, M, Ishii, R, Tsuzuki, K, Nakamura, T, Okuyama, K, et al. Item difficulty of Fugl-Meyer assessment for upper extremity in persons with chronic stroke with moderate-to-severe upper limb impairment. Front Neurol. (2020) 11:577855. doi: 10.3389/fneur.2020.577855
7. Virani, SS, Alonso, A, Aparicio, HJ, Benjamin, EJ, Bittencourt, MS, Callaway, CW, et al. Heart disease and stroke Statistics-2021 update: a report from the American Heart Association. Circulation. (2021) 143:e254–743. doi: 10.1161/CIR.0000000000000950
8. Wei, D, Hua, XY, Zheng, MX, Wu, JJ, and Xu, JG. Effectiveness of robot-assisted virtual reality mirror therapy for upper limb motor dysfunction after stroke: study protocol for a single-center randomized controlled clinical trial. BMC Neurol. (2022) 22:307. doi: 10.1186/s12883-022-02836-6
9. McCabe, JP, Henniger, D, Perkins, J, Skelly, M, Tatsuoka, C, and Pundik, S. Feasibility and clinical experience of implementing a myoelectric upper limb orthosis in the rehabilitation of chronic stroke patients: a clinical case series report. PLoS One. (2019) 14:e0215311. doi: 10.1371/journal.pone.0215311
10. Coscia, M, Wessel, MJ, Chaudary, U, Millan, JDR, Micera, S, Guggisberg, A, et al. Neurotechnology-aided interventions for upper limb motor rehabilitation in severe chronic stroke. Brain. (2019) 142:2182–97. doi: 10.1093/brain/awz181
11. Saposnik, G, and Levin, M. Virtual reality in stroke rehabilitation: a meta-analysis and implications for clinicians. Stroke. (2011) 42:1380–6. doi: 10.1161/STROKEAHA.110.605451
12. Gao, Y, Ma, L, Lin, C, Zhu, S, Yao, L, Fan, H, et al. Effects of virtual reality-based intervention on cognition, motor function, mood, and activities of daily living in patients with chronic stroke: a systematic review and Meta-analysis of randomized controlled trials. Front Aging Neurosci. (2021) 13:766525. doi: 10.3389/fnagi.2021.766525
13. Sevcenko, K, and Lindgren, I. The effects of virtual reality training in stroke and Parkinson's disease rehabilitation: a systematic review and a perspective on usability. Eur Rev Aging Phys Act. (2022) 19:4. doi: 10.1186/s11556-022-00283-3
14. Fernandez Montenegro, JM, Villarini, B, Angelopoulou, A, Kapetanios, E, Garcia-Rodriguez, J, and Argyriou, V. A survey of Alzheimer's disease early diagnosis methods for cognitive assessment. Sensors. (2020) 20:7292. doi: 10.3390/s20247292
15. Laver, KE, Lange, B, George, S, Deutsch, JE, Saposnik, G, and Crotty, M. Virtual reality for stroke rehabilitation. Cochrane Database Syst Rev. (2017) 11:CD008349. doi: 10.1002/14651858.CD008349.pub4
16. Matys-Popielska, K, Popielski, K, and Sibilska-Mroziewicz, A. Study of the possibility of using virtual reality application in rehabilitation among elderly post-stroke patients. Sensors. (2024) 24:745. doi: 10.3390/s24092745
17. Aprile, I, Guardati, G, Cipollini, V, Papadopoulou, D, Mastrorosa, A, Castelli, L, et al. Robotic rehabilitation: an opportunity to improve cognitive functions in subjects with stroke. An Explorative Study. Front Neurol. (2020) 11:588285. doi: 10.3389/fneur.2020.588285
18. Domínguez-Téllez, P, Moral-Muñoz, JA, Salazar, A, Casado-Fernández, E, and Lucena-Antón, D. Game-based virtual reality interventions to improve upper limb motor function and quality of life after stroke: systematic review and Meta-analysis. Games Health J. (2020) 9:1–10. doi: 10.1089/g4h.2019.0043
19. Hernandez, A, Bubyr, L, Archambault, PS, Higgins, J, Levin, MF, and Kairy, D. Virtual reality-based rehabilitation as a feasible and engaging tool for the Management of Chronic Poststroke Upper-Extremity Function Recovery: randomized controlled trial. JMIR Serious Games. (2022) 10:e37506. doi: 10.2196/37506
