- Division of Nephrology, Department of Medicine, Stony Brook University Hospital, , Stony Brook, NY, United States
The COVID-19 pandemic resulted in an unprecedented burden on intensive care units (ICUs). With increased demands and limited supply, critical care resources, including dialysis machines, became scarce, leading to the undertaking of value-based cost-effectiveness analyses and the rationing of resources to deliver patient care of the highest quality. A high proportion of COVID-19 patients admitted to the ICU required dialysis, resulting in a major burden on resources such as dialysis machines, nursing staff, technicians, and consumables such as dialysis filters and solutions and anticoagulation medications. Artificial intelligence (AI)-based big data analytics are now being utilized in multiple data-driven healthcare services, including the optimization of healthcare system utilization. Numerous factors can impact dialysis resource allocation to critically ill patients, especially during public health emergencies, but currently, resource allocation is determined using a small number of traditional factors. Smart analytics that take into account all the relevant healthcare information in the hospital system and patient outcomes can lead to improved resource allocation, cost-effectiveness, and quality of care. In this review, we discuss dialysis resource utilization in critical care, the impact of the COVID-19 pandemic, and how AI can improve resource utilization in future public health emergencies. Research in this area should be an important priority.
1 Introduction
In the United States, the cost of an intensive care unit (ICU) stay is approximately $4,300 per day, and the total annual critical care cost is estimated to be approximately $108 billion, which is 13.2% of total hospital costs and 4.1% of the national health expenditure (1). The past decade has seen an increasing demand for critical care due to longer life expectancy, greater comorbidity burden, and better survival rates in the ICU (1–4). “Access to critical care” is defined based on the patient’s capacity to benefit from the available resources (5). Often the sickest patients admitted to the ICU have the highest capacity to benefit in terms of reduction in mortality risk, making admissions of these patients more cost-effective (measured by the cost per life-year saved) than those of patients with a lower risk of death (6). Due to the increasing demands and limited resources and budgets, critical care has become a scarce resource, leading to the undertaking of value-based cost-effectiveness analysis (CEA) and resource rationing to achieve the most efficient and effective patient care (2).
The resource utilization policies in the ICU should be guided not just by resources, but also by the goals of equity of access and prevention of both short- and long-term morbidity and mortality (2). Although clinician judgment can be effective at identifying the patients who would benefit most, very large volumes of valuable real-time ICU data are underutilized. Artificial intelligence (AI)-based data analytics are being utilized in multiple data-driven healthcare services such as decision support (7–9), prediction of disease trends and outcomes (10), and long-term health (11). AI also has tremendous potential to improve the optimization of healthcare system utilization (12) through the analysis of extremely large datasets. In this review, we will discuss how AI can improve the utilization of scarce critical care resources, using the lessons learned from dialysis utilization during the COVID-19 pandemic.
2 Dialysis resource management in critical care
2.1 Utilization of dialysis in critically ill patients
Dialysis is one of the most common procedures utilized in the ICU (13). Although neither of the two most commonly used modalities—continuous kidney replacement therapy (CKRT) and intermittent hemodialysis (IHD)—offers a significant survival advantage over the other (14), IHD is the more common modality of choice, and CKRT is typically recommended for patients with hemodynamic instability and those with high levels of intracranial pressure (15, 16). CKRT is also used in fluid management (especially when the obligate intake is large), burns, sepsis, and heart and liver failure patients (17). The choice of modality has been variable and is based on local ICU practices and the availability of resources (18). CKRT is more costly than IHD. In a base-case analysis, CKRT was found to cost $3,679 more than IHD per patient (19). In addition to machine cost, there is a significant cost associated with the large volume of dialysis and replacement fluids involved in CKRT (20). In addition, whereas CKRT is typically conducted by the ICU nurse taking care of the patient, IHD requires dialysis nurses, which adds to the personnel cost (21). Several studies have looked at the cost-effectiveness of CKRT compared with IHD (20, 22–24). In a post-hoc analysis of the BEST Kidney Study, using data from 53 centers across 23 countries, Srisawat et al. reported that the median cost per day was $289.60 (IQR $830.8–$116.8) greater for CKRT than for IHD, and that reducing the replacement fluid volumes increased the cost savings (20). In another study of critically ill patients with AKI, despite the greater initial cost of CKRT in the ICU, the 5-year total cost, including the cost of dialysis dependence, was lower for CKRT than for IHD (24). In a recent systemic review of seven published CEAs of CKRT vs. IHD, Singh et al. reported a marginal quality-adjusted life year (QALY) gain for CKRT, and that the cost-effectiveness of CKRT was mainly due to the long-term dialysis dependence rates (23).
2.2 Cost estimation for dialysis utilization in the ICU
Cost estimation in critical care is confounded by other factors such as pre- and post-ICU healthcare and the clinical diversity of patients, making the average daily ICU cost variable from one patient to another (2). Minimizing ICU costs can potentially be plagued by “cost shifting”, that is, greater costs incurred in the provision of non-ICU health services for the same patient. Unfortunately, most of the earlier published CEAs in critical care have focused on short-term outcomes such as ICU mortality and length of stay. However, the estimation of long-term outcomes, such as Health-Related Quality of Life (HRQOL) (25), socioeconomic impact of critical illness (26), and measures of longer-term morbidity and mortality in ICU survivors are crucial to more accurately determining cost-effectiveness and guiding decision-making on resource utilization (2). Patient-centered outcomes and societal perspectives are very important to improving the quality of CEA (27–29). In a study of 959 patients with AKI who were treated with dialysis in the ICU and followed up for 3 years, the main factors found to be associated with major adverse kidney events (death, incomplete kidney recovery, or development of end-stage kidney disease [ESKD]) were severity of illness and use of CKRT (vs. IHD) during the ICU admission (30). In another study looking at ICU survivors, the QOL, although lower at 3 months post-discharge, was similar at the 1- and 4-year follow-ups among those admitted to the ICU with acute kidney injury (AKI) requiring dialysis and those without AKI (31). Therefore, both short- and long-term healthcare expenditure and outcomes in critically ill patients with AKI should be analyzed for robust cost estimation. The factors traditionally used in the allocation of dialysis are summarized in Table 1.
3 Impact of the COVID-19 pandemic on dialysis allocation
3.1 Critical care resource allocation during the COVID-19 pandemic
Healthcare emergencies such as natural disasters and infectious disease pandemics exert a severe impact on critical care resources and require the creation of comprehensive policies on resource allocation (32). The influenza pandemics at the start of the 21st century, such as the H5N1 (“bird flu”) and H1N1 (“swine flu”) pandemics, resulted in acutely limited availability of mechanical ventilators (33), and triage protocols for resource deployment based on the Sequential Organ Failure Assessment (SOFA) score (34) were created to allocate access to critical care resources (35, 36). However, critical care triage during pandemics simply based on physiological scores such as SOFA has its limitations, and protocols should be modified based on other data, such as those related to outcomes, as they emerge in real-time (36).
