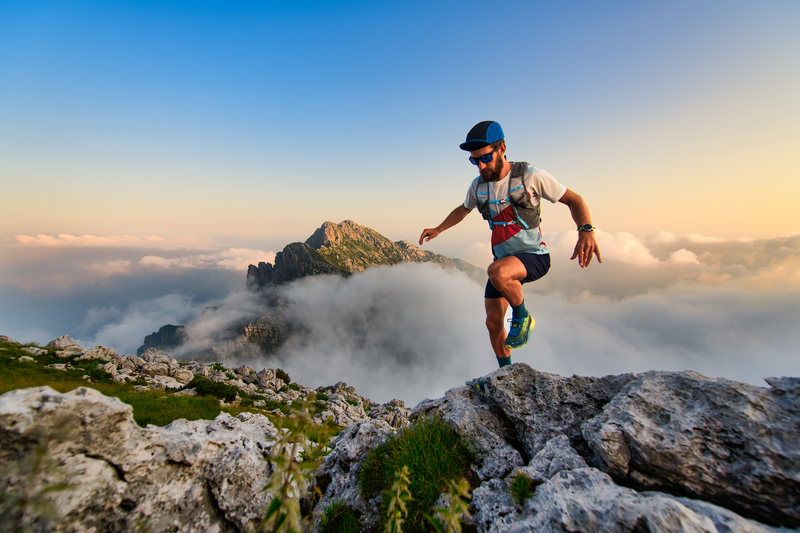
94% of researchers rate our articles as excellent or good
Learn more about the work of our research integrity team to safeguard the quality of each article we publish.
Find out more
REVIEW article
Front. Neurol. , 23 December 2024
Sec. Movement Disorders
Volume 15 - 2024 | https://doi.org/10.3389/fneur.2024.1470928
This article is part of the Research Topic The Neuroscience of Parkinson's Disease: Exploring Causes, Symptoms, and Potential Treatments View all 8 articles
Parkinson’s disease (PD) is a neurodegenerative disorder that significantly impacts patients’ quality of life. Managing PD requires accurate assessment of motor and non-motor symptoms, often complicated by the subjectivity in symptom reporting and the limited availability of neurologists. To address these challenges, commercial wearable devices have emerged to continuously monitor PD symptoms outside the clinical setting. The main devices include PKG™, Kinesia 360™, Kinesia U™, PDMonitor™, and STAT-ON™. These devices utilize advanced technologies such as accelerometers, gyroscopes, and specific algorithms to provide objective data on motor symptoms like tremors, dyskinesia, and bradykinesia. Despite their potential, the adoption of these devices is limited due to concerns about their accuracy, complexity of use, and lack of independent validation. The correlation between these devices’ measurements and traditional clinical observations varies, and patient usability and adherence remain critical areas for improvement. To optimize their utility and improve patient outcomes, it is essential to conduct validation and usability studies with a sufficient number of patients, develop standardized protocols, and ensure integration with hospital information systems.
Parkinson’s disease (PD) currently poses a global health challenge affecting millions of people, with its prevalence steadily increasing over the past decades (1–5). This neurodegenerative disorder is characterized by a wide range of symptoms, both motor and non-motor. Motor symptoms, including tremors, rigidity, and bradykinesia, profoundly impact patients’ daily lives (6). Despite significant advances in research, the management of Parkinson’s remains complex and highly dependent on the neurologist’s expertise and the accuracy of the patient’s symptom reporting. This dependency is exacerbated by the shortage of neurologists in many regions worldwide, especially in rural areas where access to movement disorder specialists is limited. Consequently, many patients must travel long distances regularly to receive specialized care, imposing a significant additional burden. This situation results in a considerable number of PD patients being managed by primary care physicians rather than neurologists. In developed countries such as Germany and the United States, over 40% of Parkinson’s patients are treated by primary care physicians (7).
Setting aside the professional’s experience in treating the patient, all healthcare providers rely on the patient’s subjective account and medical history during consultations, introducing bias in the information provided. Due to the nature of the disease and its symptoms, patients struggle to identify and distinguish specific symptoms, often confusing them (8–10). Furthermore, the patient’s account is heavily influenced by their mood at the time of the consultation, tending to report positive periods when they feel well and vice versa. This bias is often mitigated with comments or opinions from caregivers. However, both the patient and the caregiver tend to overlook mild symptoms or those with little impact on daily life, especially when recalling extended periods. Although these symptoms may seem less relevant in routine clinical practice, they are of great importance in clinical trials of new therapies or in evaluating new approaches to symptoms. The accurate assessment of mild and fluctuating symptoms, as well as their evolution over time, is of great scientific interest (7).
In response to these challenges, wearable devices emerge as highly promising tools in the management of PD. Specifically designed to assess PD, these devices offer a new approach by providing quantitative data on motor symptoms outside traditional clinical settings (11–13). These systems can continuously measure symptoms over extended periods in ambulatory conditions, providing neurologists with objective information on disease progression and treatment responses, enabling more informed decision-making and personalized interventions.
Despite their potential, the adoption of these devices remains limited. The main barrier to adoption lies in professionals’ low confidence in these solutions, which, according to the authors’ experience, is due to two clear factors. Firstly, many of the tools published or launched on the market have questionable validation studies and seem to have serious issues with false positives for the symptoms they attempt to measure. This factor, combined with the increasing number of available tools and the ensuing “commercial war” that floods professionals with information, leads to rejection and distrust towards these types of tools. Secondly, there is a tendency to maintain the classical measurement variables to assess the patient’s evolution. A clear example is the patient’s OFF time, a measure that presents multiple biases due to its definition and the way it is collected. Professionals tend to give more credibility to the patient’s account than to objective measures, including patient diaries. In a recent study, it was shown how low the correlation was between clinician observation and PD diaries (14, 15). This means that a neurologist may not adjust a patient’s treatment if the patient claims to be doing well, even if objective measures or diaries show that the patient experiences motor impairments for much of the day.
Another barrier to the adoption of these systems is their usability for both patients and professionals. The complexity of using these tools poses an obstacle for a portion of the patient population. The complexity of the data flow and doubts about consistent compliance with data protection standards are probably the main adoption barriers for professionals. It is particularly important that these tools and the data flow are as simple and clear as possible, aiming to be practically transparent for both the patient and the neurologist (16, 17). To overcome these barriers, it is necessary for the devices to have validation and usability studies in clinical practice with a sufficient number of patients and for practical recommendations and standardized protocols to be generated to optimize usefulness and ensure results for patients, caregivers, and physicians alike. Additionally, integrating these systems with hospital IT systems would be crucial to provide all stakeholders with a secure and transparent data flow.
This review focuses on the main commercialized devices for monitoring motor symptoms, which have been the most clinically validated and are recommended by the National Institute for Health and Care Excellence (NICE) (18). Additionally, all promising devices that could be useful in clinical practice and have received medical device certification, such as FDA or CE certification, have been considered and discussed in this manuscript.
In January 2024, NICE published an updated list of wearable devices that are conditionally recommended for use in clinical practice (18). The main aim of publishing this guideline was to emphasize the importance of tracking Parkinson’s disease symptoms to help clinicians make informed care decisions. However, this process is challenging in current medical practice due to the fluctuating nature of symptoms and the difficulty patients may have in accurately recalling or describing them. NICE identified a total of five technologies based on their validation and characteristics: PKG™, Kinesia 360™, Kinesia U™, PDMonitor™, and STAT-ON™. These are currently the only devices conditionally endorsed by NICE for this purpose. Table 1 shows a brief comparison between the devices described in this Section.
Table 1. Conditionally recommended devices by NICE for remote monitoring of Parkinson’s disease to inform treatment decisions.
The PKG™, from Global Kinetics Pty Ltd., is a wrist-worn device designed to continuously monitor the motor symptoms of Parkinson’s Disease. The patient uses the device for 6 days, and the data is then uploaded to a server where it is processed, and a report is sent to the neurologist. The device has been approved under CE (Class IIa), FDA (Class 2), and TGA.
The PKG™ algorithm was published in 2012 by Griffiths et al. (19). The authors introduced a method based on accelerometer signal analysis obtained from the wrist during 2 min windows. From this window, it is analyzed the frequency characteristics between 0.2 Hz and 4 Hz, the maximum acceleration reached, and the time without movement, from which two indices are generated. One is associated with bradykinesia (BKS) and the other with dyskinesia (DKS), which are then represented on a graph with interquartile ranges to determine the severity of one symptom or the other. There is no evidence of a training-evaluation data method, so it is not considered a machine-learning algorithm. Validation was performed through the median of all BKS samples over 9 h across 10 days and correlated with the UPDRS, obtaining a significant r value = 0.64, p < 0.0005. A third score called FDS was designed to measure motor fluctuations (20). This score, expressed as an algebraic combination of BKS and DKS, determines if a patient is fluctuating. The device has been widely tested in clinical conditions and compared with UPDRS (21) or diaries (8, 20). For example, the work of Santiago et al. (22) determined that in 41% of neurologists, PKG™ provided more information than classic routine visits. In a study by Nahab et al., the authors also indicate utility in clinical practice (23). Furthermore, the system has shown good results in usability (24), although there were some scores to be discussed. For example, only 27% of patients scored positively on the report of PKG, and 59% rated the use of PKG as valuable in offering useful information to clinicians. Interesting articles can be found, such as the one from Ossig et al. (8). In this study, the agreement was moderate to high in the total number of OFF and ON with and without dyskinesia (K = 0.404 in OFF with bradykinesia and K = 0.658 with ON with dyskinesia). However, a low to moderate agreement was found if the agreement on every single-hour-level (in OFF with bradykinesia K = 0.215 and in ON with dyskinesia correlation of K = 0.324). A similar situation is observed in Löhle et al. (25), where there is a moderate correlation between PKG and clinicians when total hours are compared (0.43 in bradykinesia and 0.51 in dyskinesia). However, a poor correlation is noted when outcomes are compared every 30 min (0.13 in bradykinesia and 0.21 in dyskinesia). According to Monje et al. (11), the PKG™ has been widely validated but needs further independent validation. In the same line, the NICE highlights in the published guideline that PKG is the device with more clinical validations but more evidence is needed.
