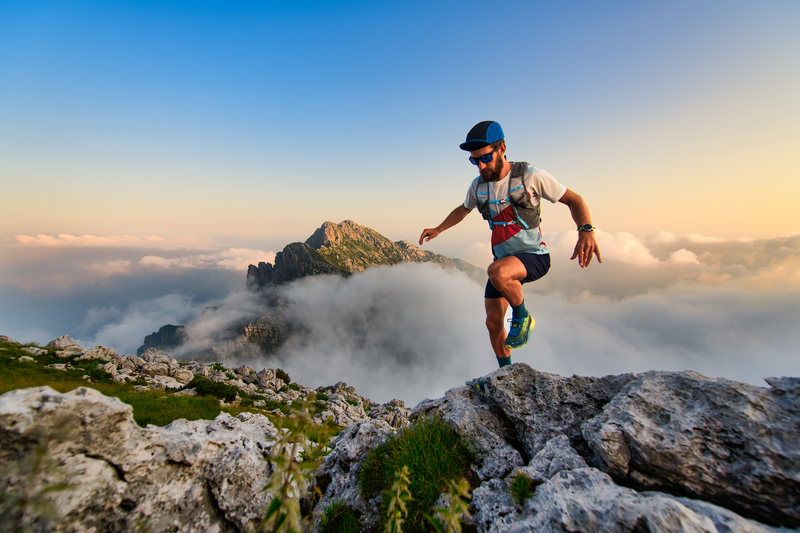
94% of researchers rate our articles as excellent or good
Learn more about the work of our research integrity team to safeguard the quality of each article we publish.
Find out more
ORIGINAL RESEARCH article
Front. Neurol. , 10 April 2024
Sec. Movement Disorders
Volume 15 - 2024 | https://doi.org/10.3389/fneur.2024.1356042
This article is part of the Research Topic Remote monitoring technologies in neurodegenerative movement disorders View all 6 articles
Introduction: In the advanced stages of Parkinson’s disease (PD), motor complications such as wearing-off and dyskinesia are problematic and vary daily. These symptoms need to be monitored precisely to provide adequate care for patients with advanced PD.
Methods: This study used wearable devices to explore biomarkers for motor complications by measuring multiple biomarkers in patients with PD residing in facilities and combining them with lifestyle and clinical assessments. Data on the pulse rate and activity index (metabolic equivalents) were collected from 12 patients over 30 days.
Results: The pulse rate and activity index during the off- and on-periods and dyskinesia were analyzed for two participants; the pulse rate and activity index did not show any particular trend in each participant; however, the pulse rate/activity index was significantly greater in the off-state compared to that in the dyskinesia and on-states, and this index in the dyskinesia state was significantly greater than that in the on-state in both participants.
Conclusion: These results suggest the pulse rate and activity index combination would be a useful indicator of wearing-off and dyskinesia and that biometric information from wearable devices may function as a digital diary. Accumulating more cases and collecting additional data are necessary to verify our findings.
Parkinson’s disease (PD) is a progressive neurodegenerative disease that can be effectively treated with levodopa during its early stages (1). However, treatment becomes challenging as the disease advances because of the emergence of motor complications such as wearing-off and levodopa-induced dyskinesia (2). In cases with medically refractory motor complications, device-aided therapies are options, including deep brain stimulation (DBS), levodopa-carbidopa intestinal gel infusion, and subcutaneous drug infusions. Even after device-aided therapies, motor complications may re-emerge over time (3). Given these challenges, continuous and objective motor complication monitoring is essential to manage PD symptoms effectively.
Wearable devices have been studied extensively for monitoring Parkinsonian symptoms (4). However, differentiating between on and off states using accelerometer-based monitoring is technically challenging when a patient is at rest. Active monitoring requires patients to perform specific tasks and can accurately detect the off-state (5). However, this method can be intrusive; maintaining adherence to these tasks presents a significant challenge. Consequently, a practical and patient-friendly method for detecting the off-state using passive monitoring with a wearable device has yet to be implemented effectively in clinical settings.