The coronavirus disease 2019 (COVID-19) (37) pandemic resulted in an unprecedented burden on critical care units, leading to the reassessment of the existing pandemic utilization and allocation policies to better prepare ICUs for the unique challenges posed by COVID-19 (38). At the start of the pandemic in the United States, an Expert Panel Report of the Task Force for Mass Critical Care and the American College of Chest Physicians recommended a triage system to guide critical care resource management toward the patients who were most likely to benefit while maintaining the standard of care (32). Over the last 3 years, the emergence of new variants, variable isolation protocols, mass vaccination policies across nations and regions, and evolving therapies have led to difficulties in enforcing resource allocation policies that need to be constantly adapted in line with the changing face of the COVID-19 pandemic (39). This has led to the recognition of the importance of utilizing large real-time datasets for institutional planning and resource allocation to ensure standards of care under crisis and to meet the egalitarian and utilitarian principles of clinical practice.
3.2 Allocation of dialysis during the COVID-19 pandemic
AKI is common in patients with COVID-19 (40, 41) and is associated with in-hospital complications and mortality (41–47). A high proportion of COVID-19 patients admitted to the ICU, especially in the first year of the pandemic, required CKRT (48–50), resulting in a major burden on critical care resources. Assessing the risk of respiratory failure and the need for ventilators were not immediately recognized as priorities when treating patients with COVID-19, and similarly, the impact of dialysis-requiring AKI was not fully understood until later in the pandemic (51, 52). In addition to dialysis machines, there was a major increase in demand for all components of hospital dialysis programs, including nursing staff, technicians, and consumables such as dialysis filters and solutions and anticoagulation medications (53). In addition to patients who developed AKI, dialysis requirements also increased for patients with ESKD, who also had significantly increased rates of ICU admission (53).
This crisis led to the development of resource management policies in acute dialysis units across hospitals (53). The shortage of CKRT machines led to the creation of regional and institutional policies on increasing supply and developing stockpiles of CKRT machines (54, 55). Some academic centers adopted crisis protocols of prolonged intermittent kidney replacement therapy (56) or accelerated venovenous hemofiltration (57), and CKRT devices could be shared between patients. This novel strategy entailing the sharing of tools among patients undergoing CKRT in ICUs in close geographical proximity reduced nursing workloads and the need for machine transportation (58). However, the sharing protocols increased the demands of dialysis fluids due to the increased flow rates required for effective clearance. To address this demand, some centers adopted the policy of “fixed hours” on CKRT (51). Alternate dialysis strategies such as sustained low efficiency dialysis (SLED) that utilize the hospital water supply, hence minimizing the need for consumable dialysis solutions, were adopted in some centers (59). However, the drawback with SLED was the requirement of a dialysis nurse since most ICU nurses are not trained in this modality. To address the issue of nursing staff shortage, at our institute we adopted the remote telemonitoring of dialysis treatments, which was found to be safe, reliable, and minimized the risk of staff exposure (60). Some hospitals also employed acute peritoneal dialysis (APD) to expand their capacity and to tackle the shortage of hemodialysis machines (61). However, the role of APD in critically ill patients with COVID-19-related respiratory failure is limited by the risks of ventilatory compromise, especially in patients with requirements for high levels of oxygen, positive end-expiratory pressures, or proning (61, 62). The increased hypercoagulability seen in COVID-19 patients posed another challenge, due to the reduced filter life span and constant need to change filters, leading to increased costs and nursing workload (63). This led to the enforcement of anticoagulation protocols to minimize filter clotting. There is a dearth of data on CEAs in critical care dialysis allocation during the COVID-19 pandemic. One study of patients with ESKD comparing dialysis modality found that IHD was associated with a greater cost than PD during the COVID-19 pandemic (64). Finally, another challenge was the arrangement of outpatient dialysis centers, especially for new patients who required long-term dialysis. The development of isolation protocols and the arrangement of isolation rooms in chronic dialysis units with limited resources did not occur at a rate equal to the rapid increase in demand. This led to prolonged hospitalizations.
In summary, a large number of factors are involved in dialysis resource allocation to critically ill patients, especially during public health emergencies (Table 2). Using large datasets and smart analytics that can take into account all the relevant healthcare information in the hospital system could lead to efficient and cost-effective dialysis resource allocation in future public health emergencies.
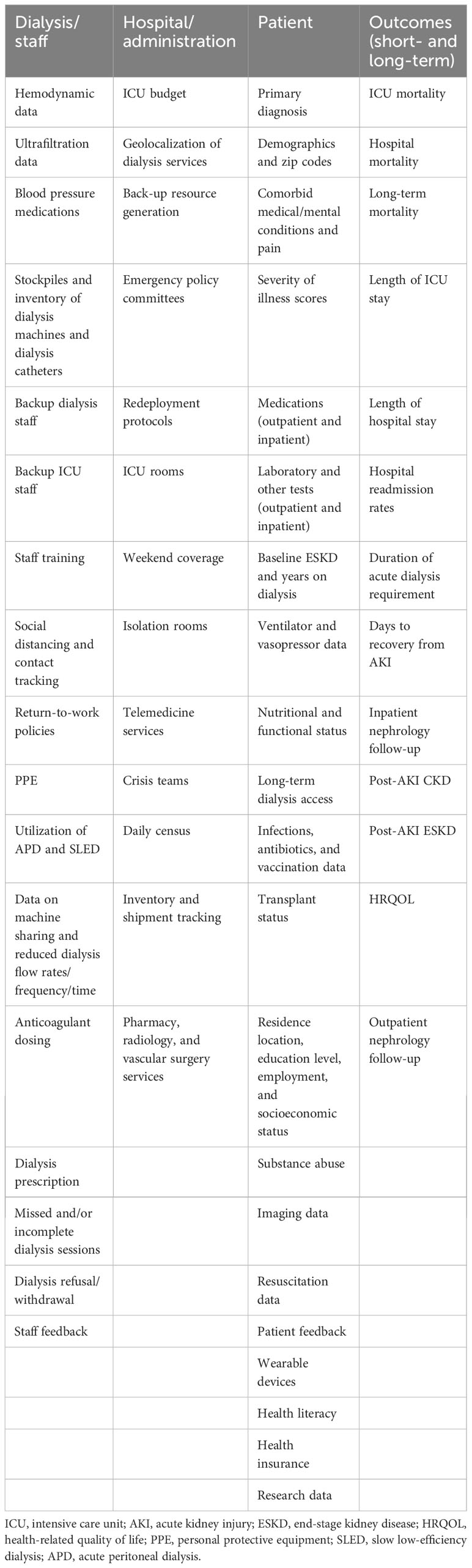
Table 2 Non-traditional data can be utilized for AI-based dialysis resource allocation and CEAs in critically ill patients during healthcare emergencies.
