- State Key Laboratory of Organ Failure Research, Guangdong Key Laboratory of Viral Hepatitis Research, Guangdong Institute of Liver Diseases, Department of Infectious Diseases and Hepatology Unit, Nanfang Hospital, Southern Medical University, Guangzhou, China
C1ORF112 is an evolutionarily conserved gene across vertebrates. Over the last decade, studies have suggested that C1ORF112 may play a role in tumorigenesis. Using The Cancer Genome Atlas datasets, we explored the role of C1ORF112 across various tumor types in this study. In most tumor types, C1ORF112 expression was increased in tumor tissues compared to corresponding non-tumor tissues. In patients with certain tumor types, higher C1ORF112 expression was correlated with shorter overall survival, disease-free survival, and progression-free survival. Further analyses of C1ORF112 genetic alteration data showed that C1ORF112 amplification and mutations may have an impact on liver hepatocellular carcinoma and uterine corpus endometrial carcinoma prognosis. In cancers including lower grade glioma and adrenocortical carcinoma, C1ORF112 expression was linked to cancer-associated fibroblast infiltration. Gene Ontology analysis showed that C1ORF112 was co-expressed with genes involved in biological processes such as cell cycle and mitotic regulation. The protein interaction network demonstrated that C1ORF112 physically interacted with RAD51, DMC1, and FIGNL1, which have well characterized functions in DNA repair and cell cycle regulation. This pan-cancer study revealed the prognostic value and oncogenic role of C1ORF112 across multiple tumor types.
Introduction
Cancer is the most common cause of death in the world (Sung et al., 2021). To widen the potential therapy options for malignancies, a better knowledge of carcinogenesis and tumor progression through the identification of oncogenes is crucial. Large-scale and multi-omics cancer datasets, such as The Cancer Genome Atlas (TCGA) (ICGC/TCGA Pan-Cancer Analysis of Whole Genomes Consortium, 2020; Ye et al., 2021; Lv et al., 2021; Cheng et al., 2021; Cui et al., 2020), have made pan-cancer analysis possible in the last decade.
Chromosome 1 Open Reading Frame 112 (C1ORF112), also known as FLJ10706 (Howe et al., 2021), is evolutionarily conserved, especially in primates (Edogbanya et al., 2021). Mice lacking BC055324 (the mouse ortholog for C1ORF112) are embryonic lethal (https://www.mousephenotype.org/data/genes/MGI:3590554), indicating that BC055324 is required for embryonic development. According to van Dam et al., genes enriched in the BRCA–Fanconi anemia-related DNA damage response pathway, such as BRCA1 and FANCI, were shown to be co-expressed with C1ORF112 (van Dam et al., 2012). And the dysregulation of this pathway is linked to a higher risk of cancer (Nalepa and Clapp, 2018). Furthermore, C1ORF112 knockdown inhibited cell proliferation of HeLa cells, implying C1ORF112 may have a role in cancer (van Dam et al., 2012). Moreover, according to previous studies, C1ORF112 was overexpressed in tumor tissues of stomach cancer and head and neck squamous cell carcinoma (Chen et al., 2020; Huang et al., 2020; Edogbanya et al., 2021). Besides, C1ORF112 expression was found to be higher in tumor samples with mutant TP53, a well-known tumor suppressor, in a study of bladder cancer progression (Sanchez-Carbayo et al., 2007). To the best of our knowledge, no comprehensive analysis of C1ORF112’s function and clinical importance at the pan-cancer level has been done.
In this study, we systematically analyzed the expression status, prognostic value, genetic alteration, and molecular function of C1ORF112 as well as the association with cancer-associated fibroblast infiltration in multiple tumor types.
Materials and Methods
Gene Expression Analysis of C1ORF112
We constructed a C1ORF112 mRNA expression plot using the Human Protein Atlas (HPA) database (version: 20.1) (https://www.proteinatlas.org/).
The “Gene DE” module of Tumor Immune Estimation Resource version 2 (TIMER2) (http://timer.cistrome.org/) was used to investigate C1ORF112 expression differences in tumor and non-tumor tissues in various tumor types. In this module, C1ORF112 expression was also evaluated between distinct breast cancer molecular subgroups, between HPV-positive and HPV-negative head and neck squamous cell carcinoma (HNSC), and between primary and metastatic skin cutaneous melanoma (SKCM).
We searched C1ORF112 in the Oncomine database (Research Edition) (https://www.oncomine.org) to generate a pooled analysis of C1ORF112 expression.
Survival Prognosis Analysis
Overall survival (OS) and disease-free survival (DFS) Kaplan–Meier (K-M) plots, as well as a survival significance map of C1ORF112 in all TCGA tumor types, were generated using the Gene Expression Profiling Interactive Analysis version 2 (GEPIA2) (http://gepia2.cancer-pku.cn/) “Survival Analysis” module. In addition, UCSC Xena Browser (https://xenabrowser.net/) was used to perform progression-free survival (PFS) analysis of C1ORF112 using TCGA Pan-Cancer datasets (version: 2018–09–13). The expression threshold was set at 50% for high C1ORF112 expression and low C1ORF112 expression.
Genetic Alteration Analysis
C1ORF112 genetic alterations were analyzed using cBioPortal (version: 3.6.20) (https://www.cbioportal.org/). Based on datasets of TCGA Pan-Cancer Atlas Studies, we calculated the frequency of C1ORF112 gene mutation and copy number alteration in the “Cancer Types Summary” module. A mutation site plot of C1ORF112 was created using the “Mutations” module.
To analyze the correlation between C1ORF112 amplification status and liver hepatocellular carcinoma (LIHC) prognosis, the molecular profile was selected as copy number alterations based on “liver hepatocellular carcinoma (TCGA Pan-Cancer)” and the survival plot was generated by dividing cases based on the presence of copy number alterations (altered and unaltered groups).
To analyze the correlation between C1ORF112 mutation status and uterine corpus endometrial carcinoma (UCEC) prognosis, the molecular profile was selected as mutations based on “uterine corpus endometrial carcinoma (TCGA Pan-Cancer)” and the survival plot was generated by dividing cases into altered and unaltered groups.
Immune Cells Infiltration Analysis
Using the “Immune” module of Tumor Immune Estimation Resource version 2 (TIMER2) (http://timer.cistrome.org/), Extended Polydimensional Immunome Characterization (EPIC) and the Tumor Immune Dysfunction and Exclusion (TIDE) algorithms were used to investigate the correlation between C1ORF112 expression and cancer-associated fibroblast infiltration.
C1ORF112-Related Gene Enrichment Analysis
The STRING tool (version: 11.0b) (https://string-db.org/) was used to create a Homo Sapiens C1ORF112 co-expression network using the following main parameters: 1) Active interaction sources: co-expression; 2) meaning of network edges: evidence; 3) maximum number of interactors: 50; and 4) minimum required interaction score: low confidence (0.150).
The GEPIA2 “Similar Gene Detection” module was used to extract 100 C1ORF112-correlated genes from the TCGA datasets that had the most similar expression pattern to C1ORF112. Gene Ontology pathway enrichment analysis was performed using the gene symbols of these 100 genes as input gene symbols in the “clusterProfiler” R package (version: 3.13). In addition, pairwise gene correlation analysis was performed using the GEPIA2 “Correlation Analysis” module.
C1ORF112-Protein Interaction Analysis
The “Network” module of BioGRID (version: 4.3) (https://thebiogrid.org/) was used to create a C1ORF112-protein interaction network, with the layout set to “Concentric Circles.”
