- 1Department of Radiology, Loma Linda University School of Medicine, Loma Linda, CA, United States
- 2UCLA Steve Tisch BrainSPORT Program, University of California, Los Angeles, Los Angeles, CA, United States
- 3Department of Statistics, Stanford University, Stanford, CA, United States
- 4Department of Kinesiology, Occidental College, Los Angeles, CA, United States
- 5Department of Neurology, David Geffen School of Medicine at UCLA, Los Angeles, CA, United States
- 6School of Medicine, University of California, Irvine, Irvine, CA, United States
- 7Department of Radiology, Wake Forest School of Medicine, Winston-Salem, NC, United States
Traumatic brain injury (TBI) is common and costly. Although neuroimaging modalities such as resting-state functional MRI (rsfMRI) promise to differentiate injured from healthy brains and prognosticate long-term outcomes, the field suffers from heterogeneous findings. To assess whether this heterogeneity stems from variability in the TBI populations studied or the imaging methods used, and to determine whether a consensus exists in this literature, we performed the first systematic review of studies comparing rsfMRI functional connectivity (FC) in patients with TBI to matched controls for seven canonical brain networks across injury severity, age, chronicity, population type, and various imaging methods. Searching PubMed, Web of Science, Google Scholar, and ScienceDirect, 1,105 manuscripts were identified, 50 fulfilling our criteria. Across these manuscripts, 179 comparisons were reported between a total of 1,397 patients with TBI and 1,179 matched controls. Collapsing across injury characteristics, imaging methods, and networks, there were roughly equal significant to null findings and increased to decreased connectivity differences reported. Whereas most factors did not explain these mixed findings, stratifying across severity and chronicity, separately, showed a trend of increased connectivity at higher severities and greater chronicities of TBI. Among methodological factors, studies were more likely to find connectivity differences when scans were longer than 360 s, custom image processing pipelines were used, and when patients kept their eyes open versus closed during scans. We offer guidelines to address this variability, focusing on aspects of study design and rsfMRI acquisition to move the field toward reproducible results with greater potential for clinical translation.
Introduction
In 2014, the Center for Disease Control and Prevention (CDC) reported 2.53 million traumatic brain injury (TBI) emergency department visits, resulting in 288,000 TBI-related hospitalizations and 56,800 TBI-related deaths (1). TBI is a broad diagnostic category encompassing massive heterogeneity in the injured population as well as injury mechanisms, severities, and chronicities. Despite the prevalence of TBI, this heterogeneity has complicated the search for objective markers of injury and predictors of disability.
Advances in neuroimaging over the last decade have produced powerful tools to detect potential markers of brain injury and recovery. Though standard clinical neuroimaging is useful for identifying structural pathology in TBI, it has limitations in identifying pathophysiological mechanisms of injury-related symptoms and outcomes (2–4). Resting-state functional MRI (rsfMRI) has recently increased in popularity to detect functional alterations in large-scale brain networks (5) that are distinct from structural abnormalities, potentially providing insights into outcomes where other imaging has not. RsfMRI relies on correlated slow wave fluctuations in the blood oxygenation level dependent (BOLD) signal between brain regions as an index of functional connectivity (FC) (6–8). FC between regions has identified several reproducible, large-scale brain networks that map nicely onto groups of brain regions that co-activate in task-based fMRI studies and/or share structural connectivity in diffusion-based MRI in human and non-human primates, such as the default mode, executive, salience, and somatomotor networks (7, 9). Comparisons of FC within networks across healthy and diseased groups can provide insight into functional mechanisms of disease and targets for treatment (5, 10, 11).
In these ways, rsfMRI has the potential to capture diffuse connectional changes to the brain that may be a hallmark of the shearing forces of trauma (12). To date, hundreds of rsfMRI studies have been conducted in TBI, including several reviews on the topic. The prior reviews have been helpful in identifying patterns of connectional differences in specific subgroups of patients with TBI (e.g., chronic pain, headache, severe TBI, blast-related injury), using specific rsfMRI analysis methods, such as graph analysis, or in combination with other imaging modalities, such as diffusion imaging (13–20). Despite the growing popularity of rsfMRI in TBI, the field suffers from heterogeneous findings. Though the prior reviews provide valuable insights into niches within the field, they were not designed to resolve the massive heterogeneity in this literature, which might be achieved through more liberal study inclusion.
We performed a comprehensive systematic review of rsfMRI studies that compared FC between patients with TBI and matched controls. Our primary goal was to perform a broader, more inclusive review of the literature to identify and characterize consistent FC differences that may only become apparent when collapsing across or parsing out the results by injury severity, chronicity, age, population type, as well as network and imaging methods. We selected these factors because they are commonly reported, have face validity, and have been studied as possible confounds or covariates to rsfMRI differences in TBI. For example, studies show that differences in FC occur over time since injury (21, 22), and outcomes vary as a function of injury severity, age, chronicity, and population type (23–27). We attempted to reduce the complexity in this review by organizing results into comparisons of FC within seven of the most commonly studied canonical ICA networks (28).
Methods
Identification
This literature review was conducted in accordance with the Preferred Reporting Items for Systematic Reviews and Meta-Analyses (PRISMA) guidelines (29), as depicted in Figure 1. PubMed, Web of Science, Google Scholar, and ScienceDirect were queried with keywords to generate articles. Articles prior to July 2020 were included, yielding 1,097 records (database search in Figure 1). Additional targeted searches were conducted to identify articles from known rsfMRI researchers prior to July 2020 that were not captured by the four database searches, yielding eight records (targeted author search in Figure 1). In total, we identified 1,105 records for screening.
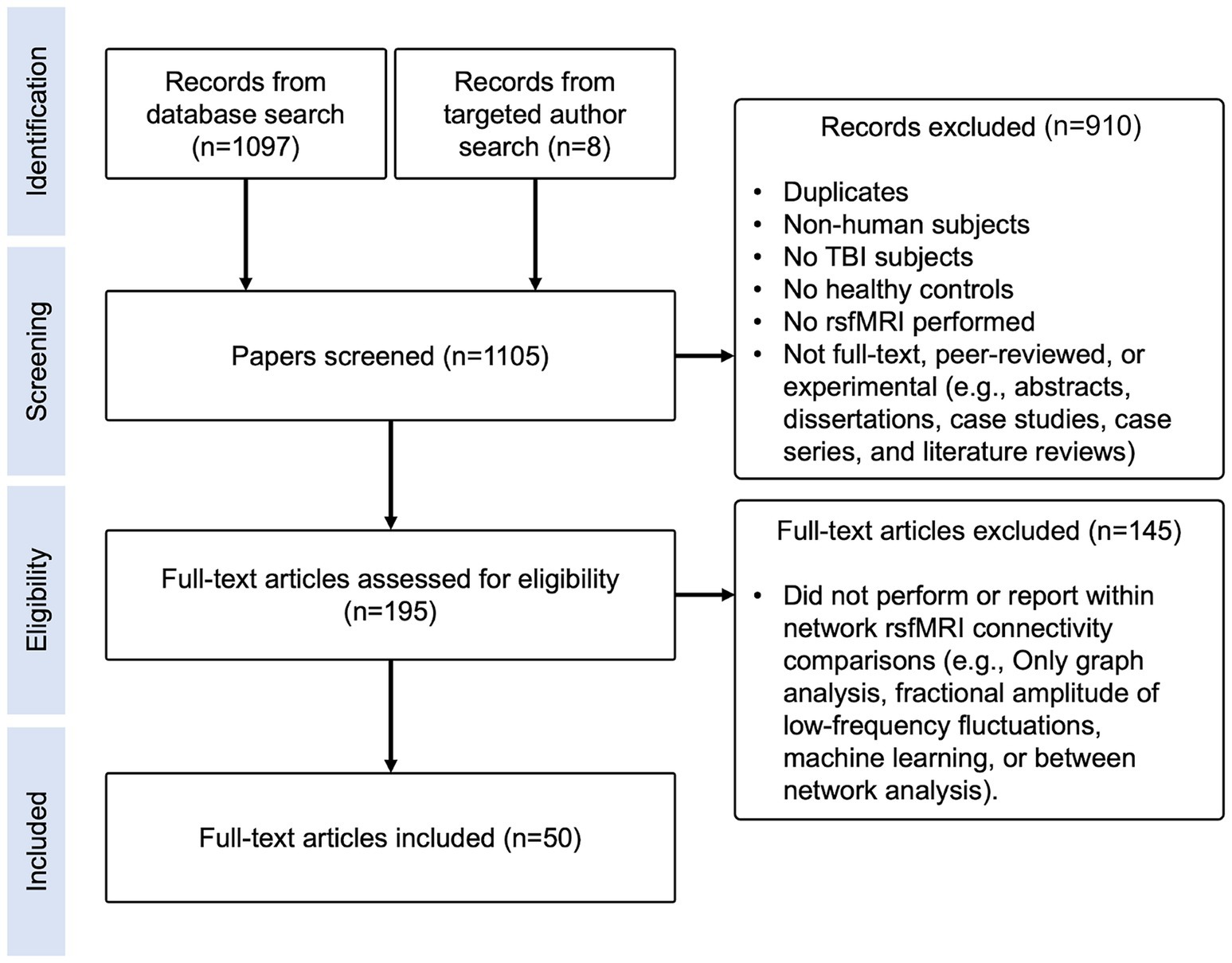
Figure 1. Literature review flow diagram. Diagram depicting identification, screening, eligibility, and inclusion steps performed for this literature review.
Keywords for PubMed included: (traumatic brain injury [Title/Abstract]) OR (tbi [Title/Abstract]) AND (rsfmri [Title/Abstract]) OR (resting-state [Title/Abstract]). Keywords for Web of Science included: TOPIC: (“resting state fMRI” “traumatic brain injury”) OR (“rsfMRI” “TBI”) OR (“resting state” “MRI” “traumatic brain injury”) OR (“rsfMRI” “traumatic brain injury”) OR (“resting state fMRI” “TBI”) across all years and databases. Keywords for Google Scholar included: “traumatic brain injury” “resting state functional MRI” -rats -dementia -"hepatic encephalopathy” - “task based.” Keywords for ScienceDirect included: Title, abstract, keywords: (“Traumatic brain injury” or “TBI”) AND (“resting state” OR “rs-fmri”).
Exclusion criteria
Exclusion criteria included: non-human subjects, no TBI subjects, no matched controls, no rsfMRI performed, task-based fMRI only, abstracts, dissertations, case studies, case series, literature reviews, studies that only performed analyses using graph theory, machine learning, other novel methods, or did not report within-network FC results (Figure 1).
Screening
Query results were converted to the.bib format and imported into a shared folder in Mendeley. Two rounds of selection were performed by evaluating titles and abstracts. Among six reviewers, two independently reviewed each manuscript for inclusion/exclusion criteria. If they disagreed, Dr. Bickart made the final decision. After screening, 910 manuscripts were excluded, 75 of which were duplicates, leaving 195 papers for final eligibility screening (Figure 1).
Eligibility
Next, the reviewers read each paper completely and excluded an additional 145 papers based on the same inclusion/exclusion criteria as above (Figure 1). In total, three papers via targeted search and 47 papers via database search were included, yielding 50 papers.
Data extraction
The following information was extracted from each manuscript: mechanism of injury, TBI severity, TBI sample information (age statistics, gender, sample size), control sample information, time post-injury, cross-sectional and/or longitudinal results, scanner manufacturer, tesla strength, coil channel, scan duration, slice thickness, eyes open or closed, sequences acquired during fMRI acquisition, image processing pipeline, software used, rsfMRI metrics computed, analysis type, functional networks/regions investigated, and statistics reported. For longitudinal studies, we extracted the results of cross-sectional, between-group FC comparisons at different time points (i.e., acute, subacute, or chronic), but did not extract within-group FC comparisons over time.
Defining canonical resting-state networks
For comparison purposes, the data extracted from each paper was organized into seven canonical networks based on a widely used and previously defined parcellation scheme (30, 31). The networks included Default Mode (DMN), Executive Control (ECN), Limbic (LN), Dorsal Attention (DAN), Salience (SN) or Ventral Attention (VAN), Visual (VN), and Sensorimotor (SMN) (Figure 2). Findings reported for seed-based or region-of-interest (ROI) analyses were assigned to the network that incorporated that seed or ROI according to the map depicted in Figure 2 and region assignment listed in Table 1. Most studies that outlined their own seed/ROI network assignments adhered to this model. With the data organized into these networks, we could then tabulate results on a comparison-by-comparison basis. Given that studies ranged in the number of networks they analyzed, we report findings for every comparison made across the 50 studies included (Table 2). We only extracted and reported results from the within-network analyses and excluded graph, between-network, and other analyses to enable a semiquantitative summary of results—quantifying the proportion of comparisons per network that were significantly different between TBI and control populations.