4 The role of big data and AI in optimizing utilization and allocation of dialysis resources
4.1 Overview of big data and AI in healthcare
The use of advanced information technologies to improve healthcare has seen a rapid advancement since the turn of the millennium owing to the rapid progress made in computer science and information technology (65). Modern health data analytics is focused on high-quality, efficient, and cost-effective care delivery and personalized medicine (66–70). This requires the ability to capture, integrate, and analyze heterogeneous unstructured and structured data in real-time from healthcare systems (71). Healthcare systems such as ICUs generate very large volumes of data constantly. The rapidly evolving AI techniques such as machine learning (ML) have the ability to analyze extremely large datasets, learn patterns, and identify non-linear associations and causal relationships (72, 73).
Healthcare data analytics include “data-driven” methods that include ML and pattern recognition algorithms and are categorized as supervised (classification) and unsupervised (clustering) learning models (74). “Knowledge-driven” methods include logic-based knowledge modeling and reasoning algorithms based on clinical data (75) and can be more readily applied to clinical decision-making (72). The applications of these models include “decision analytics” (e.g., the diagnosis and treatment of diseases), “predictive analytics” (e.g., using real-time data to predict health outcomes), “prescriptive analytics” (e.g., the simulation of future outcomes), “comparative analytics” (e.g., using data to compare impact of two different interventions on an outcome), and “semantic analytics” (e.g., using large datasets to understand complex relationships between the variables for hypothesis generation and testing) (72).
As the volume of data provided by ICUs and complexity of healthcare data have increased tremendously, there is an increasing need to develop general-purpose frameworks that allow non-experts to be able to easily apply complex AI algorithms to health management (72). It is important that non-AI experts understand the various facets of healthcare data, such as “variety” (multiple novel data sources such as biometrics and “omics” data), “veracity” (source and accuracy of data), “volume” (amount of data), “velocity” (the rate at which new data are generated), and, finally, “value” (knowledge gained and potential for clinical application) (76). All these data features are typically high in the ICU, making it an ideal setting for intelligent data analytics. However, to apply the correct analytical methods and to properly interpret the analysis, a proper understanding of each of these facets of healthcare data is critical.
4.2 The use of big data and AI in critical care resource allocation
Data mining and AI technology are now being increasingly used for human resource management systems and to improve workflows and cost–benefit ratios in healthcare systems (77–79). Previous studies have shown the utility of AI in the efficient scheduling of operating rooms (80), improvement of patient waiting times and staff workflow (81–84), reduction of hospital discharge time and length of stay (85), efficient hospital bed allocation (86), and the reduction of response time and hospital costs (87).
AI and big data analytics have great applications in mobile (“m”)-health (88) and in the recent pandemic, the use of tele-ICU in care delivery has greatly increased, especially by hospitals in remote, underserved settings (89). An intelligent remote monitoring system with innovative data analytics has been developed for the post-ICU monitoring of patients with COVID-19, which has the potential to reduce the length of ICU stays (90).
The unprecedented challenge created by the COVID-19 pandemic and the constant emergence of new data has further highlighted the importance of the integration of smart advanced information technologies in healthcare (91). Sottile et al. recently reported the use of smart analytics to predict mortality in patients hospitalized with COVID-19 using electronic health record (EHR) data (92). Cheng et al. reported the use of AI-based tools to predict the risk of ICU transfer in patients admitted for COVID-19 (93).
Therefore, the use of big data and AI-based technology has great potential to improve the management and allocation of resources in critical care.
4.3 The use of big data and AI in the allocation of dialysis
There are many factors to consider in the management of resources related to dialysis utilization. In one study conducted among hospitalized patients requiring dialysis, factors such as health insurance, ischemic heart disease, late referral to the nephrologist, and the use of temporary vascular access for the first dialysis were identified as the major factors contributing to an increased length of hospital stay (94). Other studies have identified that poorly controlled anemia, depression, and pain were factors associated with the increased utilization of hospital dialysis resources (95, 96). Limited health literacy was shown to significantly increase the risk of hospitalization among patients on chronic dialysis (97). As mentioned, the allocation of dialysis resources was a major challenge during the COVID-19 pandemic that required the development of emergency programs (98). The shortage of resources also resulted in ethical challenges for patients, families, clinicians, and policymakers for resource prioritization (99, 100).
To ensure equity and justice, there is a critical need to harness big data to maximize the cost-effective utilization of dialysis resources while maintaining the standard of care in critically ill patients. Although previous studies have identified important factors that can be addressed to improve the management of dialysis resources (51, 54–59, 61–63, 94–100), the availability of large volumes of EHR data in dialysis patients is a prime resource for the application of AI-based smart analytic tools for resource management (Table 2). The use of AI-based data analytics is rapidly increasing in the field of nephrology, and its application has increased our understanding of disease pathogenesis, outcome prediction, and the personalization of medicine (101). ML-based analytic models have been shown to outperform clinician-based predictions related to AKI (102–104). We have previously shown the use of X-Boost-based machine learning algorithms to predict the severity of AKI, the need for dialysis, and renal recovery in patients with COVID-19 (105). Data from existing repositories such as the EHR can help clinicians and administrators better triage existing dialysis resources to the hospitals that are and the patients who are predicted to need them most. Future research in this area should be an important priority.
4.4 Knowledge gaps and challenges in the use of big data and AI
As with any innovative technology, the hype and expectations surrounding intelligent data analytics have to be modified based on their limitations and drawbacks. The incorrect selection of datasets or analytic tools, erroneous processing of data, and incorrect interpretations of the results are major challenges that will need to be overcome in a field such as healthcare where the health of patients is at stake (Table 3). In a systemic review of 46 studies of the use of ML models to predict AKI, a high risk of bias and lack of external validation of the models was observed (102).
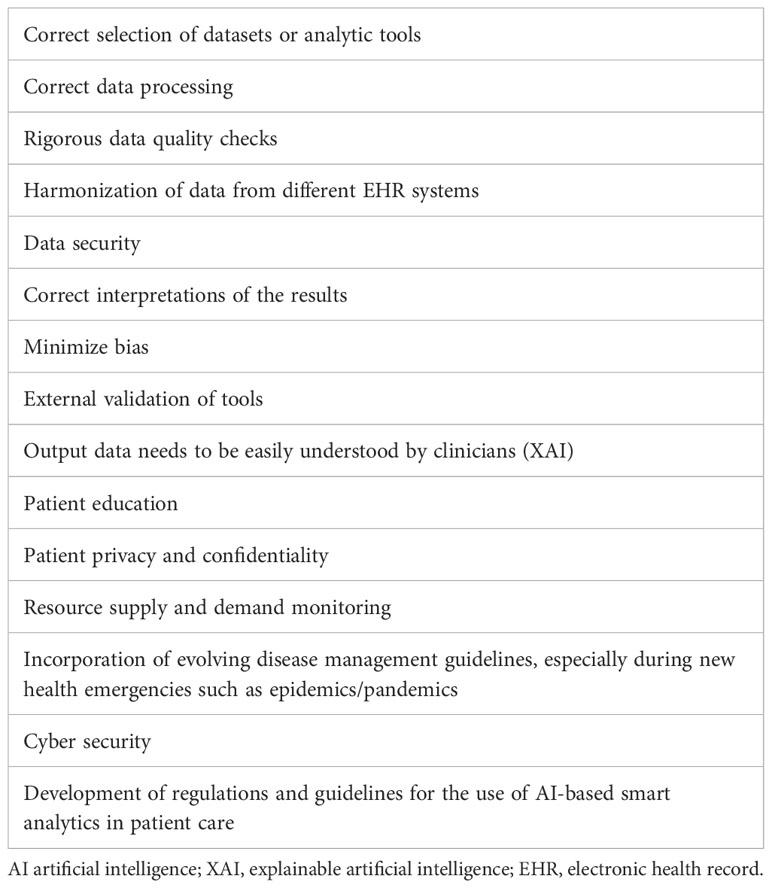
Table 3 Key factors in the application of AI-based analytics of EHR data for dialysis resource allocation.