20. Lo, HHM, Zhu, M, Zou, Z, Wong, CL, Lo, SHS, Chung, VC, et al. Immersive and nonimmersive virtual reality-assisted active training in chronic musculoskeletal pain: systematic review and Meta-analysis. J Med Internet Res. (2024) 26:e48787. doi: 10.2196/48787
21. De Miguel-Rubio, A, Rubio, MD, Alba-Rueda, A, Salazar, A, Moral-Munoz, JA, and Lucena-Anton, D. Virtual reality Systems for Upper Limb Motor Function Recovery in patients with spinal cord injury: systematic review and Meta-analysis. JMIR Mhealth Uhealth. (2020) 8:e22537. doi: 10.2196/22537
22. Hao, J, He, Z, Yu, X, and Remis, A. Comparison of immersive and non-immersive virtual reality for upper extremity functional recovery in patients with stroke: a systematic review and network meta-analysis. Neurol Sci. (2023) 44:2679–97. doi: 10.1007/s10072-023-06742-8
23. Hutton, B, Salanti, G, Caldwell, DM, Chaimani, A, Schmid, CH, Cameron, C, et al. The PRISMA extension statement for reporting of systematic reviews incorporating network meta-analyses of health care interventions: checklist and explanations. Ann Intern Med. (2015) 162:777–84. doi: 10.7326/M14-2385
24. Porta, N, Bonet, C, and Cobo, E. Discordance between reported intention-to-treat and per protocol analyses. J Clin Epidemiol. (2007) 60:663–9. doi: 10.1016/j.jclinepi.2006.09.013
25. Sterne, JAC, Savovic, J, Page, MJ, Elbers, RG, Blencowe, NS, Boutron, I, et al. RoB 2: a revised tool for assessing risk of bias in randomised trials. BMJ. (2019) 366:l4898. doi: 10.1136/bmj.l4898
26. Beliveau, A, Boyne, DJ, Slater, J, Brenner, D, and Arora, P. BUGSnet: an R package to facilitate the conduct and reporting of Bayesian network Meta-analyses. BMC Med Res Methodol. (2019) 19:196. doi: 10.1186/s12874-019-0829-2
27. Bhattacharya, R, Lin, L, and Patrangenaru, V. Markov chain Monte Carlo (MCMC) simulation and Bayes theory. New York: Springer (2016).
28. Burschinski, A, Schneider-Thoma, J, Chiocchia, V, Schestag, K, Wang, D, Siafis, S, et al. Metabolic side effects in persons with schizophrenia during mid- to long-term treatment with antipsychotics: a network meta-analysis of randomized controlled trials. World Psychiatry. (2023) 22:116–28. doi: 10.1002/wps.21036
29. Hah, JM, Cramer, E, Hilmoe, H, Schmidt, P, McCue, R, Trafton, J, et al. Factors associated with acute pain estimation, postoperative pain resolution, opioid cessation, and recovery: secondary analysis of a randomized clinical trial. JAMA Netw Open. (2019) 2:e190168. doi: 10.1001/jamanetworkopen.2019.0168
30. Dharmaratne, M, Kulkarni, AS, Taherian Fard, A, and Mar, JC. scShapes: a statistical framework for identifying distribution shapes in single-cell RNA-sequencing data. GigaScience. (2022) 12:126. doi: 10.1093/gigascience/giac126
31. Aoyama, H, Uchida, K, Aoyama, K, Pechlivanoglou, P, Englesakis, M, Yamada, Y, et al. Assessment of therapeutic interventions and lung protective ventilation in patients with moderate to severe acute respiratory distress syndrome: a systematic review and network Meta-analysis. JAMA Netw Open. (2019) 2:e198116. doi: 10.1001/jamanetworkopen.2019.8116
32. Mitra, S, Florez, ID, Tamayo, ME, Mbuagbaw, L, Vanniyasingam, T, Veroniki, AA, et al. Association of Placebo, indomethacin, ibuprofen, and acetaminophen with closure of hemodynamically significant patent ductus arteriosus in preterm infants: a systematic review and Meta-analysis. JAMA. (2018) 319:1221–38. doi: 10.1001/jama.2018.1896
