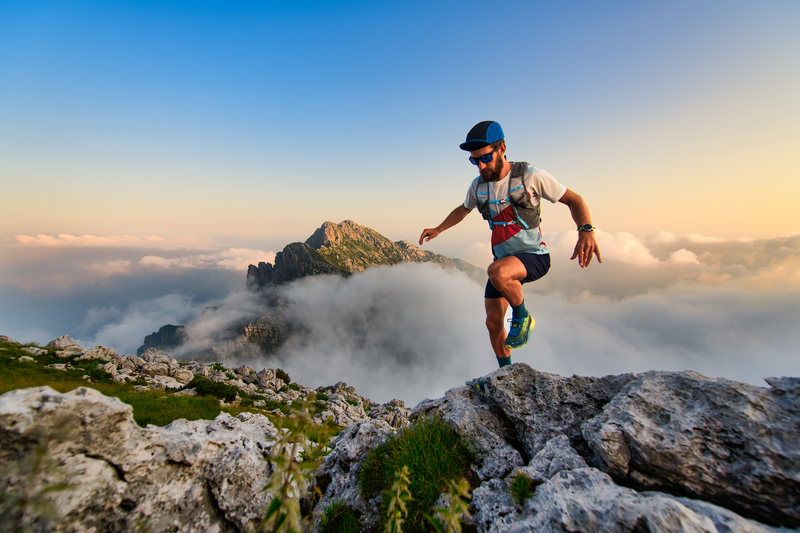
94% of researchers rate our articles as excellent or good
Learn more about the work of our research integrity team to safeguard the quality of each article we publish.
Find out more
BRIEF RESEARCH REPORT article
Front. Neurol.
Sec. Neurotrauma
Volume 16 - 2025 | doi: 10.3389/fneur.2025.1541520
The final, formatted version of the article will be published soon.
You have multiple emails registered with Frontiers:
Please enter your email address:
If you already have an account, please login
You don't have a Frontiers account ? You can register here
In chronic moderate-to-severe TBI (msTBI), depression is one of the most common psychiatric consequences. Yet to date, there is limited understanding of its neural underpinnings. This study aimed to better understand this gap by examining seed-to-voxel connectivity in depression, with all voxel-wise associations seeded to the bilateral anterior prefrontal cortices (aPFC). In a secondary analysis of 32 patients with chronic msTBI and 17 age-matched controls acquired from the Toronto Rehab TBI Recovery Study database, the Personality Assessment Inventory Depression scale scores were used to group patients into an msTBI-Dep group (T ≥ 60; n = 13) and an msTBI-Non-Dep group (T < 60; n = 19). Resting-state fMRI scans were analyzed using seed-based connectivity analyses. Ftests, controlling for age and education, were used to assess differences in bilateral aPFC rsFC across the 3 groups. After nonparametric permutation testing, the left aPFC demonstrated significantly increased rsFC with the left (p = 0.041) and right (p = 0.013) fusiform gyri, the right superior temporal lobe (p = 0.032), and the right precentral gyrus (p = 0.042) in the msTBI-Dep group compared to controls. The msTBI-Non-Dep group had no significant rsFC differences with either group. To our knowledge, this study is the first to examine aPFC rsFC in a sample of patients with msTBI exclusively. Our preliminary findings suggest a role for the aPFC in the pathophysiology of depressive symptoms in patients with chronic msTBI. Increased aPFC-sensory/motor rsFC could be associated with vulnerability to depression post-TBI, a hypothesis that warrants further investigation.
Keywords: chronic moderate-to-severe traumatic brain injury1, depression2, anterior prefrontal cortex3, seed-based connectivity analysis4, Personality assessment Inventory5, fusiform gyrus6 (Min.5-Max. 8)
Received: 07 Dec 2024; Accepted: 03 Mar 2025.
Copyright: © 2025 Elfaki, Sharma, Meusel, So, Colella, Wheeler, Harris and Green. This is an open-access article distributed under the terms of the Creative Commons Attribution License (CC BY). The use, distribution or reproduction in other forums is permitted, provided the original author(s) or licensor are credited and that the original publication in this journal is cited, in accordance with accepted academic practice. No use, distribution or reproduction is permitted which does not comply with these terms.
* Correspondence:
Layan A. Elfaki, Temerty Faculty of Medicine, University of Toronto, Toronto, M5S 1A8, Ontario, Canada
Disclaimer: All claims expressed in this article are solely those of the authors and do not necessarily represent those of their affiliated organizations, or those of the publisher, the editors and the reviewers. Any product that may be evaluated in this article or claim that may be made by its manufacturer is not guaranteed or endorsed by the publisher.
Research integrity at Frontiers
Learn more about the work of our research integrity team to safeguard the quality of each article we publish.