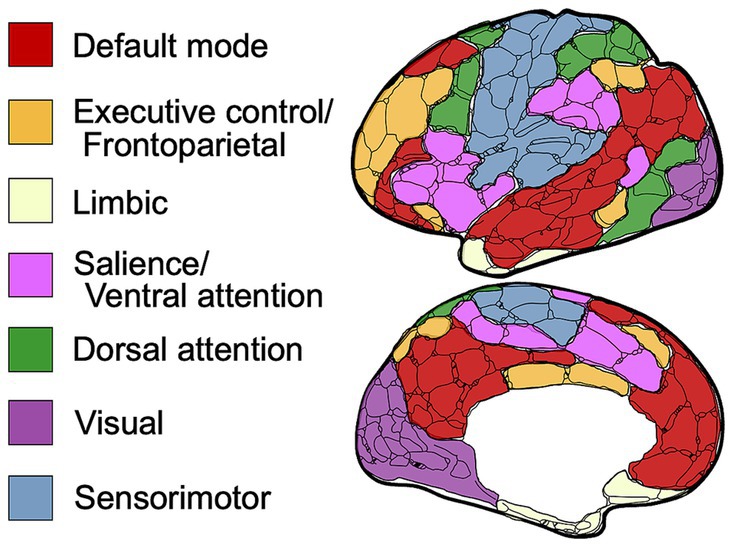
Figure 2. Schematic of resting-state networks used in this review. Visual schematic depicting the anatomical location of each defined canonical brain network.
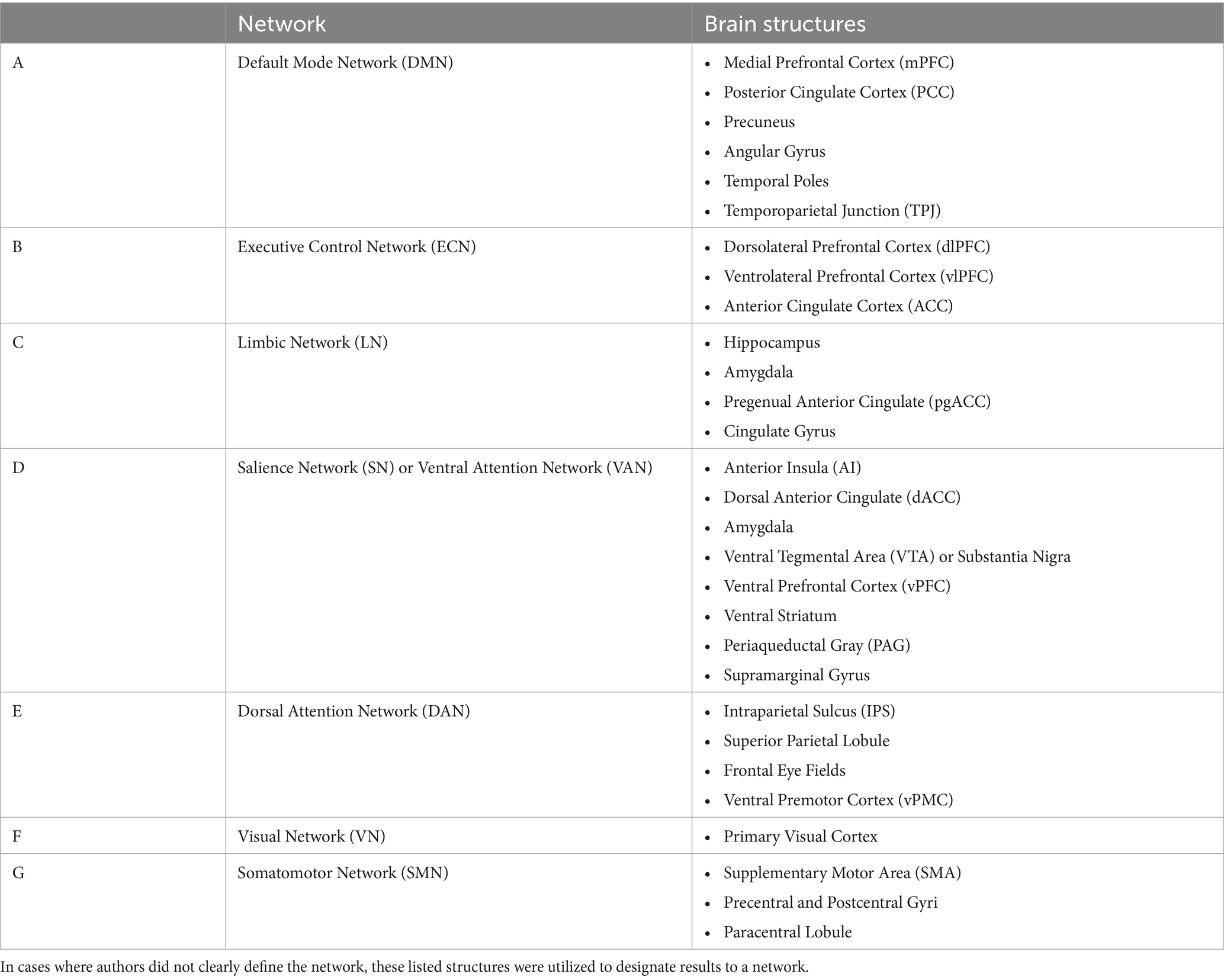
Table 1. Canonical networks broken down by most commonly referenced brain regions and in accordance with previously defined parcellation schemes (30, 31).
Quality assurance
The original authors of all included papers were emailed to verify our interpretation of the results for this review and to obtain additional statistics for a meta-analysis. Nineteen of the lead authors were able to both verify our interpretation and provide the relevant statistics. This was, however, not enough to perform a meta-analysis.
After the data was validated, the number of reported significant increases, decreases, and no differences in connectivity were manually counted by the reviewers. To ensure accurate transmission of article annotation, code was written to count and organize the data into a database (Literature Review Toolkit: rsfMRI in TBI [Source code]).1 The manual counts and code were compared and mistakes were corrected until both were in concordance.
Parsing the findings into categories
To determine if findings varied by injury characteristics, demographic variables, or methodological parameters, we parsed the findings into categories: injury severity, age, chronicity, population type, and a variety of imaging parameters (Figure 3). These categorizations are derived from the prevailing consensus across TBI literature.
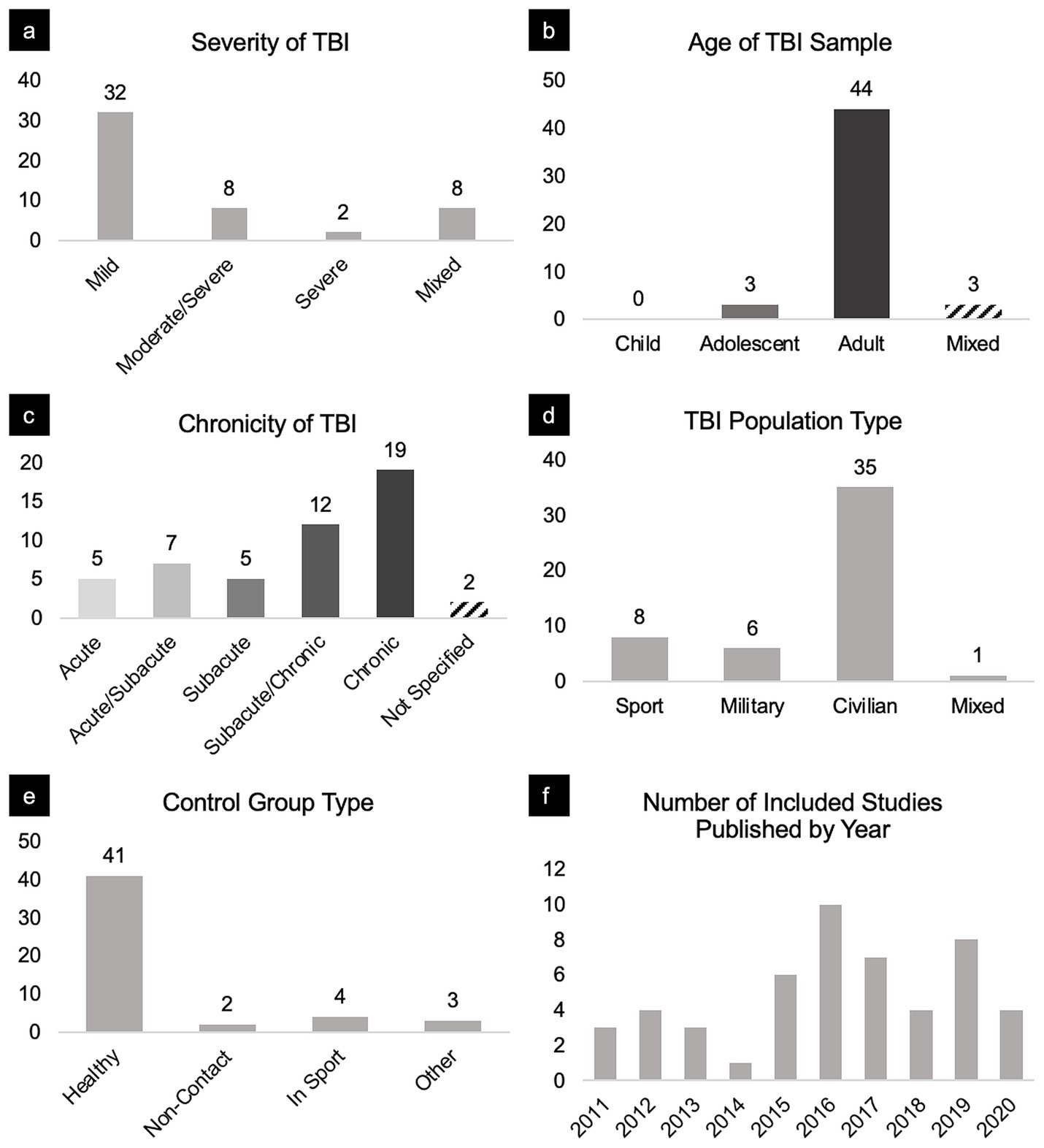
Figure 3. Breakdown of sample characteristics and studies included in this review. Panels portray the distribution of included studies broken down by (a) severity, (b) age, (c) chronicity, (d) TBI population type, (e) control group, and (f) year published.
For severity, we classified results pertaining to TBI patients based on their Glasgow Coma Scale (GCS) scores (32, 33). GCS scores greater than or equal to 13 were classified as mild, GCS scores ranging from 9 and 12 were considered moderate, and GCS scores less than or equal to 8 were considered severe. Some studies included TBI participants of varying severities. Therefore, we also categorized findings as mild/moderate, moderate/severe, or mixed (i.e., mild, moderate, and severe for such cases).
For age, we classified studies involving subjects aged 12–18 as adolescent, age 18 and greater as adult, and otherwise as mixed. Studies that met the inclusion criteria did not identify participants younger than 12 years old.
For chronicity, there is no standard or agreed-upon definition of acute, subacute, and chronic. We chose to classify TBI chronicity based on sources that use biomarkers to track injury progression. For example, such studies (34, 35) define acute TBI as within days, subacute within weeks, and chronic as months to years post-injury. Clinically, there is also some consensus around chronicity as it relates to post-concussion syndrome (PCS). Specifically, PCS refers to a characteristic cluster of symptoms lasting greater than or equal to 3 months after injury (36). We therefore classified scans acquired within the first week post-injury as acute, between 1 and 12 weeks post-injury as subacute, and >12 weeks post-injury injury as chronic. Again, some studies included TBI participants of varying chronicities. Therefore, we also categorized findings as acute/subacute and subacute/chronic to include these comparisons. For longitudinal studies with comparisons between TBI and matched control groups at various times since injury, we treated each comparison as unique, rather than reporting changes in FC over time for either group individually.
For population type, we categorized studies by sport (Sport), military personnel (Military), civilians not injured in sport (Civilian), and mixed if participants were from an array of the aforementioned populations (Mixed).
Analysis
We pooled and parsed the findings from comparisons of TBI patients to matched controls, counting the number of reported significant increases and decreases in connectivity or no significant differences in connectivity. Significance was determined by the authors of each study. We would have preferred to perform a meta-analysis to account for differences in effect sizes across studies, but very few studies reported or responded to our inquiries to produce the necessary statistics for this. Comparisons were first collapsed across injury characteristics, such that the connectivity differences could be compared across each of the canonical networks (Figure 4). Next, comparisons were collapsed across networks, allowing for connectivity differences to be compared across injury characteristics (Figure 5). Lastly, connectivity differences were examined across both network and injury characteristics to allow for analysis of each TBI category or demographic within a specified network (Figure 6).