33. Salanti, G, Kavvoura, FK, and Ioannidis, JP. Exploring the geometry of treatment networks. Ann Intern Med. (2008) 148:544–53. doi: 10.7326/0003-4819-148-7-200804010-00011
34. Ain, QU, Khan, S, Ilyas, S, Yaseen, A, Tariq, I, Liu, T, et al. Additional effects of Xbox Kinect training on upper limb function in chronic stroke patients: A randomized control trial. Healthcare. (2021) 9:242. doi: 10.3390/healthcare9030242
35. Choi, JH, Han, EY, Kim, BR, Kim, SM, Im, SH, Lee, SY, et al. Effectiveness of commercial gaming-based virtual reality movement therapy on functional recovery of upper extremity in subacute stroke patients. Ann Rehabil Med. (2014) 38:485–93. doi: 10.5535/arm.2014.38.4.485
36. da Silva Ribeiro, NM, Ferraz, DD, Pedreira, E, Pinheiro, I, da Silva Pinto, AC, Neto, MG, et al. Virtual rehabilitation via Nintendo Wii(R) and conventional physical therapy effectively treat post-stroke hemiparetic patients. Top Stroke Rehabil. (2015) 22:299–305. doi: 10.1179/1074935714Z.0000000017
37. Kim, WS, Cho, S, Park, SH, Lee, JY, Kwon, S, and Paik, NJ. A low cost kinect-based virtual rehabilitation system for inpatient rehabilitation of the upper limb in patients with subacute stroke: a randomized, double-blind, sham-controlled pilot trial. Medicine. (2018) 97:e11173. doi: 10.1097/MD.0000000000011173
38. Kottink, AI, Prange, GB, Krabben, T, Rietman, JS, and Buurke, JH. Gaming and conventional exercises for improvement of arm function after stroke: a randomized controlled pilot study. Games Health J. (2014) 3:184–91. doi: 10.1089/g4h.2014.0026
39. Mekbib, DB, Debeli, DK, Zhang, L, Fang, S, Shao, Y, Yang, W, et al. A novel fully immersive virtual reality environment for upper extremity rehabilitation in patients with stroke. Ann N Y Acad Sci. (2021) 1493:75–89. doi: 10.1111/nyas.14554
40. Kong, KH, Loh, YJ, Thia, E, Chai, A, Ng, CY, Soh, YM, et al. Efficacy of a virtual reality commercial gaming device in upper limb recovery after stroke: a randomized Controlled Study. Top Stroke Rehabil. (2016) 23:333–40. doi: 10.1080/10749357.2016.1139796
41. Hsu, HY, Kuo, LC, Lin, YC, Su, FC, Yang, TH, and Lin, CW. Effects of a virtual reality-based Mirror therapy program on improving sensorimotor function of hands in chronic stroke patients: a randomized controlled trial. Neurorehabil Neural Repair. (2022) 36:335–45. doi: 10.1177/15459683221081430
42. Henrique, PPB, Colussi, EL, and De Marchi, ACB. Effects of Exergame on Patients' balance and upper limb motor function after stroke: a randomized controlled trial. J Stroke Cerebrovasc Dis. (2019) 28:2351–7. doi: 10.1016/j.jstrokecerebrovasdis.2019.05.031
43. Huang, CY, Chiang, WC, Yeh, YC, Fan, SC, Yang, WH, Kuo, HC, et al. Effects of virtual reality-based motor control training on inflammation, oxidative stress, neuroplasticity and upper limb motor function in patients with chronic stroke: a randomized controlled trial. BMC Neurol. (2022) 22:21. doi: 10.1186/s12883-021-02547-4
44. Ogun, MN, Kurul, R, Yasar, MF, Turkoglu, SA, Avci, S, and Yildiz, N. Effect of leap motion-based 3D immersive virtual reality usage on upper extremity function in ischemic stroke patients. Arq Neuropsiquiatr. (2019) 77:681–8. doi: 10.1590/0004-282x20190129
45. Hung, JW, Chou, CX, Chang, YJ, Wu, CY, Chang, KC, Wu, WC, et al. Comparison of Kinect2Scratch game-based training and therapist-based training for the improvement of upper extremity functions of patients with chronic stroke: a randomized controlled single-blinded trial. Eur J Phys Rehabil Med. (2019) 55:542–50. doi: 10.23736/S1973-9087.19.05598-9