This study used multiple sensor technologies to explore biomarkers capable of detecting the off-state. Residents in elderly care facilities were monitored for 24 continuous hours to investigate the relationship between Parkinsonian symptoms and physiological biomarkers gathered from wearable devices.
The study included residents in elderly care facilities specializing in PD (PD houses, Sunwels Ltd., Tokyo). Inclusion criteria were (1) ≥20 years of age, (2) residents of a PD house (Imajuku, Nishimiyanosawa, Hakusan, and Arita), (3) previously diagnosed with PD, and (4) who provided written consent. Patients who had difficulty wearing the wearable device were excluded.
The study was conducted from March 2022 to June 2022. The participants wore a three-axis multisensory wearable device (iAide2, TOKAI corp., Gifu, Japan) on their left or right wrist for 24 h (except during charging) for >30 days. This device tracked the pulse rate and activity index. The activity index is calculated using a proprietary algorithm corresponding to metabolic equivalents (METs) (6). If fully charged, the battery life of the wearable device (iAide2) is 8 days. The participants re-charged the battery for only a few minutes each day, such as during bath time. In addition, the Nemuri-SCAN (Paramount Bed, Tokyo, Japan), a sensor sheet placed beneath the mattress, monitored their pulse rate during sleep and detected instances of getting out of bed. Data were collected every 20 s; the pulse rate and activity index were averaged every minute daily. The facility care staff recorded the timing of meals, medications, rehabilitation, and subjective off-state, on-state, and dyskinesia times. The on-state was defined as any period other than the off, dyskinesia, and sleep times, which the Nemuri-Scan detected. Rehabilitation was provided 0–7 times per week, 0–3 times per day, depending on the symptoms. Rehabilitation could not be performed for a period during the COVID-19 outbreak.
This study was approved by the Juntendo University Ethics Committee (#M20-0294-M01). All participants provided written informed consent.
Statistical analysis was performed using JMP software (Ver 16, SAS, United States). The main parameters analyzed were pulse rate and activity index. Because of the non-parametric nature of the data and the requirement for multiple comparison procedures, the Steel-Dwass test was used. The Kruskal–Wallis test was used to compare the pulse rate coefficient of variance and average pulse rate across different patients. Furthermore, the Pearson product–moment correlation coefficient was calculated to evaluate the relationship between pulse rate measurements from the Nemuri-SCAN and the iAide-2 devices. In all statistical tests, p < 0.05 was statistically significant.
The study included 12 participants with PD (6 males and 6 females) in four PD house facilities: Imajuku (n = 1), Nishimiyanosawa (n = 1), Hakusan (n = 4), and Arita (n = 3). The age at consent was 76.5 ± 8.4 years, disease duration was 9.7 ± 5.5 years, Hoehn and Yahr stage was 3.2 ± 0.4 at on and 3.9 ± 0.5 at off, Mini-Mental State Examination score was 27.1 ± 3.8, and Functional Independence Measure was 90.8 ± 11.2. The total levodopa equivalent daily dose was 645.4 ± 360.1, calculated using standard conversion factors (7).
Twelve participants completed the data collection over 30 days. Figure 1 shows the 30-day average pulse rate and activity index measured for 24 h using a wearable device. Among the 12 participants, 3 had wearing-off (Participants #4, #5, and #9), 1 had dyskinesia (Participant #3), and 2 had both wearing-off and dyskinesia periods (Participants #11 and #12).
Figure 1. Diurnal variation in pulse rate/activity and subjective symptoms of PD for each participant. The 30-day average pulse rate and activity level monitored for 24 h using a wearable device are plotted. The horizontal axis represents time, and the vertical axis represents either the pulse rate or activity level. The facility staff recorded medications, meals, and rehabilitation times. The time off or with dyskinesia is highlighted based on the patient’s subjective symptoms. Patients 1–12 are represented in the figure by panels (A–L).
