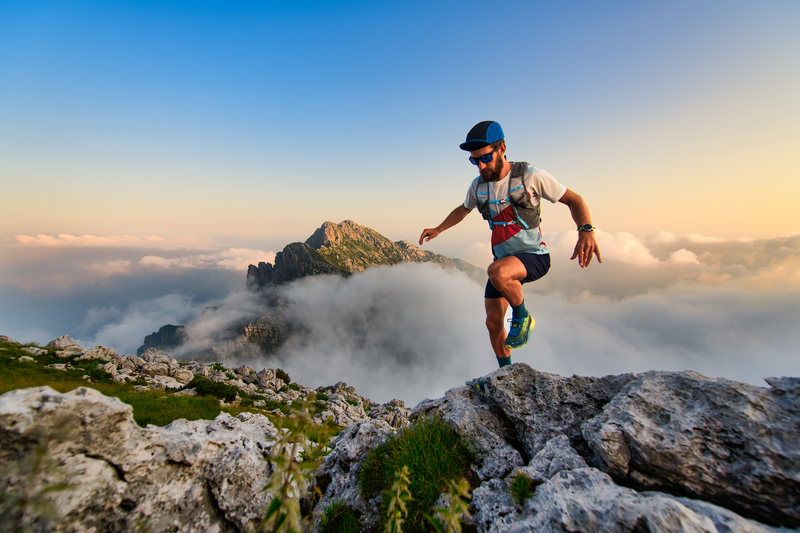
95% of researchers rate our articles as excellent or good
Learn more about the work of our research integrity team to safeguard the quality of each article we publish.
Find out more
ORIGINAL RESEARCH article
Front. Mol. Biosci. , 18 March 2025
Sec. Molecular Diagnostics and Therapeutics
Volume 12 - 2025 | https://doi.org/10.3389/fmolb.2025.1506961
This article is part of the Research Topic Interplay Between Cancer and the Nervous System: Molecular and Cellular Mechanisms View all 4 articles
Background: Discovering biomarkers is central to the research and treatment of degenerative central nervous system (CNS) diseases, playing a crucial role in early diagnosis, disease monitoring, and the development of new treatments, particularly for challenging conditions like degenerative CNS diseases and glioblastoma (GBM).
Methods: This study analyzed gene expression data from a public database, employing differential expression analyses and Gene Co-expression Network Analysis (WGCNA) to identify gene modules associated with degenerative CNS diseases and GBM. Machine learning methods, including Random Forest, Least Absolute Shrinkage and Selection Operator (LASSO), and Support Vector Machine - Recursive Feature Elimination (SVM-RFE), were used for case-control differentiation, complemented by functional enrichment analysis and external validation of key genes.
Results: Ninety-five commonly altered genes related to degenerative CNS diseases and GBM were identified, with RELN and GSTO2 emerging as significant through machine learning screening. Receiver operating characteristic (ROC) analysis confirmed their diagnostic value, which was further validated externally, indicating their elevated expression in controls.
Conclusion: The study’s integration of WGCNA and machine learning uncovered RELN and GSTO2 as potential biomarkers for degenerative CNS diseases and GBM, suggesting their utility in diagnostics and as therapeutic targets. This contributes new perspectives on the pathogenesis and treatment of these complex conditions.
Degenerative central nervous system (CNS) diseases (Xu et al., 2022), such as Alzheimer's disease (AD), Parkinson’s disease (PD), multiple sclerosis (MS), and amyotrophic lateral sclerosis (ALS), as well as neurological tumors, particularly glioblastoma (GBM) (Broekman et al., 2018), represent the most challenging diseases in the field of neuroscience. These diseases not only impose a heavy burden on patients and their families, but also pose significant pressures on society and the healthcare system (Wareham et al., 2022).
Despite the significant differences in clinical presentation and pathology between degenerative CNS diseases and GBM, they exhibit remarkable similarities in certain key biological processes, such as ferroptosis, oxidative stress, and neuroinflammation.
Ferroptosis is a form of cell death dependent on iron and lipid peroxidation, shown to play a critical role in both degenerative CNS diseases and GBM. For example, iron accumulation and the resulting oxidative damage are key pathological processes in Parkinson’s disease (Yan et al., 2024). Similarly, iron metabolism abnormalities have been observed in GBM, suggesting that ferroptosis could be a common pathological mechanism in these two types of diseases (Soo et al., 2024). Oxidative stress is important in both degenerative CNS diseases and GBM. Excess reactive oxygen species (ROS) can cause cellular damage and death. In AD and PD, oxidative stress is considered a major cause of neuronal damage (Isidro, 2018). In GBM, oxidative stress not only promotes tumor cell proliferation and migration but may also influence tumor progression by modulating interactions within the tumor microenvironment (Lauryn E et al., 2017). Neuroinflammation is common in degenerative CNS diseases. Inflammation plays a key role in the progression of AD and PD (Pei-Lun et al., 2024). In GBM, the inflammatory environment not only promotes tumor cell growth and survival but may also affect the tumor’s response to treatment by modulating immune cell function (Ana Helena Larangeira et al., 2024).
Research indicates significant overlap in gene and epigenetic regulation between degenerative CNS diseases and GBM. For example, a study (Lu et al., 2023) analyzed the expression of different isoforms (alternative splicing variants) of genes in brain tissue and identified multiple gene regulatory sites associated with neurological traits and diseases. These regulatory sites may play similar roles in different degenerative CNS diseases and GBM. Notably, isoform-ratio quantitative trait loci have been shown to regulate gene expression in brain tissue, with implications for various CNS conditions such as AD, mood fluctuations, and sleep duration. Understanding these regulatory mechanisms across degenerative CNS diseases and GBM could provide new insights into shared molecular pathways and identify novel therapeutic targets (Ashraf et al., 2023). Understanding these common regulatory mechanisms helps elucidate the shared genetic regulation in different diseases. In both brain tumors and degenerative CNS diseases, cell-cell interactions are crucial in disease development and progression. For instance, the activation of astrocytes and microglia is critical in AD progression, where microglial activation contributes to amyloid-β plaque clearance or neuroinflammation, as reported in recent study (Teresa et al., 2024). Similarly, abnormal activation of astrocytes is a key driver of GBM proliferation and invasion, as demonstrated in preclinical research (Jian and Shiwei, 2024). Despite these shared mechanisms, degenerative CNS diseases and GBM exhibit significant differences. Degenerative CNS diseases, such as AD and Parkinson’s disease, are chronic progressive conditions characterized by gradual neuronal damage and death, leading to cognitive and motor function decline (Andrea J. et al., 2020; M. et al., 2024).
In contrast, GBM is a highly invasive malignant brain tumor characterized by rapid growth and extensive damage to surrounding brain tissue. Therapeutically, the treatment of degenerative CNS diseases mainly focuses on symptom management and slowing disease progression, such as using dopamine replacement therapy in Parkinson’s disease. In contrast, GBM treatment includes surgical resection, radiotherapy, and chemotherapy, although these methods often cannot completely eradicate the tumor and it tends to recur.
Despite significant progress in understanding the molecular mechanisms underlying these diseases over the last decades, these findings are far from being fully translated into effective treatments. Therefore, the search for novel biomarkers that can be used as early diagnostic tools, indicators of disease progression, and monitoring means of treatment response, as well as the identification of new therapeutic targets, has become a cutting-edge topic in neuroscience research.
In recent years, the rapid advancements in bioinformatics (Gauthier et al., 2019), genomics (Gangfuß et al., 2022), and proteomics (Cui et al., 2022) have significantly deepened our understanding of the molecular underpinnings of neurological disorders. The application of high-throughput gene expression analyses (Hrdlickova et al., 2017) and differential expression analysis techniques (McDermaid et al., 2019) has emerged as a powerful tool for identifying disease-associated genes and pathways. By examining the gene expression patterns in degenerative CNS diseases and GBM, we aim to uncover potential common molecular mechanisms and cross-disease biomarkers between these conditions. These cross-disease biomarkers not only offer a new perspective for understanding these seemingly disparate diseases, which may share certain fundamental biological traits, but also hold promise for the development of therapeutic approaches that transcend specific diseases.
In this study, we aimed to identify potential biomarkers and pathways implicated in the progression of degenerative CNS diseases and glioblastoma. To achieve this, we integrated intersecting genes from four primary degenerative CNS diseases, sourced from GeneCards, with glioblastoma-related matrices obtained from the Gene Expression Omnibus (GEO). Weighted Gene Co-expression Network Analysis (WGCNA) was performed to identify differentially expressed genes and key modules within these diseases. Subsequently, we used intersecting genes and three machine learning approaches to identify diagnostic genes, GSTO2 and RELN, shared between the two diseases. These genes demonstrated robust diagnostic performance and were validated using external datasets. In addition, we performed Single Sample Gene Set Enrichment Analysis (GSEA) for these genes to identify common pathways associated with degenerative CNS diseases and glioblastoma. Figure 1 provides an overview of the workflow for data preparation, processing, analysis, and validation.
Genes related to AD, PD, MS, and ALS were retrieved from GeneCards, with a selection threshold set for a relevance score ≥1. The genes at the intersection of these diseases were regarded as representative of the genetic framework for degenerative CNS diseases in our research.
