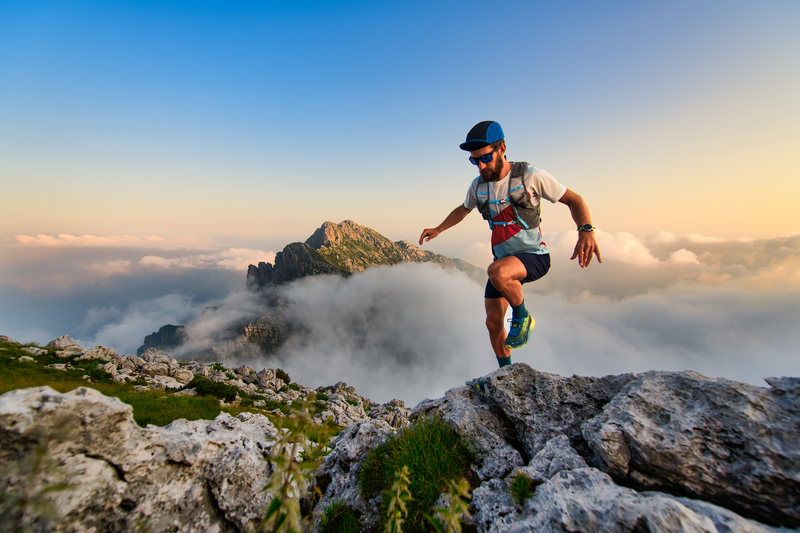
94% of researchers rate our articles as excellent or good
Learn more about the work of our research integrity team to safeguard the quality of each article we publish.
Find out more
ORIGINAL RESEARCH article
Front. Mol. Biosci.
Sec. Molecular Diagnostics and Therapeutics
Volume 12 - 2025 | doi: 10.3389/fmolb.2025.1548655
The final, formatted version of the article will be published soon.
You have multiple emails registered with Frontiers:
Please enter your email address:
If you already have an account, please login
You don't have a Frontiers account ? You can register here
Background: Lung adenocarcinoma (LUAD) is responsible for majority cases of lung cancer and considered to be the primary cause of cancer-related mortality. The imbalance of cellular proliferation and apoptosis is critically implicated in the pathogenesis and progression of LUAD. Sphingomyelin, a vital lipid component, is integral to the regulation of tumor cell growth and apoptosis, and has garnered significant attention as a target in novel anticancer therapies. The pivotal molecules involved in sphingomyelin metabolism are crucial in modulating tumor cell behavior, thereby influencing clinical outcomes.A comprehensive Consensus clustering analysis was conducted by collecting clinical LUAD figures from the TCGA and GEO databases. By employing Cox regression and Lasso regression analysis, a prognostic model for LUAD patients was established by identifying seven sphingolipid-related genes (SRGs). And validated in the GEO database. The study also delved into the clinical relevance, functional capabilities, and immune implications of prognostic signals associated with sphingolipid metabolism. Finally, experiments conducted in vitro confirmed the imbalance of sphingolipid-associated genes in LUAD.Using the prognostic model, lung adenocarcinoma (LUAD) patients can be divided into high-risk and low-risk groups. Meanwhile, we can observe marked disparities in survival times among these groups. Additionally, the model demonstrates high predictive accuracy in external Conclusions: This study demonstrates that sphingolipid-related gene prognostic characteristics correlate with tumor progression and recurrence, long-term prognosis, and immune infiltration in LUAD patients. The outcomes of our study could help shape innovative strategies for early intervention and prognosis prediction in lung adenocarcinoma.
Keywords: Sphingolipid metabolism, Lung Adenocarcinoma, Prognostic signature, immune microenvironment, immunohistochemical experiment in vitro
Received: 23 Dec 2024; Accepted: 25 Feb 2025.
Copyright: © 2025 Mao, Li, Sun, Han, Li, Dong and Zhao. This is an open-access article distributed under the terms of the Creative Commons Attribution License (CC BY). The use, distribution or reproduction in other forums is permitted, provided the original author(s) or licensor are credited and that the original publication in this journal is cited, in accordance with accepted academic practice. No use, distribution or reproduction is permitted which does not comply with these terms.
* Correspondence:
Jibin Mao, Medical School, Nantong University, Nantong, China
Chang-Sheng Dong, Department of Oncology, Longhua Hospital, Shanghai University of Traditional Chinese Medicine, Shanghai, 200032, China
Hongyu Zhao, Department of Radiation Oncology, Affiliated Hospital of Nantong University, Nantong, 226001, Jiangsu Province, China
Disclaimer: All claims expressed in this article are solely those of the authors and do not necessarily represent those of their affiliated organizations, or those of the publisher, the editors and the reviewers. Any product that may be evaluated in this article or claim that may be made by its manufacturer is not guaranteed or endorsed by the publisher.
Research integrity at Frontiers
Learn more about the work of our research integrity team to safeguard the quality of each article we publish.