Great Lakes Neurotechnologies (GLN) is the main manufacturer of Kinesia 360, a double-device system (wrist and ankle), and Kinesia U™, a wrist-worn device. The device incorporates a triaxial accelerometer and gyroscope. Among all the commercialized existing devices, GLN was the first company to achieve FDA 510(K) clearance as a tremor transducer device. In Europe, Kinesia 360 is considered a Class I device. Kinesia 360™ provides information about tremor, dyskinesia, slowness, mobility, posture, and steps (26). The quantification of bradykinesia conducted on an ankle-mounted device is based on the analysis of specific frequency characteristics derived from both the accelerometer and gyroscope and is calculated using linear regression models which are correlated with UPDRS scores (27, 28). The dyskinesia algorithm also relies on a linear regression model with sensors worn on the more affected side of the body (29). However, the number of features evaluated is significant, totaling 18. The obtained correlation with the modified Abnormal Involuntary Movement Scale (mAIMS) is significant (r = 0.77). Both models to determine bradykinesia and dyskinesia are more complex than the method presented by Griffiths et al. for the PKG due to the significant amount of features extracted from the accelerometer and gyroscope. The Kinesia 360 device also measures essential tremor, which was tested with 20 PD patients with intraclass correlation coefficients over 0.7 (30). Kinesia 360 has been evaluated in numerous studies and demonstrated its effectiveness in some therapies such as levodopa (31), rotigotine (32), deep brain stimulation (33), or subthalamic stimulation (34).
GLN also launched a device called Kinesia U™ for continuous monitoring but also for specific tasks. The main advantage of this new device was to eliminate the ankle-mounted device, improving the adherence of the patient to this technology by using only a wrist device. However, it is not clear how this affected the algorithm. The new app includes the capacity to fill in a diary and rate their symptoms. Results are provided in a 0 to 4 score indicating the severity of each symptom. In Pulliam et al. (31), it was shown that Kinesia presents a good accuracy for tremor, dyskinesia, and bradykinesia, being tremor and dyskinesia the best symptoms detected with the Area under curve (AUC) of 0.89 and 0.86, respectively. The bradykinesia algorithm has an AUC of 0.82 and a false positive rate of 0.34.
PDMonitor™ is a system composed of five devices designed to comprehensively characterize all motor symptoms of a Parkinson’s disease (PD) patient from any part of the body. The device, which is manufactured by PDNeurotechnology, is a CE Certificate device Class IIa. Each device includes an accelerometer, gyroscope, and magnetometer (7). This eliminates the need to choose the most affected side, allowing for the monitoring of movements from the upper limbs, lower limbs, and trunk. The system was designed within the PERFORM project (35, 36), and its algorithms are based on training a database of experts and utilizing machine learning algorithms. The algorithms are briefly described in different publications, including tremor (37), dyskinesia (38), bradykinesia (39), and Freezing of Gait (FoG) (40). Gait parameters and the ON and OFF states are also provided (41). Each algorithm employs a distinct classification method. For instance, the tremor detection algorithm uses Hidden Markov Models and achieves an accuracy of 0.87. The dyskinesia detection algorithm is based on a decision tree with a classification accuracy of 0.85. The bradykinesia detection algorithm utilizes Support Vector Machines and has a classification accuracy of 0.745. For this machine learning classifier, 20 patients participated in clinical settings and 24 in home environments. The algorithm for FoG, on the other hand, employs a Random Forest classifier achieving an accuracy of 0.96. The database was formed by 5 patients with FoG, 6 patients without FoG, and 5 healthy patients (40).
In another study in 2023, Antonini et al. published an article where the accuracy, sensitivity, and specificity of the different symptoms were evaluated against UPDRS and diaries. All the results on sensitivity, specificity, and accuracy for all the algorithms are over 0.8, except for the sensitivity of Gait (0.67). The accuracy and specificity achieve 0.96 or more in all the symptoms. Bradykinesia obtains a moderate-high correlation of 0.68 with UPDRS. In 2021, due to the appearance of COVID-19, a 2-cases study was presented showing the feasibility of PDMonitor™ for monitoring symptoms in home environments (42). PDMonitor™ was also evaluated against UPDRS, achieving moderate to high correlations in all their claimed detected movements/symptoms (41). This article questions the subjectivity of questionnaires, given that showing a device works does not need to correlate highly with questionnaires, which have doubtful outcomes. Although the device offers a comprehensive map of motor symptoms, there are doubts about the wearability of the device due to using five separate devices (13). However, in Antonini et al. (43), it should be mentioned that the scores obtained in wearability are significantly high except for two items: difficulty in putting on the device and that patients would wear the device if it was invisible. These items are of special importance because a patient in the morning usually suffers morning akinesia due to the deep OFF that they could suffer. Having to set up five sensors is an important barrier that could drastically reduce adherence to the technology and is something to consider.
STAT-ON™ is a medical device Class IIa which is worn on the waist (44). The device, which was designed under the project REMPARK (45, 46), has been commercialized by Sense4Care SL. The device aims to minimize the number of sensors while maximizing high accuracy by measuring symptoms from a position near the centre of the human body and using machine learning techniques trained with large databases.
The system detects bradykinesia (47, 48), dyskinesia (49), FoG (50, 51), gait parameters (48, 52), and falls (53), providing ON and OFF outcomes (54). The device is based on machine learning algorithms, more specifically in support vector machine classifiers and support vector regression models. The database was obtained in home environments, and the gold standard was the video and the UPDRS-III. For the validation of the ON and OFF algorithm, diaries were used, and a clinician called the patient every 2 h to ensure the motor state annotated by the patient, creating a robust database. A total of 92 PD patients participated in the database, and 10 extra PD patients participated in the dyskinesia algorithm. The algorithm for ON and OFF was evaluated with 91 PD patients in 3 studies (54–56) obtaining sensitivity and specificity values over 0.92. The bradykinesia algorithm was evaluated with 75 patients employing UPDRS subscales and achieving a moderate-high correlation of 0.67 p < 0.01 (57). The dyskinesia algorithm, evaluated with a leave-one-out method and 102 patients, achieved a sensitivity and specificity of 1 and 0.95 in trunk dyskinesia, 0.90 and 0.95 in strong dyskinesia in limbs and neck, 0.78 and 0.95 in mild dyskinesia in the trunk, and 0.39 and 0.95 in detecting mild dyskinesia in limbs [59]. Finally, the FoG algorithm was evaluated with 15 PD patients with FoG, achieving 0.92 and 0.87 in sensitivity and specificity, respectively.
In an Italian study performed by Zampogna et al. (58), the FoG and Dyskinesia were evaluated in 71 PD patients and an AUC was obtained of 0.92 and an accuracy of 0.8. The FoG evaluation obtained 0.83 on AUC and 0.81 on accuracy comparing FoG patients vs. PD patients without FoG. The number of FoG episodes AUC obtained a score of 0.87.
In Cabo-Lopez et al. (59), the correlation between STAT-ON™ and the clinical decision for considering a patient candidate for second-line therapy was 0.73 (p < 0.001) and a significant association in results between STAT-ON™ and the MANAGE-PD questionnaire (p = 0.004). Finally, a clinical trial with 84 PD patients comparing STAT-ON™ against diaries, having the UPDRS as the gold standard, obtained a correlation of 0.63 (p < 0.001) against the 0.24 obtained by the diaries (60).
In January 2023, the NICE recognized 5 technologies based on the information obtained from previous years. However, in the last 2 years, several technologies have emerged and obtained their medical device certification. It is fair to consider these technologies as valid as the previous ones, but considering that their validation in clinical studies is still far from the described technologies in the previous section. Table 2 provides a set of suggested guidelines that could help clinicians in selecting wearable sensors based on specific clinical needs in PD. These recommendations may assist in identifying the most suitable devices for different scenarios, considering factors such as symptom detection, usability, and patient characteristics.
Neptune™ from Orbit Health GmbH is a class IIa device that monitors bradykinesia, dyskinesia and ON/OFF states. The algorithm employed deep-learning techniques, specifically convolutional neural networks, for the detection of ON, OFF, and Dyskinesia states. The window size entered into the convolutional neural network is 1 min in length and obtained 0.654 accuracy in a three-class outcome problem. The sensitivity and specificity obtained for OFF was 0.64/0.89, for ON 0.67/0.67, and for Dyskinesia 0.64/0.89. However, the correlation between the complete data obtained and the MDS-UPDRS subscale 3.14 was high, with 0.83 for bradykinesia and with AIMS item 5 was 0.84 for dyskinesia detection. Correlations with lower windows (1 h, 30 min, 5 min, and 1 min) decrease but maintain a moderate/high correlation, going from 0.775 for dyskinesia and 0.735 for bradykinesia for 60 min to 0.703 for dyskinesia and 0.632 for bradykinesia with a window length of 1 min.