To develop the trust of the healthcare community in this technology, the output data need to be clinically explainable and the processes transparent (106). In addition to clinicians, it will also be important to develop patient trust; hence, patient education will be crucial. Explainable artificial intelligence (XAI) is the next frontier in AI-based healthcare analytics (107). As with all aspects of healthcare delivery, patient privacy and confidentiality are paramount (108) and the privacy risks should be communicated to the patients and their consent obtained (109). Several regulatory compliance steps for data security might limit the use the AI systems until transparency and understanding improve (110). Patient characteristics, the nature of diseases, and the types of diagnostic tests and treatments can change with time, and this needs to be accounted for when updating existing AI tools and developing new ones. Rigorous checks on data quality must be carried out, and the harmonization of data across different EHRs in different health systems should be conducted before their application for analysis. In our recent study on characterizing AKI in patients with COVID-19, we leveraged EHR data in patients with COVID-19 from 53 healthcare systems across the United States (41). State-of-the-art data cleaning, quality control, and harmonization processes were undertaken before the analytical steps (41). Finally, the vulnerabilities of AI models to “adversarial attacks” should be routinely monitored and appropriate precautionary and corrective measures should be applied (111).
The development of laws, regulations, and guidelines for the use of healthcare data and AI-based smart analytics needs to be developed for use in all aspects of healthcare, including resource allocation (112). Improved accuracy of the data and understanding of AI tools would strengthen the healthcare community’s trust in them (113).
5 Conclusions
The COVID-19 pandemic resulted in an unprecedented burden on critical care units worldwide. Dialysis machines became scarce, leading to value-based CEA and the rationing of resources to deliver patient care of the best quality. AI-based big data analytics are now being utilized in multiple data-driven healthcare services, including the optimization of healthcare system utilization. Data from existing repositories such as the EHR can help clinicians and administrators to better triage existing dialysis resources to the hospitals and patients who are predicted to need them most. Although the application of AI-based big data analytics for dialysis allocation in critical care holds a lot of promise, several challenges need to be taken into consideration. Future research in this area should be an important priority.
Author contributions
FK: Conceptualization, Funding acquisition, Investigation, Methodology, Resources, Validation, Visualization, Writing - original draft, Writing - review & editing. SM: Conceptualization, Funding acquisition, Resources, Validation, Visualization, Writing - review & editing.
Funding
The author(s) declare financial support was received for the research, authorship, and/or publication of this article. FK is supported by Dialysis Clinics Inc. (RF# C4180) and the National Institutes of Health (R21OH012237-01). SM is supported by funding from the National Institute of Diabetes and Digestive and Kidney Diseases (DK112984 and DK121846), Veterans Affairs (1I01BX003698 and 1I01BX005300), and Dialysis Clinic, Inc.
Conflict of interest
The authors declare that the research was conducted in the absence of any commercial or financial relationships that could be construed as a potential conflict of interest.
Publisher’s note
All claims expressed in this article are solely those of the authors and do not necessarily represent those of their affiliated organizations, or those of the publisher, the editors and the reviewers. Any product that may be evaluated in this article, or claim that may be made by its manufacturer, is not guaranteed or endorsed by the publisher.
References
1. (SCCM) SoCCM. Critical Care Statistics (2023). Available at: https://www.sccm.org/Communications/Critical-Care-Statistics.
2. Abu Al-Saad N, Skedgel C, Nortje J. Principles of resource allocation in critical care. BJA Educ (2017) 17(12):390–5. doi: 10.1093/bjaed/mkx029
3. Kaukonen KM, Bailey M, Suzuki S, Pilcher D, Bellomo R. Mortality related to severe sepsis and septic shock among critically ill patients in Australia and New Zealand, 2000-2012. JAMA (2014) 311(13):1308–16. doi: 10.1001/jama.2014.2637
4. Jukarainen S, Mildh H, Pettilä V, Häkkinen U, Peltola M, Ala-Kokko T, et al. Costs and cost-utility of critical care and subsequent health care: A multicenter prospective study. Crit Care Med (2020) 48(5):e345–e55. doi: 10.1097/CCM.0000000000004210
5. Cookson R, Dolan P. Principles of justice in health care rationing. J Med Ethics (2000) 26(5):323–9. doi: 10.1136/jme.26.5.323
6. Edbrooke DL, Minelli C, Mills GH, Iapichino G, Pezzi A, Corbella D, et al. Implications of ICU triage decisions on patient mortality: a cost-effectiveness analysis. Crit Care (2011) 15(1):R56. doi: 10.1186/cc10029
7. Abidi SSR, Roy PC, Shah MS, Yu J, Yan S. A data mining framework for glaucoma decision support based on optic nerve image analysis using machine learning methods. J Healthc Inform Res (2018) 2(4):370–401. doi: 10.1007/s41666-018-0028-7
8. Abidi S. A knowledge-modeling approach to integrate multiple clinical practice guidelines to provide evidence-based clinical decision support for managing comorbid conditions. J Med Syst (2017) 41(12):193. doi: 10.1007/s10916-017-0841-1
9. Abidi SR, Cox J, Abusharekh A, Hashemian N, Abidi SS. A digital health system to assist family physicians to safely prescribe NOAC medications. Stud Health Technol Inform (2016) 228:519–23. doi: 10.3233/978-1-61499-678-1-519
10. Asadi H, Dowling R, Yan B, Mitchell P. Machine learning for outcome prediction of acute ischemic stroke post intra-arterial therapy. PLoS One (2014) 9(2):e88225. doi: 10.1371/journal.pone.0088225
11. Fleming TP, Watkins AJ, Velazquez MA, Mathers JC, Prentice AM, Stephenson J, et al. Origins of lifetime health around the time of conception: causes and consequences. Lancet (2018) 391(10132):1842–52. doi: 10.1016/S0140-6736(18)30312-X
