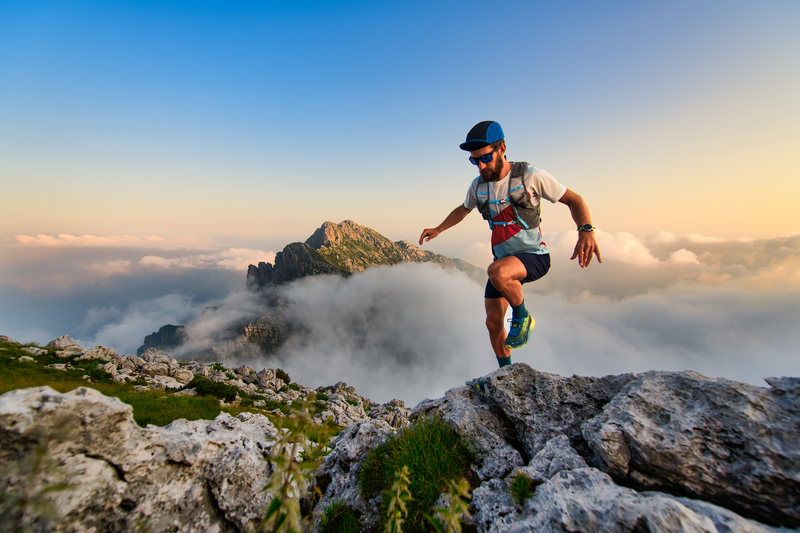
94% of researchers rate our articles as excellent or good
Learn more about the work of our research integrity team to safeguard the quality of each article we publish.
Find out more
BRIEF RESEARCH REPORT article
Front. Mol. Biosci.
Sec. Metabolomics
Volume 12 - 2025 | doi: 10.3389/fmolb.2025.1511119
The final, formatted version of the article will be published soon.
You have multiple emails registered with Frontiers:
Please enter your email address:
If you already have an account, please login
You don't have a Frontiers account ? You can register here
Real-time breath analysis has shown potential as a non-invasive method for detecting oxidative stress and airway inflammation. However, there is a lack of data on the association of full breath profiles with established urinary biomarkers of oxidative stress and respiratory inflammation, which could help to advance the implementation of this method in the clinical practice. We analyzed breath profiles of 25 tobacco smoke-exposed and 103 non-exposed children using real-time secondary electrospray ionization high-resolution mass spectrometry (SESI-HRMS) and determined in parallel urinary concentrations of biomarkers of oxidative stress and respiratory inflammation. We evaluated the correlation between breath features and urinary biomarkers and tested the prediction of these biomarkers by exhaled breath. We found 71 breath features that correlated significantly with the urinary oxidative stress marker 8-iso-prostaglandin F2α (8-iso-PGF2α). The agreement (mean (standard deviation) Lin's concordance correlation) between breath-predicted and actual urinary 8-iso-PGF2α levels was 0.37 (0.05). In conclusion, our results suggest that the real-time breath analysis with SESI-HRMS has promising potential to gauge oxidative stress.
Keywords: Urine, Oxidative Stress, exhaled breath, Child, Smoking
Received: 14 Oct 2024; Accepted: 26 Feb 2025.
Copyright: © 2025 Gisler, Singh, Marten, Decrue, Frey, Sinues and Usemann. This is an open-access article distributed under the terms of the Creative Commons Attribution License (CC BY). The use, distribution or reproduction in other forums is permitted, provided the original author(s) or licensor are credited and that the original publication in this journal is cited, in accordance with accepted academic practice. No use, distribution or reproduction is permitted which does not comply with these terms.
* Correspondence:
Jakob Usemann, University Children’s Hospital Basel, Basel, Switzerland
Disclaimer: All claims expressed in this article are solely those of the authors and do not necessarily represent those of their affiliated organizations, or those of the publisher, the editors and the reviewers. Any product that may be evaluated in this article or claim that may be made by its manufacturer is not guaranteed or endorsed by the publisher.
Research integrity at Frontiers
Learn more about the work of our research integrity team to safeguard the quality of each article we publish.