The pulse rate and activity index derived from the wearable device during the off-period, the on-period, and dyskinesia, as defined by the symptom diary, were analyzed to explore digital biomarkers for wearing-off and dyskinesia in patients with PD. The analysis included 6 participants with PD who exhibited one or both of these symptoms. Pulse rates were significantly greater in the off-state than in the on and dyskinesia states. Moreover, the pulse rates in the on-state were also significantly greater than that in the dyskinesia state (Steel-Dwass test, p < 0.001). The pulse rate coefficient of variance was not significantly different between dyskinesia and the on- and off-states (Kruskal–Wallis test, p = 0.69). The activity index was significantly greater in the order of the on-state, dyskinesia, and the off-state (Steel-Dwass test, p < 0.001). The pulse rate/activity index (METs) per minute was significantly greater in the order of the off-state, the on-state, and dyskinesia (Steel-Dwass test, p < 0.001; Figure 2).
Figure 2. Biological signals of wearing-off and dyskinesia. (A) The pulse rates were significantly greater in the order of the off-state, on-state, and dyskinesia in six participants who had off- and/or dyskinesia periods (Steel-Dwass test, p < 0.001). (B) The pulse-rate coefficient variance was not significantly different between dyskinesia, the on-period, and the off-period (Kruskal–Wallis test, p = 0.69). (C) The activity index was significantly greater in the order of the on-state, dyskinesia, and the off-state (Steel-Dwass test, p < 0.001). (D) Pulse rate/activity index (METs) per minute was significantly greater in the order of the off-state, the on-state, and, dyskinesia (Steel-Dwass test, p < 0.001).
The average pulse rate differed among the patients (Kruskal–Wallis test, p < 0.001). Pulse rates were measured using multiple devices to ensure reliability, with significant correlations between the one-minute pulse rates measured simultaneously by Nemuri-SCAN and iAide-2 (Pearson product–moment correlation coefficient, r = 0.51, p < 0.001). This cross-verification reinforces the potential of these measures as digital biomarkers of wearing-off and dyskinesia in patients with PD.
We compared the pulse rate and activity index in two participants with dyskinesia and off periods for a more detailed analysis (Table 1). In both participants, the pulse rate was significantly higher in off-state than dyskinesia, and the on-state, and in comparing dyskinesia, and the on-state, the pulse rate was significantly higher in dyskinesia state than on-state for participant 12 (Steel-Dwass test, p < 0.001), while it was significantly higher during the off-state, but not significantly different between dyskinesia and the on-state for participant 11 (Steel-Dwass test, p < 0.001). The activity index (METs) was significantly higher in the on-state, but not significantly different between dyskinesia and the off-state for participant 11 (Steel-Dwass test, p < 0.001); it was significantly higher in the order of dyskinesia, the on-state, and the off-state for participant 12 (Steel-Dwass test, p < 0.001). In both participants, the pulse rate/activity index (METs) was significantly greater in the order of the off-state, dyskinesia, and the on-state (Steel-Dwass test, p < 0.005; Figure 3).
Figure 3. Comparison of the pulse rate, activity index, and pulse rate/activity index for each condition in two participants. (A) The pulse rate was significantly higher during the off-state, but not significantly different between dyskinesia and the on-state for participant 11 (Steel-Dwass test, p < 0.001). (B) The pulse rate was significantly higher in the order of the off-state, dyskinesia, and the on-state for participant 12. (C) The activity index was significantly higher in the on-state, but not significantly different between dyskinesia and the off-state for participant 11 (Steel-Dwass test, p < 0.001). (D) The activity index was significantly higher in the order of dyskinesia, the on-state, and the off-state for participant 12 (Steel-Dwass test, p < 0.001). (E,F) In both participants, the pulse rate/activity index (METs) was significantly greater in the order of the off-state, dyskinesia, and the on-state (Steel-Dwass test, p < 0.005).
Table 1. The pulse rate, activity index, and pulse rate/activity index in the in participants with both dyskinesia and off periods.
This study demonstrated that multiple wearable devices could monitor diurnal changes in patients with PD. It is difficult to distinguish the conditions of patients with PD using only the pulse rate or activity index. However, the pulse rate per activity index was significantly greater in the order of the off-state, dyskinesia, and the on-state. These results indicate that the simultaneous monitoring of biometric and motion information using an accelerometer and wearable devices provides meaningful information for the off-state and dyskinesia. This monitoring may function as a digital diary instead of a paper-based diary for evaluating a patient’s condition. Recent advances in digital and telecommunication technologies have brought innovation to medicine. The integration of artificial intelligence, next-generation communication networks, and Internet of Things is anticipated to facilitate the management of larger datasets and enable personalized and tailor-made medical care in the future (8).