The main advantage of deep learning is the high performance of the classifier and the ability to compute complete raw inertial data without treating, extracting, and selecting the key features, being what is called a “black box” (61). However, this has been the focus of discussions due to the transparency of these methods in the field of computer science. Another characteristic of deep learning is that it is used to be a method for training large databases such as video or image with thousands or millions of signals (pixels) per sample. Unfortunately, a triaxial signal accelerometer in 30 PD patients is not considered a large database. This circumstance has the problem of overtraining a classification model leading to false positives in new evaluation data. Thus, more evidence is needed in this device to validate from a computer science point of view their approach.
PD-Watch from Biomedical Lab s.r.l. is a wrist-worn device classified as a Class I medical Device based on inertial sensors. The device is a 43 mm × 40 mm × 13 mm smartwatch and weighs 16 g. It has a battery life of 15 days. The data is recorded and then uploaded to a cloud server, from where it is processed and a report is returned. The device is capable of detecting ON/OFF, bradykinesia (62), tremor (63), dyskinesia (64), and inactivity with the absence of movement. One of the differences with other smartwatches devices is that PD-Watch can also detect dyskinesia severity. All the algorithms are based on extracting frequency features, and a structure of conditions that once met, the frequency response of the accelerometer is compared to a certain threshold (62). Results show more than 0.8 in sensitivity, specificity, and accuracy in all the algorithms. Further and external evidence is needed for this threshold-based algorithmic system.
Feetme™ from Feetme, is a wearable device that consists of two insoles, a device for connecting the insoles, and an app to manage the insoles. This device can continuously monitor movement and foot pressure in order to obtain gait parameters. The device includes a triaxial accelerometer and gyroscope and 18 capacitive pressure sensors (65). Feetme™ has received ClassIm in Europe and Class I FDA 510(k) exempt medical device.
The APP connects to the device which uploads the inertial and pressure data and processes it to provide different outcomes. Some of these outcomes are gait parameters such as stride velocity, cadence, stride length, step time, stride time, swing time, stance time, and single and double support time (66). All these features have been tested to evaluate stroke (67, 68), multiple sclerosis (69), or Parkinson’s Disease (66, 70). The device was evaluated against a recognized gold standard such as Gait-rite also in healthy adults (69, 71). Feetme™ has demonstrated to provide good results in tests such as the 6 min walk test (65). However, in the field of Parkinson’s Disease, Feetme™ has not shown any clinical utility for assessing motor symptoms such as bradykinesia, freezing, dyskinesia, or tremor.
Another similar intended-use device is Nushu, from Magnes, which has been registered in the FDA under a Class 2 device. The main difference is that Nushu are shoes instead of insoles and that the system uses a magnetometer but not pressure sensors (72). Values such as stride velocity, stride time, stride length, swing time, cadence, symmetry and variability of steps are provided. Nushu is based on inertial sensors (triaxial accelerometer and triaxial gyroscope) and a triaxial magnetometer. An orientation algorithm is set if it detects motion or not. The algorithm employed to estimate the orientation is based on Madgwick’s filter, which reduces computational burden compared to classical Kalman filters (73). A segmentation of the signal and event detection based on thresholds are executed to finally extract spatial and temporal gait parameters (74). Nushu’s algorithms also used SVM and reduced-SVM for detecting gait phases, which shows a high algorithmic level, which is used to detect Freezing of Gait and provide a vibrotactile biofeedback to avoid FoG on PD patients (75). The device has been only tested with healthy users, although authors claim that the device is under some clinical trials in the field of Parkinson’s Disease (72).
The device works with an app that allows configuring the type of activity to record. After the test or the recording, which is stored in an internal memory, the data is transferred via Bluetooth to the app and then uploaded to a cloud from where, through a dashboard, the results can be seen (76). Similarly to Feetme™, it is claimed the relation of gait parameters to motor symptoms of Parkinson’s Disease, however, further studies are needed to validate this idea rigorously.
In 2021, Powers et al. (77) published an article where a smartwatch named MM4PD could monitor tremor and dyskinesia in Parkinson’s Disease. The study was divided into three phases. The first one in clinics was video recorded with three movement disorders specialists rating. A subset of subjects participated in a 1-week out-of-clinic measurement period for obtaining data from daily living conditions. In the second phase, 225 PD patients participated, from which 143 were used to design the algorithm and 82 for validation. Finally, a third part was performed with 171 control users. For the gold standard, video was used, but also MDS-UPDRS-III was employed.
The device collected signals from a triaxial accelerometer and triaxial gyroscope at 100 Hz. The resting tremor algorithm was based on an analysis of the signal between 3 and 7 Hz in a 2.56 s window, but it’s not clear the classification method, which significantly detracts from the credibility of the method in the scientific field, as Bloem et al. reports (78). The algorithm classifies essential tremors into slight, mild, moderate, and severe tremors.
The dyskinesia algorithm uses a 10.24 s window overlapped every 2.56 s to not lose information between windows. The algorithm of dyskinesia is not explained although it is reported a low false positive rate despite a possible confusion with walking. Also, in 69 PD patients, the clinicians disagreed with the dyskinesia label.
A total of two solutions resulted from this method: StrivePD from Rune Labs and Parky from H2O Therapeutics. The two devices were FDA 510(K) cleared and claim tremor and dyskinesia in their intended use. Although the algorithms are the same and run on the Apple Watch, the layout of their solutions and their services are different.
On the other hand, NeuroRPM from NeuroRPM Inc. is another software that runs on an Apple Watch and besides detecting dyskinesia and tremor, it also detects Bradykinesia according to its intended use (FDA 510(k) Clearance number K221772). There is no scientific evidence apart from the summary report provided in the FDA clearance which shows a study with 36 PD patients and a sensitivity and specificity of 0.718 and 0.951 for tremor, 0.714 and 0.774 for bradykinesia, and 0.712 and 0.947 for dyskinesia. The outcomes are provided every 15 min and are binary for each of the three outcomes: tremor (yes/no), dyskinesia (yes/no), and bradykinesia (low to normal/severe). NeuroRPM has registered a clinical trial (NCT05680961) executed at the Parkinson’s & Movement Disorders Centre of Maryland. The lack of information on the algorithm is a major drawback, taking into account the significant amount of smartwatches that obtain these measurements.
Surface electromyography (sEMG) is a non-invasive technique used to measure the electrical activity produced by skeletal muscles, making it a valuable tool in the research and management of Parkinson’s disease (79–82). sEMG involves placing electrodes on the skin above specific muscles to detect the electrical signals generated during muscle contraction and relaxation. In the context of Parkinson’s disease, sEMG can be used to analyze the muscle activity patterns that are often disrupted by the condition (rigidity or dystonic dyskinesia).
sEMG can also help in monitoring tremors by providing detailed data on their frequency and intensity, or in assessing muscle rigidity and coordination (83). With the help of an accelerometer, the system could provide a comprehensive state of the patient (84). In the market, we can find Adamant Health (85), a company that commercializes a solution for capturing sEMG signals from different parts of the body with small wearable sEMG sensors and provide outcomes about the motor states of PD patients. Adamant Health has a wide scientific background in the recognition of motor symptoms in Parkinson’s Disease (81, 83, 84, 86–88). On the other hand, Paragit Neurotech provides a specific device with EMG that can be mounted on a patch at any part of the body (89). The device contains an accelerometer, a gyroscope, and an internal memory for data logging. The sensor fusion enables identifying the appearance of tremor, stiffness and according to the information on the webpage, bradykinesia and dyskinesia.
Other devices such as Ultium from Noraxon (90), Trigno from Delsys (91), Freeemg from BTS Bioengineering (92, 93), and Mini Wave from Cometa (94) have been used to characterize some motor symptoms or gait parameters in PD. These device systems are composed of several sensors and can be set at any part of the body. Given that there is no standardization on the point where the sensors must be located, these devices are mainly intended to be used in research and clinical studies. As far as authors know, only Adamant Health and Paragit Neurotech have incorporated algorithms that allow quantifying symptoms.
Wearable sensors have revolutionized the assessment and monitoring of gait, especially in the context of Parkinson’s Disease (PD). These devices offer a non-invasive, continuous, and objective means to capture detailed gait parameters in real-world settings, crucial for both clinical management and research. Companies like APDM Wearable Technologies, McRoberts, Gait Up, Moticon, Ephion Health, and MHealth Technologies have developed sophisticated wearable systems that are particularly useful in the management of PD.