12. Hayn D, Kreiner K, Ebner H, Kastner P, Breznik N, Rzepka A, et al. Development of multivariable models to predict and benchmark transfusion in elective surgery supporting patient blood management. Appl Clin Inform (2017) 8(2):617–31. doi: 10.4338/ACI-2016-11-RA-0195
13. Uchino S, Kellum JA, Bellomo R, Doig GS, Morimatsu H, Morgera S, et al. Acute renal failure in critically ill patients: a multinational, multicenter study. JAMA (2005) 294(7):813–8. doi: 10.1001/jama.294.7.813
14. Kellum JA, Angus DC, Johnson JP, Leblanc M, Griffin M, Ramakrishnan N, et al. Continuous versus intermittent renal replacement therapy: a meta-analysis. Intensive Care Med (2002) 28(1):29–37. doi: 10.1007/s00134-001-1159-4
15. Group KDIGO. KDIGO clinical practice guideline for acute kidney injury. Kidney Int (2012) 2:1–138. doi: 10.1038/kisup.2012.6
16. Macedo E, Mehta RL. Continuous dialysis therapies: core curriculum 2016. Am J Kidney Dis (2016) 68(4):645–57. doi: 10.1053/j.ajkd.2016.03.427
17. Davenport A, Honore PM. Continuous renal replacement therapy under special conditions like sepsis, burn, cardiac failure, neurotrauma, and liver failure. Semin Dial (2021) 34(6):457–71. doi: 10.1111/sdi.13002
18. Ronco C, Zanella M, Brendolan A, Milan M, Canato G, Zamperetti N, et al. Management of severe acute renal failure in critically ill patients: an international survey in 345 centres. Nephrol Dial Transplant (2001) 16(2):230–7. doi: 10.1093/ndt/16.2.230
19. Klarenbach S, Manns B, Pannu N, Clement FM, Wiebe N, Tonelli M, et al. Economic evaluation of continuous renal replacement therapy in acute renal failure. Int J Technol Assess Health Care (2009) 25(3):331–8. doi: 10.1017/S0266462309990134
20. Srisawat N, Lawsin L, Uchino S, Bellomo R, Kellum JA, Investigators BK. Cost of acute renal replacement therapy in the intensive care unit: results from The Beginning and Ending Supportive Therapy for the Kidney (BEST Kidney) study. Crit Care (2010) 14(2):R46. doi: 10.1186/cc8933
21. Lima AF. Direct cost of monitoring conventional hemodialysis conducted by nursing professionals. Rev Bras Enferm (2017) 70(2):357–63. doi: 10.1590/0034-7167-2016-0447
22. Hoyt DB. CRRT in the area of cost containment: is it justified? Am J Kidney Dis (1997) 30(5 Suppl 4):S102–4. doi: 10.1016/S0272-6386(97)90550-3
23. Singh A, Hussain S, Kher V, Palmer AJ, Jose M, Antony B. A systematic review of cost-effectiveness analyses of continuous versus intermittent renal replacement therapy in acute kidney injury. Expert Rev Pharmacoecon Outcomes Res (2022) 22(1):27–35. doi: 10.1080/14737167.2021.1916471
24. Ethgen O, Schneider AG, Bagshaw SM, Bellomo R, Kellum JA. Economics of dialysis dependence following renal replacement therapy for critically ill acute kidney injury patients. Nephrol Dial Transplant (2015) 30(1):54–61. doi: 10.1093/ndt/gfu314
25. Cuthbertson BH, Roughton S, Jenkinson D, Maclennan G, Vale L. Quality of life in the five years after intensive care: a cohort study. Crit Care (2010) 14(1):R6. doi: 10.1186/cc8848
26. Griffiths J, Hatch RA, Bishop J, Morgan K, Jenkinson C, Cuthbertson BH, et al. An exploration of social and economic outcome and associated health-related quality of life after critical illness in general intensive care unit survivors: a 12-month follow-up study. Crit Care (2013) 17(3):R100. doi: 10.1186/cc12745
27. Talmor D, Shapiro N, Greenberg D, Stone PW, Neumann PJ. When is critical care medicine cost-effective? A systematic review of the cost-effectiveness literature. Crit Care Med (2006) 34(11):2738–47. doi: 10.1097/01.CCM.0000241159.18620.AB
28. Arnold D, Girling A, Stevens A, Lilford R. Comparison of direct and indirect methods of estimating health state utilities for resource allocation: review and empirical analysis. BMJ (2009) 339:b2688. doi: 10.1136/bmj.b2688
29. Understanding costs and cost-effectiveness in critical care: report from the second American Thoracic Society workshop on outcomes research. Am J Respir Crit Care Med (2002) 165(4):540–50. doi: 10.1164/ajrccm.165.4.16541
30. De Corte W, Dhondt A, Vanholder R, De Waele J, Decruyenaere J, Sergoyne V, et al. Long-term outcome in ICU patients with acute kidney injury treated with renal replacement therapy: a prospective cohort study. Crit Care (2016) 20(1):256. doi: 10.1186/s13054-016-1409-z
31. Oeyen S, De Corte W, Benoit D, Annemans L, Dhondt A, Vanholder R, et al. Long-term quality of life in critically ill patients with acute kidney injury treated with renal replacement therapy: a matched cohort study. Crit Care (2015) 19(1):289. doi: 10.1186/s13054-015-1004-8
32. Maves RC, Downar J, Dichter JR, Hick JL, Devereaux A, Geiling JA, et al. Triage of scarce critical care resources in COVID-19 an implementation guide for regional allocation: an expert panel report of the task force for mass critical care and the American college of chest physicians. Chest (2020) 158(1):212–25. doi: 10.1016/j.chest.2020.03.063
33. Santini A, Messina A, Costantini E, Protti A, Cecconi M. COVID-19: dealing with ventilator shortage. Curr Opin Crit Care (2022) 28(6):652–9. doi: 10.1097/MCC.0000000000001000
34. Lambden S, Laterre PF, Levy MM, Francois B. The SOFA score-development, utility and challenges of accurate assessment in clinical trials. Crit Care (2019) 23(1):374. doi: 10.1186/s13054-019-2663-7
35. Christian MD, Hawryluck L, Wax RS, Cook T, Lazar NM, Herridge MS, et al. Development of a triage protocol for critical care during an influenza pandemic. CMAJ (2006) 175(11):1377–81. doi: 10.1503/cmaj.060911
36. Rowan KM, Harrison DA, Walsh TS, McAuley DF, Perkins GD, Taylor BL, et al. The Swine Flu Triage (SwiFT) study: development and ongoing refinement of a triage tool to provide regular information to guide immediate policy and practice for the use of critical care services during the H1N1 swine influenza pandemic. Health Technol Assess (2010) 14(55):335–492. doi: 10.3310/hta14550-05
37. Grasselli G, Zangrillo A, Zanella A, Antonelli M, Cabrini L, Castelli A, et al. Baseline characteristics and outcomes of 1591 patients infected with SARS-CoV-2 admitted to ICUs of the lombardy region, Italy. JAMA (2020) 323(16):1574–81. doi: 10.1001/jama.2020.5394
38. Knochel K, Adaktylos-Surber K, Schmolke EM, Meier LJ, Kuehlmeyer K, Ulm K, et al. Preparing for the worst-case scenario in a pandemic: intensivists simulate prioritization and triage of scarce ICU resources. Crit Care Med (2022) 50(12):1714–24. doi: 10.1097/CCM.0000000000005684