Traditionally, PD treatment relies on both patient-reported conditions and physical examination findings. However, motor complications can be prominent in the advanced stages of PD; a common finding is that some patients exhibit favorable conditions during medical examinations but experience discomfort in their daily lives. Another challenge is that some patients cannot distinguish between dyskinesia and tremor or report symptoms originating from conditions unrelated to PD. These complexities make accurately monitoring the specific PD state causing their discomfort challenging. Symptom diaries are the most commonly used method for documenting motor symptom fluctuations in daily life (9). However, because of their subjective nature, symptom diaries are often inconsistent with the physician’s diagnosis (10). Therefore, developing a tool for obtaining objective information from symptom diaries is an important and unmet need for both patients and clinicians.
Methods for collecting physiological biomarkers include surface electromyography, local field potentials from DBS leads implanted in basal ganglia, electrocorticography (ECoG), and mobile health (mHealth) devices. Surface electromyography is historically used to diagnose tremors and is a reliable biomarker (11, 12). However, the methods to detect bradykinesia and rigidity have not been established (13, 14), and distinguishing between immobility and the mobile-but-resting state is still difficult by using accelerometers or wearable devices. Beta-band activity (beta oscillation) of the local field potential (LFP) recorded in the subthalamic nucleus is a promising biomarker correlated with the severity of bradykinesia and rigidity; administration of levodopa or DBS stimulation decreases beta oscillations (15–18). However, the LFP in the subthalamic nucleus has not been established to correlate with tremors or dyskinesia (19–21) and requires invasive procedures. ECoG measures brain electrical activity by placing sheet electrodes on the cortical surface. In PD, phase-amplitude coupling detected using ECoG is elevated between beta and broadband gamma (50–150 Hz) over the motor cortex and correlates with immobility and rigidity (22). Furthermore, fine-tuned gamma oscillations (60–90 Hz) in the cortex have relatively high specificity for dyskinesia (21). Although it is less invasive than DBS, it requires the implantation of subdural grids, which risks bleeding and infection.
Health management increasingly uses mHealth devices, including smartphones and wearable devices. Smartphone applications are highly popular and have advantages such as inputting patient-reported outcomes. However, they are not always worn on a specific body part and rely on subjective patient reports (23). Wearable devices using accelerometers and gyroscopes have been studied to monitor PD symptoms (24). Wearable devices are superior at detecting tremors compared to LFP (12, 25) and are expected to be effective for detecting freezing of gait, bradykinesia, and dyskinesia (26–29). Passive monitoring using wearable devices equipped with accelerometers and gyroscopes faces challenges in accurately detecting bradykinesia. Differentiating between a patient’s voluntary rest period and the symptomatic off-state period is difficult (30, 31) and requires active monitoring, which requires patients to perform specific tasks. Although biological biomarkers for a single symptom of PD are being identified, a method to monitor all symptoms of PD with a single device has not been established; therefore, treatment focusing on only a single symptom of PD will not improve the quality of life.
This study hypothesized that combining multiple biological biomarkers obtained from wearable devices could overcome these problems. The results indicate that combining pulse rate and activity level might distinguish between three states, spontaneous resting, off, and dyskinesia, representing a novel proof of concept in monitoring the off-state in PD. Indeed, Powers et al. reported that an Apple Watch can use inertial sensors to detect tremors and dyskinesia (32) but not bradykinesia. Multiple factors such as emotion and autonomic nerve systems are thought to be involved in the higher pulse rate during the off-state than any other conditions. Patients with advanced PD have a lower skin temperature, increased sweating, and higher orthostatic blood pressure in the off-state compared to those in the on-state (33). This is thought to result from the presence of autonomic failure in patients with PD (34, 35). However, because the pulse rate and other autonomic indicators are greatly influenced by emotions and behavior, it is considered difficult to use the autonomic nervous system alone as a biomarker for wearing-off (36). Anxiety and depression, major non-motor disorders, are induced during the off-state and may also affect the pulse rate elevation (37). Low physical activity during the off-period is due to bradykinesia, which is difficult to distinguish from rest, making it more challenging to determine the status of patients with PD by activity monitoring alone. To the best of our knowledge, previous studies have only investigated the representation of motor symptoms in patients with PD by using the autonomic nervous system alone or activity monitoring alone. Herein, we have shown that it is possible to describe the condition of patients with PD by combining different indices of activity monitoring and the pulse rate.