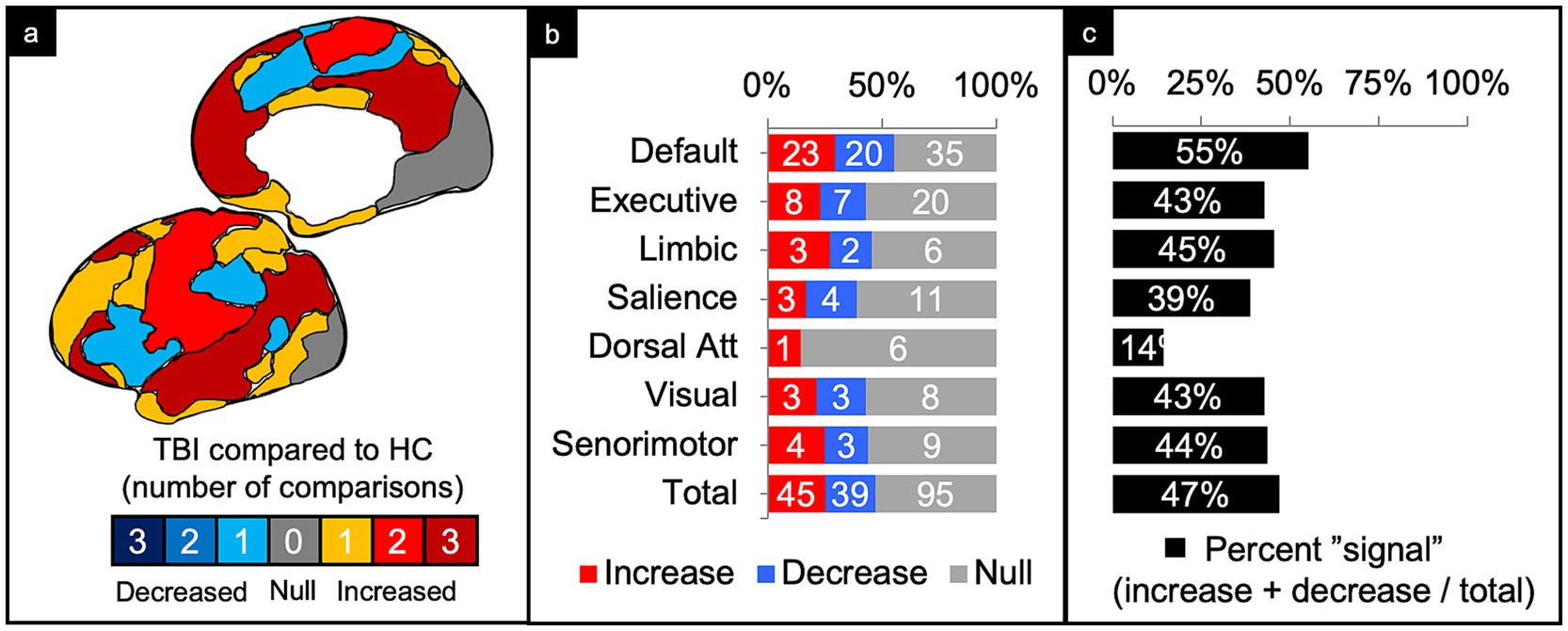
Figure 4. Connectivity comparisons collapsed across injury characteristics. Visual schematic depicting degree of network intensity corresponding to increased or decreased connectivity (a). Comparisons were summed across study and injury characteristics, then stratified by network to display the proportion of increased, decreased, or no (i.e., null) connectivity differences between TBI and control populations (b) and the percent signal of having any significant difference (c).
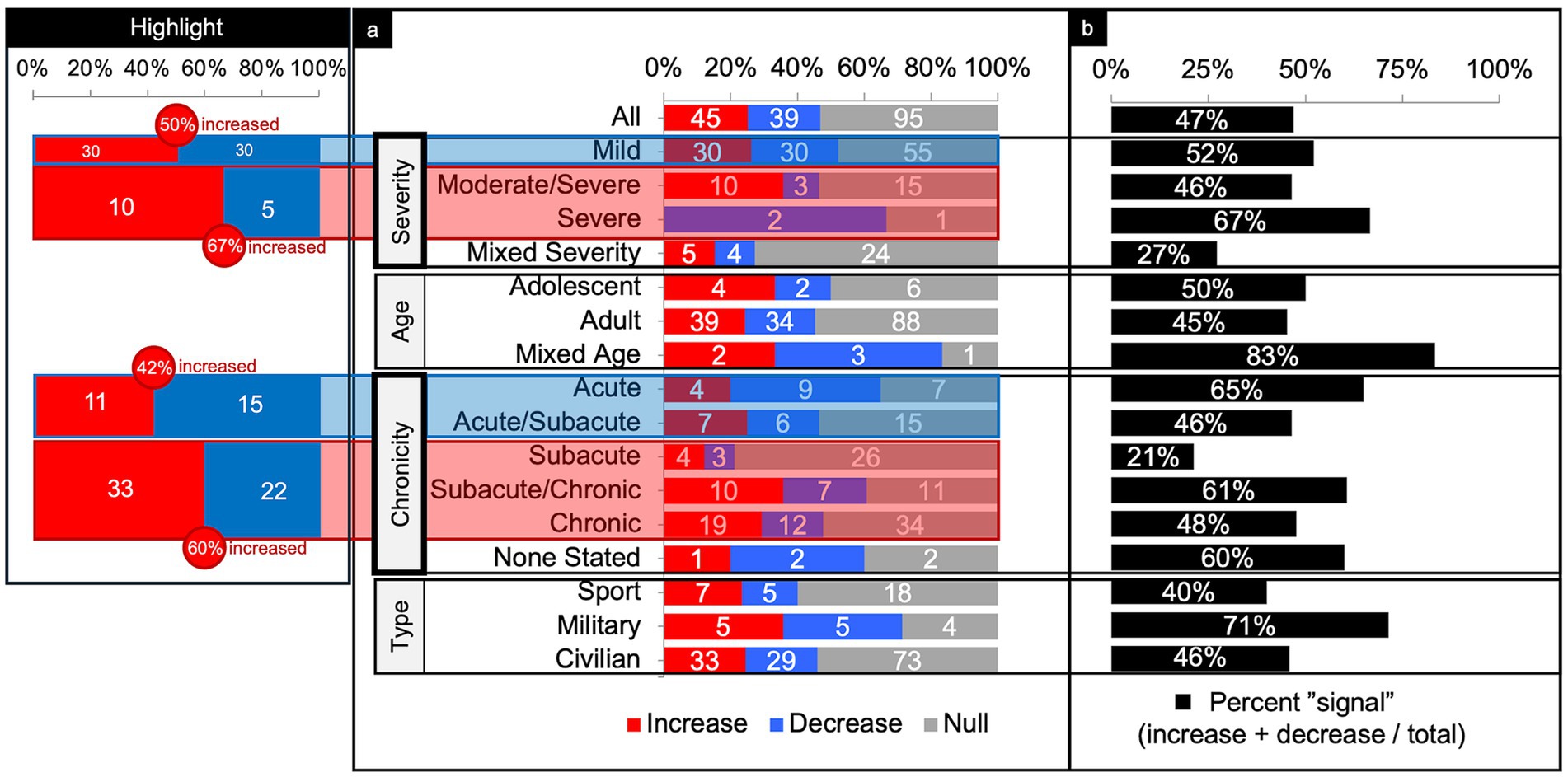
Figure 5. Connectivity comparisons collapsed across networks. Comparisons were summed across study and networks, then stratified by injury characteristics to display the proportion of increased, decreased, or no (i.e., null) connectivity differences between TBI and control populations (a) and the percent signal of having any significant difference (b). The most consistent findings were increased connectivity in the higher severities and chronicities of TBI (Highlight), independent of the other variables.
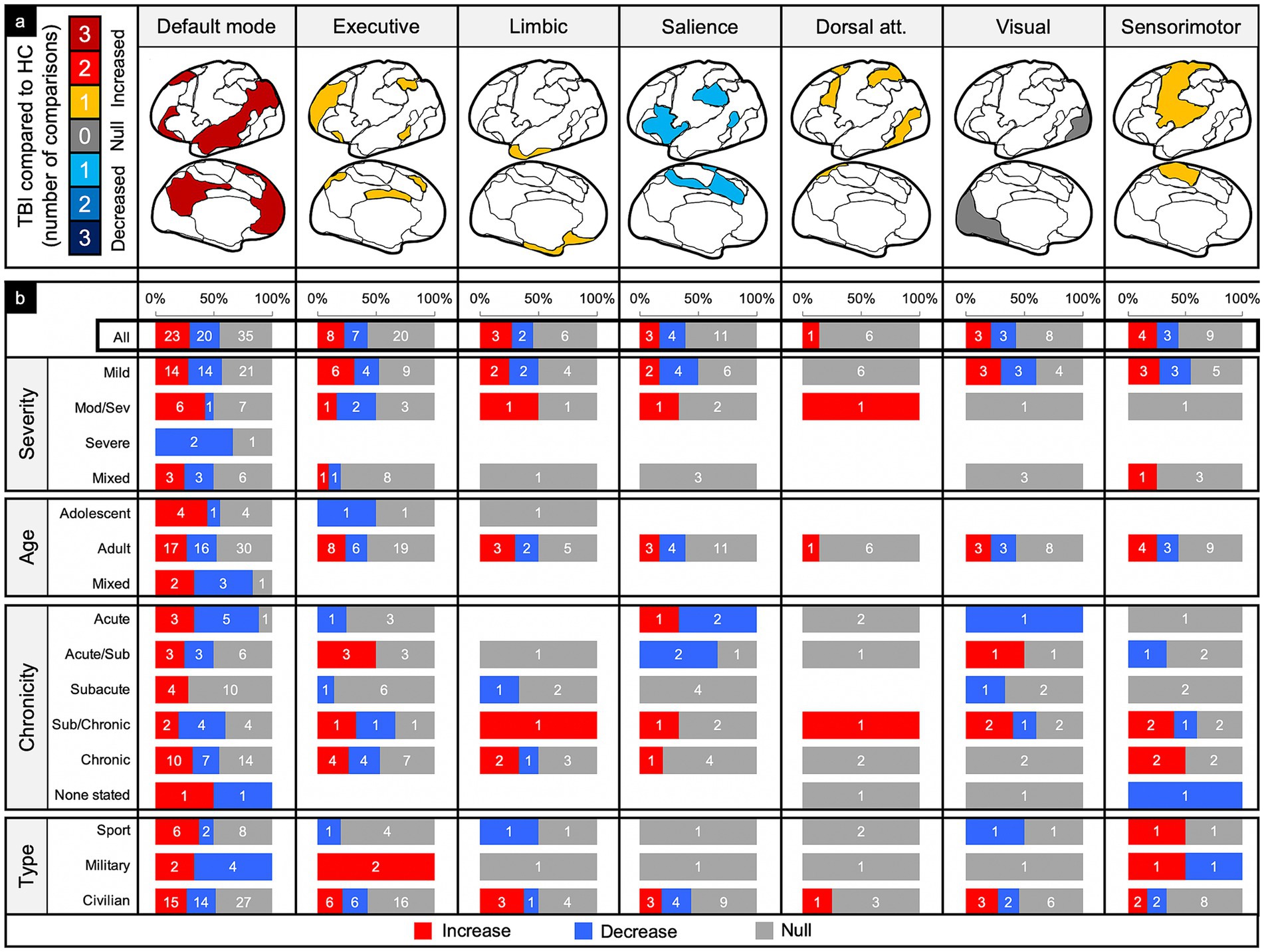
Figure 6. Connectivity comparisons stratified by network and injury characteristics. Comparisons were summed across study, then stratified by networks and injury characteristics to display the proportion of increased, decreased, or no (i.e., null) connectivity differences between TBI and control populations (a) and the percent signal of having any significant difference (b).
Alongside analysis collapsed by injury characteristics or network, percent global signal was calculated. Percent global signal was defined as the number of increases and decreases in connectivity relative to matched controls divided by the total number of connectivity comparisons. The goal was to use percent signal as a proxy for the degree to which networks reported any difference in connectivity following TBI compared to controls, regardless of whether the connectivity increased or decreased (Figures 4, 5).
Results
Studies included
From 1,105 manuscripts, 50 met inclusion criteria, reporting a total of 179 network connectivity comparisons between a total of 1,397 TBI patients and 1,179 matched controls; an average of 3.58 comparisons per manuscript. TBI cohorts primarily included civilian adults with a mild severity of TBI in the subacute to chronic stage (Figure 3).
Comparisons collapsed across injury characteristics
Of the 179 comparisons, the majority were conducted in DMN (44%) and the least in the DAN (4%) (Figure 4b). Collapsing across injury characteristics and networks, 25% of comparisons showed increased connectivity, 22% showed decreased connectivity, and 53% showed no significant differences between the TBI and control groups, yielding a percent signal of 47% (Figures 4a–c).