Conservation Analysis of C1ORF112
The UCSC genome browser (version: 2021 update) (http://www.genome.ucsc.edu/cgi-bin/hgTracks) was used to visualize gene conversation of C1ORF112 among vertebrates.
Results
Gene Expression Analysis of C1ORF112
Based on datasets of the HPA, GTEx, and FANTOM5 (function annotation of the mammalian genome), we found that C1ORF112 was highly expressed in lymphoid tissue, such as thymus and bone, and enriched in testis and the parathyroid gland (Figure 1A; Supplementary Figure S1). Moreover, based on single-cell RNA-seq, high expression of C1ORF112 was also observed in spermatocytes and spermatogonia (Figure 1A; Supplementary Figure S1). C1ORF112 expression has a low tissue specificity, according to these findings. We also found that C1ORF112 is relatively conserved among vertebrates (Figure 1B).
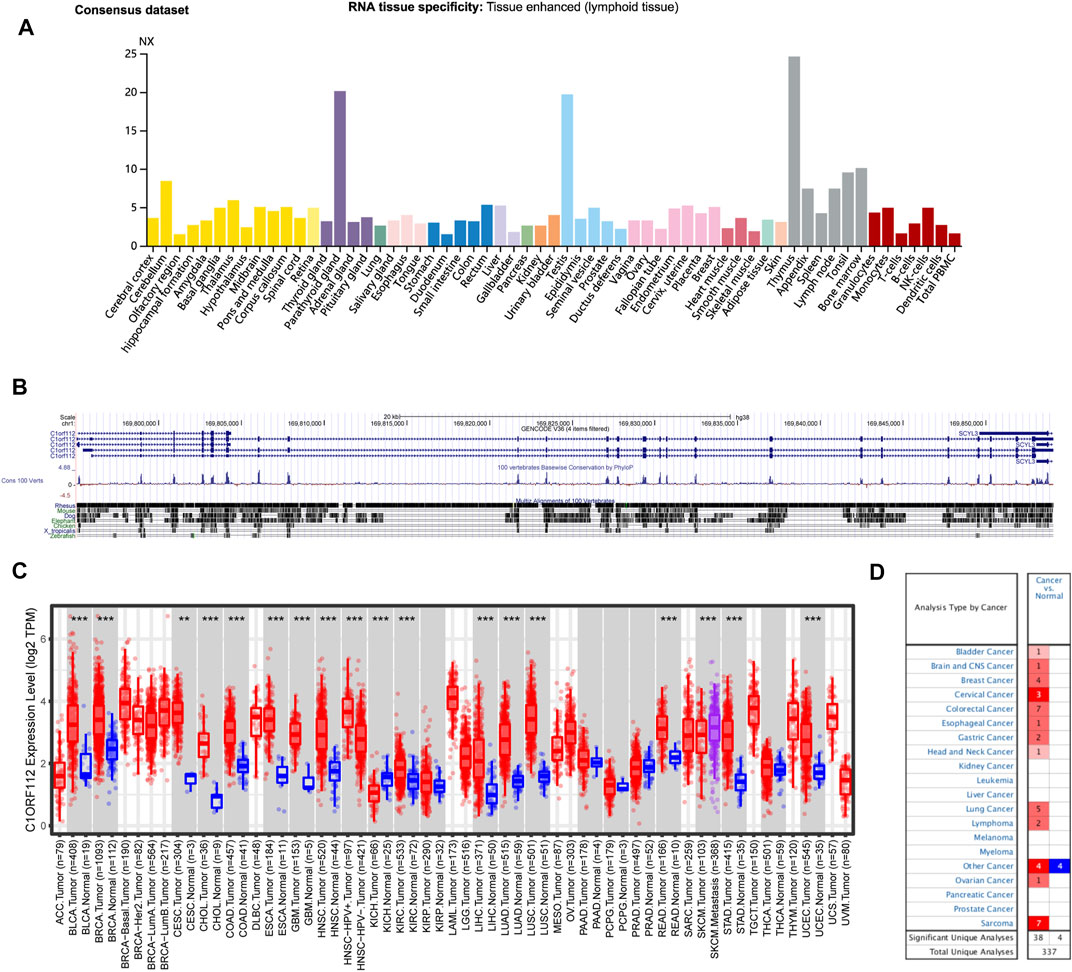
FIGURE 1. C1ORF112 expression status in different tumors and normal tissues and C1ORF112 gene conservation among vertebrates. (A) Consensus C1ORF112 tissue expression based on datasets of HPA (Human Protein Atlas), GTEx, and FANTOM5 (function annotation of the mammalian genome). (B) C1ORF112 gene conservation analysis among vertebrates was visualized using the UCSC genome browser. (C) The expression status of C1ORF112 in different tumor types was visualized by TIMER2. *p < 0.05; **p < 0.01; ***p < 0.001. (D) Oncomine pooling analysis of C1ORF112 expression in various tumor types.
The expression pattern of C1ORF112 in tumor tissues was then investigated. Similar to its distribution in normal tissue, C1ORF112 mRNA distribution also showed low tumor specificity. C1ORF112 mRNA expression was increased in various tumor tissues when compared to corresponding normal tissue (Figure 1C). Tumor tissues of bladder urothelial carcinoma (BLCA), breast invasive carcinoma (BRCA), cholangiocarcinoma (CHOL), colon adenocarcinoma (COAD), esophageal carcinoma (ESCA), glioblastoma multiforme (GBM), HNSC, kidney renal papillary cell carcinoma (KIRP), LIHC, lung adenocarcinoma (LUAD), lung squamous cell carcinoma (LUSC), rectum adenocarcinoma (READ), stomach adenocarcinoma (STAD), UCEC (all p < 0.001), cervical squamous cell carcinoma, and endocervical adenocarcinoma (CESC) (p < 0.01) had significantly higher C1ORF112 expression when compared to corresponding normal tissue (Figure 1C). Significantly higher C1ORF112 expression was observed in HPV-positive head and neck squamous cell tumor tissues compared with HPV-negative tissues (p < 0.001) (Figure 1C). Furthermore, when compared to primary SKCM tumor tissues, C1ORF112 expression was also significantly elevated in metastatic SKCM tissues (p < 0.001) (Figure 1C). Meanwhile, significantly decreased C1ORF112 expression was observed in kidney chromophobe (KICH) tumor tissues (p < 0.001) (Figure 1C).
We next used the Oncomine database to validate the differential C1ORF112 expression between tumor and normal tissues. As shown in Figure 1D, significantly elevated C1ORF112 expression was observed in most cancer types, including bladder cancer, brain and central nervous system cancer, breast cancer, cervical cancer, colorectal cancer, esophageal cancer, gastric cancer, head and neck cancer, lung cancer, lymphoma, ovarian cancer, and sarcoma.
Together these findings suggested that C1ORF112 may promote carcinogenesis in a variety of tumor types, and its clinical significance requires further investigation.