APDM Wearable Technologies, now a part of Clario, offers a suite of wearable sensors named Opal V2C. The company’s flagship product, the Opal sensor, is a small, lightweight device that can be attached to different parts of the body to capture detailed movement data. A total of 24 sensors can be attached to different parts of the body. The battery life of each sensor is 4 days (8 h per day), and every sensor contains a triaxial accelerometer and gyroscope. The Opal V2C has been designed for specific tasks such as the Timed-up and Go test (95), 2 and 6 Minutes walking test, including open-ended walk and turns (96). Balance activities, sit-to-stand, 360-degree turns, and activity and sleep data in daily living activities are also included in the set of activities that can be recorded and analyzed. In the field of Parkinson’s Disease, Opal has been mainly used for the analysis of gait, being correlated with UPDRS subscales of gait or even correlating gait and balance problems with cognitive symptoms (96–98). However, although the device provides a lot of information from specific activities, balance, and gait parameters, there is no association with PD symptoms such as bradykinesia, freezing of gait, or tremor.
An interesting device to understand both the severity and distribution of dyskinesia in several parts of the body is LID-Monitor from ClearSky. This device is based on 6 sensors that are set on the head, upper and lower limbs, and chest (99, 100). The monitoring period is up to 24 h. The algorithm is based on an evolutionary algorithm and achieves results of AUC > 0.9 (99).
One of the most interesting companies that manufactures medical devices for gait is McRoberts B.V. This company has developed three types of sensors: Dynaport7, the MoveMonitor, and the MoveTest (101). The Dynaport7 is the latest device offering extensive communication options, including USB, Wi-Fi, and Bluetooth. It collects data and generates results in under 15 min. Measuring 106.6 × 58 × 11.5 mm and weighing 55 g, it features a triaxial accelerometer and gyroscope with a battery life of 5 days. Another device, the MoveMonitor, has a battery life of 14 days and is designed primarily for long-term monitoring of activity in home environments. Lastly, the MoveTest is intended for specific evaluations. It includes a package of six tests: the 6-Minute Walk Test, Sit-to-Stand Test, Gait Test (to assess gait quality and extract spatiotemporal gait parameters), Sway Test (to measure patient balance), the Timed Up & Go Test, and the Short Physical Performance Battery (a brief test assessing general movement including gait speed, chair stand, and balance tests).
As remarked, McRoberts’ devices are focused on the analysis of gait, postures, balance, and specific tests, but there is no association with motor symptoms of Parkinson’s Disease (102). However, the device is useful for understanding the progression of PD based on gait, balance, activity of the patient or sleep patterns.
Other interesting devices that have been used in the field of Parkinson’s are Physilog 6 from Gait Up and mTest3 from mHealth, which are inertial systems focused on assessment and rehabilitation. These devices are designed to provide detailed insights into the patient’s gait, posture, and overall mobility, but not motor symptoms.
In addition, OpenGo insoles from Moticon are notable for their emphasis on research. These smart insoles are embedded with sensors that measure pressure distribution and gait patterns in real-time. They are particularly useful in research settings where detailed biomechanical data is essential for understanding the progression of Parkinson’s disease and the impact of various interventions.
In this paper, several sensors have been presented. Thus, when healthcare professionals have the chance or want to monitor a patient with Parkinson’s, many questions arise: which is the best one? Which sensor suits my necessities? Is it going to be reliable? How much time does it take to understand this new technology? Is the patient going to have good adherence to the monitoring tool? All these questions are difficult to answer because every study is completely different and there are different necessities. For example, if a healthcare professional wants to monitor ON and OFF fluctuations, the best sensors are those that offer a comprehensive view of different symptoms from different parts of the body. If the neurologist wants to measure tremors, then a wrist-worn device is the best option. Measuring, for example, dyskinesia, which happens in all parts of the body with only a shoe sensor or a wrist sensor, will lead to several false negatives. From the waist, it is feasible to detect many parts of the body due to the location close to the centre of mass, but some of the upper limb dyskinesia cannot be measured (103). Multiple sensor systems might provide a complete approach to choreic dyskinesia. On the other hand, wrist-worn devices can be an optimal solution for measuring tremor and getting an indicative estimation of the state of the patient. Wrist-worn devices have slightly better scores than waist devices in usability (104, 105), and this is an advantage for psychiatric patients or patients who do not want to use multiple or waist-worn sensors.
However, literature has demonstrated that waist devices outperform wrist-mounted devices in analyzing human movement in general. In Kluge et al. (106), sensitivity was 20% higher in waist-mounted devices, and in patients with diseases such as PD, this distance increased. The number of false positives due to random movements and that from the wrist it is not possible to measure specific movements from the body, increasing the false negatives, has been reported in several studies (107–111).
Another point is the number of sensors. The usability of a device is essential to keep good adherence of the patient and reduce the rejection rate in clinical studies. In Parkinson’s Disease and other neurodegenerative diseases, the use of numerous devices might be stigmatizing to the patient, and minimizing the number of sensors is crucial for social matters. However, although it is common to think that more devices are better for characterizing motor symptoms, which in some cases is true (112), the complexity of algorithms is also crucial. A good example is presented in Rodríguez-Martín et al. (113), where several classifiers and features of inertial signals are tested, and the performance of the algorithms increases based on the complexity of the algorithm.
Some studies compare some of the mentioned devices (51, 104, 105), but it is not clear against which gold standard they should be compared. As mentioned in this paper, questionnaires are subjective, and for example, UPDRS has demonstrated high inter-intrarater variability (11, 114), and diaries do not correlate enough with medical opinion (14, 15). Cabo et al. compared a wearable device against different scales concluding that current clinical scales are time-consuming and subjective compared to some objective wearable devices (59). Some studies with STAT-ON™, and that could be generalised to other devices, show that the amount of information obtained with wearables is higher with diaries given that patients do not recall to fill them in.
Another aspect is to remind that devices with an FDA or CE Certification only provide a guarantee of safety for the patient with very few clinical validation data presented to the authorities. In other words, a certificate does not guarantee that a sensor works accurately. A good example happens in the FDA medical devices classification, which only has a classification product for devices for monitoring Parkinson’s symptoms, which is the “tremor transducer” (GYD classification). However, although there is not a bradykinesia or dyskinesia classification, some devices such as PKG, Kinesia, or NeuroRPM have been accepted as devices to measure these symptoms.
There are three key challenges that wearable devices need to address. The first is accurately monitoring sleep patterns. Although accelerometers are commonly used to estimate sleep by detecting periods of minimal movement, they have significant limitations. These sensors cannot adequately distinguish between actual sleep and quiet wakefulness (e.g., watching a movie or being in an OFF state), as they only measure physical activity and do not capture the physiological changes that occur during different sleep phases. Zampogna et al. suggest using more advanced techniques, such as EEG, EMG, or electrooculography, to analyze REM and NREM phases; however, implementing these techniques in commercial devices remains a challenge (115). The PKG system, which also relies on accelerometers, has shown preliminary results that could be useful for sleep monitoring, although more evidence is needed to confirm its effectiveness (21, 116).
The second challenge, which still lacks sufficient evidence, involves the assessment of non-motor symptoms. While some reviews have highlighted the usefulness of certain wearable devices in different aspects, many current approaches rely on inertial systems to evaluate drowsiness or the amount of movement (117, 118). Additionally, some devices have started incorporating patient feedback to complement the data collected by sensors, which can provide extra information about symptoms such as stress or anxiety. Other methods, such as photoplethysmography, EEG, ECG, and electrodermal activity, have also been used to assess these conditions; however, further studies are needed to validate their effectiveness in this area (107).
The third challenge focuses on early detection and prediction of motor symptoms, which could greatly improve patient outcomes by enabling timely intervention. However, current advancements are limited to exploratory studies, and no commercial medical device with proven predictive capabilities exists yet (119, 120). Continued research and development are essential to make symptom prediction a clinical reality.
Wearable devices hold great potential for improving the monitoring of treatment in Parkinson’s Disease by providing continuous, objective data on patient response. Unlike traditional clinical assessments, which are limited to brief, periodic evaluations, wearables can track changes in real time, offering valuable insights into medication effectiveness and highlighting fluctuations that might be missed otherwise. This capability allows clinicians to make timely adjustments to treatment regimens, optimizing dosage and reducing the risk of adverse effects. Continuous monitoring can help identify specific periods of poor symptom control or medication wearing-off episodes, enabling more precise management. By integrating wearable data into clinical practice, we can move towards a more personalized and adaptive approach to treatment, ultimately improving patient outcomes and enhancing quality of life.
The aim of this paper is to provide comprehensive information and organize the numerous sensors available on the market. Devices for monitoring Parkinson’s Disease (PD) symptoms represent a significant advancement in managing the condition. These devices offer continuous, objective data on motor symptoms, which can help clinicians make better decisions and potentially improve patient outcomes. They provide detailed information on symptoms such as bradykinesia, freezing of gait, ON/OFF fluctuations, and dyskinesia over extended periods in real-world settings, enhancing diagnosis and monitoring. While classical methods have proven useful, they have certain limitations. The devices discussed in this paper can complement the information obtained from traditional clinical trials and studies.
However, several challenges need to be addressed for these devices to be widely adopted. More validation studies are necessary, and in many cases, external validation or validation by official authorities such as NICE, FDA, or EMA is required. Additionally, integrating these devices into hospital systems to ensure secure and transparent data flow remains a significant challenge.
Overcoming these barriers will optimize the usefulness of these tools and ensure better outcomes for patients, caregivers, and healthcare providers. This paper has presented several sensors, many of which have substantial scientific endorsement and are recommended by unbiased authorities such as NICE.