Comparisons collapsed across all networks
Severity
Across severity, the majority of comparisons (64%) were performed on patients with mild TBI (Figure 5). Parsing findings by TBI severity showed a trend toward increased connectivity with higher severity. In patients with mild TBI, connectivity differences were evenly split between increased and decreased connectivity (50% increased) with a percent signal of 52%. In higher severities however, more comparisons showed increased than decreased connectivity (60% increased, collapsing across moderate/severe and severe) with roughly comparable percent signal to that of mild TBI (46–67%).
Age
Across age, the majority of comparisons (88%) involved adult participants (Figure 5). Percent signal within the adult TBI group was 45%, with a slight tendency toward increased connectivity.
Chronicity
Across chronicity, the majority of comparisons (36%) were performed during the chronic phase (Figure 5). Parsing findings by TBI chronicity showed a trend toward increased connectivity with high chronicity. That is, comparisons performed in the acute and acute/subacute phase showed less increased than decreased connectivity (42% increased) whereas comparisons in the subacute through chronic phase showed more increased than decreased connectivity (60% increased). Percent signal across the chronicities was similar (range from 46 to 65%), except for the subacute, only, category (21%).
Population type
Across the population type, the majority of comparisons (75%) were performed in civilians (Figure 5). Percent signal across civilians was 46%, with a slight tendency toward increased connectivity (53% increased). Notably, comparisons in military samples yielded a relatively high (71%) chance of finding any difference in FC compared to controls.
Comparisons within networks
Default mode network (DMN)
Of the 78 comparisons between TBI and control groups within the DMN, 55% demonstrated a significant difference in connectivity across all categories of TBI and population factors (Figure 4c), with a slight majority showing increased connectivity (Figure 6, column 1). This pattern of mixed results generally persisted even when parsing the findings by age and population type. Notably, comparisons made in populations with moderate/severe TBI or in the subacute time frame were more likely to show increased connectivity.
Executive control network (ECN)
Of the 35 comparisons reported in the ECN, 43% demonstrated a significant difference across all categories of TBI and population factors (Figure 4c), with a nearly even split between increased and decreased connectivity (Figure 6, column 2). This pattern of mixed results persisted when parsing the data by severity, age, and population type. Notably, comparisons made in the acute/subacute time frame revealed solely increased connectivity when a significant difference was detected.
Limbic network (LN)
Of the 11 comparisons reported in the LN, 45% demonstrated a significant difference across all categories of TBI and population factors (Figure 3c), with a nearly even split between increased and decreased connectivity (Figure 6, column 3). This pattern of mixed results persisted when parsing the data by severity, age, chronicity, and population type.
Salience network (SN)
Of the 18 comparisons reported in the SN, 39% demonstrated a significant difference across all categories of TBI and population factors (Figure 4c), with a nearly even split between increased and decreased connectivity (Figure 6, column 4). This pattern of mixed results persisted when parsing the data by severity, age, chronicity, and population type. Notably, comparisons made in the SN showed the second lowest percent signal of all the networks across all categories of TBI and population factors.
Dorsal attention network (DAN)
The DAN was the least studied network. Of the seven comparisons reported in the DAN, 14% demonstrated a significant difference across all categories of TBI and population factors (Figure 4c), showing only increased connectivity (Figure 6, column 5).
Visual network (VN)
Of the 14 comparisons reported in the VN, 43% demonstrated a significant difference across all categories of TBI and population factors (Figure 4c), with an even split between increased and decreased connectivity (Figure 6, column 6). This pattern of mixed results persisted when parsing the data by severity, age, chronicity, and population type.
Sensorimotor network (SMN)
Of the 16 comparisons reported in the SMN, 44% demonstrated a significant difference across all categories of TBI and population factors (Figure 4c), with a nearly even split between increased and decreased connectivity (Figure 6, column 7). This pattern of mixed results persisted when parsing data by severity, age, chronicity, and population type.
Comparisons organized by methodology
Eye methodology
The majority of studies (75%) reported whether subjects were instructed to keep their eyes closed, open, or fixated during the scan (Figure 7). Of the comparisons conducted from scans instructing subjects to have their eyes closed (53%), 33% showed a significant difference between TBI and control samples. Of those that found a difference, there was a slight majority of decreased connectivity (55%).
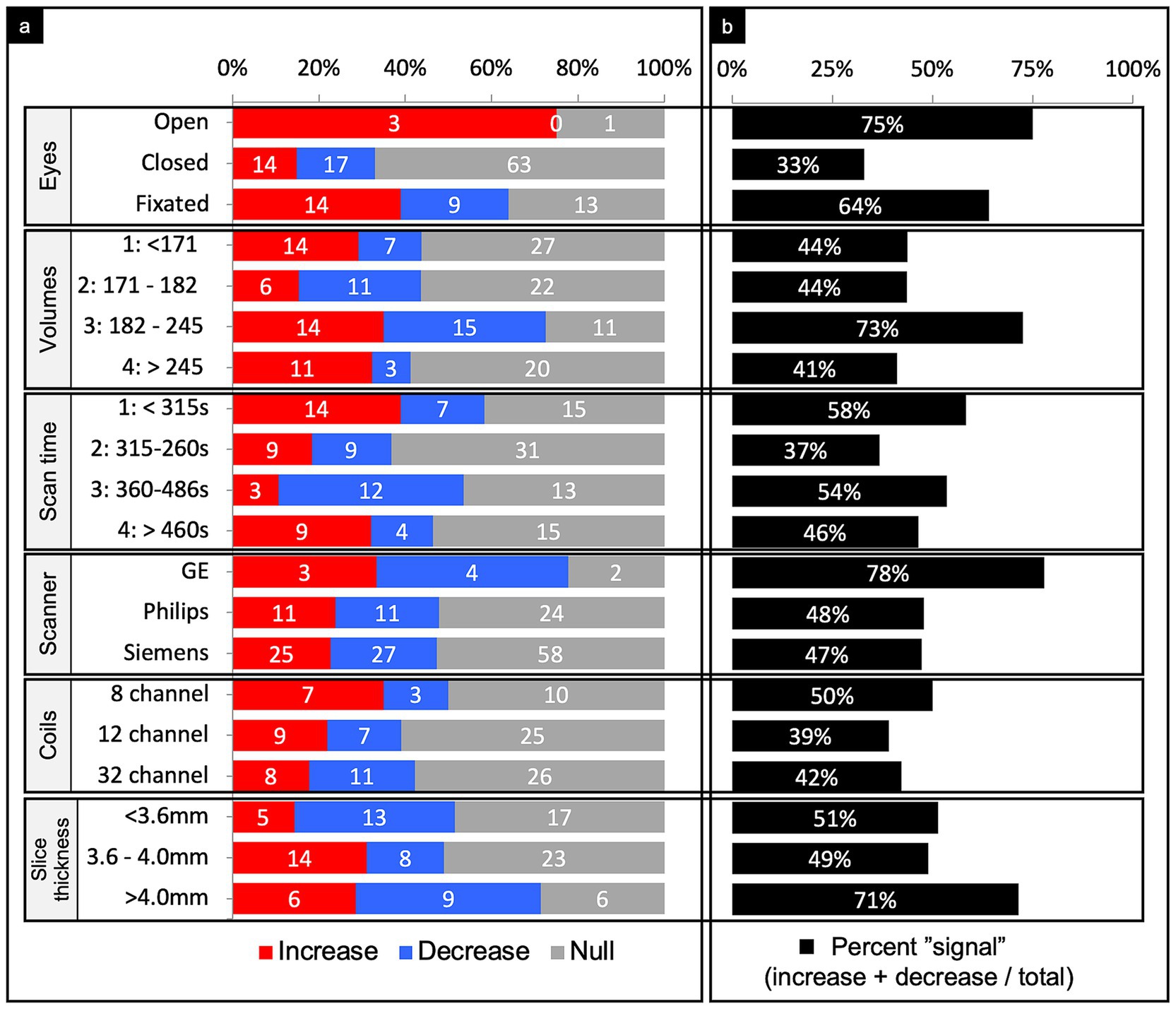
Figure 7. Connectivity comparisons stratified by scanner methodology. Comparisons were summed across study, networks, and injury characteristics, then stratified by imaging methodology to display the proportion of increased, decreased, or no (i.e., null) connectivity differences between TBI and control populations (a) and the percent signal of having any significant difference (b). For the number of volumes and scan time, we stratified the comparisons by quartiles. For the slice thickness, we stratified comparisons by tertiles. All methodological variables listed refer to the rsfMRI sequence only.
Number of volumes
Quartiles were generated based on the distribution of the number of volumes taken. Observations were then sorted according to the quartile range they fell into (Figure 7). 10% of the comparisons did not report the number of volumes acquired during the scan. Among the 3rd quartile of scan volumes, percent signal was 72.5%, which was noticeably higher than the other quartiles. Of those that found a difference in the 3rd quartile, findings of increased or decreased connectivity were roughly equal.
Scan time
Quartiles were generated based on the distribution of the scan duration in seconds. Observations were then sorted into the quartile range they fell into (Figure 7). 21% of the comparisons did not report the scan duration. Among the second quartile of scan duration, percent signal was 37%, which was noticeably lower than the other quartiles. Of those that found a difference in the 2nd quartile, findings of increased or decreased connectivity were equal.
Scanner manufacturer
Other than three studies (14 comparisons), all used a single scanner for acquisition, which included either GE, Philips, or Siemens (Figure 7). The majority used Siemens. Results were roughly equally mixed across increased, decreased, and null when organized by scanner manufacturer.
Coil channels
Only 30 studies (61% of comparisons) reported the number of coil channels used. The majority used the 32 channel coil followed by a 12 channel coil (Figure 7). As above, the findings were roughly evenly mixed results across coil channel number.
Slice thickness
Thirty four studies (56% of comparisons) reported the slice thickness. Tertiles were generated based on the distribution of slice thickness in millimeters (Figure 7). Among the 3rd tertile of slice thickness, percent signal was 71%, which was noticeably higher than the other two tertiles.
Tesla strength and acquisition sequence
We did not parse findings by tesla strength or acquisition sequence given they did not differ across the studies. Specifically, all 50 studies used a 3-Tesla MRI machine and acquired a T2 gradient-echo, echo-planar (EPI) sequence. No other fMRI sequence was consistently reported.
Preprocessing software
A variety of preprocessing software was used across the literature. The five most common softwares used were SPM8 (28%), FSL (13%), AFNI (13%), SPM12 (11%), and FMRIB (6%) (Figure 8). Further, 23% of comparisons combined multiple preprocessing softwares or used their own custom pipelines, usually based in MATLAB.