The Association Between C1ORF112 Expression and Prognosis of Patients With Cancer
To explore the potential prognostic value of C1ORF112 based on TCGA datasets, we investigated the correlation between C1ORF112 expression and prognosis of patients with different tumors by using GEPIA2. Higher C1ORF112 expression was associated with shorter OS in cases of adrenocortical carcinoma (ACC) (p = 3.2 × 10–3), KICH (p = 1.4 × 10–2), KIRP (p = 2.5 × 10–3), LGG (p = 6.2 × 10–8), LIHC (p = 3.2 × 10–2), LUAD (p = 2.1 × 10–2), mesothelioma (MESO) (p = 1.5 × 10–3), pancreatic adenocarcinoma (PAAD) (p = 4.0 × 10–2), and sarcoma (SARC) (p = 1.4 × 10–2) (Figure 2). Furthermore, DFS analysis showed that high C1ORF112 expression was a marker for poor outcome for patients with ACC (p = 4.3 × 10–3), KIRP (p = 6.6 × 10–3), LGG (p = 1.4 × 10–6), MESO (p = 3.6 × 10–2), PRAD (p = 3.2 × 10–2), and SARC (p = 1.4 × 10–2) (Figures 3A–G). Using UCSC Xena Browser, a significant association was also noted between C1ORF112 expression and PFS in several human cancers. In patients of ACC (p = 9.9 × 10–4), CHOL (p = 4.1 × 10–2), KICH (p = 2.6 × 10–2), KIRP (p = 3.0 × 10–5), LGG (p = 7.9 × 10–8), LUAD (p = 7.7 × 10–3), MESO (p = 9.4 × 10–4), PRAD (p = 1.5 × 10–2), SARC (p = 4.3 × 10–2), SKCM (p = 1.9 × 10–2), and UCEC (p = 5.5 × 10–4), higher C1ORF112 expression is associated with a worse prognosis (Supplementary Figure S2). These results indicated that increased C1ORF112 expression was associated with poor prognosis in a variety of tumor types.
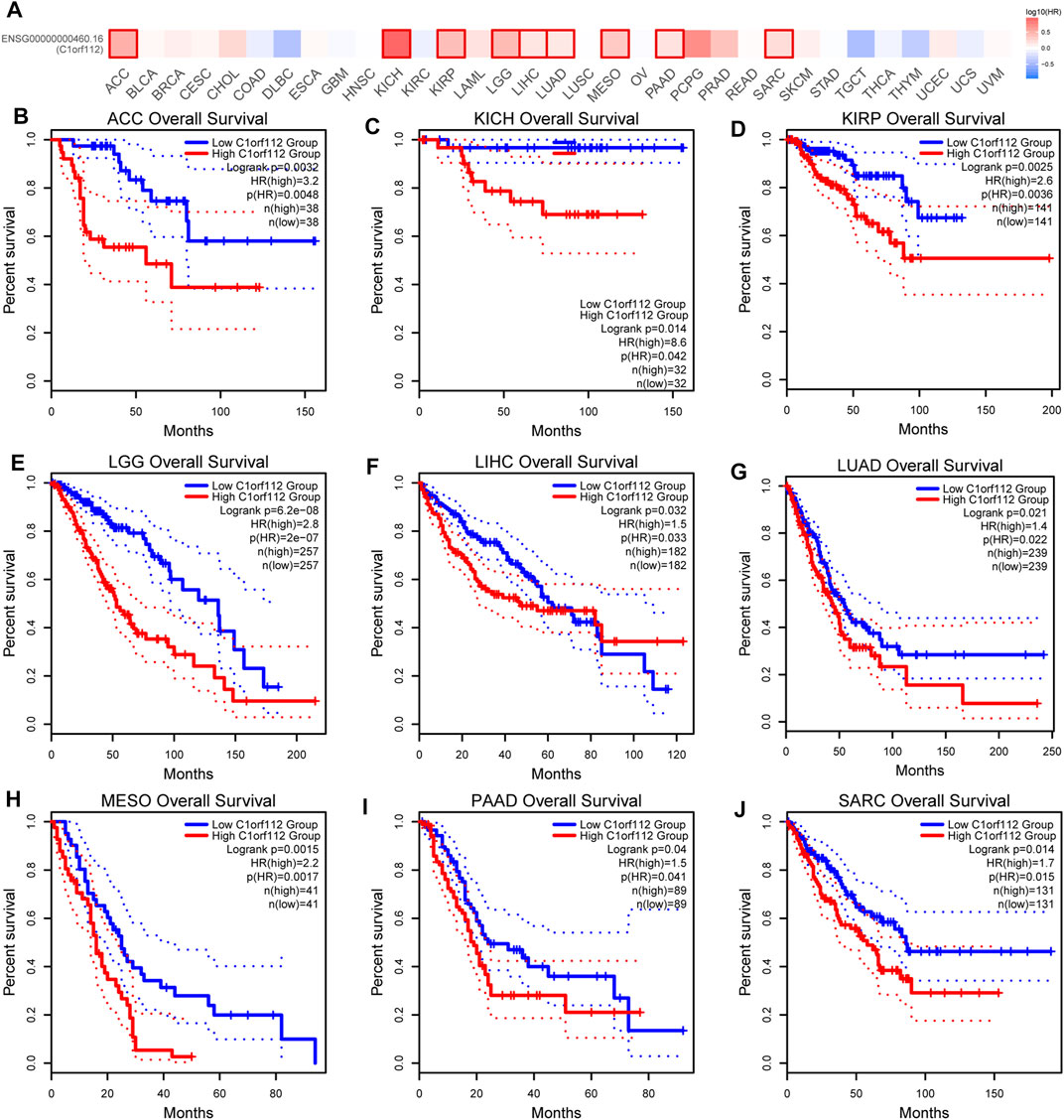
FIGURE 2. Correlation between C1ORF112 expression and overall survival in patients with different TCGA tumor types. GEPIA2 was used to build a survival map (A) and conduct overall survival analyses (B–J). The survival map and Kaplan–Meier plots with significant results are displayed. The 95% confidence intervals of overall survival are indicated by red and blue dotted lines for high and low C1ORF112 groups, respectively.