Gene expression matrices for GBM were sourced from the GEO database (GSE151352, Public on 29 May 2020). This dataset was selected due to its focus on paired normal and tumor tissue samples, providing a robust framework for comparing differential gene expression in GBM. Utilizing the platform GPL23934 (Ion Torrent S5), GSE151352 comprises RNA-seq data from 12 GBM patients, each with matched fresh tumor and normal brain tissue samples. The dataset’s design includes rigorous sample validation via immunostaining, ensuring the accuracy of tissue classification. These samples were processed using the Ion Ampliseq Transcriptome Human Gene Expression Kit, and sequencing was performed with the Ion S5 semiconductor sequencer. This high-quality dataset provides critical insights into GBM’s molecular heterogeneity, supporting the objectives of this study.
Utilizing R software (version 4.3.1), we identified DEGs of GBM in serum samples from the patient and control groups. Considering the small sample size of our study, we employed the limma package to perform differential expression analysis. Specifically, we first fitted a linear model using the lmFit () function and then applied the empirical Bayes moderation via the eBayes () function to adjust for random variance across genes. This moderated T-test approach improves the reliability of DEG identification. For each gene, we compared its average expression between the patient and control groups using a statistical test. This test produced an adjusted p-value, which indicates the likelihood that the observed difference in expression occurred by chance, and a log2 fold change, which quantifies the magnitude of the difference. Genes with an adjusted p-value of 0.05 or less and an absolute log2 fold change of at least 1 were classified as differentially expressed. The log2 scale was applied to make the expression differences easier to interpret and to align with standard practices in gene expression analysis.
To pinpoint pivotal genes, we employed the WGCNA within the R programming environment, focusing on identifying hub genes in highly correlated gene clusters, also known as modules. Initially, we constructed a Topological Overlap Matrix (TOM) to examine the correlations among genes. Subsequently, we calculated the dissimilarity of the TOM (diss TOM = 1 - TOM), which was used to generate a phylogenetic clustering tree. This allowed us to group genes with similar expression patterns into modules through a “TOM-based” approach. We set specific parameters for module aggregation, requiring modules to have at least 10 genes but no more than 500, and applied a clustering height cut-off of 0.25 to ensure clear distinction among modules. Following this, we determined module membership and assessed gene significance, selecting key genes from the primary modules for further analysis.
Utilizing the R programming language, we conducted a filtration process on previously identified genes. This step was aimed at discerning genes at the intersection of three critical categories: genes within GBM’s pivotal WGCNA modules, DEGs in GBM, and commonly altered genes associated with degenerative CNS diseases. This methodological approach enabled us to pinpoint target genes shared across these significant domains, offering potential insights into shared molecular mechanisms and identifying targets for further investigation and therapeutic development.
We identified genes from relevant modules and intersected them with previously determined commonly altered genes to pinpoint commonly altered genes. Subsequently, we utilized R software to perform enrichment analyses on these commonly altered genes, employing both the Kyoto Encyclopedia of Genes and Genomes (KEGG) and Gene Ontology (GO) frameworks.
To single out key candidate genes, we employed three advanced machine learning techniques: Random Forest, Least Absolute Shrinkage and Selection Operator (LASSO), and Support Vector Machine - Recursive Feature Elimination (SVM-RFE). These methods were used to refine the selection of hub genes from those identified as co-expressed, focusing on genes that were consistently highlighted across all three techniques. Each method was applied to prioritize genes based on their relevance and potential as key candidates.
To confirm the significance of the identified hub genes, we assessed their diagnostic capabilities through the construction of receiver operating characteristic (ROC) curves and examination of their expression levels within the dataset. To accomplish this, we employed ROC-specific packages in R, facilitating the creation of these curves. We then determined the area under the curve (AUC) for each gene. An AUC value nearing 1 signifies enhanced predictive accuracy, indicating that the gene in question possesses a high potential for distinguishing between disease states. This validation process is crucial for establishing the reliability of our findings, as it directly tests the ability of the hub genes to serve as effective markers for diagnosis. By leveraging the analytical power of R and its ROC-related functionalities, we are able to quantitatively evaluate the diagnostic performance of each gene, ensuring that only those with significant discriminative power are considered in the context of disease identification and analysis.
To validate the diagnostic accuracy of the two identified genes further, we turned to the external dataset GSE196553, which contains data for GBM. This dataset, comprising samples from 9 healthy controls and 61 GBM patients, served as our external validation cohort. We depicted the expression patterns of the diagnostic genes in cohort using boxplots and also computed AUC to assess their diagnostic performance. This step is essential for verifying the reliability and applicability of our findings in a broader context, ensuring that the diagnostic genes maintain their predictive power across different sample sets. By analyzing their expression in an independent group of subjects, we solidify the evidence for these genes’ roles as biomarkers, enhancing confidence in their potential clinical utility.
In our exploration of the hub genes’ functions, we utilized the NetworkAnalyst platform (https://www.networkanalyst.ca/), a dedicated tool for in-depth data analysis, available online. This platform provided us access to essential resources such as the ChEA database, which we used to predict transcription factors (TFs) linked to our hub genes, and the TarBase database, which aided in identifying miRNAs associated with these genes. This thorough methodology allowed us to delineate the intricate network of interactions and regulatory pathways involving the hub genes, offering insights into their roles within biological systems.
The Single-sample Gene Set Enrichment Analysis (ssGSEA) approach is designed to evaluate the presence and activity level of specific gene sets within individual samples, with a particular focus on transcriptomic data. It sorts genes based on their expression levels and generates enrichment scores for selected gene sets. This method is instrumental in identifying potential biomarkers and elucidating biological pathways relevant to disease and therapeutic investigations. In our research, we applied ssGSEA to forecast the expression profiles of key hub genes.
In order to comprehensively analyze gene expression and their correlations with immune data, we first conducted Gene Set Variation Analysis (GSVA) to quantify gene set enrichment scores across different samples. This enabled us to assess the biological variations in gene expression related to specific pathways or processes. Subsequently, we focused on differential expression analysis, comparing the gene expression patterns between the control and disease groups. For correlation analysis, we calculated Spearman correlation coefficients between individual gene expression levels and immune data to identify potential associations. These correlation coefficients and their significance were visualized using heatmaps
This analysis is crucial for understanding how these hub genes function within various biological contexts, enabling us to uncover their roles in disease mechanisms and potential therapeutic interventions. By leveraging ssGSEA, we gain detailed insights into the gene expression patterns that characterize different states or responses, enhancing our ability to pinpoint genes that are critical for disease progression or response to treatment.
Following the specified screening criteria, a total of 1,309 differentially expressed genes (DEGs) were identified in normal and tumor samples. Among these, 726 genes were downregulated, and 583 were upregulated (Supplementary Table S1). The distribution and characteristics of these DEGs are illustrated in a volcano plot (Figure 2A) and a heatmap (Figure 2B).
Figure 2. Differential gene expression analysis revealed significant variations between conjunctiva and pterygium. (A) Volcano diagram shows that red points indicate upregulated genes, while blue points indicate downregulated genes. Points with black circles represent genes meeting stricter criteria (P < 0.01 and |logFC| > 1), while points without black circles represent genes meeting standard criteria (P < 0.05 and |logFC| > 0.5). (B) DEGs heatmap shows the expression of the all upregulated and downregulated genes.
WGCNA was employed to identify key modules associated with glioblastoma. A sample clustering tree was constructed to visualize the dataset structure (Figure 3A), and the soft threshold was set to 10 to achieve scale-free topology (Figure 3B). After merging similar modules (Figure 3C), seven modules were identified, with their associations to disease traits depicted in Figure 3D. Among these, the MEbrown module showed strong positive correlations, while MEblack, MEturquoise, and MEyellow exhibited significant negative correlations with glioblastoma traits (P < 0.05). These four critical modules collectively encompassed 10,918 genes (Figure 3E; Supplementary Table S2). These modules provided an initial understanding of genes potentially associated with glioblastoma and were used for further analysis.
Figure 3. Identification of gene co-expression modules and their relationships with GBM traits. (A) The sample dendrogram and trait heatmap show the clustering of samples based on gene expression profiles. The trait heatmap highlights the correlation between samples and glioblastoma traits, aiding in identifying relevant modules for further analysis. (B) Scale independence analysis determines the soft threshold power (β) for constructing a scale-free network. The plot shows that a scale-free topology is achieved when β equals 10, ensuring the robustness of the network. (C) The dynamic tree cut method is used to identify initial gene modules, which are then merged based on similarity. The plot illustrates the merging of modules to create distinct clusters of commonly altered genes. (D) The module-trait relationship heatmap displays the correlation between identified modules and glioblastoma traits. Modules with strong positive or negative correlations are highlighted for further investigation. (E) The plot shows the genes assigned to each module and their respective module memberships. This provides an overview of gene clustering and their potential functional relevance.
Using the GeneCards database, genes associated with AD, PD, ALS, and MS were retrieved. Genes with a correlation score ≥1 were selected, and the intersection of these genes yielded 1,141 commonly altered genes for degenerative CNS diseases (Supplementary Table S3; Figure 4A).
Figure 4. Identification of shared genes between degenerative CNS diseases and glioblastoma. (A) Venn diagram shows the overlap of genes associated with AD, PD, MS, and ALS, revealing 1,141 commonly altered genes shared among these degenerative CNS diseases. (B) Venn diagram illustrates the intersection of glioblastoma-related WGCNA modules, DEGs, and the shared genes from degenerative CNS diseases, identifying 95 commonly altered genes as potential molecular links between glioblastoma and degenerative CNS diseases.