The limitations of this study include the small number of participants, its observational nature, and the possibility of underestimating exercise and stress. Furthermore, the data were collected in a homogenized environment, the same group of facilities specializing in the care of patients with PD, and clinical data were collected daily by the facilities’ staff; therefore, our results cannot be generalized to real-world situations. The wearable device was worn on any arm in this study; thus, the activity index may have changed during the movement depending on whether or not the device was worn on the dominant hand. Further analysis is needed to identify a biomarker correlating spontaneous movement with a dyskinesia state and to confirm our concept of developing digital diaries using biological biomarkers. Moreover, the decision time to distinguish the condition of the patient needs to be set for practical use. Since irregular values may be obtained due to sudden activity or stress factors, a relatively longer judgment time may more accurately represent the condition. Alternatively, real-time judgment is desired for practical application. In future research, it is necessary to search for a judgment time that balances accuracy and practicality.
This study demonstrated that the combination of biological signals and movement data obtained from wearable devices has the potential to be a biomarker for the fluctuating symptoms of PD. This approach could be instrumental in developing a digital daily tailored to PD management. However, further studies are required to validate these hypotheses. Such studies should involve collecting more comprehensive data and applying a machine-learning approach to develop a robust model that accurately corresponds to the precise condition of patients with PD.
The raw data supporting the conclusions of this article will be made available by the authors, without undue reservation.
The studies involving humans were approved by Juntendo University Ethics Committee (#M20-0294-M01). The studies were conducted in accordance with the local legislation and institutional requirements. The participants provided their written informed consent to participate in this study.
HK: Writing – review & editing, Writing – original draft, Project administration, Methodology, Formal analysis, Data curation, Conceptualization. GO: Writing – review & editing, Supervision, Methodology, Investigation, Conceptualization. YY: Writing – review & editing, Methodology, Investigation, Funding acquisition, Conceptualization. TN: Writing – review & editing, Funding acquisition. RN: Writing – review & editing, Funding acquisition. NH: Writing – review & editing.
The author(s) declare financial support was received for the research, authorship, and/or publication of this article. This work was partially supported by JSPS KAKENHI Grant Numbers 23K19409 and 21K12711. Department of Home Medical Care System, Based on Information and Communication Technology, Juntendo University Graduate School of Medicine is a collaborative research program supported by Sunwels Ltd.
YY and TN are employees of Sunwels. GO reports a relationship with Medtronic, Boston Scientific, Otsuka Pharmaceutical, Sumitomo Pharma, FP Pharmaceutical Corporation, Kyowa Hakko Kirin, Takeda Pharmaceutical Company Ltd, Eisai Co., Ltd, Mylan EPD, and Abbvie that includes: speaking and lecture fees. NH has received speaker honoraria from Sumitomo, Otsuka, Takeda, Kyowa Hakko-Kirin, Boehringer Ingelheim, FP, Eisai, Kissei, Nihon Medi-physics, Novartis, Biogen Idec Japan, AbbVie, Boston Scientific Japan, Sanofi, Alexion, Mylan N. V., and Daiichi Sankyo. NH has received consultancies and subcontracting from Sumitomo, Biogen Idec, Otsuka, Takeda, Kyowa Hakko-Kirin, Meiji Seika, Hisamitsu, Kao, and Ono. Department of Home Medical Care System, based on Information and Communication Technology, Juntendo University Graduate School of Medicine is donated course by Sunwels.
The remaining authors declare that the research was conducted in the absence of any commercial or financial relationships that could be construed as a potential conflict of interest.
The author(s) declared that they were an editorial board member of Frontiers, at the time of submission. This had no impact on the peer review process and the final decision.