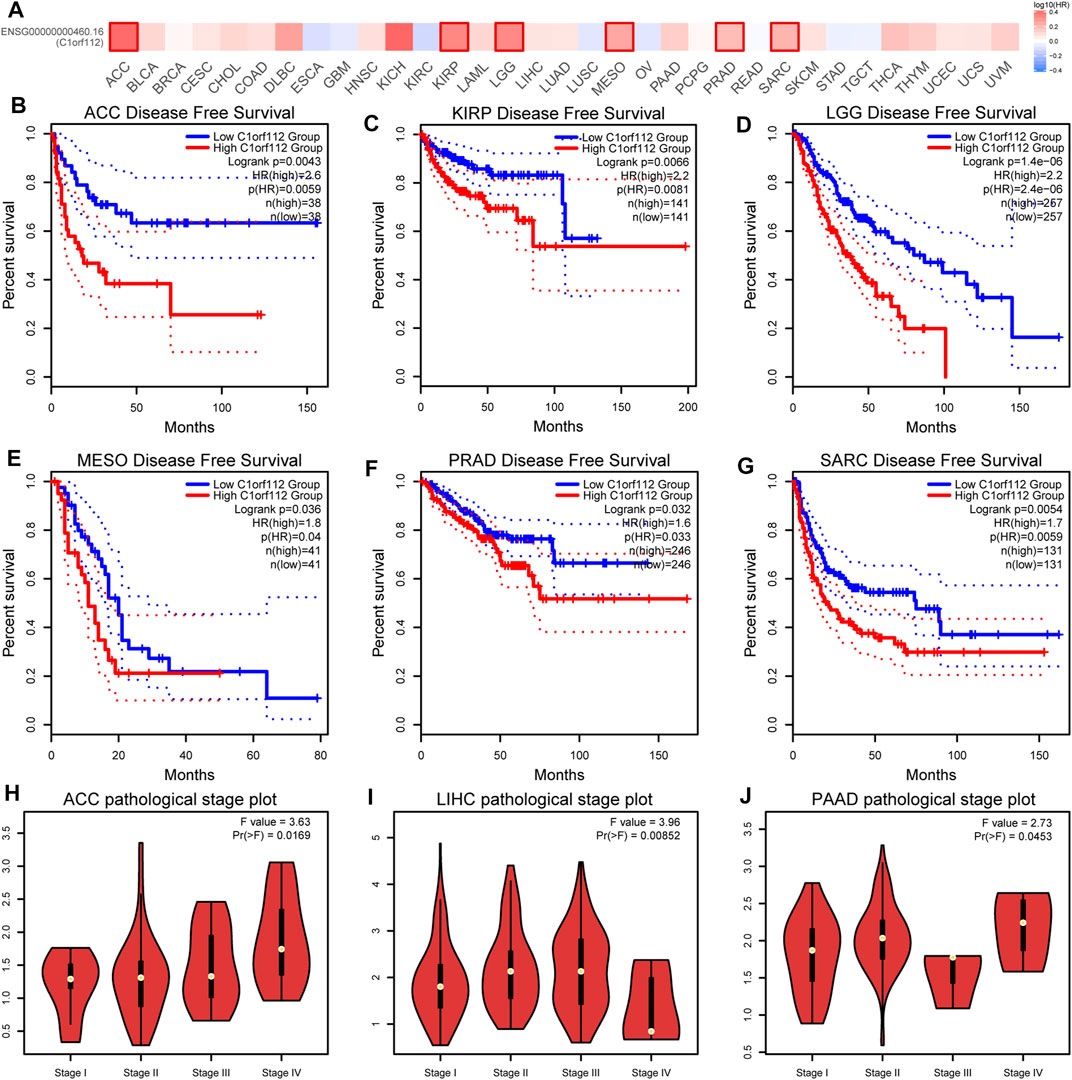
FIGURE 3. Correlation between C1ORF112 expression and disease-free survival in patients with different TCGA tumor types. GEPIA2 was used to build a survival map (A) and conduct disease-free survival (B–G) analyses. The survival map and Kaplan–Meier plots with significant results are displayed. The 95% confidence intervals of disease-free survival are indicated by red and blue dotted lines for high and low C1ORF112 groups, respectively. (H–J) Correlation between C1ORF112 expression and pathological stages of ACC, LIHC, and PAAD from TCGA datasets. Log2 (TPM + 1) was applied for log-scale.
Moreover, we also investigated the correlation between C1ORF112 expression and pathological stages of tumors by GEPIA2. The high expression of C1ORF112 was significantly correlated with the advanced stage of ACC, LIHC, and PAAD (Figures 3H–J).
The Genetic Alteration Landscape of C1ORF112 in Different Tumors
The genetic alteration of C1ORF112 in various tumor types in TCGA datasets was then investigated using cBioPortal. We found that CHOL tumor samples had the highest C1ORF112 genetic alteration frequency (>10%). All of the genetic alterations occurring in CHOL tumor samples were copy number amplification (Figure 4A; Supplementary Table S1), which was the major type of genetic alteration in all TCGA tumor samples. In addition to CHOL cancer, more than 8% of LIHC, BRCA, and UCEC samples showed genetic alteration of C1ORF112 (Figure 4A; Supplementary Table S1). As shown in Figure 4B, a total of 137 C1ORF112 mutations, including 111 missense mutations, 21 truncating mutations, 4 fusion mutations, and 1 in-frame mutation, were detected in TCGA tumor samples (Supplementary Table S2). The residues 250–253 of the protein encoded by C1ORF112 had nine mutations, making it the most frequently mutated region in the C1ORF112 protein (Figure 4B).
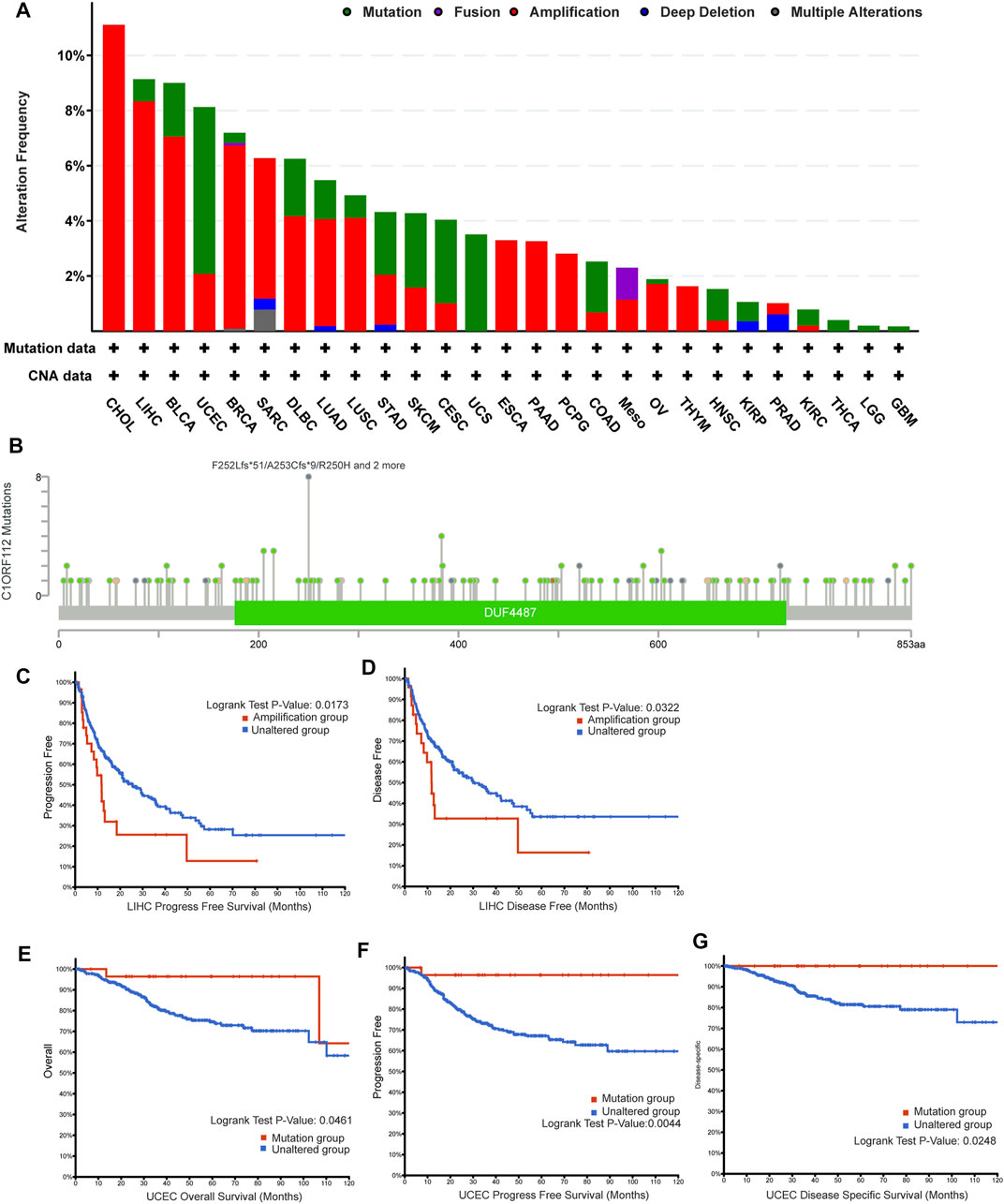
FIGURE 4. C1ORF112 genetic alteration in various tumor types of TCGA. The alteration frequency with C1ORF112 genetic alteration type (A) and C1ORF112 mutation site (B) were generated by cBioPortal. The correlations between C1ORF112 amplification status and progression-free survival and disease-free survival of LIHC (C,D) were analyzed by cBioPortal. The correlations between mutation status and overall survival, progression-free survival, and disease-specific survival of UCEC (E–G) were analyzed by cBioPortal.