To investigate shared molecular mechanisms between glioblastoma and degenerative CNS diseases, we intersected three gene sets: (1) genes from the critical WGCNA modules, (2) DEGs in glioblastoma, and (3) degenerative CNS disease genes. This analysis identified 95 commonly altered genes (Figure 4B). These genes serve as a link between glioblastoma and degenerative CNS diseases, forming the basis for downstream functional and pathway analyses.
Functional enrichment analyses were performed to explore the roles of these 95 genes in glioblastoma and degenerative CNS diseases. Gene Ontology (GO) analysis revealed enrichment in biological processes (e.g., synaptic transmission, axon development), cellular components (e.g., myelin sheath, synaptic vesicles), and molecular functions (e.g., low-density lipoprotein receptor binding) (Figure 5A). KEGG analysis identified pathways such as neurodegeneration, ALS, PD, and proteoglycans in cancer as highly relevant to both glioblastoma and degenerative CNS diseases (Figure 5B). These pathways were visualized through a network diagram to elucidate their interconnected roles (Figures 5C, D). The results of these analyses provide insights into shared molecular mechanisms and highlight potential pathways for further exploration.
Figure 5. Functional enrichment analysis of commonly altered genes (A) GO enrichment analysis shows the biological processes, cellular components, and molecular functions significantly associated with the 95 commonly altered genes. Key enriched processes include synaptic transmission and axon development. (B) KEGG pathway analysis identifies significantly enriched pathways. (C) KEGG cnetplot visualizes the connections between key pathways and the commonly altered genes. (D) KEGG network plot depicts the relationships among enriched pathways, providing insights into the molecular mechanisms linking glioblastoma and degenerative CNS diseases.
Machine learning approaches were used to refine the identification of key genes. LASSO regression selected seven genes using cross-validation (Figure 6A). Random Forest analysis identified nine genes based on feature importance (Figures 6B, C), while SVM-RFE highlighted 33 genes with an accuracy of 0.867 (Figure 6D). By intersecting the results of these methods, two genes, RELN and GSTO2, emerged as shared biomarkers between glioblastoma and degenerative CNS diseases (Figure 7A). These two genes showed robust potential for diagnostic applications and were further validated in subsequent analyses.
Figure 6. Machine learning-based identification of key genes in glioblastoma and degenerative CNS diseases. (A) The LASSO regression plot shows the coefficient profiles of the genes as the regularization parameter (log λ) changes, with the binomial deviance plot identifying the optimal λ value (log λ = −3), where 7 genes were selected based on cross-validation, demonstrating high predictive performance for distinguishing disease states. (B) Random Forest model performance is illustrated by the error plot, where the minimum error stabilizes after approximately 50 trees, and the importance scores of the top 9 genes. (C) The Random Forest gene importance plot further emphasizes the contributions of top genes. (D) The SVM-RFE results depict cross-validation error and accuracy as a function of the number of selected genes, with 33 features achieving the lowest error (0.133) and highest accuracy (0.867), demonstrating the effectiveness of this method in selecting a concise yet predictive subset of genes.
Figure 7. Identification and validation of hub genes in glioblastoma and degenerative CNS diseases (A) The Venn diagram shows the overlap of hub genes identified by three machine learning methods (SVM-RFE, Random Forest, and LASSO), revealing two common hub genes: RELN and GSTO2. (B) The nomogram prediction model integrates the two hub genes (RELN and GSTO2) to calculate the total points and predict the likelihood of disease, providing a practical clinical tool. (C) The ROC curves for RELN and GSTO2 demonstrate their diagnostic performance in the training dataset, with AUC values of 0.982 and 0.981, respectively, indicating high accuracy. (D) Box plots show the expression levels of RELN and GSTO2 in tumor (P) and control (C) groups, with both genes showing significantly higher expression in the control group (p < 0.0001). (E) ROC curves for RELN and GSTO2 in the out-group validation dataset confirm their diagnostic performance, with AUC values of 0.895 and 0.894, respectively. (F) Box plots of the out-group validation dataset show consistent trends, with RELN and GSTO2 expression levels significantly higher in the control group compared to the glioblastoma group (p = 0.012 and p = 0.014, respectively).
To construct a hub gene nomogram model (Figure 7B), we assessed the diagnostic potential of RELN and GSTO2 using ROC curve analysis. In the primary dataset, both genes achieved an AUC of 1, demonstrating excellent diagnostic accuracy (Figure 7C). The expression levels of RELN and GSTO2 were significantly lower in glioblastoma samples compared to controls (Figure 7D). For external validation, we analyzed the GSE196533 dataset, where both genes also exhibited high diagnostic performance with AUC values exceeding 0.8 (Figure 7E). Similarly, expression levels of RELN and GSTO2 significantly decreased glioblastoma samples compared to controls (Figure 7F). Furthermore, regulatory network analysis, leveraging the ChEA and TarBase databases, identified key transcription factors and miRNAs that potentially interact with RELN and GSTO2 (Figure 8A).
Figure 8. Regulatory network and pathway enrichment analysis of RELN and GSTO2 in glioblastoma and degenerative CNS diseases. (A) The genes-TFs-miRNAs network illustrates the regulatory interactions among the two hub genes (RELN and GSTO2), their associated TFs, and miRNAs. (B) The ssGSEA analysis results for RELN and GSTO2 reveal their involvement in key biological pathways.
We performed single-gene GSEA analysis for each pivotal gene (RELN and GSTO2) and visualized the top five upregulated and downregulated pathways (Figure 8B). The results revealed that these genes are enriched in pathways such as DNA replication, GABAergic synapse, homologous recombination, and the synaptic vesicle cycle. Furthermore, they are associated with pathways related to various addictions, including morphine and nicotine addiction. These findings highlight the involvement of RELN and GSTO2 in both CNS functions and broader physiological processes. However, it is important to note that GO analysis identifies commonly associated mechanisms and does not confirm specific functional relationships in the context of disease. Experimental validation is required to establish such relationships.
To further understand the expression patterns of these genes, boxplots were generated to illustrate their distribution across different sample groups (Figure 9A). Compared to control samples, glioblastoma samples exhibited increased expression of pathways such as coagulation, p53 signaling, epithelial-mesenchymal transition, and MYC targets V1/V2. These pathways, which are linked to tumor progression, underscore the potential roles of RELN and GSTO2 in glioblastoma biology.
Figure 9. ssGSEA analysis and correlation of RELN and GSTO2 with hallmark pathways. (A) Boxplots illustrate the differences in ssGSEA pathway enrichment scores between glioblastoma (P) and control (C) samples. (B) Heatmap displays the Spearman correlation analysis between hallmark pathways and hub gene expression levels. (C) Heatmap of gene-pathway correlations provides an intuitive depiction of the relationships between RELN, GSTO2, and hallmark pathways.
We also examined the correlations between hub gene expression levels and hallmark pathways to explore potential regulatory linkages. Spearman correlation analysis revealed that RELN and GSTO2 expression positively correlates with pathways such as spermatogenesis, heme metabolism, and oxidative phosphorylation, while negatively correlating with pathways like unfolded protein response, TNFA signaling via NFKB, p53 signaling, and MYC targets (Figure 9B). These correlations provide insights into the functional roles of these genes in distinct biological processes and their potential involvement in cancer development. The relationship between these pathways and hub gene expression is further depicted in a heatmap for intuitive visualization (Figure 9C), supporting the hypothesis that RELN and GSTO2 are critical mediators at the intersection of glioblastoma and degenerative CNS disease mechanisms.
Nearly one-sixth of the world's population is affected by CNS diseases (Zhou et al., 2021). These disorders range from mild neurological impairment (which may manifest as motor, sensory, visual, speech, cognitive impairment, or a combination of these symptoms) to more severe conditions, such as coma and brain death. The central nervous system, including the brain and spinal cord, carries important tasks for processing sensory information, controlling movement, and coordinating all higher functions of the body, such as thinking, memory, and affect (Capani et al., 2016).
In many CNS diseases, ongoing progressive neurological damage is often caused by primary neuronal dysfunction. However, not all central nervous system disorders are degenerative. For example, although brain trauma or stroke affects the CNS, they are usually acute events and differ from chronic degenerative processes (Campbell and Khatri, 2020). In contrast, some CNS diseases such as Alzheimer's disease, Parkinson’s disease, and multiple sclerosis are indeed degenerative, and their main features are gradual deterioration in the structure and function of nerve cells, particularly neurons (Fan and Huo, 2021). These degenerative diseases progress over time, and damage and death of nerve cells lead to various dysfunctions. For example, cognitively function-associated neurons are particularly affected in Alzheimer's disease (Tiwari et al., 2019). Pathological mechanisms of these diseases may include factors such as abnormal protein folding, mitochondrial and energy metabolism disorders (Ferrer, 2018), oxidative stress (Singh et al., 2019), inflammation (Voet et al., 2019), and imbalances in neurotransmitter systems (Mahalakshmi et al., 2020). These complex pathological processes not only lead to the decline and/or death of neuronal function, but also further affect the overall function of the brain and spinal cord.