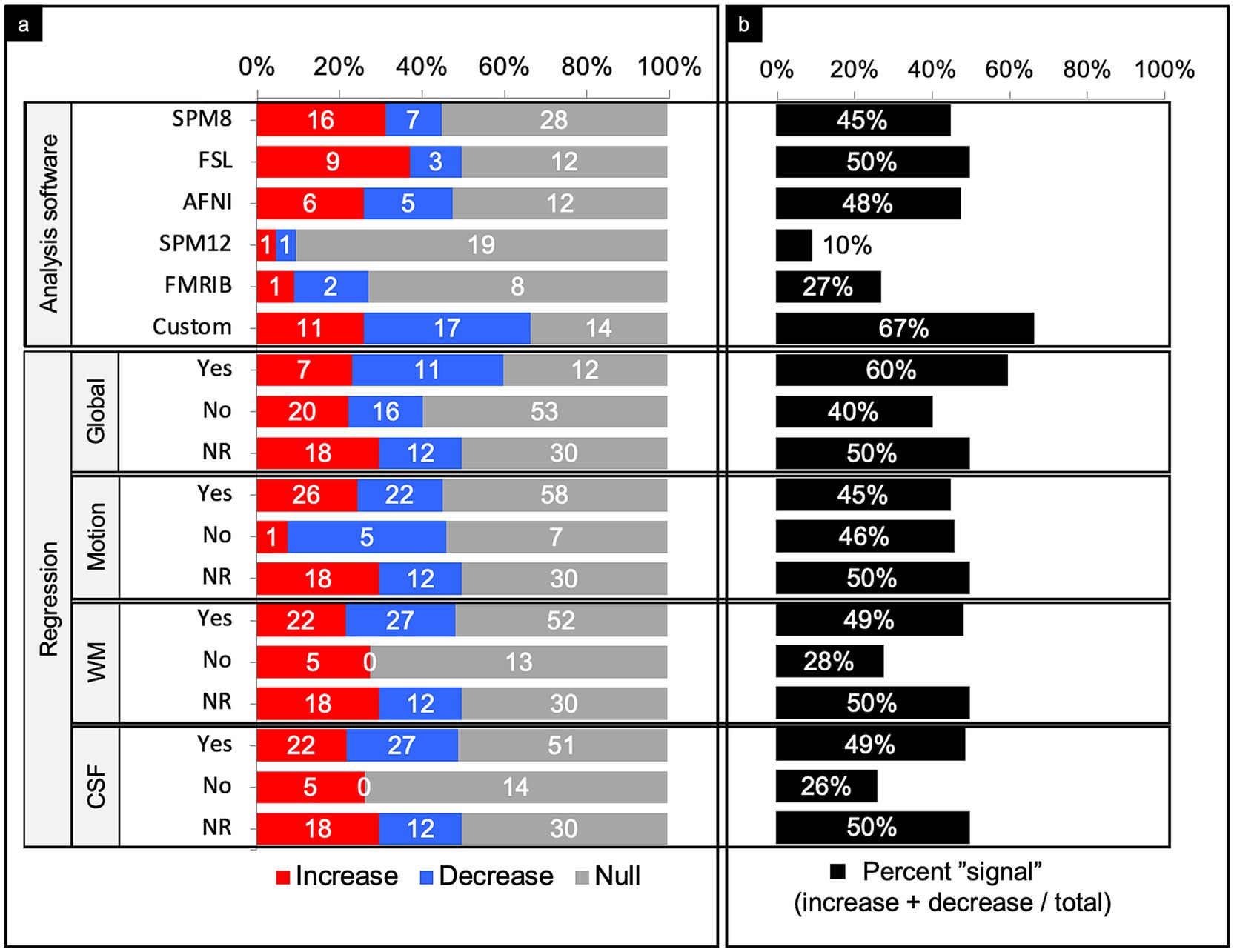
Figure 8. Connectivity comparisons stratified by image analysis. Comparisons were summed across study, networks, and injury characteristics, then stratified by image analysis to display the proportion of increased, decreased, or no (i.e., null) connectivity difference between TBI and control populations and (a) and the percent signal of having any significant difference (b). NR, not reported; WM, white matter; CSF, cerebrospinal fluid.
SPM8 yielded a percent signal of 45%, with nearly 70% of comparisons with connectivity differences showing increased connectivity. In contrast, SPM12 yielded a percent signal of just ~10%. FSL and AFNI both yielded a percent signal of around 50%. Of the significant differences in studies that used FSL, 75% detected an increase in connectivity. Of the significant differences in studies that used AFNI, there was a near even split of increased and decreased connectivity. Studies that used mixed methods or custom pipelines yielded the highest percent signal of 67%.
Signal regression
Of the 179 comparisons, the authors only reported whether they performed signal regression in 119 comparisons (66%) (Figure 8). Overall, performing a signal regression in most categories, other than motion, revealed an increased sensitivity for finding a significant difference in connectivity between people with TBI and matched controls. Among those that performed white matter and cerebrospinal fluid (CSF) regression, percent signal was notably higher than those who did not perform these regression methods. Of those that did not perform white matter or CSF regression, all comparisons that yielded a signal showed increased connectivity. It should be noted that nearly every study with the exception of 1 utilized white matter and CSF regression in tandem, and thus had roughly equal distributions.
Discussion
Traumatic Brain Injury (TBI) can cause both focal and diffuse brain changes. Whereas standard clinical neuroimaging identifies structural pathology, it does not reveal functional alterations that may underlie poorer outcomes. Resting-state fMRI has been used to detect functional alterations in network architecture unique to TBI, offering potential insights where other imaging methods have not. However, despite its growing use, there is no clear consensus on functional connectivity (FC) differences between TBI and control populations. To address this gap, we performed a systematic review to identify driving factors that may explain the heterogeneity when comparing FC between TBI and control populations. Specifically, we combined and stratified findings by injury severity, age, chronicity, as well as the studied population type, network, and imaging methods across 50 studies that performed 179 comparisons in 1,397 TBI patients and 1,179 matched controls.
The most consistent findings were a trend toward increased connectivity in chronic stages of TBI and those with more severe head injuries, independent of the other variables (Figure 5). Increased connectivity may reflect compensatory processes that are only needed with more severe or chronic injury states (37–39). We also found a handful of trends when examining individual networks collapsed across all other factors. Otherwise, we found little consensus in the direction or significance of connectivity differences. Based on our findings, we provide recommendations for future work in efforts to standardized rsfMRI imaging in TBI and lead the field toward more reproducible results.
Collapsing across all variables
When collapsing across all networks, injury characteristics, patient demographics, and imaging techniques (Figure 4b, “Total” row), the findings were generally mixed. There were about an equal number of comparisons showing a significant difference versus no difference in connectivity (47% signal) between patients with TBI and matched controls. Of the significant differences, again, the findings were fairly mixed, but with slight predominance of increased connectivity (45 increased and 39 decreased).
Severity
Parsing the comparisons by severity revealed that patients with severe and moderate/severe TBI were more likely to show increased (67%) over decreased connectivity (33%) as compared to controls, whereas patients with mild TBI showed a 50/50 split between increased and decreased connectivity as compared to controls (Figure 5). It may be that higher severity injuries disrupt more axonal connections, warranting compensatory processes (37–39), such as cortical reorganization, neurogenesis, axonal sprouting, and angiogenesis, which have been demonstrated in animal models (40, 41).
Surprisingly, however, studies show a similar likelihood of finding any significant difference in FC whether in mild TBI (52%) or moderate/severe TBI combined (56%) as compared to controls (Figure 5). Despite the obvious difference in structural and neurological consequences of injury between these TBI severities, the differences in FC as compared to controls is not as apparent. Studies directly comparing FC in patients across TBI severities are lacking.
Chronicity
Parsing the comparisons by chronicity revealed a cross-sectional trend of increased connectivity by time since injury. Because we dealt with longitudinal studies as discrete, between-group comparisons of FC at acute, subacute, or chronic times since injury, our descriptive findings do not enable conclusions about within group changes in FC over time. Nevertheless, cross-sectionally, patients with TBI who were imaged in the acute and acute/subacute window showed less increased (42%) than decreased connectivity (58%) as compared to controls (Figure 5). From the subacute to chronic window however, the differences flipped to more increased (60%) than decreased connectivity (40%) as compared to controls. Though these results are cross-sectional, this trend suggests connectivity may increase over time post-injury.
Several of the studies included in our review contained longitudinal data, the majority of which report a relative within-group increase in connectivity at the chronic as compared to the more acute time points post-injury (21, 42, 43). Studies that did not find increased connectivity in the chronic time points showed no difference as a function of time since injury (44, 45) or revealed more complex findings, such as increased and decreased connectivity within a single network as a function of time since injury (e.g., increased connectivity at the left anterior prefrontal cortex, but decreased connectivity at the middle cingulate gyrus) (46). Most notably, however, no longitudinal study reported a whole-network decrease in connectivity in the chronic stage. Studies utilizing graph theory have also noted a pattern of initially decreased to subsequently increased connectivity over time since injury (15, 47, 48). Increased connectivity has correlated with better function (42) and worse function (49) demonstrating again that the direction of connectivity change or difference compared to control may not be as important as the finding of any change or difference. These longitudinal effects have been interpreted as adaptive or compensatory when relating to improved function (44, 50, 51).
Age
Parsing the comparisons by age did not reveal any discernible pattern. Trends based on age alone (adolescent vs. adult) are difficult to draw given the large majority (90%) of studies included only adults. Furthermore, adult age ranges were restricted, with the first quartile less than 26.88 years and the fourth quartile greater than 38.49 years. Because most of the TBI patients were scanned in early adulthood, we cannot infer whether connectivity patterns emerge after injury in younger and older ages due to the paucity of studies overall, and in our study in particular, for these age groups.
Population type
Parsing comparisons by population type revealed a notably larger percent signal (71%) in the military population. Overall, however, the military population showed an even split of increased and decreased connectivity findings, which is consistent with the other populations studied. The military population was also the least studied (7.8% of total comparisons). Because injury in military personnel can include unique mechanisms and are often comorbid with sequelae of psychological trauma as compared to civilian and sport-related injuries (52), it is possible that the brain differences are more striking.
Networks
The DMN was the most studied network (78 comparisons) and had the highest signal of any of the networks included (55% signal). The ratio of increased to decreased connectivity between people with TBI and matched controls was, however, roughly equal (23–20, respectively). It is well known that the DMN plays a critical role in intrinsic cognitive processes. Though the DMN generally had mixed results across most patient characteristics, it trended toward increased connectivity in the moderate/severe TBI population and at the subacute time frame (Figure 6b, column 1). Previous studies have interpreted this increased connectivity as a compensatory or adaptive mechanism because of its association with positive cognitive outcomes (44, 50, 51). It is possible compensatory processes do not readily occur unless the injury is sufficiently damaging. Perhaps such compensation does not occur until at least weeks to months after the injury, which may follow periods of increased metabolic activity (53, 54).
The DAN showed the lowest signal (14%) of all the networks, suggesting this network may not be affected by TBI and/or whatever changes that may occur are not sensitive to rsfMRI methods. Of note, the DAN also had the lowest number of total comparisons across the literature (Figure 4). Further investigation of the DAN may be required to rule out the DAN as a potential marker of TBI. Alternatively, this may be a network to leave out of analyses to retain valuable degrees of freedom.
The SN had the next lowest percent signal (39%) (Figure 4). Given the putative role of the SN in affective processes (55, 56), including affective dysregulation in some patients with TBI (57), its low signal is surprising. However, because the SN serves as a switch between intrinsic and extrinsic cognitive processes (58), rsfMRI may not be sensitive enough to capture changes in connectivity without patients performing goal-directed activity or without looking at internetwork connectivity differences. Again, further investigation of this network at rest and during tasks will provide greater insight to its sensitivity as a potential biomarker of TBI.
Methodological factors
Parsing the comparisons by whether patients were instructed to keep their eyes open, fixated, or closed revealed a striking pattern. Eyes open or fixated showed 75 and 64% signal, respectively, whereas eyes closed only revealed 33% signal (Figure 7). Interestingly, about half of the studies instructed participants to close their eyes, despite newer evidence that eyes open may enhance connectivity strengths (59).
Generating quartiles of the number of volumes captured during scans revealed a notably higher percent signal among the third quartile (73%), which had a range of 182–245 volumes per scan (Figure 7). In contrast, all other quartiles had a markedly lower percent signal (<48%). These results suggest the number of volumes captured during scans may have an ideal value that could serve to increase the sensitivity of rsfMRI in detecting connectivity differences.
Generating quartiles of the scan duration revealed a notably lower percent signal among the second quartile (37%), which had a range of 315–360 s (Figure 7). In contrast, the first, third, and fourth quartiles all demonstrated markedly higher percent signals. Recent studies support greater reliability with increasing scan length, though that cutoff point is debated and not well defined (60, 61).
Other than GE, which was rarely used, scanner manufacturer and coil channel did not meaningfully impact the findings in that there were similar proportions of increased, decreased, and null findings no matter which scanner or head coil was used (Figure 7). GE did demonstrate a percent signal of 77% suggesting more likely to show some difference across groups as compared to null but only four studies utilized GE scanners, which is not enough to make a strong conclusion. However, the fact that the other two most commonly used scanner manufacturers did not appreciably impact the findings suggests that this pattern of findings are likely generalizable and could be expected to hold across sites in future research.
Creating tertiles of the slice thickness demonstrated a substantially higher percent signal among the third tertile, which had a thickness of >4 mm per slice (Figure 7). The choice in slice thickness is determined by the time to acquire a set brain volume, spatial resolution, minimization of signal dropout, and BOLD signal-to-noise (SNR) ratio, which largely determines the statistical power to detect nodal activation. Therefore, any increase in spatial resolution with thinner slices will be offset by a reduction in the statistical significance due to increased SNR (62–64). Prior work demonstrates that thicker slices will yield more low-percentage signal changes, consistent with our results (63). However, the optimal slice thickness depends on the study, as certain brain regions may require higher resolution, which may come at the cost of sensitivity per unit time, to enhance localization and reduce signal dropout.
When evaluating preprocessing software as a factor, custom pipelines achieved a notably higher signal than the other softwares (Figure 8). It may be that custom pipelines are designed for the specific dataset obtained, and thus software can be designed to achieve higher sensitivity in detecting connectivity differences. However, because this study did not have access to the custom softwares, there is the possibility that a higher signal could result from poorly implemented pipelines that are not as rigorous at mitigating specious findings.
Parsing the comparisons by regression type revealed performing regression in all categories (global, white matter, CSF), except motion, increased the likelihood of detecting a significant difference between TBI and control populations (Figure 8). Because percent signal was similar between comparisons that did and did not perform motion regression, it appears that motion regression does not significantly affect sensitivity of finding a difference in connectivity in these studies.