All claims expressed in this article are solely those of the authors and do not necessarily represent those of their affiliated organizations, or those of the publisher, the editors and the reviewers. Any product that may be evaluated in this article, or claim that may be made by its manufacturer, is not guaranteed or endorsed by the publisher.
1. Reich, SG, and Savitt, JM. Parkinson's disease. Med Clin North Am. (2019) 103:337–50. doi: 10.1016/j.mcna.2018.10.014
2. Armstrong, MJ, and Okun, MS. Diagnosis and treatment of Parkinson disease: a review. JAMA. (2020) 323:548–60. doi: 10.1001/jama.2019.22360
3. Tanner, CM . A second honeymoon for Parkinson's disease? N Engl J Med. (2013) 368:675–6. doi: 10.1056/NEJMe1214913
4. Moreau, C, Rouaud, T, Grabli, D, Benatru, I, Remy, P, Marques, AR, et al. Overview on wearable sensors for the management of Parkinson's disease. NPJ Parkinsons Dis. (2023) 9:153. doi: 10.1038/s41531-023-00585-y
5. Oyama, G, Burq, M, Hatano, T, Marks, WJ Jr, Kapur, R, Fernandez, J, et al. Analytical and clinical validity of wearable, multi-sensor technology for assessment of motor function in patients with Parkinson's disease in Japan. Sci Rep. (2023) 13:3600. doi: 10.1038/s41598-023-29382-6
6. Miyamoto, KaHKaKMaKM . Wearable Sensors Corresponding to Various Applications in Medical/Healthcare Field. (2020). p. 115–118.
7. Nyholm, D, and Jost, WH. An updated calculator for determining levodopa-equivalent dose. Neurol Res Pract. (2021) 3:58. doi: 10.1186/s42466-021-00157-6
8. Espay, AJ, Bonato, P, Nahab, FB, Maetzler, W, Dean, JM, Klucken, J, et al. Technology in Parkinson's disease: challenges and opportunities. Mov Disord. (2016) 31:1272–82. doi: 10.1002/mds.26642
9. Hauser, RA, Friedlander, J, Zesiewicz, TA, Adler, CH, Seeberger, LC, O'Brien, CF, et al. A home diary to assess functional status in patients with Parkinson's disease with motor fluctuations and dyskinesia. Clin Neuropharmacol. (2000) 23:75–81. doi: 10.1097/00002826-200003000-00003
10. Reimer, J, Grabowski, M, Lindvall, O, and Hagell, P. Use and interpretation of on/off diaries in Parkinson's disease. J Neurol Neurosurg Psychiatry. (2004) 75:396–400. doi: 10.1136/jnnp.2003.022780
11. Scholz, E, Diener, HC, Noth, J, Friedemann, H, Dichgans, J, and Bacher, M. Medium and long latency EMG responses in leg muscles: Parkinson's disease. J Neurol Neurosurg Psychiatry. (1987) 50:66–70. doi: 10.1136/jnnp.50.1.66
12. Khobragade, N, Graupe, D, and Tuninetti, D. Towards fully automated closed-loop deep brain stimulation in Parkinson's disease patients: a LAMSTAR-based tremor predictor. Annu Int Conf IEEE Eng Med Biol Soc. (2015) 2015:2616–9. doi: 10.1109/EMBC.2015.7318928
13. Askari, S, Zhang, M, and Won, DS. An EMG-based system for continuous monitoring of clinical efficacy of Parkinson's disease treatments. Annu Int Conf IEEE Eng Med Biol Soc. (2010) 2010:98–101. doi: 10.1109/IEMBS.2010.5626133
14. Levin, J, Krafczyk, S, Valkovic, P, Eggert, T, Claassen, J, and Bötzel, K. Objective measurement of muscle rigidity in parkinsonian patients treated with subthalamic stimulation. Mov Disord. (2009) 24:57–63. doi: 10.1002/mds.22291
15. Weinberger, M, Mahant, N, Hutchison, WD, Lozano, AM, Moro, E, Hodaie, M, et al. Beta oscillatory activity in the subthalamic nucleus and its relation to dopaminergic response in Parkinson's disease. J Neurophysiol. (2006) 96:3248–56. doi: 10.1152/jn.00697.2006