In 2014, GBM represented 16% of all primary brain and central nervous system tumors. The age-adjusted mean incidence rate was 3.19 per 100,000 (Thakkar et al., 2014). By 2023, however, the incidence of malignant brain tumors reached an alarming 7 cases per 100,000 and increased with age. The 5-year survival rate is approximately 36%. Older patients have a lower survival rate than younger patients. Survival rates ranged from approximately 71.5% in patients aged 15–39 years and 21% in patients aged 40 years and older. About 49% of these primary malignant brain tumors are glioblastomas (Schaff and Mellinghoff, 2023). Glioblastoma is a very aggressive brain tumor classified as grade IV in malignant glioma, which is the most severe grade. This tumor is characterized by rapid growth and high invasiveness to surrounding brain tissue. Symptoms of GBM include headache, seizures, neurocognitive impairment, and focal neurological deficits (Ostrom et al., 2022).
Recent research has unveiled a novel therapeutic strategy against cancer, drawing inspiration from treatments for neurodegenerative disorders (Soragni et al., 2016). This approach involves the development of ReACp53, a designed inhibitor that targets the aggregation of the p53 protein, a common pathological feature in various cancers, notably ovarian carcinomas. By preventing p53 aggregation, ReACp53 restores its tumor-suppressive function, leading to a reduction in tumor growth and an increase in cancer cell apoptosis. This breakthrough highlights the potential of leveraging neurodegenerative disease therapies to combat cancer, offering a promising avenue for future cancer treatment research.
Our study employed a novel approach that integrates WGCNA, multiple machine learning algorithms, and functional enrichment analyses to identify biomarkers shared between GBM and degenerative CNS diseases. This integrated pipeline offers several advantages over conventional methods.
First, traditional biomarker discovery methods often rely on a single dataset or apply univariate statistical methods to identify significant genes. These approaches may overlook subtle but biologically important interactions and fail to generalize across datasets. By contrast, our method combines data from multiple independent datasets, ensuring robustness and minimizing potential biases. The WGCNA framework, in particular, enables the identification of gene modules based on co-expression patterns, providing a systems-level understanding of disease-associated networks that traditional differential expression analyses cannot capture (Oluwatosin A et al., 2025).
To further refine the identification of key candidate genes from the WGCNA results, we integrated three machine learning techniques: SVM-RFE, LASSO, and Random Forest. Each method contributes unique strengths to the selection process. SVM-RFE is well-known for its ability to select relevant features while minimizing the risk of overfitting, making it particularly useful for analyzing datasets with a high number of variables (Hector et al., 2018). LASSO is effective in both feature selection and regularization, promoting models that are sparse, interpretable, and generalizable (Maike and Andreas, 2024). Random Forest, on the other hand, is highly precise in handling large datasets, managing data imbalance effectively, and providing valuable insights into feature importance (Ibrahim et al., 2024). By combining these methodologies, we capitalize on the unique strengths of each: LASSO’s simplicity and interpretability, SVM-RFE’s adaptability to complex datasets, and Random Forest’s comprehensive evaluation of feature relevance. This integrated strategy ensures thorough and reliable identification of crucial genes, enhancing the predictive capabilities and robustness of our analysis.
Traditional methods often neglect downstream functional validation at the pathway level, limiting the understanding of the broader biological context of identified biomarkers. In this study, we addressed this limitation by incorporating functional enrichment analyses (GO and KEGG) to link the identified biomarkers with specific biological processes and pathways. This approach not only strengthens the biological relevance of the biomarkers but also provides insights into their potential roles in disease mechanisms, paving the way for future experimental validation (Nguyen et al., 2024).
Building on these analyses, we further explored the biological context of the identified hub genes using the NetworkAnalyst platform, an online tool for comprehensive data analysis (Guangyan et al., 2019). This platform provided access to resources such as the ChEA database to predict TFs associated with the hub genes and the TarBase database to identify miRNAs linked to these genes. By integrating these analyses, our approach enhances the understanding of the pathways and regulatory networks in which the hub genes operate, shedding light on the potential mechanisms through which they influence disease processes.
When we delve into diseases of the central nervous system, we find unexpected associations between GBM and degenerative CNS diseases. Although there are clear differences in clinical presentation and pathogenesis between these two types of diseases, they show striking similarities in certain critical biological processes. Ferroptosis, as a key mode of cell death, plays a crucial role in both diseases. In degenerative neurological diseases such as PD, iron deposition and consequent oxidative damage are among the critical pathological processes (Martin-Bastida et al., 2017; Mitre et al., 2022). Further studies have shown that intervening in the metabolic pathway of iron can not only bring new therapeutic strategies for Parkinson’s disease (Wang et al., 2023), but also provide new ideas for the treatment of glioblastoma.
Lipid rafts serve as dynamic hubs for integrating signaling events in both tumor and neuronal cells, including those associated with RELN and Wnt signaling (Yunden and Ven, 2024). These lipid-rich microdomains play a pivotal role in organizing and coordinating the interaction of various signaling pathways, including the Wnt pathway, which intersects with RELN signaling through LRP6. This intersection is particularly significant in both tumor progression and neurodevelopmental processes (Lenz et al., 2024). In the case of tumors, including GBM, lipid rafts enable the integration of multiple pro-survival and growth-promoting signals, enhancing cellular responses to external stimuli (Reza et al., 2023; Pampa et al., 2024). Similarly, in neuronal cells, lipid rafts facilitate the RELN-mediated signaling crucial for processes like neuronal migration and synaptic plasticity, by coordinating the activation of downstream molecules such as Dab1 (Matthew et al., 2022). Additionally, oxidative stress is modulated within these lipid domains, influencing cellular fate decisions by affecting both Wnt and RELN pathways (Akira et al., 2024). Alterations in lipid raft composition or function have been shown to disrupt this integration, contributing to both tumorigenesis (Momoko et al., 2023) and neurodegeneration (Yi Sak et al., 2024). Therefore, lipid rafts are indispensable for coordinating complex signaling networks in these vital biological processes.
While glioblastoma and degenerative central nervous system diseases differ fundamentally in their molecular mechanisms and pathological outcomes—such as cell death in degenerative CNS diseases (Salem et al., 2025) versus cell proliferation and resistance to cell death in cancer (Talvard-Balland et al., 2024)—our findings suggest that certain molecular pathways, such as those involving iron metabolism, may be shared (Brown et al., 2024). This observation broadens our understanding of these distinct neurological disorders and suggests potential overlapping therapeutic targets. Based on this, we investigated markers common to degenerative CNS diseases and GBM, identifying RELN and GSTO2 as key candidates.
RELN is a large extracellular matrix protein first identified during brain development, crucial for regulating neuronal migration and cortical stratification. It involves processes like neuronal migration, dendritic outgrowth, dendritic spine formation, synapse generation, and synaptic plasticity (Khialeeva and Carpenter, 2017). Research has shown that RELN is expressed in the adult brain and plays roles in neuronal plasticity, memory formation, and various neurological diseases. Additionally, RELN impacts the immune system, liver fibrosis, and cancers (Canet-Pons et al., 2018).
In addition to their general role in cellular signaling, lipid rafts are essential for the precise regulation of the RELN/ApoER2 signaling pathway, which is crucial for neuronal migration, synaptic plasticity, and neurodevelopment (Ling Xiao et al., 2024). These lipid-enriched microdomains serve as platforms that facilitate the clustering of signaling receptors such as ApoER2, enhancing the signal transduction efficiency. The interaction between RELN and ApoER2 within lipid rafts has been shown to modulate the activation of downstream signaling molecules, including Dab1, a key kinase involved in neuronal migration (Mitsuki et al., 2024). Recent study had demonstrated that lipid rafts play a pivotal role in ensuring that this signaling cascade is both robust and specific, thereby facilitating the proper formation of neural networks (Harald et al., 2005). Moreover, lipid rafts may influence the trafficking and internalization of ApoER2 receptors, which is necessary for effective signal initiation.
Disruption of lipid rafts can lead to a failure in receptor clustering and signaling efficiency, impairing essential neurodevelopmental processes like synaptogenesis and axon guidance. Emerging evidence suggests that such disruption could contribute to various degenerative CNS diseases by altering synaptic integrity and neuronal survival. Notably, research on AD and other neurodegenerative conditions has revealed that the perturbation of lipid raft domains can impair RELN/ApoER2 signaling, resulting in deficits in memory, learning, and neuroplasticity (Christina M et al., 2024). Furthermore, alterations in lipid raft composition and function are thought to affect the balance between neuroprotection and neurodegeneration, implicating lipid rafts as a key player in both disease onset and progression. These findings underscore the critical role of lipid rafts not only in the RELN/ApoER2 pathway but also in the overall maintenance of neuronal function and resilience (S Sakura et al., 2011).
RELN expression and function are crucial in degenerative CNS diseases such as AD, PD, and Huntington’s disease. Research indicates that RELN expression is typically decreased in these diseases, contributing to pathological processes such as the loss of neuronal connectivity, neuronal death, and cognitive decline. These findings suggest that RELN’s functional activity may be inhibited, further exacerbating disease progression. (Lidón et al., 2020). RELN’s mechanism involves neurodevelopment and neuronal plasticity. Core components of the RELN pathway include VLDLR, ApoER2, Src family kinases, and Dab1 (Jossin, 2020). RELN binds to its receptors (LRP1/2 and ApoER2/VLDLR), activating downstream pathways like Dab1. This activation is essential for regulating intracellular calcium levels, reorganizing the cytoskeleton, and maintaining synapses (Bock and May 2016). In AD and other neurodegenerative disorders, reduced RELN signaling can alter synaptic structure and function, affecting neural network stability and plasticity, leading to cognitive decline. Reduced RELN signaling may impair NMDA receptor function, crucial for learning and memory (Joly-Amado et al., 2023).