39. Supady A, Curtis JR, Abrams D, Lorusso R, Bein T, Boldt J, et al. Allocating scarce intensive care resources during the COVID-19 pandemic: practical challenges to theoretical frameworks. Lancet Respir Med (2021) 9(4):430–4. doi: 10.1016/S2213-2600(20)30580-4
40. Robbins-Juarez SY, Qian L, King KL, Stevens JS, Husain SA, Radhakrishnan J, et al. Outcomes for patients with COVID-19 and acute kidney injury: A systematic review and meta-analysis. Kidney Int Rep (2020) 5(8):1149–60. doi: 10.1016/j.ekir.2020.06.013
41. Yoo YJ, Wilkins KJ, Alakwaa F, Liu F, Torre-Healy LA, Krichevsky S, et al. Geographic and temporal trends in COVID-associated acute kidney injury in the national COVID cohort collaborative. Clin J Am Soc Nephrol (2023) 18(8):1006–18. doi: 10.2215/CJN.0000000000000192
42. Stony Brook C-RC. Geospatial distribution and predictors of mortality in hospitalized patients with COVID-19: A cohort study. Open Forum Infect Dis (2020) 7(10):ofaa436. doi: 10.1093/ofid/ofaa436
43. Cheng Y, Luo R, Wang K, Zhang M, Wang Z, Dong L, et al. Kidney disease is associated with in-hospital death of patients with COVID-19. Kidney Int (2020) 97(5):829–38. doi: 10.1016/j.kint.2020.03.005
44. Hirsch JS, Ng JH, Ross DW, Sharma P, Shah HH, Barnett RL, et al. Acute kidney injury in patients hospitalized with Covid-19. Kidney Int (2020) 98(1):209–18. doi: 10.1016/j.kint.2020.05.006
45. Richardson S, Hirsch JS, Narasimhan M, Crawford JM, McGinn T, Davidson KW, et al. Presenting characteristics, comorbidities, and outcomes among 5700 patients hospitalized with COVID-19 in the New York City area. JAMA (2020) 323(20):2052–9. doi: 10.1001/jama.2020.6775
46. Rubin S, Orieux A, Prevel R, Garric A, Bats M-L, Dabernat S, et al. Characterization of acute kidney injury in critically ill patients with severe coronavirus disease 2019. Clin Kidney J (2020) 13(3):354–61. doi: 10.1093/ckj/sfaa099
47. Chao CT, Tsai HB, Wu CY, Lin Y-F, Hsu N-C, Chen J-S, et al. The severity of initial acute kidney injury at admission of geriatric patients significantly correlates with subsequent in-hospital complications. Sci Rep (2015) 5:13925. doi: 10.1038/srep13925
48. Baduashvili A, Oberle LP, Devitt J. Frequency of continuous renal replacement therapy use early in coronavirus disease 2019 pandemic. Crit Care Explor (2020) 2(5):e0129. doi: 10.1097/CCE.0000000000000129
49. Cummings MJ, Baldwin MR, Abrams D, Jacobson SD, Meyer BJ, Balough EM, et al. Epidemiology, clinical course, and outcomes of critically ill adults with COVID-19 in New York City: a prospective cohort study. Lancet (2020) 395(10239):1763–70. doi: 10.1016/S0140-6736(20)31189-2
50. Gupta S, Hayek SS, Wang W, Chan L, Mathews KS, Melamed ML, et al. Factors associated with death in critically ill patients with coronavirus disease 2019 in the US. JAMA Intern Med (2020) 180(11):1436–47. doi: 10.1001/jamainternmed.2020.3596
51. Division of Nephrology CUVCoP. Disaster response to the COVID-19 pandemic for patients with kidney disease in New York City. J Am Soc Nephrol (2020) 31(7):1371–9. doi: 10.1681/ASN.2020040520
52. Mohamed MMB, Lukitsch I, Torres-Ortiz AE, Walker JB, Varghese V, Hernandez-Arroyo CF, et al. Acute kidney injury associated with coronavirus disease 2019 in urban new Orleans. Kidney360 (2020) 1(7):614–22. doi: 10.34067/KID.0002652020
53. Stevens JS, Velez JCQ, Mohan S. Continuous renal replacement therapy and the COVID pandemic. Semin Dial (2021) 34(6):561–6. doi: 10.1111/sdi.12962
54. Reddy YNV, Walensky RP, Mendu ML, Green N, Reddy KP. Estimating shortages in capacity to deliver continuous kidney replacement therapy during the COVID-19 pandemic in the United States. Am J Kidney Dis (2020) 76(5):696–709.e1. doi: 10.1053/j.ajkd.2020.07.005
55. Anger MS, Mullon C, Ficociello LH, Thompson D, Kraus MA, Newcomb P, et al. Meeting the demand for renal replacement therapy during the COVID-19 pandemic: A manufacturer's perspective. Kidney360 (2021) 2(2):350–4. doi: 10.34067/KID.0006192020
56. Bellomo R, Baldwin I, Fealy N. Prolonged intermittent renal replacement therapy in the intensive care unit. Crit Care Resusc (2002) 4(4):281–90.
57. Allegretti AS, Endres P, Parris T, Zhao S, May M, Sylvia-Reardon M, et al. Accelerated venovenous hemofiltration as a transitional renal replacement therapy in the intensive care unit. Am J Nephrol (2020) 51(4):318–26. doi: 10.1159/000506412
58. Akomeah J, Apostol A, Barnes E, Charytan C, Enriquez U, Katikaneni M, et al. Optimizing kidney replacement therapy during the COVID-19 pandemic across a complex healthcare system. Front Med (Lausanne) (2020) 7:604182. doi: 10.3389/fmed.2020.604182
59. Wen Y, LeDoux JR, Mohamed M, Ramanand A, Scharwath K, Mundy D, et al. Dialysis filter life, anticoagulation, and inflammation in COVID-19 and acute kidney injury. Kidney360 (2020) 1(12):1426–31. doi: 10.34067/KID.0004322020
60. Khan S, Mallipattu SK. Monitoring hospitalized dialysis patients with COVID-19: repurposing baby monitors for patient and staff safety. Kidney Med (2021) 3(1):136–8. doi: 10.1016/j.xkme.2020.10.004
61. Chen W, Caplin N, El Shamy O, Sharma S, Sourial MY, Ross MJ, et al. Use of peritoneal dialysis for acute kidney injury during the COVID-19 pandemic in New York City: a multicenter observational study. Kidney Int (2021) 100(1):2–5. doi: 10.1016/j.kint.2021.04.017
62. Shankaranarayanan D, Neupane SP, Varma E, Shimonov D, Gerardine S, Bhasin A, et al. Peritoneal dialysis for acute kidney injury during the COVID-19 pandemic in New York City. Kidney Int Rep (2020) 5(9):1532–4. doi: 10.1016/j.ekir.2020.07.017
63. Shankaranarayanan D, Muthukumar T, Barbar T, Bhasin A, Gerardine S, Lamba P, et al. Anticoagulation strategies and filter life in COVID-19 patients receiving continuous renal replacement therapy: A single-center experience. Clin J Am Soc Nephrol (2020) 16(1):124–6. doi: 10.2215/CJN.08430520
64. Behlul S, Artac Ozdal M. Risk of COVID-19 and cost burden in end-stage renal disease patients and policy implications for managing nephrology services during the COVID-19 pandemic. Healthcare (Basel) (2022) 10(12):2351. doi: 10.3390/healthcare10122351
65. Siau K. Interorganizational systems and competitive advantages – lessons from history. J Comput Inf Syst (2003) 44(1):33–9.