DR-M: Conceptualization, Methodology, Writing – original draft, Writing – review & editing. CP-L: Conceptualization, Methodology, Writing – original draft, Writing – review & editing.
The author(s) declare that no financial support was received for the research, authorship, and/or publication of this article.
The authors declare that are shareholders of Sense4Care SL, a company that manufactures STAT-ON, a device included in this review.
All claims expressed in this article are solely those of the authors and do not necessarily represent those of their affiliated organizations, or those of the publisher, the editors and the reviewers. Any product that may be evaluated in this article, or claim that may be made by its manufacturer, is not guaranteed or endorsed by the publisher.
1. Kalia, LV, and Lang, AE. Parkinson’s disease. Lancet. (2015) 386:896–912. doi: 10.1016/S0140-6736(14)61393-3
2. Nussbaum, RL, and Ellis, CE. Alzheimer’s disease and Parkinson’s disease. N Engl J Med. (2003) 348:1356–64. doi: 10.1056/NEJM2003ra020003
3. Maserejian, N, Vinikoor-Imler, L, and Dilley, A. Estimation of the 2020 global population of Parkinson’s disease (PD) International Congress of Parkinson’s Disease and Movement Disorders (2020) Available at: https://www.mdsabstracts.org/abstract/estimation-of-the-2020-global-population-of-parkinsons-disease-pd (Accessed December 15, 2024).
4. Dorsey, ER, Elbaz, A, Nichols, E, Abbasi, N, Abd-Allah, F, Abdelalim, A, et al. Global, regional, and national burden of Parkinson’s disease, 1990–2016: a systematic analysis for the global burden of disease study 2016. Lancet Neurol. (2018) 17:939–53. doi: 10.1016/S1474-4422(18)30295-3
5. Rossi, A, Berger, K, Chen, H, Leslie, D, Mailman, RB, and Huang, X. Projection of the prevalence of Parkinson’s disease in the coming decades: revisited. Mov Disord. (2018) 33:156–9. doi: 10.1002/mds.27063
6. Stoker, TB, and Greenland, JC. Parkinson’s disease: Pathogenesis and clinical aspects. Brisbane, Australia: Codon Publications. (2018).
7. Reichmann, H, Klingelhoefer, L, and Bendig, J. The use of wearables for the diagnosis and treatment of Parkinson’s disease. J Neural Transm. (2023) 130:783–91. doi: 10.1007/s00702-022-02575-5
8. Ossig, C, Gandor, F, Fauser, M, Bosredon, C, Churilov, L, Reichmann, H, et al. Correlation of quantitative motor state assessment using a Kinetograph and patient diaries in advanced PD: data from an observational study. PLoS One. (2016) 11:e0161559. doi: 10.1371/journal.pone.0161559
9. Papapetropoulos, SS. Patient diaries as a clinical endpoint in Parkinson’s disease clinical trials. CNS Neurosci Ther. (2012) 18:380–7. doi: 10.1111/j.1755-5949.2011.00253.x
10. Goetz, CG, Stebbins, GT, Blasucci, LM, and Grobman, MS. Efficacy of a patient-training videotape on motor fluctuations for on-off diaries in parkinson’s disease. Mov Disord. (1997) 12:1039–41. doi: 10.1002/mds.870120631
11. Monje, MHG, Foffani, G, Obeso, J, and Sánchez-Ferro, Á. New sensor and wearable technologies to aid in the diagnosis and treatment monitoring of Parkinson’s disease. Annu Rev Biomed Eng. (2019) 21:111–43. doi: 10.1146/annurev-bioeng-062117-121036
12. Luis-Martínez, R, Monje, MHG, Antonini, A, Sánchez-Ferro, Á, and Mestre, TA. Technology-enabled care: integrating multidisciplinary Care in Parkinson’s disease through digital technology. Front Neurol. (2020) 11:1–10. doi: 10.3389/fneur.2020.575975
13. Moreau, C, Rouaud, T, Grabli, D, Benatru, I, Remy, P, Marques, AR, et al. Overview on wearable sensors for the management of Parkinson’s disease. NPJ Parkinsons Dis. (2023) 9:153. doi: 10.1038/s41531-023-00585-y
14. Timpka, J, Löhle, M, Bremer, A, Christiansson, S, Gandor, F, Ebersbach, G, et al. Objective observer vs. patient motor state assessments using the PD home diary in advanced Parkinson’s disease. Front Neurol. (2022) 13:935664. doi: 10.3389/fneur.2022.935664
15. Janz, C, Timpka, J, Löhle, M, Bremer, A, Gandor, F, Ebersbach, G, et al. Agreement between Parkinson disease home diary and observer assessments before and after structured patient training. Acta Neurol Scand. (2023) 2023:1–10. doi: 10.1155/2023/8667591
16. Brognara, L, Palumbo, P, Grimm, B, and Palmerini, L. Assessing gait in Parkinson’s disease using wearable motion sensors: a systematic review. Diseases. (2019) 7:18. doi: 10.3390/diseases7010018
17. Li, F, Shirahama, K, Nisar, M, Köping, L, and Grzegorzek, M. Comparison of feature learning methods for human activity recognition using wearable sensors. Sensors. (2018) 18:679. doi: 10.3390/s18020679
18. Devices for remote monitoring of Parkinson’s disease. Diagnostics guidance [DG51]. National Institute for Health and Care Excellence (NICE). Devices for remote monitoring of Parkinson’s disease. Diagnostics guidance [DG51]. (2024) Available at: https://www.nice.org.uk”nice.org.uk.
19. Griffiths, RI, Kotschet, K, Arfon, S, Xu, ZM, Johnson, W, Drago, J, et al. Automated assessment of bradykinesia and dyskinesia in Parkinson’s disease. J Parkinsons Dis. (2012) 2:47–55. doi: 10.3233/JPD-2012-11071
20. Horne, MK, McGregor, S, and Bergquist, F. An objective fluctuation score for Parkinson’s disease. PLoS One. (2015) 10:e0124522. doi: 10.1371/journal.pone.0124522
21. Chen, L, Cai, G, Weng, H, Yu, J, Yang, Y, Huang, X, et al. More sensitive identification for bradykinesia compared to tremors in Parkinson’s disease based on Parkinson’s KinetiGraph (PKG). Front Aging Neurosci. (2020) 12:594701. doi: 10.3389/fnagi.2020.594701
22. Santiago, A, Langston, JW, Gandhy, R, Dhall, R, Brillman, S, Rees, L, et al. Qualitative evaluation of the personal KinetiGraphTM movement recording system in a Parkinson’s clinic. J Parkinsons Dis. (2019) 9:207–19. doi: 10.3233/JPD-181373
23. Nahab, FB, Abu-Hussain, H, and Moreno, L. Evaluation of clinical utility of the personal KinetiGraph in the Management of Parkinson Disease. Adv Parkinsons Dis. (2019) 8:42–61. doi: 10.4236/apd.2019.83005
24. Dominey, T, Kehagia, AA, Gorst, T, Pearson, E, Murphy, F, King, E, et al. Introducing the Parkinson’s KinetiGraph into routine Parkinson’s disease care: a 3-year single Centre experience. J Parkinsons Dis. (2020) 10:1827–32. doi: 10.3233/JPD-202101
25. Löhle, M, Timpka, J, Bremer, A, Khodakarami, H, Gandor, F, Horne, M, et al. Application of single wrist-wearable accelerometry for objective motor diary assessment in fluctuating Parkinson’s disease. NPJ Digit Med. (2023) 6:194. doi: 10.1038/s41746-023-00937-1
26. Kinesia objective assessment. (2021). Available at: http://glneurotech.com/kinesia/products/kinesia-360/ (Accessed September 2, 2021).