16. Eusebio, A, Thevathasan, W, Doyle Gaynor, L, Pogosyan, A, Bye, E, Foltynie, T, et al. Deep brain stimulation can suppress pathological synchronisation in parkinsonian patients. J Neurol Neurosurg Psychiatry. (2011) 82:569–73. doi: 10.1136/jnnp.2010.217489
17. Rosa, M, Arlotti, M, Marceglia, S, Cogiamanian, F, Ardolino, G, Fonzo, AD, et al. Adaptive deep brain stimulation controls levodopa-induced side effects in parkinsonian patients. Mov Disord. (2017) 32:628–9. doi: 10.1002/mds.26953
18. Little, S, Pogosyan, A, Neal, S, Zavala, B, Zrinzo, L, Hariz, M, et al. Adaptive deep brain stimulation in advanced Parkinson disease. Ann Neurol. (2013) 74:449–57. doi: 10.1002/ana.23951
19. Weinberger, M, Hutchison, WD, Lozano, AM, Hodaie, M, and Dostrovsky, JO. Increased gamma oscillatory activity in the subthalamic nucleus during tremor in Parkinson's disease patients. J Neurophysiol. (2009) 101:789–802. doi: 10.1152/jn.90837.2008
20. Hirschmann, J, Özkurt, TE, Butz, M, Homburger, M, Elben, S, Hartmann, CJ, et al. Differential modulation of STN-cortical and cortico-muscular coherence by movement and levodopa in Parkinson's disease. NeuroImage. (2013) 68:203–13. doi: 10.1016/j.neuroimage.2012.11.036
21. Wiest, C, Torrecillos, F, Tinkhauser, G, Pogosyan, A, Morgante, F, Pereira, EA, et al. Finely-tuned gamma oscillations: spectral characteristics and links to dyskinesia. Exp Neurol. (2022) 351:113999. doi: 10.1016/j.expneurol.2022.113999
22. de Hemptinne, C, Swann, NC, Ostrem, JL, Ryapolova-Webb, ES, San Luciano, M, Galifianakis, NB, et al. Therapeutic deep brain stimulation reduces cortical phase-amplitude coupling in Parkinson's disease. Nat Neurosci. (2015) 18:779–86. doi: 10.1038/nn.3997
23. Zhang, H, Song, C, Rathore, AS, Huang, MC, Zhang, Y, and Xu, W. mHealth technologies towards Parkinson's disease detection and monitoring in daily life: a comprehensive review. IEEE Rev Biomed Eng. (2021) 14:71–81. doi: 10.1109/RBME.2020.2991813
24. Sánchez-Ferro, Á, Elshehabi, M, Godinho, C, Salkovic, D, Hobert, MA, Domingos, J, et al. New methods for the assessment of Parkinson's disease (2005 to 2015): a systematic review. Mov Disord. (2016) 31:1283–92. doi: 10.1002/mds.26723
25. Basu, I, Graupe, D, Tuninetti, D, Shukla, P, Slavin, KV, Metman, LV, et al. Pathological tremor prediction using surface electromyogram and acceleration: potential use in 'ON-OFF' demand driven deep brain stimulator design. J Neural Eng. (2013) 10:036019. doi: 10.1088/1741-2560/10/3/036019
26. Delrobaei, M, Baktash, N, Gilmore, G, McIsaac, K, and Jog, M. Using wearable technology to generate objective Parkinson's disease dyskinesia severity score: possibilities for home monitoring. IEEE Trans Neural Syst Rehabil Eng. (2017) 25:1853–63. doi: 10.1109/TNSRE.2017.2690578
27. Rodríguez-Martín, D, Samà, A, Pérez-López, C, Català, A, Moreno Arostegui, JM, Cabestany, J, et al. Home detection of freezing of gait using support vector machines through a single waist-worn triaxial accelerometer. PLoS One. (2017) 12:e0171764. doi: 10.1371/journal.pone.0171764
28. Griffiths, RI, Kotschet, K, Arfon, S, Xu, ZM, Johnson, W, Drago, J, et al. Automated assessment of bradykinesia and dyskinesia in Parkinson's disease. J Parkinsons Dis. (2012) 2:47–55. doi: 10.3233/JPD-2012-11071
29. Hasan, H, Athauda, DS, Foltynie, T, and Noyce, AJ. Technologies assessing limb bradykinesia in Parkinson's disease. J Parkinsons Dis. (2017) 7:65–77. doi: 10.3233/JPD-160878