Limitations
It is impossible to account for all the factors that contribute to the heterogeneity in neuroimaging of TBI. This study aimed to isolate individual variables across 50 studies, but it is important to acknowledge that many of these variables may influence one another (e.g., most moderate/severe TBI cases were studied in the chronic phase). MRI sequence parameters vary between sites, evolve over time, and may interact in ways that impact results. Inconsistent reporting of certain parameters (e.g., acquisition direction, reconstruction plane, slice thickness), made it difficult to extract and parse our findings by an exhaustive list of sequence parameters. Nevertheless, we extracted the parameters we found to be most consistently reported and that previous work suggests may impact results. Therefore, careful consideration should be taken when interpreting individual variables as interactions may confound results.
Given discrepancies in the number of comparisons within categories (e.g., majority of comparisons in mild TBI, middle age range, civilian population, default mode network), we caution making strong conclusions for variables that received less attention (e.g., much less comparisons in more severe injuries, younger and older ages, dorsal attention network). This is a limitation of our study, but also reflective of the current assumptions for what might be most biologically or clinically important in this field.
Given the large quantity of results generated in rsfMRI research, there exists a severe underreporting of null findings throughout the literature. However, reported results would be unreadable if the entirety of results were explicitly reported. We chose not to infer null findings when primary authors did not clearly report null findings as interpretation would be prone to inconsistency. This aspect of rsfMRI literature makes it difficult to gather an accurate representation of percent signals across the literature, and thus our percent signals are likely skewed toward higher values.
Recommendations for future studies
As discussed throughout this review, there are multiple factors that may contribute to the heterogeneity of rsfMRI connectivity patterns in TBI. Parsing the findings by these factors, however, did not reveal a dramatic consensus, which strongly supports the need for consistency and best practices across studies. Below, we make several recommendations to establish greater consistency and reproducibility for future studies. We also identify areas of study where literature is lacking and therefore encourage greater investigation.
1. Networks: The low percent signal observed in the DAN and SN suggest they may not be affected by TBI and/or are not sensitive to rsfMRI methods. We recommend that future studies pay particular attention to the DAN and SN to provide greater insight into their sensitivity. However, their low sensitivity would make it reasonable to exclude these networks from analysis to preserve degrees of freedom.
2. Demographics: Functional connectivity in TBI may be affected by multiple demographic factors, such as severity, chronicity, age, and population or mechanism of TBI. We encourage future studies to either narrow their demographic inclusion criteria to evaluate subgroups within heterogeneous populations or obtain large enough sample sizes to account for the heterogeneity. Some of these challenges are seemingly insurmountable. However, in the event that sample size is limited or heterogeneous, combining datasets across sites or with freely downloadable datasets might provide the power needed for more reliable analyses through techniques such as harmonization and meta-analysis. ENIGMA (65) and FITBIR (66) are both viable solutions for this challenge.
3. Age: Age range was restricted to early-middle adulthood across the studies, and thus the current literature has yet to evaluate changes in connectivity across child and elderly populations. We encourage future studies to focus on these populations that may be potentially more vulnerable.
4. Injury mechanism: Restricting studies to a specific injury mechanism, rather than population type, may help to reduce variability. For example, studies could focus on car accidents, blast exposure, or sport-related injuries. Thus, studies should narrow their inclusion criteria to obtain a more focused assessment of mechanisms’ role in functional alterations.
5. Longitudinality: The vast majority of studies are cross-sectional rather than longitudinal. Adding multiple imaging time points to study designs will effectively increase power, make it possible to derive changes over time, and reduce natural variability in rsfMRI patterns.
6. Canonical network: We recommend future studies classify results to an established canonical network to facilitate interpretation. It is critical for replication that authors clearly indicate how regions of interest or networks were derived and what expected differences they hypothesize.
7. Methodology: We recommend future studies utilize fixated or open eye scans as well as volume ranges between 182 and 245 volumes given their relatively higher sensitivity in signal detection. Though scan duration had mixed findings, percent signal was consistently higher among longer scan durations. In line with previous literature, we also recommend utilizing scan durations of at least 360 s.
8. Regression: All forms of regression, except motion regression, yielded higher sensitivity than studies that did not perform regression. In any case, we recommend all studies clearly identify whether they performed global, CSF, white matter, and motion regression as this ensures future studies can either replicate or refute the findings in independent samples.
9. Preprocessing software: Though recommendations on specific software is beyond the scope of this paper, FSL and SPM8 had the highest sensitivity in detecting a signal. Studies utilizing custom software are encouraged to also process their data with established software to compare and confirm results.
Conclusion
Despite the obvious potential for neuroimaging as a biomarker in TBI, there seems to be no clear set of rsfMRI findings that universally differentiate the injured from healthy brain across all injury severities, mechanisms, chronicities, and population types. Although it is reasonable to hypothesize the heterogeneity in TBI populations studied drives the heterogeneity of findings, it is unclear from our study that any one factor (e.g., age, chronicity, population type, etc.) was a strong predictor of connectivity trends. Our study confirms that rsfMRI literature is largely mixed when considering connectivity differences between brain injured and healthy individuals no matter how the data is parsed. We identified a few patterns that may reflect mechanisms of brain injury or recovery, but ultimately our findings highlight the need for multi-site and longitudinal studies, greater methodological consensus across studies, and/or consortia to combine data retrospectively using the same analysis methods. These findings will guide future research and should help clinicians understand the early state of advanced imaging modalities, like rsfMRI, which are not yet ready for clinical use in individual patients.
Data availability statement
The original contributions presented in the study are included in the article/supplementary material, further inquiries can be directed to the corresponding author.
Author contributions
AK: Conceptualization, Data curation, Formal analysis, Investigation, Methodology, Project administration, Resources, Supervision, Validation, Visualization, Writing – original draft, Writing – review & editing. DF: Conceptualization, Data curation, Formal analysis, Investigation, Methodology, Software, Validation, Visualization, Writing – original draft, Writing – review & editing. KK: Conceptualization, Data curation, Formal analysis, Investigation, Validation, Writing – original draft, Writing – review & editing. CP: Data curation, Formal analysis, Investigation, Methodology, Validation, Visualization, Writing – review & editing. HH: Conceptualization, Data curation, Investigation, Methodology, Writing – review & editing. JF: Conceptualization, Data curation, Investigation, Methodology, Writing – review & editing. PR: Conceptualization, Project administration, Supervision, Writing – review & editing. MB: Conceptualization, Project administration, Supervision, Writing – review & editing. CS: Conceptualization, Project administration, Supervision, Writing – review & editing. KB: Conceptualization, Data curation, Formal analysis, Funding acquisition, Investigation, Methodology, Project administration, Resources, Supervision, Validation, Visualization, Writing – original draft, Writing – review & editing.
Funding
The author(s) declare that no financial support was received for the research, authorship, and/or publication of this article.
Conflict of interest
The authors declare that the research was conducted in the absence of any commercial or financial relationships that could be construed as a potential conflict of interest.
Publisher’s note
All claims expressed in this article are solely those of the authors and do not necessarily represent those of their affiliated organizations, or those of the publisher, the editors and the reviewers. Any product that may be evaluated in this article, or claim that may be made by its manufacturer, is not guaranteed or endorsed by the publisher.
Footnotes
References
1. Capizzi, A, Woo, J, and Verduzco-Gutierrez, M. Traumatic brain injury. Med Clin North Am. (2020) 104:213–38. doi: 10.1016/j.mcna.2019.11.001
2. Chong, CD, and Schwedt, TJ. Research imaging of brain structure and function after concussion. Headache. (2018) 58:827–35. doi: 10.1111/head.13269
3. Schwedt, TJ . Structural and functional brain alterations in post-traumatic headache attributed to mild traumatic brain injury: a narrative review. Front Neurol. (2019) 10:615. doi: 10.3389/fneur.2019.00615
4. Bischof, GN, and Cross, DJ. Brain trauma imaging. J Nucl Med. (2023) 64:20–9. doi: 10.2967/jnumed.121.263293
5. Fox, MD, and Greicius, M. Clinical applications of resting state functional connectivity. Front Syst Neurosci. (2010) 4:19. doi: 10.3389/fnsys.2010.00019
6. Buxton, RB, Uludağ, K, Dubowitz, DJ, and Liu, TT. Modeling the hemodynamic response to brain activation. NeuroImage. (2004) 23:S220–33. doi: 10.1016/j.neuroimage.2004.07.013
7. Fox, MD, and Raichle, ME. Spontaneous fluctuations in brain activity observed with functional magnetic resonance imaging. Nat Rev Neurosci. (2007) 8:700–11. doi: 10.1038/nrn2201
8. Buxton, RB . The physics of functional magnetic resonance imaging (fMRI). Rep Prog Phys. (2013) 76:096601. doi: 10.1088/0034-4885/76/9/096601
9. Deco, G, Jirsa, VK, and McIntosh, AR. Emerging concepts for the dynamical organization of resting-state activity in the brain. Nat Rev Neurosci. (2011) 12:43–56. doi: 10.1038/nrn2961
10. Li, K, Guo, L, Nie, J, Li, G, and Liu, T. Review of methods for functional brain connectivity detection using fMRI. Comput Med Imaging Graph. (2009) 33:131–9. doi: 10.1016/j.compmedimag.2008.10.011
11. Lee, MH, Smyser, CD, and Shimony, JS. Resting-state fMRI: a review of methods and clinical applications. AJNR Am J Neuroradiol. (2013) 34:1866–72. doi: 10.3174/ajnr.A3263
12. Sharp, DJ, and Ham, TE. Investigating white matter injury after mild traumatic brain injury. Curr Opin Neurol. (2011) 24:558–63. doi: 10.1097/WCO.0b013e32834cd523
13. Puig, J, Ellis, MJ, Kornelsen, J, Figley, TD, Figley, CR, Daunis-i-Estadella, P, et al. Magnetic resonance imaging biomarkers of brain connectivity in predicting outcome after mild traumatic brain injury: a systematic review. J Neurotrauma. (2020) 37:1761–76. doi: 10.1089/neu.2019.6623