The role of RELN in tumors is complex. Research shows that it plays a role in regulating the invasion and proliferation of cancer, and inhibits the migration and invasion of pancreatic cancer cells (Ramaker et al., 2017). The expression of RELN decreased in breast cancer, colorectal cancer and pancreatic cancer, but increased in retinoblastoma, myeloma and prostate cancer (Ndoye et al., 2021; Biamonte et al., 2021; Seigel et al., 2007; Qin et al., 2017). Some studies have found that the expression level of RELN in GBM is different from that of normal brain tissue or other types of brain tumors, which may be related to tumor invasiveness, growth rate, and treatment response. (Perrone et al., 2007). Moreover, recent studies have suggested that inhibiting heparanase, an enzyme involved in the remodeling of the extracellular matrix (Minghong et al., 2025), may significantly affect the regulation of autophagy and apoptosis in glioblastoma (Valeria et al., 2023). Heparanase inhibition has been shown to alter the balance between cell survival and cell death by modulating key signaling pathways involved in these processes (Vy M et al., 2017). By interfering with the autophagic flux and promoting apoptosis, heparanase inhibitors may enhance the sensitivity of glioblastoma cells to therapeutic agents, providing a promising approach for treatment strategies (Carolyn G and Renato V, 2022).
In GBM, the expression of RELN and its main downstream effector molecule Dab1 is inhibited, and mRNA expression is inversely proportional to the degree of malignancy (Talebian et al., 2019). In addition, RELN expression was positively associated with survival in patients in two large independent clinical annotation datasets. Silencing of RELN occurs through promoter hypermethylation (Serrano-Morales et al., 2017). At the functional level, RELN regulates GBM cell migration in a Dab1 (tyrosine phosphorylation) dependent and non dependent manner, depending on the substrate provided. Activation of RELN signaling significantly reduces the proliferation of GBM cells, a phenotype dependent on RELN stimulation of Dab1. Mutants lacking all RELN induced tyrosine phosphorylation sites (DAB1-5F) failed to induce growth arrest. Proteomic analysis shows that these effects are mediated by reducing the dephosphorylation of E2F targets and ERK1/2. RELN may also indirectly affect tumor growth and spread by affecting astrocytes and microglia in the tumor microenvironment. This interaction is achieved by altering the secretion of cytokines and chemokines, thereby affecting the interaction between tumor cells and their microenvironment (Jandial et al., 2017; Zeng et al., 2023).
GSTO2 is a member of GST family, belonging to the Omega subfamily (Peng et al., 2023). This family plays a critical role in cellular detoxification processes. GST enzymes, found in animals, plants, and microorganisms, protect cells from oxidative stress and chemical damage by facilitating the conjugation of glutathione with various electrophilic compounds, including drugs, environmental toxins, and metabolic by-products, thereby promoting their excretion (Zhang et al., 2013).
Degenerative CNS diseases are often associated with increased oxidative stress and inflammation (Schmuck et al., 2005). Oxidative stress during these diseases can lead to cellular damage and death, accelerating disease progression. GSTO2, through its antioxidative activity, may help neutralize excess free radicals and reactive oxygen species, alleviating oxidative stress-induced damage to neurons and other brain cells. Studies suggest a significant correlation between GSTO2 and the age of onset in AD and PD (Ozturk et al., 2005). Cha et al. (2023) found that knocking down the GSTO2 gene in fruit flies leads to an age-related increase in Cabeza protein levels in neurons. Cabeza, a homolog of the human FUS protein associated with degenerative CNS diseases like ALS and Frontotemporal Dementia, shows increased mislocalization and aggregation in the cytoplasm of neurons and reduced solubility in aging neurons when GSTO2 is knocked out. This suggests GSTO2 plays a crucial role in regulating Cabeza localization and aggregation, potentially impacting neurodegenerative disease development (Machamer et al., 2018).
Abnormal expression of GSTO2 is also related to the occurrence and development of tumors. Interestingly, GSTO2 exhibits a bidirectional regulatory role in tumors. Several studies (Peng et al., 2023; Masoudi et al., 2009; Djukic et al., 2015) have discussed the association of GSTO2 with cancers. Sumiya R (Sumiya et al., 2022) revealed that GSTO2 is uniquely expressed in various lung stem cells but silenced in lung squamous cell carcinoma (LSCC) due to DNA hypermethylation. GSTO2 regulates cell growth, β-catenin expression, and mitochondrial respiration through p38 phosphorylation. Activation of p38 MAPK by GSTO2 leads to the downregulation of β-catenin, likely via ubiquitination, contributing to lung tissue homeostasis and preventing malignant transformation. Loss of GSTO2 in LSCC disrupts this mechanism, allowing β-catenin to avoid degradation, leading to increased mitochondrial oxidative phosphorylation and enhanced energy production, promoting LSCC growth. These findings highlight the potential importance of GSTO2 in preventing LSCC and provide insights into lung carcinogenesis pathways. However, the specific role and mechanism of GSTO2 in GBM require further investigation. It is hypothesized that GSTO2, by regulating oxidative stress responses and metabolic processes, could influence GBM cell survival, proliferation, and treatment response. Upregulation of GSTO2 might enhance tumor cells’ ability to clear chemotherapeutic drugs, reducing treatment efficacy. Conversely, reducing GSTO2 activity or expression could increase oxidative stress, promoting cancer cell death, and potentially serving as a therapeutic strategy.
Although RELN and GSTO2 have different functions and regulatory mechanisms, studies suggest they may have potential interrelated mechanisms in certain diseases. RELN plays a crucial role in neural development and synaptic plasticity, and its abnormal expression is associated with degenerative CNS diseases. RELN combats oxidative stress by regulating synapse formation and stability (Sajjad et al., 2014), while GSTO2 is important in the cellular antioxidant response, helping to neutralize excess free radicals and ROS, thereby reducing neuronal damage (Lauren E et al., 2019). RELN and GSTO2 may jointly regulate oxidative stress and inflammatory responses in neurons, protecting them from damage. Additionally, RELN affects the stability and plasticity of neural networks by regulating synapse structure and function (Murat S et al., 2009), whereas GSTO2, through its antioxidant activity, reduces apoptosis and autophagy induced by oxidative stress, thus protecting neuronal survival. They may co-operate in the pathways of neuronal apoptosis and autophagy, maintaining normal neuronal function and survival (Xifeng et al., 2022). The signaling pathways involving RELN and GSTO2 may interact at certain points; for instance, RELN influences intracellular calcium levels by regulating synaptic function and stability (Yehui et al., 2019), while GSTO2 maintains cellular homeostasis by mitigating oxidative stress (Tatjana et al., 2022). They may influence each other in cellular signal transduction, jointly maintaining neuronal function and survival.
Clinically, our findings indicate that RELN and GSTO2 show significant expression changes in GBM, suggesting their potential utility as biomarkers for this disease. Specifically, the observed differential expression of these genes in GBM samples compared to controls highlights their diagnostic relevance. However, we acknowledge that further experimental and clinical validation is essential to substantiate their roles in early diagnosis, disease progression monitoring, and treatment response evaluation.
Regarding neurodegenerative CNS diseases, we did not evaluate RELN and GSTO2 in relevant cohorts; thus, their potential roles in neurodegenerative CNS diseases remain speculative. While shared molecular mechanisms between neurodegenerative CNS diseases and GBM suggest possible connections, we refrain from making definitive claims about their biomarker or therapeutic potential in these diseases without supporting evidence. Future research should validate these findings in disease-specific cohorts and investigate whether RELN and GSTO2 could serve as progression markers or therapeutic targets for GBM and other CNS diseases.
This study involves collecting cross-gene data for AD, PD, MS, and ALS from the GeneCards database, and performing differential expression analysis of GBM using GEO datasets. Machine learning is used to analyze these data, aiming to find common molecular biomarkers between degenerative CNS diseases and GBM. This approach could uncover novel biomarkers and shared pathological processes in these disorders, guiding future therapy strategies. The study integrates molecular characteristics with clinical features to improve understanding of these disorders and to identify molecular targets for diagnosis, monitoring, and treatment. The results could also support personalized medicine based on molecular mechanisms. In this study, we identified co-expressed biomarkers for degenerative CNS diseases and GBM: RELN and GSTO2. Validated experimental results indicated that, in GBM patient samples, the expression levels of RELN and GSTO2 were significantly reduced compared to healthy controls. Based on these findings, we hypothesize that RELN and GSTO2 may act as protective factors against the development of these neurological disorders.
Overall, the study seeks to advance the diagnosis, treatment, and prognosis of degenerative CNS diseases and GBM by identifying biomarkers, using cross-disease analysis, and applying machine learning, potentially benefiting patients.
The original contributions presented in the study are included in the article/Supplementary Material, further inquiries can be directed to the corresponding author.
FB: Writing–original draft, Writing–review and editing, Conceptualization. JZ: Writing–original draft, Writing–review and editing. RG: Validation, Visualization, Writing–original draft, Writing–review and editing.
The author(s) declare that no financial support was received for the research and/or publication of this article.
The authors declare that the research was conducted in the absence of any commercial or financial relationships that could be construed as a potential conflict of interest.
The author(s) declare that no Generative AI was used in the creation of this manuscript.
All claims expressed in this article are solely those of the authors and do not necessarily represent those of their affiliated organizations, or those of the publisher, the editors and the reviewers. Any product that may be evaluated in this article, or claim that may be made by its manufacturer, is not guaranteed or endorsed by the publisher.