30. Graupe, D, Khobragade, N, Tuninetti, D, Basu, I, Slavin, KV, and Verhagen, ML. Who may benefit from on-demand control of deep brain stimulation? Noninvasive Evaluation of Parkinson Patients. Neuromodulation. (2018) 21:611–6. doi: 10.1111/ner.12752
31. Kubota, KJ, Chen, JA, and Little, MA. Machine learning for large-scale wearable sensor data in Parkinson's disease: concepts, promises, pitfalls, and futures. Mov Disord. (2016) 31:1314–26. doi: 10.1002/mds.26693
32. Powers, R, Etezadi-Amoli, M, Arnold, EM, Kianian, S, Mance, I, Gibiansky, M, et al. Smartwatch inertial sensors continuously monitor real-world motor fluctuations in Parkinson's disease. Sci Transl Med. (2021) 13:7865. doi: 10.1126/scitranslmed.abd7865
33. Pursiainen, V, Haapaniemi, TH, Korpelainen, JT, Sotaniemi, KA, and Myllylä, VV. Sweating in parkinsonian patients with wearing-off. Mov Disord. (2007) 22:828–32. doi: 10.1002/mds.21422
34. Esen, F, Celebi, G, Ertekin, C, and Colakoglu, Z. Electrodermal activity in patients with Parkinson's disease. Clin Auton Res. (1997) 7:35–40. doi: 10.1007/BF02267624
35. Suzuki, M, Nakamura, T, Hirayama, M, Ueda, M, Katsuno, M, and Sobue, G. Cardiac parasympathetic dysfunction in the early phase of Parkinson's disease. J Neurol. (2017) 264:333–40. doi: 10.1007/s00415-016-8348-0
36. Arasteh, E, Mirian, MS, Verchere, WD, Surathi, P, Nene, D, Allahdadian, S, et al. An Individualized multi-modal approach for detection of medication "off" episodes in Parkinson's disease via wearable sensors. J Pers Med. (2023) 13:265. doi: 10.3390/jpm13020265
Keywords: Parkinson’s disease, wearable device, physiological biomarker, heart rate, wearing off, dyskinesia
Citation: Kamo H, Oyama G, Yamasaki Y, Nagayama T, Nawashiro R and Hattori N (2024) A proof of concept: digital diary using 24-hour monitoring using wearable device for patients with Parkinson’s disease in nursing homes. Front. Neurol. 15:1356042. doi: 10.3389/fneur.2024.1356042
Received: 14 December 2023; Accepted: 26 February 2024;
Published: 10 April 2024.
Edited by:
Pramod Kumar Pal, National Institute of Mental Health and Neurosciences (NIMHANS), IndiaReviewed by:
Vikram Venkappayya Holla, National Institute of Mental Health and Neurosciences, IndiaCopyright © 2024 Kamo, Oyama, Yamasaki, Nagayama, Nawashiro and Hattori. This is an open-access article distributed under the terms of the Creative Commons Attribution License (CC BY). The use, distribution or reproduction in other forums is permitted, provided the original author(s) and the copyright owner(s) are credited and that the original publication in this journal is cited, in accordance with accepted academic practice. No use, distribution or reproduction is permitted which does not comply with these terms.
*Correspondence: Genko Oyama, Z19veWFtYUBqdW50ZW5kby5hYy5qcA==
Disclaimer: All claims expressed in this article are solely those of the authors and do not necessarily represent those of their affiliated organizations, or those of the publisher, the editors and the reviewers. Any product that may be evaluated in this article or claim that may be made by its manufacturer is not guaranteed or endorsed by the publisher.
Research integrity at Frontiers
Learn more about the work of our research integrity team to safeguard the quality of each article we publish.