46. Lee, M, Son, J, Kim, J, Pyun, S-B, Eun, S-D, and Yoon, B. Comparison of individualized virtual reality- and group-based rehabilitation in older adults with chronic stroke in community settings: a pilot randomized controlled trial. Eur J Integr Med. (2016) 8:738–46. doi: 10.1016/j.eujim.2016.08.166
47. Junior, V, Santos, MS, Ribeiro, N, and Maldonado, IL. Combining proprioceptive neuromuscular facilitation and virtual reality for improving sensorimotor function in stroke survivors: a randomized clinical trial. J Central Nerv Syst Dis. (2019) 11:1179573519863826. doi: 10.1177/1179573519863826
48. Teremetz, M, Garcia Alvarez, A, Hanneton, S, Roby-Brami, A, Roche, N, Bensmail, D, et al. Improving upper-limb and trunk kinematics by interactive gaming in individuals with chronic stroke: a single-blinded RCT. Ann Phys Rehabil Med. (2022) 65:101622. doi: 10.1016/j.rehab.2021.101622
49. Kiper, P, Szczudlik, A, Agostini, M, Opara, J, Nowobilski, R, Ventura, L, et al. Virtual reality for upper limb rehabilitation in subacute and chronic stroke: a randomized controlled trial. Arch Phys Med Rehabil. (2018) 99:834–842.e4. doi: 10.1016/j.apmr.2018.01.023
50. Oh, YB, Kim, GW, Han, KS, Won, YH, Park, SH, Seo, JH, et al. Efficacy of virtual reality combined with real instrument training for patients with stroke: a randomized controlled trial. Arch Phys Med Rehabil. (2019) 100:1400–8. doi: 10.1016/j.apmr.2019.03.013
51. Piron, L, Turolla, A, Agostini, M, Zucconi, CS, Ventura, L, Tonin, P, et al. Motor learning principles for rehabilitation: a pilot randomized controlled study in poststroke patients. Neurorehabil Neural Repair. (2010) 24:501–8. doi: 10.1177/1545968310362672
52. Shin, JH, Kim, MY, Lee, JY, Jeon, YJ, Kim, S, Lee, S, et al. Effects of virtual reality-based rehabilitation on distal upper extremity function and health-related quality of life: a single-blinded, randomized controlled trial. J Neuroeng Rehabil. (2016) 13:17. doi: 10.1186/s12984-016-0125-x
53. Shin, S, Lee, HJ, Chang, WH, Ko, SH, Shin, YI, and Kim, YH. A smart glove digital system promotes restoration of upper limb motor function and enhances cortical hemodynamic changes in subacute stroke patients with mild to moderate weakness: a randomized controlled trial. J Clin Med. (2022) 11:343. doi: 10.3390/jcm11247343
54. Anwar, N, Karimi, H, Ahmad, A, Gilani, SA, Khalid, K, Aslam, AS, et al. Virtual reality training using Nintendo Wii games for patients with stroke: randomized controlled trial. JMIR Serious Games. (2022) 10:e29830. doi: 10.2196/29830
55. Huang, Q, Jiang, X, Jin, Y, Wu, B, Vigotsky, AD, Fan, L, et al. Immersive virtual reality-based rehabilitation for subacute stroke: a randomized controlled trial. J Neurol. (2024) 271:1256–66. doi: 10.1007/s00415-023-12060-y
56. Shin, JH, Bog Park, S, and Ho, JS. Effects of game-based virtual reality on health-related quality of life in chronic stroke patients: a randomized, controlled study. Comput Biol Med. (2015) 63:92–8. doi: 10.1016/j.compbiomed.2015.03.011
57. Alves, SS, Ocamoto, GN, de Camargo, PS, Santos, ATS, and Terra, A. Effects of virtual reality and motor imagery techniques using Fugl Meyer assessment scale in post-stroke patients. Int J Ther Rehabil. (2018) 25:587–96. doi: 10.12968/ijtr.2018.25.11.587
58. Assadi, SH, Lazar, IZ, and Rand, D. Video-games for treating the weaker upper extremity of individuals with subacute stroke: a pilot randomized Controlled Trail. Israel J Occup Therapy. (2016) 25:E24.