66. Obermeyer Z, Emanuel EJ. Predicting the future - big data, machine learning, and clinical medicine. N Engl J Med (2016) 375(13):1216–9. doi: 10.1056/NEJMp1606181
67. Abidi SS. Knowledge management in healthcare: towards 'knowledge-driven' decision-support services. Int J Med Inform (2001) 63(1-2):5–18. doi: 10.1016/S1386-5056(01)00167-8
68. Gabriel SE, Normand SL. Getting the methods right–the foundation of patient-centered outcomes research. N Engl J Med (2012) 367(9):787–90. doi: 10.1056/NEJMp1207437
69. Roy PC, Abidi SR, Abidi SSR. Possibilistic activity recognition with uncertain observations to support medication adherence in an assisted ambient living setting. Knowledge-Based Syst (2017) 133:156–73. doi: 10.1016/j.knosys.2017.07.008
70. Murdoch TB, Detsky AS. The inevitable application of big data to health care. JAMA (2013) 309(13):1351–2. doi: 10.1001/jama.2013.393
71. Jiang F, Jiang Y, Zhi H, Dong Y, Li H, Ma S, et al. Artificial intelligence in healthcare: past, present and future. Stroke Vasc Neurol (2017) 2(4):230–43. doi: 10.1136/svn-2017-000101
72. Abidi SSR, Abidi SR. Intelligent health data analytics: A convergence of artificial intelligence and big data. Healthc Manage Forum (2019) 32(4):178–82. doi: 10.1177/0840470419846134
73. Beam AL, Kohane IS. Translating artificial intelligence into clinical care. JAMA (2016) 316(22):2368–9. doi: 10.1001/jama.2016.17217
74. Darcy AM, Louie AK, Roberts LW. Machine learning and the profession of medicine. JAMA (2016) 315(6):551–2. doi: 10.1001/jama.2015.18421
75. Abidi SSR. Healthcare knowledge management: the art of the possible. In: Riaño D, editor. Knowledge Management for Health Care Procedures, vol. 2008 . Berlin, Heidelberg: Springer Berlin Heidelberg (2008). p. 1–20.
76. Bates DW, Saria S, Ohno-MaChado L, Shah A, Escobar G. Big data in health care: using analytics to identify and manage high-risk and high-cost patients. Health Aff (Millwood) (2014) 33(7):1123–31. doi: 10.1377/hlthaff.2014.0041
77. Yang Y. Artificial intelligence-based organizational human resource management and operation system. Front Psychol (2022) 13. doi: 10.3389/fpsyg.2022.962291
78. Gomes MAS, Kovaleski JL, Pagani RN, da Silva VL, Pasquini TCS. Transforming healthcare with big data analytics: technologies, techniques and prospects. J Med Eng Technol (2023) 47(1):1–11. doi: 10.1080/03091902.2022.2096133
79. Cozzoli N, Salvatore FP, Faccilongo N, Milone M. How can big data analytics be used for healthcare organization management? Literary framework and future research from a systematic review. BMC Health Serv Res (2022) 22(1):809. doi: 10.1186/s12913-022-08167-z
80. Sectra's VNA and Universal Viewer For All Enterprise Imaging Selected by UC San Diego Health (2021). Available at: https://www.prnewswire.com/news-releases/sectras-vna-and-universal-viewer-for-all-enterprise-imaging-selected-by-uc-health-301267485.html.
81. Ordu M, Demir E, Tofallis C. A comprehensive modelling framework to forecast the demand for all hospital services. Int J Health Plann Manage (2019) 34(2):e1257–e71. doi: 10.1002/hpm.2771
82. Cote MJ, Smith MA. Forecasting the demand for radiology services. Health Syst (Basingstoke) (2018) 7(2):79–88. doi: 10.1080/20476965.2017.1390056
83. Jones SS, Thomas A, Evans RS, Welch SJ, Haug PJ, Snow GL. Forecasting daily patient volumes in the emergency department. Acad Emerg Med (2008) 15(2):159–70. doi: 10.1111/j.1553-2712.2007.00032.x
84. Murphy MP, Brown NM. CORR synthesis: when should the orthopaedic surgeon use artificial intelligence, machine learning, and deep learning? Clin Orthop Relat Res (2021) 479(7):1497–505. doi: 10.1097/CORR.0000000000001679
85. Emes M, Smith S, Ward S, Smith A. Improving the patient discharge process: implementing actions derived from a soft systems methodology study. Health Syst (Basingstoke) (2019) 8(2):117–33. doi: 10.1080/20476965.2018.1524405
86. Zhu T, Liao P, Luo L, Ye HQ. Data-driven models for capacity allocation of inpatient beds in a Chinese public hospital. Comput Math Methods Med (2020) 2020:8740457. doi: 10.1155/2020/8740457
87. Yu X, Zhang C, Wang C. Construction of hospital human resource information management system under the background of artificial intelligence. Comput Math Methods Med (2022) 2022:8377674. doi: 10.1155/2022/8377674
88. Khan ZF, Alotaibi SR. Applications of artificial intelligence and big data analytics in m-health: A healthcare system perspective. J Healthc Eng (2020) 2020:8894694. doi: 10.1155/2020/8894694
89. Kemp Van Ee S, McKelvey H, Williams T, Shao B, Lin W-T, Luu J, et al. Telemedicine intensive care unit (Tele-ICU) implementation during COVID-19: A scoping review. Cureus (2022) 14(5):e25133. doi: 10.7759/cureus.25133
90. Panagopoulos C, Menychtas A, Jahaj E, Vassiliou AG, Gallos P, Dimopoulou I, et al. Intelligent pervasive monitoring solution of COVID-19 patients. Stud Health Technol Inform (2022) 295:570–3. doi: 10.3233/SHTI220792
91. Shiau W-L, Siau K, Yu Y, Guo J. Research commentary on IS/IT role in emergency and pandemic management: current and future research. J Database Manage (JDM) (2021) 32(2):67–75. doi: 10.4018/JDM.2021040105
92. Sottile PD, Albers D, DeWitt PE, Russell S, Stroh JN, Kao DP, et al. Real-time electronic health record mortality prediction during the COVID-19 pandemic: a prospective cohort study. J Am Med Inform Assoc (2021) 28(11):2354–65. doi: 10.1093/jamia/ocab100
93. Cheng FY, Joshi H, Tandon P, Freeman R, Reich DL, Mazumdar M, et al. Using machine learning to predict ICU transfer in hospitalized COVID-19 patients. J Clin Med (2020) 9(6):1668. doi: 10.3390/jcm9061668