Following that, we explored the link between C1ORF112 genetic alterations and clinical outcomes of cancer patients. C1ORF112 amplification was associated with poor prognosis in LIHC patients in terms of PFS (p = 1.7 × 10–2) and DFS (p = 3.2 × 10–2) (Figures 4C,D; Supplementary Table S3). In addition, UCEC patients with C1ORF112 mutations (6.05%; 32 cases) showed a better prognosis in terms of OS (p = 4.6 × 10–2), PFS (p = 4.4 × 10–3), and DFS (p = 2.5 × 10–2) (Figures 4E–G; Supplementary Table S4).
Cancer-Associated Fibroblast Infiltration Analysis
Previous studies have found that cancer-associated fibroblasts in the stroma are involved in the regulation of different tumor-infiltrating immune cells (Chen and Song, 2019). We therefore employed the EPIC and TIDE algorithms to investigate the correlation between cancer-associated fibroblast infiltration and C1ORF112 expression in different malignancies. C1ORF112 expression was positively correlated with cancer-associated fibroblast infiltration in ACC, CESC, KIRC, KIRP, LGG, MESO, and thyroid carcinoma (THCA) (Figure 5).
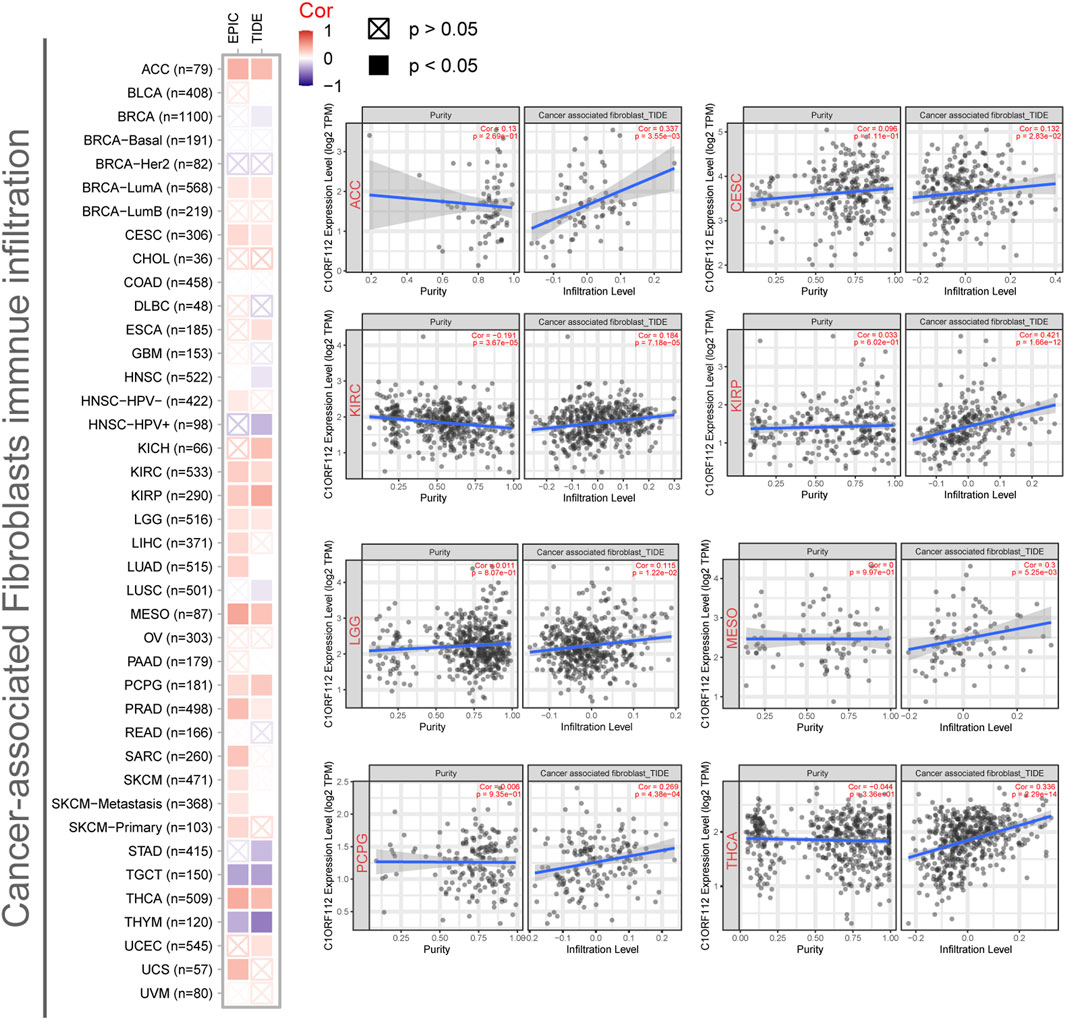
FIGURE 5. Correlation between C1ORF112 expression and cancer-associated fibroblast immune infiltration. EPIC and TIDE algorithms were used to calculate the correlation between C1ORF112 expression and cancer-associated fibroblast immune infiltration in all tumor types from TCGA.
C1ORF112-Related Gene Enrichment Analysis
To investigate the functional mechanism of C1ORF112 in carcinogenesis, we used GEPIA2 to extract the top 100 genes with expression patterns similar to C1ORF112 from all tumor types in the TCGA datasets (Supplementary Table S5). Gene Ontology enrichment analysis indicated that these genes were closely linked to cell cycle or mitosis regulation (Figure 6A). Following that, 50 genes co-expressed with C1ORF112 were obtained by the STRING tool to validate the result of Gene Ontology enrichment analysis. As shown in Figure 6B, the correlations of these 50 genes were mutually close; also, the genes were also enriched in cell cycle and mitotic regulation (Supplementary Table S6). These findings prompted us to wonder whether C1ORF112 plays a role in these biological processes by interacting with key proteins involved in cell cycle and mitotic regulation. According to the BioGRID4.3 database, C1ORF112 physically interacts with RAD51, DMC1, and FIGNL1 (Figure 6C), which have well-characterized functions in the cell cycle, mitotic regulation, and tumorigenesis (Supplementary Figure S3) (Yuan and Chen, 2013; Laurini et al., 2020). Moreover, C1ORF112 expression is strongly correlated with expression levels of RAD51 and FIGNL1 (Figures 6D,E). Based on these results, we speculate that C1ORF112 may play a tumor-promoting role in cancers by driving the cell cycle and facilitating cell division.
