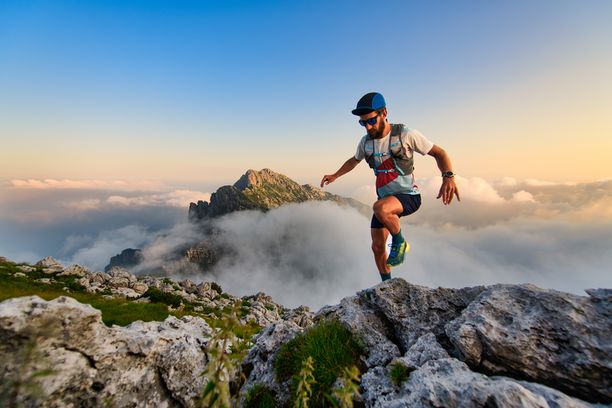
94% of researchers rate our articles as excellent or good
Learn more about the work of our research integrity team to safeguard the quality of each article we publish.
Find out more
ORIGINAL RESEARCH article
Front. Mol. Biosci.
Sec. Molecular Diagnostics and Therapeutics
Volume 12 - 2025 | doi: 10.3389/fmolb.2025.1549223
This article is part of the Research TopicMedical Knowledge-Assisted Machine Learning Technologies in Individualized Medicine Volume IIView all 12 articles
The final, formatted version of the article will be published soon.
Select one of your emails
You have multiple emails registered with Frontiers:
Notify me on publication
Please enter your email address:
If you already have an account, please login
You don't have a Frontiers account ? You can register here
Cholangiocarcinoma (CCA) is a highly aggressive malignancy, and early diagnosis remains challenging. Metabolic biomarkers are increasingly recognized as promising tools for the early detection of cancer. However, a comprehensive exploration of metabolic alterations in CCA, especially from a global metabolic perspective, has yet to be fully realized.To identify reliable metabolic markers for the early diagnosis of CCA and to explore its potential pathogenesis through an in-depth analysis of global metabolism.Serum samples from 30 CCA patients and 31 healthy individuals were analyzed using an unbiased UPLC-Q-TOF-MS based metabolomics approach. Principal component analysis (PCA) and orthogonal projections to latent structures discriminant analysis (OPLS-DA) were applied to identify potential biomarkers. High-resolution MS/MS and available standards were used to further confirm the identified metabolites. A systematic metabolic pathway analysis was conducted to interpret the biological roles of these biomarkers and explore their relevance to CCA progression.
Keywords: Cholangiocarcinoma, diagnosis, Unbiased serum metabolomics, UPLC-Q-ToF-MS, biomarker screen and identification 1
Received: 20 Dec 2024; Accepted: 17 Mar 2025.
Copyright: © 2025 Wang, Xu, Jia, XU and Hu. This is an open-access article distributed under the terms of the Creative Commons Attribution License (CC BY). The use, distribution or reproduction in other forums is permitted, provided the original author(s) or licensor are credited and that the original publication in this journal is cited, in accordance with accepted academic practice. No use, distribution or reproduction is permitted which does not comply with these terms.
* Correspondence:
Yuanhong XU, Department of Clinical Laboratory, First Affiliated Hospital of Anhui Medical University, Hefei, Anhui Province, China
Ping Hu, School of Chemistry and Molecular Engineering, East China Normal University, Shanghai, 200241, Shanghai, China
Disclaimer: All claims expressed in this article are solely those of the authors and do not necessarily represent those of their affiliated organizations, or those of the publisher, the editors and the reviewers. Any product that may be evaluated in this article or claim that may be made by its manufacturer is not guaranteed or endorsed by the publisher.
Supplementary Material
Research integrity at Frontiers
Learn more about the work of our research integrity team to safeguard the quality of each article we publish.