14. Ofoghi, Z, Dewey, D, and Barlow, KM. A systematic review of structural and functional imaging correlates of headache or pain after mild traumatic brain injury. J Neurotrauma. (2020) 37:907–23. doi: 10.1089/neu.2019.6750
15. Caeyenberghs, K, Verhelst, H, Clemente, A, and Wilson, PH. Mapping the functional connectome in traumatic brain injury: what can graph metrics tell us? NeuroImage. (2017) 160:113–23. doi: 10.1016/j.neuroimage.2016.12.003
16. Hayes, JP, Bigler, ED, and Verfaellie, M. Traumatic brain injury as a disorder of brain connectivity. J Int Neuropsychol Soc. (2016) 22:120–37. doi: 10.1017/S1355617715000740
17. Eierud, C, Craddock, RC, Fletcher, S, Aulakh, M, King-Casas, B, Kuehl, D, et al. Neuroimaging after mild traumatic brain injury: review and meta-analysis. Neuroimage Clin. (2014) 4:283–94. doi: 10.1016/j.nicl.2013.12.009
18. Hallquist, MN, and Hillary, FG. Graph theory approaches to functional network organization in brain disorders: a critique for a brave new small-world. Netw Neurosci. (2019) 3:1–26. doi: 10.1162/netn_a_00054
19. Hannawi, Y, Lindquist, MA, Caffo, BS, Sair, HI, and Stevens, RD. Resting brain activity in disorders of consciousness: a systematic review and meta-analysis. Neurology. (2015) 84:1272–80. doi: 10.1212/WNL.0000000000001404
20. Verhulst, MMLH, Glimmerveen, AB, van Heugten, CM, Helmich, RCG, and Hofmeijer, J. MRI factors associated with cognitive functioning after acute onset brain injury: systematic review and meta-analysis. Neuroimage Clin. (2023) 38:103415. doi: 10.1016/j.nicl.2023.103415
21. Venkatesan, UM, Dennis, NA, and Hillary, FG. Chronology and chronicity of altered resting-state functional connectivity after traumatic brain injury. J Neurotrauma. (2015) 32:252–64. doi: 10.1089/neu.2013.3318
22. Morelli, N, Johnson, NF, Kaiser, K, Andreatta, RD, Heebner, NR, and Hoch, MC. Resting state functional connectivity responses post-mild traumatic brain injury: a systematic review. Brain Inj. (2021) 35:1326–37. doi: 10.1080/02699052.2021.1972339
23. Rabinowitz, AR, and Levin, HS. Cognitive sequelae of traumatic brain injury. Psychiatr Clin North Am. (2014) 37:1–11. doi: 10.1016/j.psc.2013.11.004
24. Marquez de la Plata, CD, Hart, T, Hammond, FM, Frol, AB, Hudak, A, Harper, CR, et al. Impact of age on long-term recovery from traumatic brain injury. Arch Phys Med Rehabil. (2008) 89:896–903. doi: 10.1016/j.apmr.2007.12.030
25. Røe, C, Sveen, U, Alvsåker, K, and Bautz-Holter, E. Post-concussion symptoms after mild traumatic brain injury: influence of demographic factors and injury severity in a 1-year cohort study. Disabil Rehabil. (2009) 31:1235–43. doi: 10.1080/09638280802532720
26. Stocchetti, N, and Zanier, ER. Chronic impact of traumatic brain injury on outcome and quality of life: a narrative review. Crit Care. (2016) 20:148. doi: 10.1186/s13054-016-1318-1
27. Kim, SY, Yeh, PH, Ollinger, JM, Morris, HD, Hood, MN, Ho, VB, et al. Military-related mild traumatic brain injury: clinical characteristics, advanced neuroimaging, and molecular mechanisms. Transl Psychiatry. (2023) 13:289. doi: 10.1038/s41398-023-02569-1
28. Yeo, BTT, Krienen, FM, Chee, MWL, and Buckner, RL. Estimates of segregation and overlap of functional connectivity networks in the human cerebral cortex. NeuroImage. (2014) 88:212–27. doi: 10.1016/j.neuroimage.2013.10.046
29. Page, MJ, McKenzie, JE, Bossuyt, PM, Boutron, I, Hoffmann, TC, Mulrow, CD, et al. The PRISMA 2020 statement: an updated guideline for reporting systematic reviews. Rev Esp Cardiol. (2021) 74:790–9. doi: 10.1016/j.recesp.2021.06.016
30. Schaefer, A, Kong, R, Gordon, EM, Laumann, TO, Zuo, XN, Holmes, AJ, et al. Local-global parcellation of the human cerebral cortex from intrinsic functional connectivity MRI. Cereb Cortex. (2018) 28:3095–114. doi: 10.1093/cercor/bhx179
31. Thomas Yeo, BT, Krienen, FM, Sepulcre, J, Sabuncu, MR, Lashkari, D, Hollinshead, M, et al. The organization of the human cerebral cortex estimated by intrinsic functional connectivity. J Neurophysiol. (2011) 106:1125–65. doi: 10.1152/jn.00338.2011
32. Teasdale, G, and Jennett, B. Assessment of coma and impaired consciousness. Lancet. (1974) 304:81–4. doi: 10.1016/S0140-6736(74)91639-0
33. Teasdale, G, Maas, A, Lecky, F, Manley, G, Stocchetti, N, and Murray, G. The Glasgow coma scale at 40 years: standing the test of time. Lancet Neurol. (2014) 13:844–54. doi: 10.1016/S1474-4422(14)70120-6
34. Wang, KK, Yang, Z, Zhu, T, Shi, Y, Rubenstein, R, Tyndall, JA, et al. An update on diagnostic and prognostic biomarkers for traumatic brain injury. Expert Rev Mol Diagn. (2018) 18:165–80. doi: 10.1080/14737159.2018.1428089
35. Hossain, I, Marklund, N, Czeiter, E, Hutchinson, P, and Buki, A. Blood biomarkers for traumatic brain injury: a narrative review of current evidence. Brain Spine. (2024) 4:102735. doi: 10.1016/j.bas.2023.102735
36. Boake, C, McCauley, SR, Levin, HS, Pedroza, C, Contant, CF, Song, JX, et al. Diagnostic criteria for postconcussional syndrome after mild to moderate traumatic brain injury. J Neuropsychiatry Clin Neurosci. (2005) 17:350–6. doi: 10.1176/jnp.17.3.350
37. Sharp, DJ, Scott, G, and Leech, R. Network dysfunction after traumatic brain injury. Nat Rev Neurol. (2014) 10:156–66. doi: 10.1038/nrneurol.2014.15
38. Kinnunen, KM, Greenwood, R, Powell, JH, Leech, R, Hawkins, PC, Bonnelle, V, et al. White matter damage and cognitive impairment after traumatic brain injury. Brain. (2011) 134:449–63. doi: 10.1093/brain/awq347
39. Smith, DH, Meaney, DF, and Shull, WH. Diffuse axonal injury in head trauma. J Head Trauma Rehabil. (2003) 18:307–16. doi: 10.1097/00001199-200307000-00003
40. Nudo, RJ . Recovery after brain injury: mechanisms and principles. Front Hum Neurosci. (2013) 7:887. doi: 10.3389/fnhum.2013.00887
41. Hylin, MJ, Kerr, AL, and Holden, R. Understanding the mechanisms of recovery and/or compensation following injury. Neural Plast. (2017) 2017:7125057. doi: 10.1155/2017/7125057
42. Manning, KY, Schranz, A, Bartha, R, Dekaban, GA, Barreira, C, Brown, A, et al. Multiparametric MRI changes persist beyond recovery in concussed adolescent hockey players. Neurology. (2017) 89:2157–66. doi: 10.1212/WNL.0000000000004669
43. Sours, C, Zhuo, J, Roys, S, Shanmuganathan, K, and Gullapalli, RP. Disruptions in resting state functional connectivity and cerebral blood flow in mild traumatic brain injury patients. PLoS One. (2015) 10:e0134019. doi: 10.1371/journal.pone.0134019
44. Mayer, AR, Mannell, MV, Ling, J, Gasparovic, C, and Yeo, RA. Functional connectivity in mild traumatic brain injury. Hum Brain Mapp. (2011) 32:1825–35. doi: 10.1002/hbm.21151
45. van der Horn, HJ, Scheenen, ME, de Koning, ME, Liemburg, EJ, Spikman, JM, and van der Naalt, J. The default mode network as a biomarker of persistent complaints after mild traumatic brain injury: a longitudinal functional magnetic resonance imaging study. J Neurotrauma. (2017) 34:3262–9. doi: 10.1089/neu.2017.5185
46. Murdaugh, DL, King, TZ, Sun, B, Jones, RA, Ono, KE, Reisner, A, et al. Longitudinal changes in resting state connectivity and white matter integrity in adolescents with sports-related concussion. J Int Neuropsychol Soc. (2018) 24:781–92. doi: 10.1017/S1355617718000413
47. Hillary, FG . Neuroimaging of working memory dysfunction and the dilemma with brain reorganization hypotheses. J Int Neuropsychol Soc. (2008) 14:526–34. doi: 10.1017/S1355617708080788
48. Hillary, FG, Roman, CA, Venkatesan, U, Rajtmajer, SM, Bajo, R, and Castellanos, ND. Hyperconnectivity is a fundamental response to neurological disruption. Neuropsychology. (2015) 29:59–75. doi: 10.1037/neu0000110
49. Bickart, KC, Olsen, A, Dennis, EL, Babikian, T, Hoffman, AN, Snyder, A, et al. Frontoamygdala hyperconnectivity predicts affective dysregulation in adolescent moderate-severe TBI. Front Rehabil Sci. (2023) 3:1064215. doi: 10.3389/fresc.2022.1064215
50. Palacios, EM, Sala-Llonch, R, Junque, C, Roig, T, Tormos, JM, Bargallo, N, et al. Resting-state functional magnetic resonance imaging activity and connectivity and cognitive outcome in traumatic brain injury. JAMA Neurol. (2013) 70:845–51. doi: 10.1001/jamaneurol.2013.38
51. Bonnelle, V, Leech, R, Kinnunen, KM, Ham, TE, Beckmann, CF, de Boissezon, X, et al. Default mode network connectivity predicts sustained attention deficits after traumatic brain injury. J Neurosci. (2011) 31:13442–51. doi: 10.1523/JNEUROSCI.1163-11.2011
52. Chapman, JC, and Diaz-Arrastia, R. Military traumatic brain injury: a review. Alzheimers Dement. (2014) 10:S97–S104. doi: 10.1016/j.jalz.2014.04.012
53. Giza, CC, and Hovda, DA. The new neurometabolic cascade of concussion. Neurosurgery. (2014) 75:S24–33. doi: 10.1227/NEU.0000000000000505
54. Hillary, FG, and Grafman, JH. Injured brains and adaptive networks: the benefits and costs of Hyperconnectivity. Trends Cogn Sci. (2017) 21:385–401. doi: 10.1016/j.tics.2017.03.003
55. Schimmelpfennig, J, Topczewski, J, Zajkowski, W, and Jankowiak-Siuda, K. The role of the salience network in cognitive and affective deficits. Front Hum Neurosci. (2023) 17:1133367. doi: 10.3389/fnhum.2023.1133367
56. Uddin, LQ . Salience processing and insular cortical function and dysfunction. Nat Rev Neurosci. (2015) 16:55–61. doi: 10.1038/nrn3857
57. Weis, CN, Webb, EK, deRoon-Cassini, TA, and Larson, CL. Emotion dysregulation following trauma: shared neurocircuitry of traumatic brain injury and trauma-related psychiatric disorders. Biol Psychiatry. (2022) 91:470–7. doi: 10.1016/j.biopsych.2021.07.023
58. Seeley, WW, Menon, V, Schatzberg, AF, Keller, J, Glover, GH, Kenna, H, et al. Dissociable intrinsic connectivity networks for salience processing and executive control. J Neurosci. (2007) 27:2349–56. doi: 10.1523/JNEUROSCI.5587-06.2007
59. van Dijk, KRA, Hedden, T, Venkataraman, A, Evans, KC, Lazar, SW, and Buckner, RL. Intrinsic functional connectivity as a tool for human connectomics: theory, properties, and optimization. J Neurophysiol. (2010) 103:297–321. doi: 10.1152/jn.00783.2009
60. Birn, RM, Molloy, EK, Patriat, R, Parker, T, Meier, TB, Kirk, GR, et al. The effect of scan length on the reliability of resting-state fMRI connectivity estimates. NeuroImage. (2013) 83:550–8. doi: 10.1016/j.neuroimage.2013.05.099
61. Anderson, JS, Ferguson, MA, Lopez-Larson, M, and Yurgelun-Todd, D. Reproducibility of single-subject functional connectivity measurements. AJNR Am J Neuroradiol. (2011) 32:548–55. doi: 10.3174/ajnr.A2330
62. Frahm, J, Merboldt, KD, and Hänicke, W. Functional MRI of human brain activation at high spatial resolution. Magn Reson Med. (1993) 29:139–44. doi: 10.1002/mrm.1910290126
63. Howseman, AM, Grootoonk, S, Porter, DA, Ramdeen, J, Holmes, AP, and Turner, R. The effect of slice order and thickness on fMRI activation data using multislice echo-planar imaging. NeuroImage. (1999) 9:363–76. doi: 10.1006/nimg.1998.0418
64. Paradis, AL, Van de Moortele, PF, Le Bihan, D, and Poline, JB. Slice acquisition order and blood oxygenation level dependent frequency content: an event-related functional magnetic resonance imaging study. MAGMA. (2001) 13:91–100. doi: 10.1007/BF02668157
65. Caeyenberghs, K, Imms, P, Irimia, A, Monti, MM, Esopenko, C, de Souza, NL, et al. ENIGMA's simple seven: recommendations to enhance the reproducibility of resting-state fMRI in traumatic brain injury. NeuroImage Clin. (2024) 42:103585. doi: 10.1016/j.nicl.2024.103585
66. van Horn, J, and Gazzaniga, MS. Why share data? Lessons learned from the fMRIDC. NeuroImage. (2013) 82:677–82. doi: 10.1016/j.neuroimage.2012.11.010
67. Arenivas, A, Diaz-Arrastia, R, Spence, J, Cullum, CM, Krishnan, K, Bosworth, C, et al. Three approaches to investigating functional compromise to the default mode network after traumatic axonal injury. Brain Imaging Behav. (2014) 8:407–19. doi: 10.1007/s11682-012-9191-2