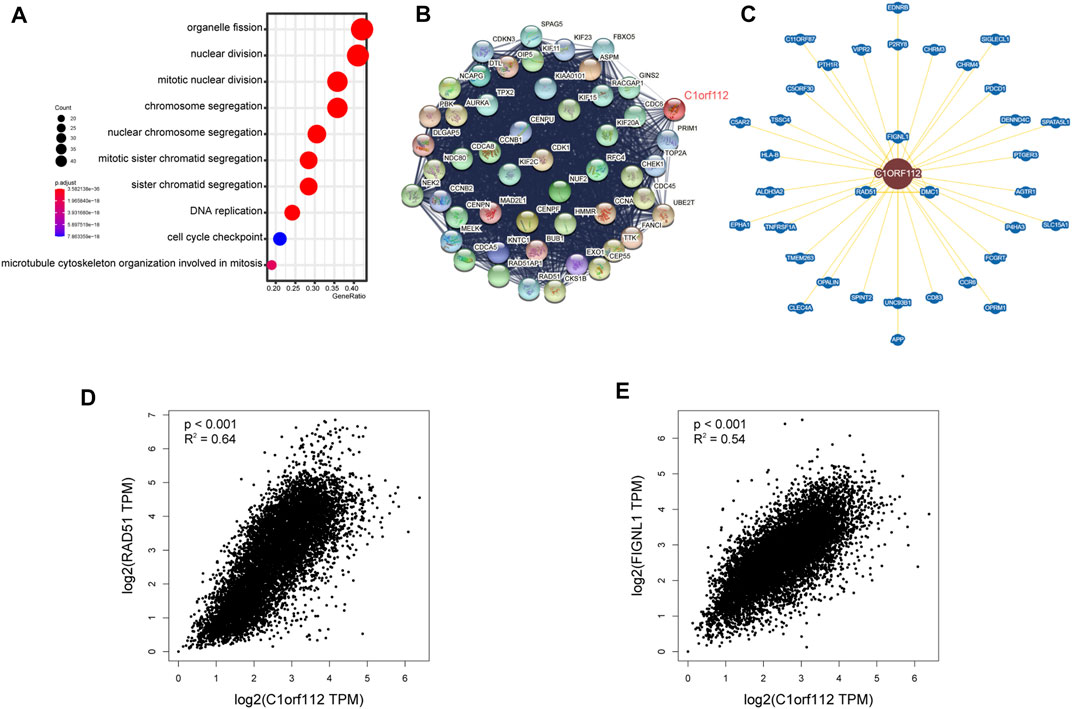
FIGURE 6. C1ORF112-related gene enrichment analysis. (A) Gene Ontology (GO) analysis of the top 100 genes co-expressed with C1ORF112 obtained by the GEPIA2. (B) Co-expression network of 50 genes co-expressed with C1ORF112 obtained by the STRING tool. (C)C1ORF112-protein interactions obtained by BioGRID. (D,E) Correlation analysis between C1ORF112 and RAD51 and FIGNL1 conducted by GEPIA2 across all tumor samples from TCGA.
Discussion
TCGA project has profiled 33 prevalent tumor types with multi-omics data and provided an unprecedented opportunity to discover molecular aberrations at the pan-cancer level (Malta et al., 2018; Way et al., 2018; Schaub et al., 2018; Weinstein et al., 2013). Thanks to the development of bioinformatics algorithms and databases (Cerami et al., 2012; Carithers and Moore, 2013; Tang et al., 2019), numerous studies have been undertaken in recent years to identify pan-cancer molecular biomarkers and their functional roles (Ye et al., 2021; Lv et al., 2021; Hong et al., 2020). In this study, we analyzed the prognostic value and oncogenic role of C1ORF112 in a variety of tumor types.
C1ORF112 is located at chromosome 1q24.2 and its gene synonym is FLJ10706. C1ORF112 encodes nine transcripts, five of which are protein-coding (Howe et al., 2021). The C1ORF112 protein with the longest amino acid sequence is translated from two distinct transcripts, ENST00000286031 and ENST00000359326 (Howe et al., 2021). According to the Ensembl database, C1ORF112 orthologues have been found in 194 species. The protein-coding sequence of C1ORF112 has a relatively high level of conservation among vertebrates, and a previous study revealed that C1ORF112 might have evolved from the ancestors of eukaryotes (Edogbanya et al., 2021). Mice lacking BC055324 (the mouse ortholog of C1ORF112) are embryonic fatal, and heterozygous mice have lower bone mineral density, circulating glucose level, and cardiac output (https://www.mousephenotype.org/data/genes/MGI:3590554). In the last decade, C1ORF112 has drawn attention for its potential role in tumorigenesis. Van Dam et al. found that C1ORF112 knockdown in HeLa cancer cells significantly lowered cell growth rate (van Dam et al., 2012). A recent study revealed that C1ORF112 was co-expressed with stem cell-related genes, and these genes had elevated expression in gastric cancer tissues (Huang et al., 2020). Another study identified C1ORF112 as one of the genes in a nine-gene risk model for predicting prognosis in patients with gastric cancer (Chen et al., 2020). However, the significance of C1ORF112 in various tumor types has not been comprehensively explored. Therefore, we systemically characterized C1ORF112 in 33 TCGA tumor types by analyzing features such as gene expression, genetic alteration, and immune infiltration.
In the present study, we found that C1ORF112 is widely expressed in a variety of tissues and C1ORF112 expression is upregulated in the majority of tumors. We further explored the relationship between C1ORF112 overexpression and clinical parameters or prognosis. Survival analysis revealed that C1ORF112 overexpression was associated with poor OS, DFS, and PFS. High C1ORF112 expression was associated with poor prognosis in different tumor types involving ACC, CHOL, KIHC, KIRP, LGG, LUAD, MESO, PAAD, and SARC. Furthermore, upregulated C1ORF112 expression is also significantly associated with the advanced cancer stage suggesting malignant progression. Growing evidence indicates that genomic mutations involved tumor progression and chemotherapy response (Yang et al., 2011; Cha et al., 2021). For example, Yang et al. reported that BRCA1 and BRCA2 mutations are significantly associated with patient survival, which may be a result of distinct response to platinum-based treatment (Yang et al., 2011). A large-scale study identified that mutations in four genes (ESR1, CDH1, RICTOR, and TP53) tended to occur in specific metastatic sites, which could be biomarkers or therapeutic targets of metastatic breast cancer patients (Cha et al., 2021). In this study, we revealed that mutations of C1ORF112 were most common in CHOL (>10%), followed by LIHC, BRCA, and UCEC. To analyze whether C1ORF112 genetic alterations have an impact on clinical outcomes of cancer patients, we discovered that C1ORF112 amplification could be a risk factor for patients with liver cancer, while C1ORF112 mutation may be protective in UCEC patients. Collectively, these findings indicate that C1ORF112 acts as an oncogene in the progression of a variety of cancers and is a promising predictor for practical application in cancer prognosis.
Immune cells extensively intertwine with cancer cells and exert an essential effect on cancer migration and metastasis in various tumor types (Angelova et al., 2015). Recent studies have also reported that tumor immune microenvironment was associated with the expression level of various genes (Ju et al., 2020a; Ju et al, 2020b). In this study, we found C1ORF112 expression was positively correlated with CAFs infiltration in several tumor types. CAFs are prominent components of stromal cells and have been reported to be associated with worse prognosis, chemotherapy resistance, and disease recurrence in various cancers (Calon et al., 2015; Ryner et al., 2015; Liu et al., 2016; Fiori et al., 2019). Taken together, our work elucidates the underlying effect of C1ORF112 in tumor immunity and its prognostic values for multiple cancers.