94. Arora P, Kausz AT, Obrador GT, Ruthazer R, Khan S, Jenuleson CS, et al. Hospital utilization among chronic dialysis patients. J Am Soc Nephrol (2000) 11(4):740–6. doi: 10.1681/ASN.V114740
95. Weisbord SD, Mor MK, Sevick MA, Shields AM, Rollman BL, Palevsky PM, et al. Associations of depressive symptoms and pain with dialysis adherence, health resource utilization, and mortality in patients receiving chronic hemodialysis. Clin J Am Soc Nephrol (2014) 9(9):1594–602. doi: 10.2215/CJN.00220114
96. St Peter WL, Guo H, Kabadi S, Gilbertson DT, Peng Y, Pendergraft T, et al. Prevalence, treatment patterns, and healthcare resource utilization in Medicare and commercially insured non-dialysis-dependent chronic kidney disease patients with and without anemia in the United States. BMC Nephrol (2018) 19(1):67. doi: 10.1186/s12882-018-0861-1
97. Green JA, Mor MK, Shields AM, Sevick MA, Arnold RM, Palevsky PM, et al. Associations of health literacy with dialysis adherence and health resource utilization in patients receiving maintenance hemodialysis. Am J Kidney Dis (2013) 62(1):73–80. doi: 10.1053/j.ajkd.2012.12.014
98. Raina R, Chakraborty R, Sethi SK, Bunchman T. Kidney replacement therapy in COVID-19 induced kidney failure and septic shock: A pediatric continuous renal replacement therapy [PCRRT] position on emergency preparedness with resource allocation. Front Pediatr (2020) 8:413. doi: 10.3389/fped.2020.00413
99. Luyckx VA, Miljeteig I, Ejigu AM, Moosa MR. Ethical challenges in the provision of dialysis in resource-constrained environments. Semin Nephrol (2017) 37(3):273–86. doi: 10.1016/j.semnephrol.2017.02.007
100. Carson RC, Forzley B, Thomas S, Preto N, Hargrove G, Virani A, et al. Balancing the needs of acute and maintenance dialysis patients during the COVID-19 pandemic: A proposed ethical framework for dialysis allocation. Clin J Am Soc Nephrol (2021) 16(7):1122–30. doi: 10.2215/CJN.07460520
101. Kaur N, Bhattacharya S, Butte AJ. Big data in nephrology. Nat Rev Nephrol (2021) 17(10):676–87. doi: 10.1038/s41581-021-00439-x
102. Vagliano I, Chesnaye NC, Leopold JH, Jager KJ, Abu-Hanna A, Schut MC. Machine learning models for predicting acute kidney injury: a systematic review and critical appraisal. Clin Kidney J (2022) 15(12):2266–80. doi: 10.1093/ckj/sfac181
103. Rank N, Pfahringer B, Kempfert J, Stamm C, Kühne T, Schoenrath F, et al. Deep-learning-based real-time prediction of acute kidney injury outperforms human predictive performance. NPJ Digit Med (2020) 3:139. doi: 10.1038/s41746-020-00346-8
104. Tomasev N, Glorot X, Rae JW, Zielinski M, Askham H, Saraiva A, et al. A clinically applicable approach to continuous prediction of future acute kidney injury. Nature (2019) 572(7767):116–9. doi: 10.1038/s41586-019-1390-1
105. Sun S, Annadi RR, Chaudhri I, Munir K, Hajagos J, Saltz J, et al. Short- and Long-Term Recovery after Moderate/Severe AKI in Patients with and without COVID-19. Kidney360 (2022) 3(2):242–57. doi: 10.34067/KID.0005342021
106. Angelov P, Soares E, Jiang R, Arnold N, Atkinson P. Explainable artificial intelligence: an analytical review. WIREs Data Mining Knowl Discov (2021) 11(5):e1424. doi: 10.1002/widm.1424
107. Rahman A, Hossain MS, Muhammad G, Kundu D, Debnath T, Rahman M, et al. Federated learning-based AI approaches in smart healthcare: concepts, taxonomies, challenges and open issues. Cluster Comput (2022) 2022:1–41. doi: 10.1007/s10586-022-03658-4
108. Sohn JH, Chillakuru YR, Lee S, Lee AY, Kelil T, Hess CP, et al. An open-source, vender agnostic hardware and software pipeline for integration of artificial intelligence in radiology workflow. J Digit Imaging (2020) 33(4):1041–6. doi: 10.1007/s10278-020-00348-8
109. Liu Y, Chen PC, Krause J, Peng L. How to read articles that use machine learning: users' Guides to the medical literature. JAMA (2019) 322(18):1806–16. doi: 10.1001/jama.2019.16489
110. Zednik C. Solving the black box problem: A normative framework for explainable artificial intelligence. Philosophy Technol (2021) 34(2):265–88. doi: 10.1007/s13347-019-00382-7
111. Finlayson SG, Bowers JD, Ito J, Zittrain JL, Beam AL, Kohane IS. Adversarial attacks on medical machine learning. Science (2019) 363(6433):1287–9. doi: 10.1126/science.aaw4399
112. Gerke S, Minssen T, Cohen G. Chapter 12 - Ethical and legal challenges of artificial intelligence-driven healthcare. In: Bohr A, Memarzadeh K, editors. Artificial Intelligence in Healthcare. Elsevier Inc.: Academic Press (2020). p. 295–336. doi: 10.1016/B978-0-12-818438-7.00012-5
Keywords: critical care, resource deployment, dialysis, COVID-19, big data, artificial intelligence, machine learning
Citation: Koraishy FM and Mallipattu SK (2023) Dialysis resource allocation in critical care: the impact of the COVID-19 pandemic and the promise of big data analytics. Front. Nephrol. 3:1266967. doi: 10.3389/fneph.2023.1266967
Received: 25 July 2023; Accepted: 05 October 2023;
Published: 26 October 2023.
Edited by:
Motonobu Nakamura, The University of Tokyo, JapanReviewed by:
Yoshifumi Hamasaki, The University of Tokyo Hospital, JapanDaisuke Katagiri, National Center for Global Health and Medicine, Japan
Copyright © 2023 Koraishy and Mallipattu. This is an open-access article distributed under the terms of the Creative Commons Attribution License (CC BY). The use, distribution or reproduction in other forums is permitted, provided the original author(s) and the copyright owner(s) are credited and that the original publication in this journal is cited, in accordance with accepted academic practice. No use, distribution or reproduction is permitted which does not comply with these terms.
*Correspondence: Farrukh M. Koraishy, RmFycnVraC5Lb3JhaXNoeUBzdG9ueWJyb29rbWVkaWNpbmUuZWR1