68. Clough, M, Mutimer, S, Wright, DK, Tsang, A, Costello, DM, Gardner, AJ, et al. Oculomotor cognitive control abnormalities in Australian rules football players with a history of concussion. J Neurotrauma. (2018) 35:730–8. doi: 10.1089/neu.2017.5204
69. Dailey, NS, Smith, R, Vanuk, JR, Raikes, AC, and Killgore, WDS. Resting-state functional connectivity as a biomarker of aggression in mild traumatic brain injury. Neuroreport. (2018) 29:1413–7. doi: 10.1097/WNR.0000000000001127
70. de Simoni, S, Grover, PJ, Jenkins, PO, Honeyfield, L, Quest, RA, Ross, E, et al. Disconnection between the default mode network and medial temporal lobes in post-traumatic amnesia. Brain. (2016) 139:3137–50. doi: 10.1093/brain/aww241
71. Dretsch, MN, Rangaprakash, D, Katz, JS, Daniel, TA, Goodman, AM, Denney, TS, et al. Strength and temporal variance of the default mode network to investigate chronic mild traumatic brain injury in service members with psychological trauma. J Exp Neurosci. (2019) 13:1179069519833966. doi: 10.1177/1179069519833966
72. Grossner, EC, Bernier, RA, Brenner, EK, Chiou, KS, Hong, J, and Hillary, FG. Enhanced default mode connectivity predicts metacognitive accuracy in traumatic brain injury. Neuropsychology. (2019) 33:922–33. doi: 10.1037/neu0000559
73. Guo, H, Liu, R, Sun, Z, Liu, B, Xiang, Y, Mao, J, et al. Evaluation of prognosis in patients with severe traumatic brain injury using resting-state functional magnetic resonance imaging. World Neurosurg. (2019) 121:e630–9. doi: 10.1016/j.wneu.2018.09.178
74. Han, K, Chapman, SB, and Krawczyk, DC. Disrupted intrinsic connectivity among default, dorsal attention, and frontoparietal control networks in individuals with chronic traumatic brain injury. J Int Neuropsychol Soc. (2016) 22:263–79. doi: 10.1017/S1355617715001393
75. Hou, W, Sours Rhodes, C, Jiang, L, Roys, S, Zhuo, J, JaJa, J, et al. Dynamic functional network analysis in mild traumatic brain injury. Brain Connect. (2019) 9:475–87. doi: 10.1089/brain.2018.0629
76. Iraji, A, Benson, RR, Welch, RD, O'Neil, BJ, Woodard, JL, Ayaz, SI, et al. Resting state functional connectivity in mild traumatic brain injury at the acute stage: independent component and seed-based analyses. J Neurotrauma. (2015) 32:1031–45. doi: 10.1089/neu.2014.3610
77. Lancaster, K, Venkatesan, UM, Lengenfelder, J, and Genova, HM. Default mode network connectivity predicts emotion recognition and social integration after traumatic brain injury. Front Neurol. (2019) 10:825. doi: 10.3389/fneur.2019.00825
78. Li, F, Lu, L, Chen, H, Wang, P, Zhang, H, Chen, YC, et al. Neuroanatomical and functional alterations of insula in mild traumatic brain injury patients at the acute stage. Brain Imaging Behav. (2020) 14:907–16. doi: 10.1007/s11682-019-00053-3
79. Li, F, Lu, L, Shang, S', Hu, L, Chen, H, Wang, P, et al. Disrupted functional network connectivity predicts cognitive impairment after acute mild traumatic brain injury. CNS Neurosci Ther. (2020) 26:1083–91. doi: 10.1111/cns.13430
80. Lu, L, Li, F, Ma, Y, Chen, H, Wang, P, Peng, M, et al. Functional connectivity disruption of the substantia nigra associated with cognitive impairment in acute mild traumatic brain injury. Eur J Radiol. (2019) 114:69–75. doi: 10.1016/j.ejrad.2019.03.002
81. Mayer, AR, Ling, JM, Allen, EA, Klimaj, SD, Yeo, RA, and Hanlon, FM. Static and dynamic intrinsic connectivity following mild traumatic brain injury. J Neurotrauma. (2015) 32:1046–55. doi: 10.1089/neu.2014.3542
82. Meier, TB, Lancaster, MA, Mayer, AR, Teague, TK, and Savitz, J. Abnormalities in functional connectivity in collegiate football athletes with and without a concussion history: implications and role of neuroactive kynurenine pathway metabolites. J Neurotrauma. (2017) 34:824–37. doi: 10.1089/neu.2016.4599
83. Messé, A, Caplain, S, Pélégrini-Issac, M, Blancho, S, Lévy, R, Aghakhani, N, et al. Specific and evolving resting-state network alterations in post-concussion syndrome following mild traumatic brain injury. PLoS One. (2013) 8:e65470. doi: 10.1371/journal.pone.0065470
84. Militana, AR, Donahue, MJ, Sills, AK, Solomon, GS, Gregory, AJ, Strother, MK, et al. Alterations in default-mode network connectivity may be influenced by cerebrovascular changes within 1 week of sports related concussion in college varsity athletes: a pilot study. Brain Imaging Behav. (2016) 10:559–68. doi: 10.1007/s11682-015-9407-3
85. Nathan, DE, Oakes, TR, Yeh, PH, French, LM, Harper, JF, Liu, W, et al. Exploring variations in functional connectivity of the resting state default mode network in mild traumatic brain injury. Brain Connect. (2015) 5:102–14. doi: 10.1089/brain.2014.0273
86. Newsome, MR, Li, X, Lin, X, Wilde, EA, Ott, S, Biekman, B, et al. Functional connectivity is altered in concussed adolescent athletes despite medical clearance to return to play: a preliminary report. Front Neurol. (2016) 7:116. doi: 10.3389/fneur.2016.00116
87. Newsome, MR, Scheibel, RS, Mayer, AR, Chu, ZD, Wilde, EA, Hanten, G, et al. How functional connectivity between emotion regulation structures can be disrupted: preliminary evidence from adolescents with moderate to severe traumatic brain injury. J Int Neuropsychol Soc. (2013) 19:911–24. doi: 10.1017/S1355617713000817
88. Newsome, MR, Mayer, AR, Lin, X, Troyanskaya, M, Jackson, GR, Scheibel, RS, et al. Chronic effects of blast-related TBI on subcortical functional connectivity in veterans. J Int Neuropsychol Soc. (2016) 22:631–42. doi: 10.1017/S1355617716000448
89. Orr, CA, Albaugh, MD, Watts, R, Garavan, H, Andrews, T, Nickerson, JP, et al. Neuroimaging biomarkers of a history of concussion observed in asymptomatic young athletes. J Neurotrauma. (2016) 33:803–10. doi: 10.1089/neu.2014.3721
90. Pagulayan, KF, Petrie, EC, Cook, DG, Hendrickson, RC, Rau, H, Reilly, M, et al. Effect of blast-related mTBI on the working memory system: a resting state fMRI study. Brain Imaging Behav. (2020) 14:949–60. doi: 10.1007/s11682-018-9987-9
91. Plourde, V, Rohr, CS, Virani, S, Bray, S, Yeates, KO, and Brooks, BL. Default mode network functional connectivity after multiple concussions in children and adolescents. Arch Clin Neuropsychol. (2020) 35:302–11. doi: 10.1093/arclin/acz073
92. Rajesh, A, Cooke, GE, Monti, JM, Jahn, A, Daugherty, AM, Cohen, NJ, et al. Differences in brain architecture in remote mild traumatic brain injury. J Neurotrauma. (2017) 34:3280–7. doi: 10.1089/neu.2017.5047
93. Rigon, A, Duff, MC, McAuley, E, Kramer, AF, and Voss, MW. Is traumatic brain injury associated with reduced inter-hemispheric functional connectivity? A study of large-scale resting state networks following traumatic brain injury. J Neurotrauma. (2016) 33:977–89. doi: 10.1089/neu.2014.3847
94. Roy, A, Bernier, RA, Wang, J, Benson, M, French, JJ, Good, DC, et al. The evolution of cost-efficiency in neural networks during recovery from traumatic brain injury. PLoS One. (2017) 12:e0170541. doi: 10.1371/journal.pone.0170541
95. Santhanam, P, Wilson, SH, Oakes, TR, and Weaver, LK. Effects of mild traumatic brain injury and post-traumatic stress disorder on resting-state default mode network connectivity. Brain Res. (2019) 1711:77–82. doi: 10.1016/j.brainres.2019.01.015
96. Sharp, DJ, Beckmann, CF, Greenwood, R, Kinnunen, KM, Bonnelle, V, de Boissezon, X, et al. Default mode network functional and structural connectivity after traumatic brain injury. Brain. (2011) 134:2233–47. doi: 10.1093/brain/awr175
97. Shumskaya, E, Andriessen, TMJC, Norris, DG, and Vos, PE. Abnormal whole-brain functional networks in homogeneous acute mild traumatic brain injury. Neurology. (2012) 79:175–82. doi: 10.1212/WNL.0b013e31825f04fb
98. Shumskaya, E, van Gerven, MAJ, Norris, DG, Vos, PE, and Kessels, RPC. Abnormal connectivity in the sensorimotor network predicts attention deficits in traumatic brain injury. Exp Brain Res. (2017) 235:799–807. doi: 10.1007/s00221-016-4841-z
99. Slobounov, SM, Gay, M, Zhang, K, Johnson, B, Pennell, D, Sebastianelli, W, et al. Alteration of brain functional network at rest and in response to YMCA physical stress test in concussed athletes: RsFMRI study. NeuroImage. (2011) 55:1716–27. doi: 10.1016/j.neuroimage.2011.01.024
100. Sours, C, Chen, H, Roys, S, Zhuo, J, Varshney, A, and Gullapalli, RP. Investigation of multiple frequency ranges using discrete wavelet decomposition of resting-state functional connectivity in mild traumatic brain injury patients. Brain Connect. (2015) 5:442–50. doi: 10.1089/brain.2014.0333
101. Stevens, MC, Lovejoy, D, Kim, J, Oakes, H, Kureshi, I, and Witt, ST. Multiple resting state network functional connectivity abnormalities in mild traumatic brain injury. Brain Imaging Behav. (2012) 6:293–318. doi: 10.1007/s11682-012-9157-4
102. Tang, C, Eaves, E, Dams-O’Connor, K, Ho, L, Leung, E, Wong, E, et al. Diffuse disconnectivity in traumatic brain injury: a resting state fMRI and DTI study. Transl Neurosci. (2012) 3:9–14. doi: 10.2478/s13380-012-0003-3
103. Threlkeld, ZD, Bodien, YG, Rosenthal, ES, Giacino, JT, Nieto-Castanon, A, Wu, O, et al. Functional networks reemerge during recovery of consciousness after acute severe traumatic brain injury. Cortex. (2018) 106:299–308. doi: 10.1016/j.cortex.2018.05.004
104. Vakhtin, AA, Calhoun, VD, Jung, RE, Prestopnik, JL, Taylor, PA, and Ford, CC. Changes in intrinsic functional brain networks following blast-induced mild traumatic brain injury. Brain Inj. (2013) 27:1304–10. doi: 10.3109/02699052.2013.823561
105. Vergara, VM, Mayer, AR, Damaraju, E, Kiehl, KA, and Calhoun, V. Detection of mild traumatic brain injury by machine learning classification using resting state functional network connectivity and fractional anisotropy. J Neurotrauma. (2017) 34:1045–53. doi: 10.1089/neu.2016.4526
106. Zhang, H, Dai, R, Qin, P, Tang, W, Hu, J, Weng, X, et al. Posterior cingulate cross-hemispheric functional connectivity predicts the level of consciousness in traumatic brain injury. Sci Rep. (2017) 7:387. doi: 10.1038/s41598-017-00392-5
107. Zhou, Y, Milham, MP, Lui, YW, Miles, L, Reaume, J, Sodickson, DK, et al. Default-mode network disruption in mild traumatic brain injury. Radiology. (2012) 265:882–92. doi: 10.1148/radiol.12120748
108. van der Horn, HJ, Liemburg, EJ, Scheenen, ME, de Koning, ME, Marsman, JBC, Spikman, JM, et al. Brain network dysregulation, emotion, and complaints after mild traumatic brain injury. Hum Brain Mapp. (2016) 37:1645–54. doi: 10.1002/hbm.23126
Keywords: resting-state functional MRI, rsfMRI, traumatic brain injury, TBI—traumatic brain injury, neuroimaging, functional connectivity, systematic literature review (SLR)
Citation: Kashou AW, Frees DM, Kang K, Parks CO, Harralson H, Fischer JT, Rosenbaum PE, Baham M, Sheridan C and Bickart KC (2024) Drivers of resting-state fMRI heterogeneity in traumatic brain injury across injury characteristics and imaging methods: a systematic review and semiquantitative analysis. Front. Neurol. 15:1487796. doi: 10.3389/fneur.2024.1487796
Edited by:
V. Sujith Sajja, Walter Reed Army Institute of Research, United StatesReviewed by:
Ekaterina Dobryakova, Kessler Foundation, United StatesDan Wang, Shanghai Jiao Tong University, China
Copyright © 2024 Kashou, Frees, Kang, Parks, Harralson, Fischer, Rosenbaum, Baham, Sheridan and Bickart. This is an open-access article distributed under the terms of the Creative Commons Attribution License (CC BY). The use, distribution or reproduction in other forums is permitted, provided the original author(s) and the copyright owner(s) are credited and that the original publication in this journal is cited, in accordance with accepted academic practice. No use, distribution or reproduction is permitted which does not comply with these terms.
*Correspondence: Kevin C. Bickart, a2JpY2thcnRAbWVkbmV0LnVjbGEuZWR1