Using STRING and GEPIA2, we identified a number of genes that were co-expressed with C1ORF112 across different tumors and other tissues. Gene enrichment analysis revealed that these genes were strongly correlated with cell cycle or mitosis regulation, which was consistent with previous studies (van Dam et al., 2012; Edogbanya et al., 2021). Moreover, our results showed that C1ORF112 physically interacts with RAD51, FIGNL1, and DMC1. And the expressions of RAD51 and FIGNL1 were strongly correlated with C1ORF112 expression. RAD51, FIGNL1, and DMC1 are well-characterized genes that encode proteins involved in DNA repair and cell cycle regulation (Yuan and Chen, 2013; Laurini et al., 2020). These findings validated the results of our gene enrichment analysis and paved the way for further exploration of C1ORF112 molecular function.
This study had several limitations as well. First, the sample sizes for some uncommon tumor types were relatively small, which may cause batch effects or inaccurate results. Second, this study only provides preliminary findings linking C1ORF112 to cancer progression in various tumors, and more experimental work is needed to determine the precise molecular function of C1ORF112 in tumorigenesis.
In conclusion, C1ORF112 is widely overexpressed in diverse cancers and its expression and genetic alteration are statistically associated with clinical outcomes in patients with certain tumors. Furthermore, immune infiltration analysis and C1ORF112-related gene enrichment analysis offer potential mechanisms by which C1ORF112 may regulate tumor immunity, cell cycle, or DNA repair in cancers. Hence, further experimental and clinical studies are warranted to investigate C1ORF112’s practical application in cancer therapy and prognosis prediction.
Data Availability Statement
The datasets presented in this study can be found in online repositories. The names of the repository/repositories and accession number(s) can be found in the article/Supplementary Material.
Ethics Statement
Ethical review and approval were not required for the study on human participants in accordance with the local legislation and institutional requirements. Written informed consent for participation was not required for this study in accordance with the national legislation and the institutional requirements.
Author Contributions
JC and HM performed the data analysis and prepared the draft of this manuscript. HC and BZ revised the draft. JH and D-KJ designed the research and supervised all the work. All authors contributed to the article and approved the submitted version.
Funding
This study was supported by the Local Innovative and Research Teams Project of Guangdong Pearl River Talents Program (No. 2017BT01S131), the General Program from the Natural Science Foundation of Guangdong Province (No. 2019A1515011423), the General Programs from the National Natural Science Foundation of China (Nos. 81472618 and 81670535), the National Science and Technology Major Project (Nos. 2017ZX10202202 and 2018ZX10301202), the Key-Area Research and Development Program of Guangdong Province (No. 2019B020227004), the Innovative Research Team Project of Guangxi Province (No. 2017GXNSFGA198002), the Dean Fund of Nanfang Hospital, Southern Medical University (No. 2018Z005), the Grant for Recruited Talents to Start Scientific Research from Nanfang Hospital, and the Outstanding Youth Development Scheme of Nanfang Hospital, Southern Medical University (No. 2017J001).
Conflict of Interest
The authors declare that the research was conducted in the absence of any commercial or financial relationships that could be construed as a potential conflict of interest.
Publisher’s Note
All claims expressed in this article are solely those of the authors and do not necessarily represent those of their affiliated organizations, or those of the publisher, the editors, and the reviewers. Any product that may be evaluated in this article, or claim that may be made by its manufacturer, is not guaranteed or endorsed by the publisher.
Supplementary Material
The Supplementary Material for this article can be found online at: https://www.frontiersin.org/articles/10.3389/fmolb.2021.693651/full#supplementary-material
References
Angelova, M., Charoentong, P., Hackl, H., Fischer, M., Snajder, R., Krogsdam, A., et al. (2015). Characterization of the Immunophenotypes and Antigenomes of Colorectal Cancers Reveals Distinct Tumor Escape Mechanisms and Novel Targets for Immunotherapy. Genome Biol. 16, 64. doi:10.1186/s13059-015-0620-6
Calon, A., Lonardo, E., Berenguer-Llergo, A., Espinet, E., Hernando-Momblona, X., Iglesias, M., et al. (2015). Stromal Gene Expression Defines Poor-Prognosis Subtypes in Colorectal Cancer. Nat. Genet. 47 (4), 320–329. doi:10.1038/ng.3225
Carithers, L. J., and Moore, H. M. (2013). The Genotype-Tissue Expression (GTEx) Project. Nat. Genet. 45 (6), 580–585.doi:10.1038/ng.2653
Cerami, E., Gao, J., Dogrusoz, U., Gross, B. E., Sumer, S. O., Aksoy, B. A., et al. (2012). The cBio Cancer Genomics Portal: An Open Platform for Exploring Multidimensional Cancer Genomics Data: Figure 1. Cancer Discov. 2 (5), 401–404. doi:10.1158/2159-8290.cd-12-0095
Cha, S., Lee, E., and Won, H. (2021). Comprehensive Characterization of Distinct Genetic Alterations in Metastatic Breast Cancer across Various Metastatic Sites. NPJ breast cancer 7 (1), 93. doi:10.1038/s41523-021-00303-y
Chen, X., and Song, E. (2019). Turning Foes to Friends: Targeting Cancer-Associated Fibroblasts. Nat. Rev. Drug Discov. 18 (2), 99–115. doi:10.1038/s41573-018-0004-1
Chen, X., Zhang, D., Jiang, F., Shen, Y., Li, X., Hu, X., et al. (2020). Prognostic Prediction Using a Stemness Index-Related Signature in a Cohort of Gastric Cancer. Front. Mol. Biosci. 7, 570702. doi:10.3389/fmolb.2020.570702
Cheng, X., Wang, X., Nie, K., Cheng, L., Zhang, Z., Hu, Y., et al. (2021). Systematic Pan-Cancer Analysis Identifies TREM2 as an Immunological and Prognostic Biomarker. Front. Immunol. 12, 646523. doi:10.3389/fimmu.2021.646523
Cui, X., Zhang, X., Liu, M., Zhao, C., Zhang, N., Ren, Y., et al. (2020). A Pan-Cancer Analysis of the Oncogenic Role of Staphylococcal Nuclease Domain-Containing Protein 1 (SND1) in Human Tumors. Genomics 112 (6), 3958–3967. doi:10.1016/j.ygeno.2020.06.044
Edogbanya, J., Tejada‐Martinez, D., Jones, N. J., Jaiswal, A., Bell, S., and Cordeiro, R. (2021). Evolution, Structure and Emerging Roles of C1ORF112 in DNA Replication, DNA Damage Responses, and Cancer. Cell Mol Life Sci. 78 (9), 4365–4376. doi:10.1007/s00018-021-03789-8