27. Mera TO Filipkowski, DE, Riley, DE, Whitney, CM, Walter, BL, Gunzler, SA, et al. Quantitative analysis of gait and balance response to deep brain stimulation in Parkinson’s disease. Gait Posture. (2013) 38:109–14. doi: 10.1016/j.gaitpost.2012.10.025
28. Heldman, DA, Filipkowski, DE, Riley, DE, Whitney, CM, Walter, BL, Gunzler, SA, et al. Automated motion sensor quantification of gait and lower extremity bradykinesia. Annu Int Conf IEEE Eng Med Biol Soc. (2012) 2012:1956–9. doi: 10.1109/EMBC.2012.6346338
29. Pulliam, CL, Burack, MA, Heldman, DA, and Giuffrida, JP Mera TO. Motion sensor dyskinesia assessment during activities of daily living. J Parkinsons Dis. (2014) 4:609–15. doi: 10.3233/JPD-140348
30. Pulliam, CL, Eichenseer, SR, Goetz, CG, Waln, O, Hunter, CB, Jankovic, J, et al. Continuous in-home monitoring of essential tremor. Parkinsonism Relat Disord. (2014) 20:37–40. doi: 10.1016/j.parkreldis.2013.09.009
31. Pulliam, CL, Heldman, DA, Brokaw, EB, Mera TO Mari, ZK, and Burack, MA. Continuous assessment of levodopa response in Parkinson’s disease using wearable motion sensors. IEEE Trans Biomed Eng. (2018) 65:159–64. doi: 10.1109/TBME.2017.2697764
32. Pahwa, R, Isaacson, SH, Torres-Russotto, D, Nahab, FB, Lynch, PM, and Kotschet, KE. Role of the personal KinetiGraph in the routine clinical assessment of Parkinson’s disease: recommendations from an expert panel. Expert Rev Neurother. (2018) 18:669–80. doi: 10.1080/14737175.2018.1503948
33. Sasaki, F, Oyama, G, Sekimoto, S, Nuermaimaiti, M, Iwamuro, H, Shimo, Y, et al. Closed-loop programming using external responses for deep brain stimulation in Parkinson’s disease. Parkinsonism Relat Disord. (2021) 84:47–51. doi: 10.1016/j.parkreldis.2021.01.023
34. Tamás, G, Kelemen, A, Radics, P, Valálik, I, Heldman, D, Klivényi, P, et al. Effect of subthalamic stimulation on distal and proximal upper limb movements in Parkinson’s disease. Brain Res. (2016) 1648:438–44. doi: 10.1016/j.brainres.2016.08.019
35. Tzallas, AT, Tsipouras, MG, Rigas, G, Tsalikakis, DG, Karvounis, EC, Chondrogiorgi, M, et al. Perform: a system for monitoring, assessment and management of patients with Parkinson’s disease. Sensors (Switzerland). (2014) 14:21329–57. doi: 10.3390/s141121329
36. Cancela, J, Pastorino, M, Arredondo, MT, and Hurtado, O. A telehealth system for Parkinson’s disease remote monitoring. The PERFORM approach. Annu Int Conf IEEE Eng Med Biol Soc. (2013) 2013:7492–5. doi: 10.1109/EMBC.2013.6611291
37. Rigas, G, Tzallas, AT, Tsipouras, MG, Bougia, P, Tripoliti, EE, Baga, D, et al. Assessment of tremor activity in the Parkinson’s disease using a set of wearable sensors. IEEE Trans Inf Technol Biomed. (2012) 16:478–87. doi: 10.1109/TITB.2011.2182616
38. Tsipouras, MG, Tzallas, AT, Rigas, G, Tsouli, S, Fotiadis, DI, and Konitsiotis, S. An automated methodology for levodopa-induced dyskinesia: assessment based on gyroscope and accelerometer signals. Artif Intell Med. (2012) 55:127–35. doi: 10.1016/j.artmed.2012.03.003
39. Pastorino, M, Cancela, J, Arredondo, MT, Pansera, M, Pastor-Sanz, L, Villagra, F, et al. Assessment of bradykinesia in Parkinson’s disease patients through a multi-parametric system. Annu Int Conf IEEE Eng Med Biol Soc. 2011, 2011:1810–3. doi: 10.1109/IEMBS.2011.6090516
40. Tripoliti, EE, Tzallas, AT, Tsipouras, MG, Rigas, G, Bougia, P, Leontiou, M, et al. Automatic detection of freezing of gait events in patients with Parkinson’s disease. Comput Methods Prog Biomed. (2013) 110:12–26. doi: 10.1016/j.cmpb.2012.10.016
41. Kanellos, FS, Tsamis, KI, Rigas, G, Simos, YV, Katsenos, AP, Kartsakalis, G, et al. Clinical evaluation in Parkinson’s disease: is the Golden standard shiny enough? Sensors. (2023) 23:3807. doi: 10.3390/s23083807
42. Tsamis, K, Rigas, G, and Nikolaos, K. Fotiadis Dimitrios, Konitsiotis S. Accurate monitoring of Parkinson’s disease symptoms with a wearable device during COVID-19 pandemic. In Vivo. (2021) 35:2327–30. doi: 10.21873/invivo.12507
43. Antonini, A, Reichmann, H, Gentile, G, Garon, M, Tedesco, C, Frank, A, et al. Toward objective monitoring of Parkinson’s disease motor symptoms using a wearable device: wearability and performance evaluation of PDMonitor®. Front Neurol. (2023) 14:1080752. doi: 10.3389/fneur.2023.1080752
44. Rodríguez-Martín, D, Cabestany, J, Pérez-López, C, Pie, M, Calvet, J, Samà, A, et al. A new paradigm in Parkinson’s disease evaluation with wearable medical devices: a review of STAT-ONTM. Front Neurol. (2022) 13:912343. doi: 10.3389/fneur.2022.912343
45. The REMPARK project (Personal Health Device for the Remote and Autonomous Management of Parkinson’s Disease), funded under the European Union’s Seventh Framework Programme (FP7), was coordinated by Joan Cabestany from the Universitat Politècnica de Catalunya (UPC)e. (2011–2014) Barcelona, Spain. Available at: https://www.upc.edu”www.upc.edu.
46. Cabestany, J, and Bayés, À. Parkinson’s disease management through ICT: the REMPARK approach. Delft, The Netherlands: River Publishers (2017).
47. Samà, A, Pérez-López, C, Rodríguez-Martín, D, Català, A, Moreno-Aróstegui, JM, Cabestany, J, et al. Estimating bradykinesia severity in Parkinson’s disease by analysing gait through a waist-worn sensor. Comput Biol Med. (2017) 84:114–23. doi: 10.1016/j.compbiomed.2017.03.020
48. Samà, A, Perez-Lopez, C, Romagosa, J, Rodriguez-Martin, D, Català, A, Cabestany, J, et al. Dyskinesia and motor state detection in Parkinson’ s disease patients with a single movement sensor. Annu Int Conf IEEE Eng Med Biol Soc. (2012) 2012:1194–7. doi: 10.1109/EMBC.2012.6346150
49. Pérez-López, C, Samà, A, Rodríguez-Martín, D, Moreno-Aróstegui, JM, Cabestany, J, Bayes, A, et al. Dopaminergic-induced dyskinesia assessment based on a single belt-worn accelerometer. Artif Intell Med. (2016) 67:47–56. doi: 10.1016/j.artmed.2016.01.001
50. Rodríguez-Martín, D, Samà, A, Pérez-López, C, Català, A, Arostegui, JMM, Cabestany, J, et al. Home detection of freezing of gait using support vector machines through a single waist-worn triaxial accelerometer. PLoS One. (2017) 12:e0171764. doi: 10.1371/journal.pone.0171764
51. Samà, A, Rodríguez-Martín, D, Pérez-López, C, Català, A, Alcaine, S, Mestre, B, et al. Determining the optimal features in freezing of gait detection through a single waist accelerometer in home environments. Pattern Recogn Lett. (2018) 105:135–43. doi: 10.1016/j.patrec.2017.05.009
52. Sayeed, T, Samà, A, Català, A, Rodríguez-Molinero, A, and Cabestany, J. Adapted step length estimators for patients with Parkinson’s disease using a lateral belt worn accelerometer. Technol Health Care. (2015) 23:179–94. doi: 10.3233/THC-140882
53. Cabestany, J, Moreno, JM, Perez, C, Sama, A, and Catala, A. FATE: one step towards an automatic aging people fall detection service. 20th International Conference on Mixed Design of Integrated Circuits and Systems. (2013). Available at: https://ieeexplore.ieee.org/document/6613414
54. Pérez-López, C, Samà, A, Rodríguez-Martín, D, Català, A, Cabestany, J, Moreno-Arostegui, J, et al. Assessing motor fluctuations in Parkinson’s disease patients based on a single inertial sensor. Sensors. (2016) 16:2132. doi: 10.3390/s16122132
55. Rodríguez-Molinero, A, Samà, A, Pérez-Martínez, DA, Pérez López, C, Romagosa, J, Bayés, À, et al. Validation of a portable device for mapping motor and gait disturbances in Parkinson’s disease. JMIR Mhealth Uhealth. (2015) 3:e9. doi: 10.2196/mhealth.3321
56. Bayés, À, Samá, A, Prats, A, Pérez-López, C, Crespo-Maraver, M, Moreno, JM, et al. A “HOLTER” for Parkinson’s disease: validation of the ability to detect on-off states using the REMPARK system. Gait Posture. (2018) 59:1–6. doi: 10.1016/j.gaitpost.2017.09.031