The Supplementary Material for this article can be found online at: https://www.frontiersin.org/articles/10.3389/fmolb.2025.1506961/full#supplementary-material
SUPPLEMENTARY TABLE S1DEGs.
SUPPLEMENTARY TABLE S2Genes of WGCNA.
SUPPLEMENTARY TABLE S3Degenerative CNS diseases.
Akira, U., Kazuyoshi, K., Maki, M., Hiroo, F., Reiko, Y., Kyoko, F., et al. (2024). Palmitic acid induces insulin resistance and oxidative stress-mediated cell injury through accumulation of lipid rafts in murine hepatocytes. Biochem. Biophys. Res. Commun. 745. doi:10.1016/j.bbrc.2024.151242
Ana Helena Larangeira, N., Rafael Sampaio, P., Ana Paula, P., Jenifer, G., Rudimar Luiz, F., and Andressa, B. (2024). Neuroinflammation in glioblastoma: the role of the microenvironment in tumour progression. Curr. Cancer Drug Targets 24, 579–594. doi:10.2174/0115680096265849231031101449
Andrea J, M., Marlon, C., Lili, H., Philippe P, M., Yi-Fang, C., Bernhard K, M., et al. (2020). Delayed administration of the human anti-RGMa monoclonal antibody elezanumab promotes functional recovery including spontaneous voiding after spinal cord injury in rats. Neurobiol. Dis. 143, 104995. doi:10.1016/j.nbd.2020.104995
Ashraf, D., Desiree, R., Timothy L, M., Nicholas M, F., Jacob M, P., and Mufeed M, B. (2023). Endocannabinoids are potential inhibitors of glioblastoma multiforme proliferation. J. Integr. Med. 21, 120–129. doi:10.1016/j.joim.2023.01.005
Biamonte, F., Sica, G., Filippini, A., and D'Alessio, A. (2021). Evidence of reelin signaling in GBM and its derived cancer stem cells. Brain Sci. 11, 745. doi:10.3390/brainsci11060745
Bock, H., and May, P. (2016). Canonical and non-canonical reelin signaling. Front. Cell. Neurosci. 10, 166. doi:10.3389/fncel.2016.00166
Broekman, M., Maas, S., Abels, E., Mempel, T., Krichevsky, A., and Breakefield, X. (2018). Multidimensional communication in the microenvirons of glioblastoma. Nat. Rev. Neurol. 14, 482–495. doi:10.1038/s41582-018-0025-8
Brown, A., Hirschhorn, T., and Stockwell, B. (2024). Ferroptosis-disease perils and therapeutic promise. Sci. (New York, N.Y.) 386, 848–849. doi:10.1126/science.adn7030
Campbell, B., and Khatri, P. (2020). Stroke. Lancet London, Engl. 396, 129–142. doi:10.1016/S0140-6736(20)31179-X
Canet-Pons, J., Schubert, R., Duecker, R., Schrewe, R., Wölke, S., Kieslich, M., et al. (2018). Ataxia telangiectasia alters the ApoB and reelin pathway. Neurogenetics 19, 237–255. doi:10.1007/s10048-018-0557-5
Capani, F., Quarracino, C., Caccuri, R., and Sica, R. (2016). Astrocytes as the main players in primary degenerative disorders of the human central nervous system. Front. aging Neurosci. 8, 45. doi:10.3389/fnagi.2016.00045
Carolyn G, C., and Renato V, I. (2022). Extracellular matrix guidance of autophagy: a mechanism regulating cancer growth. Open Biol. 12, 210304. doi:10.1098/rsob.210304
Cha, S., Yoon, J., Han, Y., and Kim, K. (2023). Knockdown of glutathione S-transferase leads to mislocalization and accumulation of cabeza, a drosophila homolog of FUS, in the brain. J. neurogenetics 37, 20–24. doi:10.1080/01677063.2022.2149747
Christina M, G., Sabrina A, K., Anna, N., Adam T, L., Uwe, B., and Angela, H. (2024). APOER2 splicing repertoire in Alzheimer's disease: insights from long-read RNA sequencing. PLoS Genet. 20, e1011348. doi:10.1371/journal.pgen.1011348
Cui, M., Cheng, C., and Zhang, L. (2022). High-throughput proteomics: a methodological mini-review. Laboratory investigation; a J. Tech. methods pathology 102, 1170–1181. doi:10.1038/s41374-022-00830-7
Djukic, T., Simic, T., Radic, T., Matic, M., Pljesa-Ercegovac, M., Suvakov, S., et al. (2015). GSTO1*C/GSTO2*G haplotype is associated with risk of transitional cell carcinoma of urinary bladder. Int. urology Nephrol. 47, 625–630. doi:10.1007/s11255-015-0933-0
Fan, Y., and Huo, J. (2021). A1/A2 astrocytes in central nervous system injuries and diseases: angels or devils? Neurochem. Int. 148, 105080. doi:10.1016/j.neuint.2021.105080
Ferrer, I. (2018). Oligodendrogliopathy in neurodegenerative diseases with abnormal protein aggregates: the forgotten partner. Prog. Neurobiol. 169, 24–54. doi:10.1016/j.pneurobio.2018.07.004
Gangfuß, A., Schara-Schmidt, U., and Roos, A. (2022). Genomics and proteomics in the research of neuromuscular diseases. Der Nervenarzt 93, 114–121. doi:10.1007/s00115-021-01201-1
Gauthier, J., Vincent, A., Charette, S., and Derome, N. (2019). A brief history of bioinformatics. Briefings Bioinforma. 20, 1981–1996. doi:10.1093/bib/bby063
Guangyan, Z., Othman, S., Jessica, E., Robert E W, H., Niladri, B., and Jianguo, X. (2019). NetworkAnalyst 3.0: a visual analytics platform for comprehensive gene expression profiling and meta-analysis. Nucleic Acids Res. 47, W234-W241. doi:10.1093/nar/gkz240
Harald, M., Sarah, D., Christoph, H., Wolfgang J, S., and Johannes, N. (2005). Reconstitution of the Reelin signaling pathway in fibroblasts demonstrates that Dab1 phosphorylation is independent of receptor localization in lipid rafts. Mol. Cell Biol. 26. doi:10.1128/MCB.26.1.19-27.2006
Hector, S., Clarissa, V., Esteban, V., Josep M, O., and Ferran, R. (2018). SVM-RFE: selection and visualization of the most relevant features through non-linear kernels. BMC Bioinforma. 19, 432. doi:10.1186/s12859-018-2451-4
Hrdlickova, R., Toloue, M., and Tian, B. (2017). RNA-Seq methods for transcriptome analysis. Wiley Interdiscip. Rev. RNA 8. doi:10.1002/wrna.1364
Ibrahim, S., Kelly M, P., Nabil M, A. J., and Ghaleb A, H. (2024). Predicting calcein release from ultrasound-targeted liposomes: a comparative analysis of random forest and support vector machine. Technol. Cancer Res. Treat. 23, 15330338241296725. doi:10.1177/15330338241296725
Isidro, F. (2018). Oligodendrogliopathy in neurodegenerative diseases with abnormal protein aggregates: the forgotten partner. Prog. Neurobiol. 169, 24–54. doi:10.1016/j.pneurobio.2018.07.004
Jandial, R., Choy, C., Levy, D., Chen, M., and Ansari, K. (2017). Astrocyte-induced Reelin expression drives proliferation of Her2+ breast cancer metastases. Clin. and Exp. metastasis 34, 185–196. doi:10.1007/s10585-017-9839-9
Jian, S., and Shiwei, H. (2024). Comparative insight into microglia/macrophages-associated pathways in glioblastoma and alzheimer's disease. Int. J. Mol. Sci. 25. doi:10.3390/ijms25010016
Joly-Amado, A., Kulkarni, N., and Nash, K. (2023). Reelin signaling in neurodevelopmental disorders and neurodegenerative diseases. Brain Sci. 13, 1479. doi:10.3390/brainsci13101479
Jossin, Y. (2020). Reelin functions, mechanisms of action and signaling pathways during brain development and maturation. Biomolecules 10, 964. doi:10.3390/biom10060964
Khialeeva, E., and Carpenter, E. (2017). Nonneuronal roles for the reelin signaling pathway. Dev. Dyn. official Publ. Am. Assoc. Anatomists 246, 217–226. doi:10.1002/dvdy.24462
Lenz, H. J., Argilés, G., de Jonge, M. J. A., Yaeger, R., Doi, T., El-Khoueiry, A., et al. (2024). A phase I dose-escalation study of LRP5/6 antagonist BI 905677 in patients with advanced solid tumors. ESMO Open 9, 103729. doi:10.1016/j.esmoop.2024.103729
Lauren E, H., Michal, Z., Jiude, M., Wei, X., Peter, S., Cristian, O. F., et al. (2019). GSTO2 isoforms participate in the oxidative regulation of the plasmalemma in eutherian spermatozoa during capacitation. Antioxidants (Basel) 8, 601. doi:10.3390/antiox8120601
Lauryn E, H., Christine A, P., David N, K., Jennifer N, S., Susan, L., Robert G, H., et al. (2017). Palliative and end-of-life care in glioblastoma: defining and measuring opportunities to improve care. Neurooncol Pract. 4, 182–188. doi:10.1093/nop/npw022