59. In, TS, Jung, KS, Lee, WL, and Song, CH. Virtual reality reflection therapy improves motor recovery and motor function in the upper extremities of people with chronic stroke. J Phys Ther Sci. (2012) 24:339–43. doi: 10.1589/jpts.24.339
60. Johnson, L, Bird, ML, Muthalib, M, and Teo, WP. An innovative STRoke interactive virtual thErapy (STRIVE) online platform for community-dwelling stroke survivors: a randomized controlled trial. Arch Phys Med Rehabil. (2020) 101:1131–7. doi: 10.1016/j.apmr.2020.03.011
61. Kiper, P, Agostini, M, Luque-Moreno, C, Tonin, P, and Turolla, A. Reinforced feedback in virtual environment for rehabilitation of upper extremity dysfunction after stroke: preliminary data from a randomized controlled trial. Biomed Res Int. (2014) 2014:752128:1–8. doi: 10.1155/2014/752128
62. Lam, SSL, Liu, TW, Ng, SSM, Lai, CWK, and Woo, J. Bilateral movement-based computer games improve sensorimotor functions in subacute stroke survivors. J Rehabil Med. (2022) 54:jrm00307. doi: 10.2340/jrm.v54.913
63. Levin, MF, Snir, O, Liebermann, DG, Weingarden, H, and Weiss, PL. Virtual reality versus conventional treatment of reaching ability in chronic stroke: clinical feasibility study. Neurol Therapy. (2012) 1:3. doi: 10.1007/s40120-012-0003-9
64. Park, M, Ko, MH, Oh, SW, Lee, JY, Ham, Y, Yi, H, et al. Effects of virtual reality-based planar motion exercises on upper extremity function, range of motion, and health-related quality of life: a multicenter, single-blinded, randomized, controlled pilot study. J Neuroeng Rehabil. (2019) 16:122. doi: 10.1186/s12984-019-0595-8
65. Piron, L, Tombolini, P, Turolla, A, Zucconi, C, Agostini, M, and Dam, M. Reinforced feedback in virtual environment facilitates the arm motor recovery in patients after a recent stroke. Virtual Rehabil. (2007) 2007:121–3. doi: 10.1109/ICVR.2007.4362151
66. Turolla, A, Dam, M, Ventura, L, Tonin, P, Agostini, M, Zucconi, C, et al. Virtual reality for the rehabilitation of the upper limb motor function after stroke: a prospective controlled trial. J Neuroeng Rehabil. (2013) 10:85. doi: 10.1186/1743-0003-10-85
67. Turolla, A, Tonin, P, Zucconi, C, Agostini, M, Piccione, F, and Dam, MPL. Reinforcement feedback in virtual environment vs. conventional physical therapy for arm motor deficit after stroke. Virtual Rehabilit. (2007) 2007:49–52. doi: 10.1109/ICVR.2007.4362129
68. Gladstone, DJ, Danells, CJ, and Black, SE. The fugl-meyer assessment of motor recovery after stroke: a critical review of its measurement properties. Neurorehabil Neural Repair. (2002) 16:232–40. doi: 10.1177/154596802401105171
69. Augenstein, TE, Nagalla, D, Mohacey, A, Cubillos, LH, Lee, MH, Ranganathan, R, et al. A novel virtual robotic platform for controlling six degrees of freedom assistive devices with body-machine interfaces. Comput Biol Med. (2024) 178:108778. doi: 10.1016/j.compbiomed.2024.108778
70. Lim, I, Cha, B, Cho, DR, Park, E, Lee, KS, and Kim, M. Safety and potential usability of immersive virtual reality for brain rehabilitation: a pilot study. Games Health J. (2023) 12:34–41. doi: 10.1089/g4h.2022.0048