57. Rodríguez-Molinero, A, Samà, A, Pérez-López, C, Rodríguez-Martín, D, Alcaine, S, Mestre, B, et al. Analysis of correlation between an accelerometer-based algorithm for detecting parkinsonian gait and UPDRS subscales. Front Neurol. (2017) 8:3–8. doi: 10.3389/fneur.2017.00431
58. Zampogna, A, Borzì, L, Rinaldi, D, Artusi, CA, Imbalzano, G, Patera, M, et al. Unveiling the unpredictable in Parkinson’s disease: sensor-based monitoring of Dyskinesias and freezing of gait in daily life. Bioengineering. (2024) 11:440. doi: 10.3390/bioengineering11050440
59. Cabo-Lopez, I, Puy-Nuñez, A, Redondo-Rafales, N, Teixeira Baltazar, S, and Calderón-Cruz, B. Holter STAT-ON™ against other tools for detecting MF in advanced Parkinson’s disease: an observational study. Front Neurol. (2023) 14:1249385. doi: 10.3389/fneur.2023.1249385
60. Pérez-López, C, Hernández-Vara, J, Caballol, N, Bayes, À, Buongiorno, M, Lopez-Ariztegui, N, et al. Comparison of the results of a Parkinson’s Holter monitor with patient diaries, in real conditions of use: a sub-analysis of the MoMoPa-EC clinical trial. Front Neurol. (2022) 13:835249. doi: 10.3389/fneur.2022.835249
61. Buhrmester, V, Münch, D, and Arens, M. Analysis of explainers of black box deep neural networks for computer vision: a survey. Mach Learn Knowl Extr. (2021) 3:966–89. doi: 10.3390/make3040048
62. Battista, L, Casali, M, Brusa, L, Radicati, FG, and Stocchi, F. Clinical assessment of a new wearable tool for continuous and objective recording of motor fluctuations and ON/OFF states in patients with Parkinson’s disease. PLoS One. (2023) 18:e0287139. doi: 10.1371/journal.pone.0287139
63. Battista, L, and Romaniello, A. A wearable tool for continuous monitoring of movement disorders: clinical assessment and comparison with tremor scores. Neurol Sci. (2021) 42:4241–8. doi: 10.1007/s10072-021-05120-6
64. Battista, L, and Romaniello, A. A wearable tool for selective and continuous monitoring of tremor and dyskinesia in parkinsonian patients. Parkinsonism Relat Disord. (2020) 77:43–7. doi: 10.1016/j.parkreldis.2020.06.020
65. Mostovov, A, Jacobs, D, Farid, L, Dhellin, P, and Baille, G. Validation of the six-minute walking distance measured by FeetMe® insoles. BMC Digit Health. (2023) 1:48. doi: 10.1186/s44247-023-00038-9
66. Parati, M, Gallotta, M, Muletti, M, Pirola, A, Bellafà, A, De Maria, B, et al. Validation of pressure-sensing insoles in patients with Parkinson’s disease during Overground walking in single and cognitive dual-task conditions. Sensors. (2022) 22:6392. doi: 10.3390/s22176392
67. Farid, L, Jacobs, D, Do Santos, J, Simon, O, Gracies, J-M, and Hutin, E. FeetMe® monitor-connected insoles are a valid and reliable alternative for the evaluation of gait speed after stroke. Top Stroke Rehabil. (2021) 28:127–34. doi: 10.1080/10749357.2020.1792717
68. David, R, Billot, M, Delaire, L, Roulaud, M, Lorgeoux, B, Foucault, P, et al. Use of FeetMe monitor® connected soles for real-time measurement of spatial and temporal walking parameters: an illustrative case from the REWALK pilot study highlighting the objectivity of a spastic hemiparetic patient assessment. Comput Methods Biomech Biomed Eng. (2019) 22:S257–9. doi: 10.1080/10255842.2020.1714264
69. Granja Domínguez, A, Romero Sevilla, R, Alemán, A, Durán, C, Hochsprung, A, Navarro, G, et al. Study for the validation of the FeetMe® integrated sensor insole system compared to GAITRite® system to assess gait characteristics in patients with multiple sclerosis. PLoS One. (2023) 18:e0272596. doi: 10.1371/journal.pone.0272596
70. Farid, L, Jacobs, D, Moreau, C, Baille, G, and Jacobs, S. Évaluation à domicile de la marche chez les patients parkinsoniens à l’aide de semelles connectées. Rev Epidemiol Sante Publique. (2020) 68:S79. doi: 10.1016/j.respe.2020.04.032
71. Jacobs, D, Farid, L, Ferré, S, Herraez, K, Gracies, J-M, and Hutin, E. Evaluation of the validity and reliability of connected insoles to measure gait parameters in healthy adults. Sensors. (2021) 21:6543. doi: 10.3390/s21196543
72. Wu, J, Kuruvithadam, K, Schaer, A, Stoneham, R, Chatzipirpiridis, G, Easthope, CA, et al. An intelligent in-shoe system for gait monitoring and analysis with optimized sampling and real-time visualization capabilities. Sensors. (2021) 21:2869. doi: 10.3390/s21082869
73. Madgwick, S. O. H. An efficient orientation filter for inertial and inertial/magnetic sensor arrays. Bristol: University of Bristol. (2010).
74. Wu, J, Maurenbrecher, H, Schaer, A, Becsek, B, Awai Easthope, C, Chatzipirpiridis, G, et al. Human gait-labeling uncertainty and a hybrid model for gait segmentation. Front Neurosci. (2022) 16:976594. doi: 10.3389/fnins.2022.976594
75. Wu, J, Becsek, B, Schaer, A, Maurenbrecher, H, Chatzipirpiridis, G, Ergeneman, O, et al. Real-time gait phase detection on wearable devices for real-world free-living gait. IEEE J Biomed Health Inform. (2023) 27:1295–306. doi: 10.1109/JBHI.2022.3228329
76. Magnes, AG. Nushu shoes webpage. Available at: https://www.magnes.ch/solutions/nushu/ (2024) (Accessed December 15, 2024).
77. Powers, R, Etezadi-Amoli, M, Arnold, EM, Kianian, S, Mance, I, Gibiansky, M, et al. Smartwatch inertial sensors continuously monitor real-world motor fluctuations in Parkinson’s disease. Sci Transl Med. (2021) 13:eabd7865. doi: 10.1126/scitranslmed.abd7865
78. Bloem, BR, Post, E, and Hall, D. An apple a day to keep the Parkinson’s disease doctor away? Ann Neurol. (2023) 93:681–5. doi: 10.1002/ana.26612
79. Nieuwboer, A, Dom, R, De Weerdt, W, Desloovere, K, Janssens, L, and Stijn, V. Electromyographic profiles of gait prior to onset of freezing episodes in patients with Parkinson’s disease. Brain. (2004) 127:1650–60. doi: 10.1093/brain/awh189
80. Rissanen, S. Analysis of surface EMG signal morphology in Parkinson’s disease. Physiol Meas. (2007) 28:1507–21. doi: 10.1088/0967-3334/28/12/005
81. Meigal, AY, Rissanen, SM, Zaripova, YR, Miroshnichenko, GG, and Karjalainen, P. Nonlinear parameters of surface electromyogram for diagnostics of neuromuscular disorders and normal conditions of the human motor system. Hum Physiol. (2015) 41:672–9. doi: 10.1134/S0362119715050102
82. Meigal, AY, Rissanen, SM, Tarvainen, MP, Airaksinen, O, Kankaanpää, M, and Karjalainen, PA. Non-linear EMG parameters for differential and early diagnostics of Parkinson’s disease. Front Neurol. (2013) 4:135. doi: 10.3389/fneur.2013.00135
83. Ruonala, V, Meigal, A, Rissanen, SM, Airaksinen, O, Kankaanpää, M, and Karjalainen, PA. EMG signal morphology and kinematic parameters in essential tremor and Parkinson’s disease patients. J Electromyogr Kinesiol. (2014) 24:300–6. doi: 10.1016/j.jelekin.2013.12.007
84. Rissanen, SM, Koivu, M, Hartikainen, P, and Pekkonen, E. Ambulatory surface electromyography with accelerometry for evaluating daily motor fluctuations in Parkinson’s disease. Clin Neurophysiol. (2021) 132:469–79. doi: 10.1016/j.clinph.2020.11.039
85. Adamant Health. Adamant health. Available at: https://adamanthealth.com/ (2024) (Accessed December 15, 2024).
86. Meigal, AY, Rissanen, SM, Tarvainen, MP, Ruonala, V, Airaksinen, O, Kankaanpää, M, et al. Novel sEMG parameters for early diagnostics of neurological diseases and aging. J Biomed Technol. (2014) 1:2–9. doi: 10.15393/j6.art.2014.3041
87. Rissanen, SM, Ruonala, V, Pekkonen, E, Kankaanpää, M, Airaksinen, O, and Karjalainen, PA. Signal features of surface electromyography in advanced Parkinson’s disease during different settings of deep brain stimulation. Clin Neurophysiol. (2015) 126:2290–8. doi: 10.1016/j.clinph.2015.01.021
88. Miroshnichenko, GG, Meigal, AY, Saenko, IV, Gerasimova-Meigal, LI, Chernikova, LA, Subbotina, NS, et al. Parameters of surface electromyogram suggest that dry immersion relieves motor symptoms in patients with parkinsonism. Front Neurosci. (2018) 12:667. doi: 10.3389/fnins.2018.00667