Lidón, L., Urrea, L., Llorens, F., Gil, V., Alvarez, I., Diez-Fairen, M., et al. (2020). Disease-specific changes in reelin protein and mRNA in neurodegenerative diseases. Cells 9, 1252. doi:10.3390/cells9051252
Ling Xiao, Y., Li, Z., Qing, W., Eng King, T., and Zhi Dong, Z. (2024). Reelin links apolipoprotein E4, tau, and amyloid-β in alzheimer’s disease. Ageing Res. Rev. 98, 102339. doi:10.1016/j.arr.2024.102339
Lu, P., Chenqing, Z., Zhijian, Y., Yudi, P., Trung Nghia, V., and Xia, S. (2023). Hidden genetic regulation of human complex traits via brain isoforms. Phenomics 3, 217–227. doi:10.1007/s43657-023-00100-6
Machamer, J., Woolums, B., Fuller, G., and Lloyd, T. (2018). FUS causes synaptic hyperexcitability in Drosophila dendritic arborization neurons. Brain Res. 1693, 55–66. doi:10.1016/j.brainres.2018.03.037
Mahalakshmi, B., Maurya, N., Lee, S., and Bharath Kumar, V. (2020). Possible neuroprotective mechanisms of physical exercise in neurodegeneration. Int. J. Mol. Sci. 21, 5895. doi:10.3390/ijms21165895
Maike, H., and Andreas, G. (2024). A flexible adaptive lasso cox frailty model based on the full likelihood. Biom J. 66, e202300020. doi:10.1002/bimj.202300020
Martin-Bastida, A., Ward, R., Newbould, R., Piccini, P., Sharp, D., Kabba, C., et al. (2017). Brain iron chelation by deferiprone in a phase 2 randomised double-blinded placebo controlled clinical trial in Parkinson's disease. Sci. Rep. 7, 1398. doi:10.1038/s41598-017-01402-2
Masoudi, M., Saadat, I., Omidvari, S., and Saadat, M. (2009). Genetic polymorphisms of GSTO2, GSTM1, and GSTT1 and risk of gastric cancer. Mol. Biol. Rep. 36, 781–784. doi:10.1007/s11033-008-9245-0
Matthew, B.-S., Ganna, L., Emily, B., Karen, C., Andrew C, G., Dervis A, S., et al. (2022). Whole genome analysis in APOE4 homozygotes identifies the DAB1-RELN pathway in Alzheimer's disease pathogenesis. Neurobiol. Aging 119, 67–76. doi:10.1016/j.neurobiolaging.2022.07.009
McDermaid, A., Monier, B., Zhao, J., Liu, B., and Ma, Q. (2019). Interpretation of differential gene expression results of RNA-seq data: review and integration. Briefings Bioinforma. 20, 2044–2054. doi:10.1093/bib/bby067
Minghong, N., Michela, P., Emiliano, E., Margherita, D., Ornela, C., Laura, M., et al. (2025). Synthetic glycol-split heparin tri- and tetrasaccharides provide new insights into structural peculiarities for antiheparanase activity. Bioorg Med. Chem. 118, 118052. doi:10.1016/j.bmc.2024.118052
Mitre, A., Florian, A., Buruiana, A., Boer, A., Moldovan, I., Soritau, O., et al. (2022). Ferroptosis involvement in glioblastoma treatment. Med. Kaunas. Lith. 58, 319. doi:10.3390/medicina58020319
Mitsuki, H., Keisuke, I., Mitsuharu, H., and Takao, K. (2024). EphA4 induces the phosphorylation of an intracellular adaptor protein Dab1 via Src family kinases. Biol. Pharm. Bull. 47, 1314–1320. doi:10.1248/bpb.b24-00273
M, L., J-X, Y., W-X, Z., F-X, L., and H-C, H. (2024). Roles of TREM2 in the pathological mechanism and the therapeutic strategies of alzheimer's disease. J. Prev. Alzheimers Dis. 11, 1682–1695. doi:10.14283/jpad.2024.164
Momoko, G., Yi, L., Rina, F.-T., Shiori, M., Ruiko, O., Shingo, K., et al. (2023). Lovastatin treatment inducing apoptosis in human pancreatic cancer cells by inhibiting cholesterol rafts in plasma membrane and mitochondria. Int. J. Mol. Sci. 24, 16814. doi:10.3390/ijms242316814
Murat S, D., Ying, C., Charles L, W., Ege T, K., and Joachim, H. (2009). Reelin signaling antagonizes beta-amyloid at the synapse. Proc. Natl. Acad. Sci. U. S. A. 106, 15938–15943. doi:10.1073/pnas.0908176106
Ndoye, A., Miskin, R., and DiPersio, C. (2021). Integrin α3β1 represses reelin expression in breast cancer cells to promote invasion. Cancers 13, 344. doi:10.3390/cancers13020344
Nguyen, H., Pham, V., Nguyen, H., Tran, B., Petereit, J., and Nguyen, T. (2024). CCPA: cloud-based, self-learning modules for consensus pathway analysis using GO, KEGG and Reactome. Briefings Bioinforma. 25, bbae222. doi:10.1093/bib/bbae222
Oluwatosin A, O., Nicholas K, O. N., Jenny A, E., Payton, B., Junming, H., Melissa, W., et al. (2025). Basic science and pathogenesis. Alzheimers Dement. doi:10.1002/alz.088905
Ostrom, Q., Price, M., Ryan, K., Edelson, J., Neff, C., Cioffi, G., et al. (2022). CBTRUS statistical report: pediatric brain tumor foundation childhood and adolescent primary brain and other central nervous system tumors diagnosed in the United States in 2014-2018. Neuro-oncology 24, iii1–iii38. doi:10.1093/neuonc/noac161
Ozturk, A., Desai, P., Minster, R., Dekosky, S., and Kamboh, M. (2005). Three SNPs in the GSTO1, GSTO2 and PRSS11 genes on chromosome 10 are not associated with age-at-onset of Alzheimer's disease. Neurobiol. aging 26, 1161–1165. doi:10.1016/j.neurobiolaging.2004.11.001
Pampa, P., Ashutosh, T., and Prakash P, P. (2024). Involvement of PDGFR-integrin interactions in the regulation of anoikis resistance in glioblastoma progression. Cell Biol. Int. 49, 3–15. doi:10.1002/cbin.12257
Pei-Lun, X., Meng-Yu, Z., Ran, H., Wei-Xin, C., and Jin-Hua, M. (2024). Pharmacological mTOR inhibitors in ameliorating Alzheimer's disease: current review and perspectives. Front. Pharmacol. 15, 1366061. doi:10.3389/fphar.2024.1366061
Peng, X., Zhu, J., Liu, S., Liu, Z., Luo, C., Wu, X., et al. (2023). The upregulation of GSTO2 is associated with colon cancer progression and a poor prognosis. J. Oncol. 2023, 4931650. doi:10.1155/2023/4931650
Perrone, G., Vincenzi, B., Zagami, M., Santini, D., Panteri, R., Flammia, G., et al. (2007). Reelin expression in human prostate cancer: a marker of tumor aggressiveness based on correlation with grade. Mod. pathology official J. U. S. Can. Acad. Pathology, Inc 20, 344–351. doi:10.1038/modpathol.3800743
Qin, X., Lin, L., Cao, L., Zhang, X., Song, X., Hao, J., et al. (2017). Extracellular matrix protein Reelin promotes myeloma progression by facilitating tumor cell proliferation and glycolysis. Sci. Rep. 7, 45305. doi:10.1038/srep45305
Ramaker, R., Lasseigne, B., Hardigan, A., Palacio, L., Gunther, D., Myers, R., et al. (2017). RNA sequencing-based cell proliferation analysis across 19 cancers identifies a subset of proliferation-informative cancers with a common survival signature. Oncotarget 8, 38668–38681. doi:10.18632/oncotarget.16961
Reza, M., Charlotte, D. M., Marina, L., Ana, N., Mehul, K., Frank, V., et al. (2023). Single-cell spatial analysis identifies regulators of brain tumor-initiating cells. Cancer Res. 83, 1725–1741. doi:10.1158/0008-5472.CAN-22-3004
Sajjad, K., Zeenat, M., Shakeel A, A., Mahmood, R., Zafar, I., Sayed S, S., et al. (2014). Transcriptomics study of neurodegenerative disease: emphasis on synaptic dysfunction mechanism in Alzheimer's disease. CNS Neurol. Disord. Drug Targets 13, 1202–1212. doi:10.2174/1871527313666140917113446
Salem, I., Blais, M., Zuluaga-Sánchez, V., Rouleau, L., Becker, E., and Dupré, N. (2025). ARSACS: clinical features, pathophysiology and iPS-derived models. Cerebellum Lond. Engl. 24, 24. doi:10.1007/s12311-024-01777-9
Schaff, L., and Mellinghoff, I. (2023). Glioblastoma and other primary brain malignancies in adults: a review. JAMA 329, 574–587. doi:10.1001/jama.2023.0023
Schmuck, E., Board, P., Whitbread, A., Tetlow, N., Cavanaugh, J., Blackburn, A., et al. (2005). Characterization of the monomethylarsonate reductase and dehydroascorbate reductase activities of Omega class glutathione transferase variants: implications for arsenic metabolism and the age-at-onset of Alzheimer's and Parkinson's diseases. Pharmacogenetics genomics 15, 493–501. doi:10.1097/01.fpc.0000165725.81559.e3
Seigel, G., Hackam, A., Ganguly, A., Mandell, L., and Gonzalez-Fernandez, F. (2007). Human embryonic and neuronal stem cell markers in retinoblastoma. Mol. Vis. 13, 823–832.
