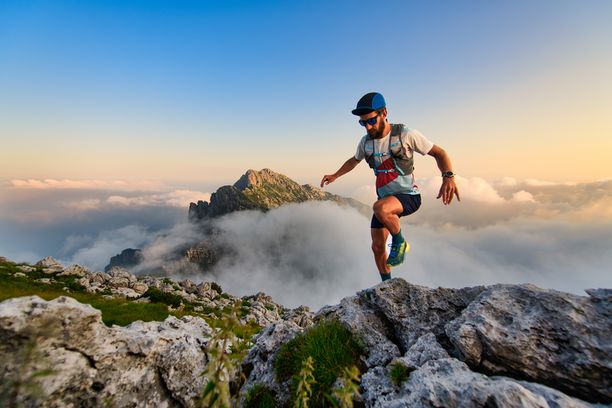
94% of researchers rate our articles as excellent or good
Learn more about the work of our research integrity team to safeguard the quality of each article we publish.
Find out more
ORIGINAL RESEARCH article
Front. Mol. Biosci., 11 January 2021
Sec. Molecular Diagnostics and Therapeutics
Volume 7 - 2020 | https://doi.org/10.3389/fmolb.2020.603701
A retraction of this article was approved in:
Retraction: Identification of an immunologic signature of lung adenocarcinomas based on genome-wide immune expression profiles
Citation: Ling B, Ye G, Zhao Q, Jiang Y, Liang L and Tang Q (2021) Identification of an Immunologic Signature of Lung Adenocarcinomas Based on Genome-Wide Immune Expression Profiles. Front. Mol. Biosci. 7:603701. doi: 10.3389/fmolb.2020.603701
Received: 07 September 2020; Accepted: 03 December 2020;
Published: 11 January 2021; Retracted: 31 July 2024.
Edited by:
Mahendra Pratap Kashyap, University of Alabama at Birmingham, United StatesReviewed by:
Rakesh Pathak, National Institutes of Health Clinical Center (NIH), United StatesDisclaimer: All claims expressed in this article are solely those of the authors and do not necessarily represent those of their affiliated organizations, or those of the publisher, the editors and the reviewers. Any product that may be evaluated in this article or claim that may be made by its manufacturer is not guaranteed or endorsed by the publisher.
Research integrity at Frontiers
Learn more about the work of our research integrity team to safeguard the quality of each article we publish.