71. Ren, Y, Wang, Q, Liu, H, Wang, G, and Lu, A. Effects of immersive and non-immersive virtual reality-based rehabilitation training on cognition, motor function, and daily functioning in patients with mild cognitive impairment or dementia: a systematic review and meta-analysis. Clin Rehabil. (2024) 38:305–21. doi: 10.1177/02692155231213476
72. Zhang, J, Huang, M, Chen, Y, Liao, KL, Shi, J, Liang, HN, et al. TouchMark: partial tactile feedback Design for Upper Limb Rehabilitation in virtual reality. IEEE Trans Vis Comput Graph. (2024) 30:7430–40. doi: 10.1109/TVCG.2024.3456173
73. Wang, N, Liu, N, Liu, S, and Gao, Y. Effects of nonimmersive virtual reality intervention on children with spastic cerebral palsy: a Meta-analysis and systematic review. Am J Phys Med Rehabil. (2023) 102:1130–8. doi: 10.1097/PHM.0000000000002321
74. Schuck, SO, Whetstone, A, Hill, V, Levine, P, and Page, SJ. Game-based, portable, upper extremity rehabilitation in chronic stroke. Top Stroke Rehabil. (2011) 18:720–7. doi: 10.1310/tsr1806-720
75. Su, YS, Cheng, HW, and Lai, CF. Study of virtual reality immersive technology enhanced mathematics geometry learning. Front Psychol. (2022) 13:760418. doi: 10.3389/fpsyg.2022.760418
76. Slater, M . Immersion and the illusion of presence in virtual reality. Br J Psychol. (2018) 109:431–3. doi: 10.1111/bjop.12305
77. Shin, R, Choi, B, Choi, SM, and Lee, S. Implementation and evaluation of walk-in-place using a low-cost motion-capture device for virtual reality applications. Sensors. (2024) 24:848. doi: 10.3390/s24092848
Keywords: stroke, upper limb, rehabilitation, virtual reality, network meta-analysis, systematic review
Citation: Zhang J, Liu M, Yue J, Yang J, Xiao Y, Yang J and Cai E (2025) Effects of virtual reality with different modalities on upper limb recovery: a systematic review and network meta-analysis on optimizing stroke rehabilitation. Front. Neurol. 16:1544135. doi: 10.3389/fneur.2025.1544135
Received: 17 December 2024; Accepted: 17 March 2025;
Published: 01 April 2025.
Edited by:
Simona Bonavita, University of Campania Luigi Vanvitelli, ItalyReviewed by:
Gelu Onose, University of Medicine and Pharmacy “Carol Davila”, RomaniaCopyright © 2025 Zhang, Liu, Yue, Yang, Xiao, Yang and Cai. This is an open-access article distributed under the terms of the Creative Commons Attribution License (CC BY). The use, distribution or reproduction in other forums is permitted, provided the original author(s) and the copyright owner(s) are credited and that the original publication in this journal is cited, in accordance with accepted academic practice. No use, distribution or reproduction is permitted which does not comply with these terms.
*Correspondence: Jie Yang, a21zemNzc2RAMTYzLmNvbQ==; Enli Cai, eTE1OTI1MTEyOTEzQDE2My5jb20=
‡ORCID: Jiali Zhang, https://orcid.org/0009-0006-5632-8090
Enli Cai https://orcid.org/0000-0002-9916-1829
†These authors have contributed equally to this work
Disclaimer: All claims expressed in this article are solely those of the authors and do not necessarily represent those of their affiliated organizations, or those of the publisher, the editors and the reviewers. Any product that may be evaluated in this article or claim that may be made by its manufacturer is not guaranteed or endorsed by the publisher.
Research integrity at Frontiers
Learn more about the work of our research integrity team to safeguard the quality of each article we publish.