Serrano-Morales, J., Vázquez-Carretero, M., Peral, M., Ilundáin, A., and García-Miranda, P. (2017). Reelin-Dab1 signaling system in human colorectal cancer. Mol. Carcinog. 56, 712–721. doi:10.1002/mc.22527
Singh, A., Kukreti, R., Saso, L., and Kukreti, S. (2019). Oxidative stress: a key modulator in neurodegenerative diseases. Mol. Basel, Switz. 24, 1583. doi:10.3390/molecules24081583
Soo, Y. K., Miaolu, T., Tong, L., Stephen Y, C., and Wei, L. (2024). Ferroptosis in glioma therapy: advancements in sensitizing strategies and the complex tumor-promoting roles. Brain Res. 1840, 149045. doi:10.1016/j.brainres.2024.149045
Soragni, A., Janzen, D., Johnson, L., Lindgren, A., Thai-Quynh Nguyen, A., Tiourin, E., et al. (2016). A designed inhibitor of p53 aggregation rescues p53 tumor suppression in ovarian carcinomas. Cancer cell 29, 90–103. doi:10.1016/j.ccell.2015.12.002
S Sakura, M., Hyang-Sook, H., and William, R. G. (2011). Fyn kinase regulates the association between amyloid precursor protein and Dab1 by promoting their localization to detergent-resistant membranes. J. Neurochem. 118, 879–890. doi:10.1111/j.1471-4159.2011.07296.x
Sumiya, R., Terayama, M., Hagiwara, T., Nakata, K., Sekihara, K., Nagasaka, S., et al. (2022). Loss of GSTO2 contributes to cell growth and mitochondria function via the p38 signaling in lung squamous cell carcinoma. Cancer Sci. 113, 195–204. doi:10.1111/cas.15189
Talebian, S., Gharesouran, J., Ghafouri-Fard, S., Esfahani, B., Arsang-Jang, S., Omrani, M., et al. (2019). Assessment of expression of RELN signaling pathway in multiple sclerosis patients. Immunobiology 224, 402–407. doi:10.1016/j.imbio.2019.02.007
Talvard-Balland, N., Braun, L., Dixon, K., Zwick, M., Engel, H., Hartmann, A., et al. (2024). Oncogene-induced TIM-3 ligand expression dictates susceptibility to anti-TIM-3 therapy in mice. J. Clin. investigation 134, e177460. doi:10.1172/JCI177460
Tatjana, D., Goran, S., Vesna, C., Zoran, B., Marija, P.-E., Marija, M., et al. (2022). GSTO1, GSTO2 and ACE2 polymorphisms modify susceptibility to developing COVID-19. J. Pers. Med. 12, 458. doi:10.3390/jpm12030458
Teresa, F.-B., Héctor, C.-R., Elisa, R.-I., Esther, G., José María, Z., Beatriz, d.P.-T., et al. (2024). Pharmacological inhibition of receptor protein tyrosine phosphatase β/ζ decreases Aβ plaques and neuroinflammation in the hippocampus of APP/PS1 mice. Front. Pharmacol. 15. doi:10.3389/fphar.2024.1506049
Thakkar, J., Dolecek, T., Horbinski, C., Ostrom, Q., Lightner, D., Barnholtz-Sloan, J., et al. (2014). Epidemiologic and molecular prognostic review of glioblastoma. Cancer Epidemiol. biomarkers and Prev. a Publ. Am. Assoc. Cancer Res. cosponsored by Am. Soc. Prev. Oncol. 23, 1985–1996. doi:10.1158/1055-9965.EPI-14-0275
Tiwari, S., Atluri, V., Kaushik, A., Yndart, A., and Nair, M. (2019). Alzheimer's disease: pathogenesis, diagnostics, and therapeutics. Int. J. nanomedicine 14, 5541–5554. doi:10.2147/IJN.S200490
Valeria, M., Roberta, M., Gloria, R., Antonella, C., Vincenzo, M., Tuba Rana, C., et al. (2023). Role of a novel heparanase inhibitor on the balance between apoptosis and autophagy in U87 human glioblastoma cells. Cells 12, 1891. doi:10.3390/cells12141891
Voet, S., Srinivasan, S., Lamkanfi, M., and van Loo, G. (2019). Inflammasomes in neuroinflammatory and neurodegenerative diseases. EMBO Mol. Med. 11, e10248. doi:10.15252/emmm.201810248
Vy M, T., Anna, W., Andrew, M., Katharine, C., Olle R, L., Jane R, E., et al. (2017). Heparan sulfate glycosaminoglycans in glioblastoma promote tumor invasion. Mol. Cancer Res. 15, 1623–1633. doi:10.1158/1541-7786.MCR-17-0352
Wang, C., Wen, L., Wang, K., Wu, R., Li, M., Zhang, Y., et al. (2023). Visualization of ferroptosis in brain diseases and ferroptosis-inducing nanomedicine for glioma. Am. J. Nucl. Med. Mol. imaging 13, 179–194.
Wareham, L., Liddelow, S., Temple, S., Benowitz, L., Di Polo, A., Wellington, C., et al. (2022). Solving neurodegeneration: common mechanisms and strategies for new treatments. Mol. Neurodegener. 17, 23. doi:10.1186/s13024-022-00524-0
Xifeng, W., Chunguang, Z., Guowu, Z., Kai, Z., Zhiyuan, L., Yunxia, S., et al. (2022). Molecular characterization of a novel GSTO2 of Fasciola hepatica and its roles in modulating murine macrophages. Parasite 29, 16. doi:10.1051/parasite/2022016
Xu, J., Du, W., Zhao, Y., Lim, K., Lu, L., Zhang, C., et al. (2022). Mitochondria targeting drugs for neurodegenerative diseases-Design, mechanism and application. Acta Pharm. Sin. B 12, 2778–2789. doi:10.1016/j.apsb.2022.03.001
Yan, S., Yue, J., Sheng, C., Yang, X., Qi, W., and Xianwen, C. (2024). The molecular mechanism of ferroptosis and its relationship with Parkinson's disease. Brain Res. Bull. 213, 110991. doi:10.1016/j.brainresbull.2024.110991
Yehui, D., Wenfeng, S., Jian, C., Hao, C., Zefeng, X., Long, Z., et al. (2019). The potassium channel KCa3.1 promotes cell proliferation by activating SKP2 and metastasis through the EMT pathway in hepatocellular carcinoma. Int. J. Cancer 145, 503–516. doi:10.1002/ijc.32121
Yi Sak, K., Soo-Ho, C., Keun-Young, K., Juliana M, N.-P., Guy A, P., Seunghwan, C., et al. (2024). AIBP controls TLR4 inflammarafts and mitochondrial dysfunction in a mouse model of Alzheimer's disease. J. Neuroinflammation 21, 245. doi:10.1186/s12974-024-03214-4
Yunden, B., and Ven, N. (2024). Aberrant Wnt/β-catenin signaling in the mesenchymal stem cells of LZTFL1-depleted mice leads to increased adipogenesis, with implications for obesity. J. Biol. Chem. 301. doi:10.1016/j.jbc.2024.108057
Zeng, W., Takashima, K., Tang, Q., Zou, X., Ojiro, R., Ozawa, S., et al. (2023). Natural antioxidant formula ameliorates lipopolysaccharide-induced impairment of hippocampal neurogenesis and contextual fear memory through suppression of neuroinflammation in rats. J. Chem. Neuroanat. 131, 102285. doi:10.1016/j.jchemneu.2023.102285
Zhang, Y., Yan, H., Lu, W., Li, Y., Guo, X., and Xu, B. (2013). A novel Omega-class glutathione S-transferase gene in Apis cerana cerana: molecular characterisation of GSTO2 and its protective effects in oxidative stress. Cell stress and chaperones 18, 503–516. doi:10.1007/s12192-013-0406-2
Keywords: neurodegenerative, glioblastoma, biomarker, algorithm, therapeutic molecular target
Citation: Bu F, Zhong J and Guan R (2025) Biomarkers in glioblastoma and degenerative CNS diseases: defining new advances in clinical usefulness and therapeutic molecular target. Front. Mol. Biosci. 12:1506961. doi: 10.3389/fmolb.2025.1506961
Received: 06 October 2024; Accepted: 28 February 2025;
Published: 18 March 2025.
Edited by:
Maksym V. Kopanitsa, The Francis Crick Institute, United KingdomReviewed by:
Gloria Riitano, Sapienza University of Rome, ItalyCopyright © 2025 Bu, Zhong and Guan. This is an open-access article distributed under the terms of the Creative Commons Attribution License (CC BY). The use, distribution or reproduction in other forums is permitted, provided the original author(s) and the copyright owner(s) are credited and that the original publication in this journal is cited, in accordance with accepted academic practice. No use, distribution or reproduction is permitted which does not comply with these terms.
*Correspondence: Ruiqian Guan, MTk2MjI0OTE3QHFxLmNvbQ==
Disclaimer: All claims expressed in this article are solely those of the authors and do not necessarily represent those of their affiliated organizations, or those of the publisher, the editors and the reviewers. Any product that may be evaluated in this article or claim that may be made by its manufacturer is not guaranteed or endorsed by the publisher.
Research integrity at Frontiers
Learn more about the work of our research integrity team to safeguard the quality of each article we publish.