Fiori, M., Di Franco, S., Villanova, L., Bianca, P., Stassi, G., and De Maria, R. (2019). Cancer-associated Fibroblasts as Abettors of Tumor Progression at the Crossroads of EMT and Therapy Resistance. Mol. Cancer 18 (1), 70. doi:10.1186/s12943-019-0994-2
Hong, W., Gu, Y., Guan, R., Xie, D., Zhou, H., and Yu, M. (2020). Pan-cancer Analysis of the CASP Gene Family in Relation to Survival, Tumor-Infiltrating Immune Cells and Therapeutic Targets. Genomics 112 (6), 4304–4315. doi:10.1016/j.ygeno.2020.07.026
Howe, K. L., Achuthan, P., Allen, J., Allen, J., Alvarez-Jarreta, J., Amode, M. R., et al. (2021). Ensembl 2021. Nucleic Acids Res. 49, D884–D891. doi:10.1093/nar/gkaa942
Huang, C., Hu, C.-G., Ning, Z.-K., Huang, J., and Zhu, Z.-M. (2020). Identification of Key Genes Controlling Cancer Stem Cell Characteristics in Gastric Cancer. Wjgs 12 (11), 442–459. doi:10.4240/wjgs.v12.i11.442
ICGC/TCGA Pan-Cancer Analysis of Whole Genomes Consortium(2020). Pan-cancer Analysis of Whole Genomes. nature 578 (7793), 82–93. https://pubmed.ncbi.nlm.nih.gov/?term=ICGC%2FTCGA+Pan-Cancer+Analysis+of+Whole+Genomes+Consortium%5BCorporate+Author%5D
Ju, Q., Li, X., Zhang, H., Yan, S., Li, Y., and Zhao, Y. (2020a). NFE2L2 Is a Potential Prognostic Biomarker and Is Correlated with Immune Infiltration in Brain Lower Grade Glioma: A Pan-Cancer Analysis. Oxid. Med. Cell. longev. 2020, 3580719. doi:10.1155/2020/3580719
Ju, Q., Li, X., Zhang, H., and Zhao, Y. (2020b). BRCA1-Associated Protein Is a Potential Prognostic Biomarker and Is Correlated with Immune Infiltration in Liver Hepatocellular Carcinoma: A Pan-Cancer Analysis. Front. Mol. Biosci. 7, 573619. doi:10.3389/fmolb.2020.573619
Laurini, E., Marson, D., Fermeglia, A., Aulic, S., Fermeglia, M., Pricl, S., et al. (2020). Role of Rad51 and DNA Repair in Cancer: A Molecular Perspective. Pharmacol. Ther. 208, 107492. doi:10.1016/j.pharmthera.2020.107492
Liu, L., Liu, L., Yao, H., Zhu, Z., Ning, Z., and Huang, Q. (2016). Stromal Myofibroblasts Are Associated with Poor Prognosis in Solid Cancers: A Meta-Analysis of Published Studies. PloS one 11 (7), e0159947. doi:10.1371/journal.pone.0159947
Lv, Z., Qi, L., Hu, X., Mo, M., Jiang, H., Fan, B., et al. (2021). Zic Family Member 2 (ZIC2): a Potential Diagnostic and Prognostic Biomarker for Pan-Cancer. Front. Mol. Biosci. 8, 631067. doi:10.3389/fmolb.2021.631067
Malta, T. M., Sokolov, A., Gentles, A. J., Burzykowski, T., Poisson, L., Weinstein, J. N., et al. (2018). Machine Learning Identifies Stemness Features Associated with Oncogenic Dedifferentiation. Cell 173 (2), 338–e15. doi:10.1016/j.cell.2018.03.034
Nalepa, G., and Clapp, D. W. (2018). Fanconi Anaemia and Cancer: an Intricate Relationship. Nat. Rev. Cancer 18 (3), 168–185. doi:10.1038/nrc.2017.116
Ryner, L., Guan, Y., Firestein, R., Xiao, Y., Choi, Y., Rabe, C., et al. (2015). Upregulation of Periostin and Reactive Stroma Is Associated with Primary Chemoresistance and Predicts Clinical Outcomes in Epithelial Ovarian Cancer. Clin. Cancer Res. 21 (13), 2941–2951. doi:10.1158/1078-0432.ccr-14-3111
Sanchez-Carbayo, M., Socci, N. D., Richstone, L., Corton, M., Behrendt, N., Wulkfuhle, J., et al. (2007). Genomic and Proteomic Profiles Reveal the Association of Gelsolin to TP53 Status and Bladder Cancer Progression. Am. J. Pathol. 171 (5), 1650–1658. doi:10.2353/ajpath.2007.070338
Schaub, F. X., Dhankani, V., Berger, A. C., Trivedi, M., Richardson, A. B., Shaw, R., et al. (2018). Pan-cancer Alterations of the MYC Oncogene and its Proximal Network across the Cancer Genome Atlas. Cell Syst. 6 (3), 282–e2. doi:10.1016/j.cels.2018.03.003
Sung, H., Ferlay, J., Siegel, R. L., Laversanne, M., Soerjomataram, I., Jemal, A., et al. (2021). Global Cancer Statistics 2020: GLOBOCAN Estimates of Incidence and Mortality Worldwide for 36 Cancers in 185 Countries. CA Cancer J Clin. 71, 209–249. doi:10.3322/caac.21660
Tang, Z., Kang, B., Li, C., Chen, T., and Zhang, Z. (2019). GEPIA2: an Enhanced Web Server for Large-Scale Expression Profiling and Interactive Analysis. Nucleic Acids Res. 47, W556–W560. doi:10.1093/nar/gkz430
van Dam, S., Cordeiro, R., Craig, T., van Dam, J., Wood, S. H., and de Magalhães, J. (2012). GeneFriends: an Online Co-expression Analysis Tool to Identify Novel Gene Targets for Aging and Complex Diseases. BMC Genomics 13, 535. doi:10.1186/1471-2164-13-535
Way, G. P., Sanchez-Vega, F., La, K., Armenia, J., Chatila, W. K., Luna, A., et al. (2018). Machine Learning Detects Pan-Cancer Ras Pathway Activation in the Cancer Genome Atlas. Cell Rep. 23 (1), 172–180.e3. doi:10.1016/j.celrep.2018.03.046
Weinstein, J. N., Collisson, E., Collisson, E. A., Mills, G. B., Shaw, K. R. M., Ozenberger, B. A., et al. (2013). The Cancer Genome Atlas Pan-Cancer Analysis Project. Nat. Genet. 45 (10), 1113–1120. doi:10.1038/ng.2764
Yang, D., Khan, S., Sun, Y., Hess, K., Shmulevich, I., Sood, A. K., et al. (2011). Association of BRCA1 and BRCA2 Mutations with Survival, Chemotherapy Sensitivity, and Gene Mutator Phenotype in Patients with Ovarian Cancer. JAMA 306 (14), 1557–1565. doi:10.1001/jama.2011.1456
Ye, W., Luo, C., Liu, F., Liu, Z., and Chen, F. (2021). CD96 Correlates with Immune Infiltration and Impacts Patient Prognosis: A Pan-Cancer Analysis. Front. Oncol. 11, 634617. doi:10.3389/fonc.2021.634617
Keywords: C1ORF112, prognosis, pan-cancer, biomarker, cell cycle
Citation: Chen J, Mai H, Chen H, Zhou B, Hou J and Jiang D-K (2021) Pan-Cancer Analysis Identified C1ORF112 as a Potential Biomarker for Multiple Tumor Types. Front. Mol. Biosci. 8:693651. doi: 10.3389/fmolb.2021.693651
Received: 11 April 2021; Accepted: 26 July 2021;
Published: 19 August 2021.
Edited by:
Ismail Hosen, University of Dhaka, BangladeshCopyright © 2021 Chen, Mai, Chen, Zhou, Hou and Jiang. This is an open-access article distributed under the terms of the Creative Commons Attribution License (CC BY). The use, distribution or reproduction in other forums is permitted, provided the original author(s) and the copyright owner(s) are credited and that the original publication in this journal is cited, in accordance with accepted academic practice. No use, distribution or reproduction is permitted which does not comply with these terms.
*Correspondence: De-Ke Jiang, dekejiang17@smu.edu.cn; Jinlin Hou, jlhousmu@163.com
†These authors have contributed equally to this work and share first authorship