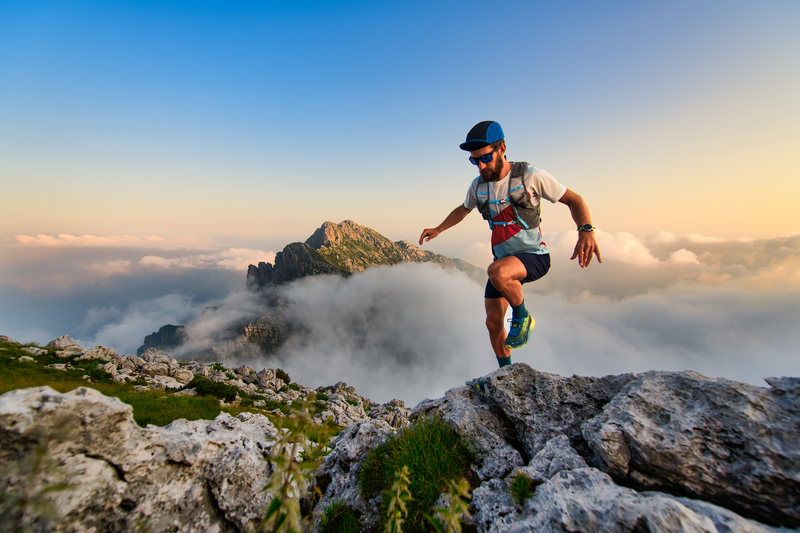
94% of researchers rate our articles as excellent or good
Learn more about the work of our research integrity team to safeguard the quality of each article we publish.
Find out more
SYSTEMATIC REVIEW article
Front. Neurol.
Sec. Neuro-Oncology and Neurosurgical Oncology
Volume 16 - 2025 | doi: 10.3389/fneur.2025.1532398
This article is part of the Research Topic Artificial Intelligence in Neurosurgical Practices: Current Trends and Future Opportunities View all articles
The final, formatted version of the article will be published soon.
You have multiple emails registered with Frontiers:
Please enter your email address:
If you already have an account, please login
You don't have a Frontiers account ? You can register here
Objective: This study systematically reviewed deep learning (DL) applications in neurosurgical practice to provide a comprehensive understanding of DL in neurosurgery. The review process included a systematic overview of recent developments in DL technologies, an examination of the existing literature on their applications in neurosurgery, and insights into the future of neurosurgery. The study also summarized the most widely used DL algorithms, their specific applications in neurosurgical practice, their limitations, and future directions.Materials and Methods: An advanced search using medical subject heading terms was conducted in Medline (via PubMed), Scopus, and Embase databases restricted to articles published in English. Two independent neurosurgically experienced reviewers screened selected articles.Results: A total of 456 articles were initially retrieved. After screening, 162 were found eligible and included in the study. Reference lists of all 162 articles were checked, and 19 additional articles were also found eligible and included in the study. The 181 included articles were divided into 6 categories according to the subspecialties: general neurosurgery (n=64), neuro-oncology (n=49), functional neurosurgery (n=32), vascular neurosurgery (n=17), neurotrauma (n=9), and spine and peripheral nerve (n=10). The leading procedures in which DL algorithms were most commonly used were deep brain stimulation and subthalamic and thalamic nuclei localization (n=24) in the functional neurosurgery group; segmentation, identification, classification, and diagnosis of brain tumors (n=29) in the neuro-oncology group; and neuronavigation and image-guided neurosurgery (n=13) in the general neurosurgery group. Apart from various video and image datasets, computed tomography, magnetic resonance imaging, and ultrasonography were the most frequently used datasets to train DL algorithms in all groups overall (n=79). Although there were few studies involving DL applications in neurosurgery in 2016, research interest began to increase in 2019 and has continued to grow in the 2020s.Conclusions: DL algorithms can enhance neurosurgical practice by improving surgical workflows, real-time monitoring, diagnostic accuracy, outcome prediction, volumetric assessment, and neurosurgical education. However, their integration into neurosurgical practice involves challenges and limitations. Future studies should focus on refining DL models with a wide variety of datasets, developing effective implementation techniques, and assessing their affect on time and cost efficiency.
Keywords: Artificial ıntelligence, Convolutional Neural Network, deep learning, machine learning, Neurological Surgery, neurosurgery AI, artificial intelligence, CBCT, cone-beam computed tomography, CLE, confocal laser endomicroscopy
Received: 21 Nov 2024; Accepted: 04 Mar 2025.
Copyright: © 2025 Yangi, Hong, Gholami, On, Reed, Puppalla, Chen, Calderon Valero, Xu, Li, Santello, Lawton and Preul. This is an open-access article distributed under the terms of the Creative Commons Attribution License (CC BY). The use, distribution or reproduction in other forums is permitted, provided the original author(s) or licensor are credited and that the original publication in this journal is cited, in accordance with accepted academic practice. No use, distribution or reproduction is permitted which does not comply with these terms.
* Correspondence:
Mark Preul, Division of Neurological Surgery, Barrow Neurological Institute (BNI), Phoenix, 85013, Arizona, United States
Disclaimer: All claims expressed in this article are solely those of the authors and do not necessarily represent those of their affiliated organizations, or those of the publisher, the editors and the reviewers. Any product that may be evaluated in this article or claim that may be made by its manufacturer is not guaranteed or endorsed by the publisher.
Research integrity at Frontiers
Learn more about the work of our research integrity team to safeguard the quality of each article we publish.