89. Paragit Solutions. Paragit solutions. Available at: https://www.paragit.com/ (2024) (Accessed December 15, 2024).
90. Cantú, H, Nantel, J, Millán, M, Paquette, C, and Côté, JN. Abnormal muscle activity and variability before, during, and after the occurrence of freezing in Parkinson’s disease. Front Neurol. (2019) 10:951. doi: 10.3389/fneur.2019.00951
91. Kugler, P, Jaremenko, C, Schlachetzki, J, Winkler, J, Klucken, J, and Eskofier, B. Automatic recognition of Parkinson’s disease using surface electromyography during standardized gait tests. Annu Int Conf IEEE Eng Med Biol Soc. (2013) 2013:5781–4. doi: 10.1109/EMBC.2013.6610865
92. Bailey, CA, Corona, F, Murgia, M, Pili, R, Pau, M, and Côté, JN. Electromyographical gait characteristics in Parkinson’s disease: effects of combined physical therapy and rhythmic auditory stimulation. Front Neurol. (2018) 9:211. doi: 10.3389/fneur.2018.00211
93. Haufe, S, Isaias, IU, Pellegrini, F, and Palmisano, C. Gait event prediction using surface electromyography in parkinsonian patients. Bioengineering. (2023) 10:212. doi: 10.3390/bioengineering10020212
94. Pacini Panebianco, G, Ferrazzoli, D, Frazzitta, G, Fonsato, M, Bisi, MC, Fantozzi, S, et al. A statistical approach for the assessment of muscle activation patterns during gait in Parkinson’s disease. Electronics (Basel). (2020) 9:1641. doi: 10.3390/electronics9101641
95. Podsiadlo, D, and Richardson, S. The timed “up & go”: a test of basic functional mobility for frail elderly persons. J Am Geriatr Soc. (1991) 39:142–8. doi: 10.1111/j.1532-5415.1991.tb01616.x
96. Mancini, M, and Horak, FB. Potential of APDM mobility lab for the monitoring of the progression of Parkinson’s disease. Expert Rev Med Devices. (2016) 13:455–62. doi: 10.1586/17434440.2016.1153421
97. Dewey, DC, Chitnis, S, McCreary, MC, Gerald, A, Dewey, CH, Pantelyat, A, et al. APDM gait and balance measures fail to predict symptom progression rate in Parkinson’s disease. Front Neurol. (2022) 13:1041014. doi: 10.3389/fneur.2022.1041014
98. Pal, G, O’Keefe, J, Robertson-Dick, E, Bernard, B, Anderson, S, and Hall, D. Global cognitive function and processing speed are associated with gait and balance dysfunction in Parkinson’s disease. J Neuroeng Rehabil. (2016) 13:94. doi: 10.1186/s12984-016-0205-y
99. Lones, MA, Alty, JE, Cosgrove, J, Duggan-Carter, P, Jamieson, S, Naylor, RF, et al. A new evolutionary algorithm-based home monitoring device for Parkinson’s dyskinesia. J Med Syst. (2017) 41:176. doi: 10.1007/s10916-017-0811-7
100. Lones, MA, Alty, JE, Duggan-Carter, P, Turner, AJ, DRS, Jamieson, and Smith, SL. Classification and characterisation of movement patterns during levodopa therapy for parkinson’s disease. Proceedings of the Companion Publication of the 2014 Annual Conference on Genetic and Evolutionary Computation. New York, NY, USA: ACM (2014). p. 1321–1328.
101. McRoberts products. (2024). Available at: http://www.mcroberts.nl/products (accessed October 17, 2016).
102. Hill, EJ, Mangleburg, CG, Alfradique-Dunham, I, Ripperger, B, Stillwell, A, Saade, H, et al. Quantitative mobility measures complement the MDS-UPDRS for characterization of Parkinson’s disease heterogeneity. Parkinsonism Relat Disord. (2021) 84:105–11. doi: 10.1016/j.parkreldis.2021.02.006
103. Rodríguez-Molinero, A, Pérez-López, C, Samà, A, Rodríguez-Martín, D, Alcaine, S, Mestre, B, et al. Estimating dyskinesia severity in Parkinson’s disease by using a waist-worn sensor: concurrent validity study. Sci Rep. (2019) 9:13434. doi: 10.1038/s41598-019-49798-3
104. Grahn, F. Evaluation of two commercial sensor systems for monitoring parkinsonism and their possible influence on management of Parkinson’s disease. [Degree Project in Medicine]. Gothenburg: Institute of Neuroscience and Physiology Sahlgrenska Academy University of Gothenburg. (2022). 1–51. Available at: http://hdl.handle.net/2077/70780 (Accessed December 15, 2024).
105. Kilincalp, G, Grahn, F, Sabir, H, von Below, D, Jeppsson, A, Sjöström, A, et al. Comparision of objective Parkinson’s disease monitoring systems and the interpretations of results, vol. 38 Copenhagen, Denmark: International Congress of Parkinson’s Disease and Movement Disorders (2023).
106. Kluge, F, Brand, YE, Micó-Amigo, ME, Bertuletti, S, D’Ascanio, I, Gazit, E, et al. Real-world gait detection using a wrist-worn inertial sensor: validation study. JMIR Form Res. (2024) 8:e50035. doi: 10.2196/50035
107. Gjoreski, M, Gjoreski, H, Luštrek, M, and Gams, M. How accurately can your wrist device recognize daily activities and detect falls? Sensors. (2016) 16:800. doi: 10.3390/s16060800
108. Shcherbina, A, Mattsson, C, Waggott, D, Salisbury, H, Christle, J, Hastie, T, et al. Accuracy in wrist-worn, sensor-based measurements of heart rate and energy expenditure in a diverse cohort. J Pers Med. (2017) 7:3. doi: 10.3390/jpm7020003
109. Kondama Reddy, R, Pooni, R, Zaharieva, DP, Senf, B, El Youssef, J, Dassau, E, et al. Accuracy of wrist-worn activity monitors during common daily physical activities and types of structured exercise: evaluation study. JMIR Mhealth Uhealth. (2018) 6:e10338. doi: 10.2196/10338
110. Kim, DW, Hassett, LM, Nguy, V, and Allen, NE. A comparison of activity monitor data from devices worn on the wrist and the waist in people with Parkinson’s disease. Mov Disord Clin Pract. (2019) 6:693–9. doi: 10.1002/mdc3.12850
111. Gall, N, Sun, R, and Smuck, M. A comparison of wrist- versus hip-worn ActiGraph sensors for assessing physical activity in adults: a systematic review. J Meas Phys Behav. (2022) 5:252–62. doi: 10.1123/jmpb.2021-0045
112. Gjoreski, H, Lustrek, M, and Gams, M. Accelerometer placement for posture recognition and fall detection. 2011 Seventh International Conference on Intelligent Environments. Nottingham, United Kingdom: IEEE (2011). p. 47–54.
113. Rodríguez-Martín, D, Samà, A, Pérez-López, C, Català, A, and Cabestany, J. Posture transition analysis with barometers: contribution to accelerometer-based algorithms. Neural Comput Appl. (2019) 32, 335–349. doi: 10.1007/s00521-018-3759-8
114. Channa, A, Popescu, N, and Ciobanu, V. Wearable solutions for patients with Parkinson’s disease and neurocognitive disorder: a systematic review. Sensors. (2020) 20:2713. doi: 10.3390/s20092713
115. Zampogna, A, Manoni, A, Asci, F, Liguori, C, Irrera, F, and Suppa, A. Shedding light on nocturnal movements in parkinson’s disease: evidence from wearable technologies. Sensors (Switzerland). (2020) 20:5171. doi: 10.3390/s20185171
116. Klingelhoefer, L, Rizos, A, Sauerbier, A, McGregor, S, Martinez-Martin, P, Reichmann, H, et al. Night-time sleep in Parkinson’s disease – the potential use of Parkinson’s KinetiGraph: a prospective comparative study. Eur J Neurol. (2016) 23:1275–88. doi: 10.1111/ene.13015
117. Espay, AJ, Bonato, P, Nahab, FB, Maetzler, W, Dean, JM, Klucken, J, et al. Technology in Parkinson’s disease: challenges and opportunities. Mov Disord. (2016) 31:1272–82. doi: 10.1002/mds.26642
118. Janssen Daalen, JM, van den Bergh, R, Prins, EM, Moghadam, MSC, van den Heuvel, R, Veen, J, et al. Digital biomarkers for non-motor symptoms in Parkinson’s disease: the state of the art. NPJ Digit Med. (2024) 7:186. doi: 10.1038/s41746-024-01144-2
119. Mazilu, S, Calatroni, A, Gazit, E, Mirelman, A, Hausdorff, JM, and Troster, G. Prediction of freezing of gait in Parkinson’s from physiological wearables: an exploratory study. IEEE J Biomed Health Inform. (2015) 19:1843–54. doi: 10.1109/JBHI.2015.2465134
Keywords: wearables, motor symptoms, early detection, medical devices, objective evaluation
Citation: Rodríguez-Martín D and Pérez-López C (2024) Commercial symptom monitoring devices in Parkinson’s disease: benefits, limitations, and trends. Front. Neurol. 15:1470928. doi: 10.3389/fneur.2024.1470928
Received: 26 July 2024; Accepted: 09 December 2024;
Published: 23 December 2024.
Edited by:
Sandra Neumann, University College, Bristol, United KingdomReviewed by:
Stefania Roxana Diaconu, Transilvania University of Brașov, RomaniaCopyright © 2024 Rodríguez-Martín and Pérez-López. This is an open-access article distributed under the terms of the Creative Commons Attribution License (CC BY). The use, distribution or reproduction in other forums is permitted, provided the original author(s) and the copyright owner(s) are credited and that the original publication in this journal is cited, in accordance with accepted academic practice. No use, distribution or reproduction is permitted which does not comply with these terms.
*Correspondence: Carlos Pérez-López, Y3BlcmV6bEBjc2FwZy5jYXQ=
†These authors have contributed equally to this work and share first authorship
Disclaimer: All claims expressed in this article are solely those of the authors and do not necessarily represent those of their affiliated organizations, or those of the publisher, the editors and the reviewers. Any product that may be evaluated in this article or claim that may be made by its manufacturer is not guaranteed or endorsed by the publisher.
Research integrity at Frontiers
Learn more about the work of our research integrity team to safeguard the quality of each article we publish.