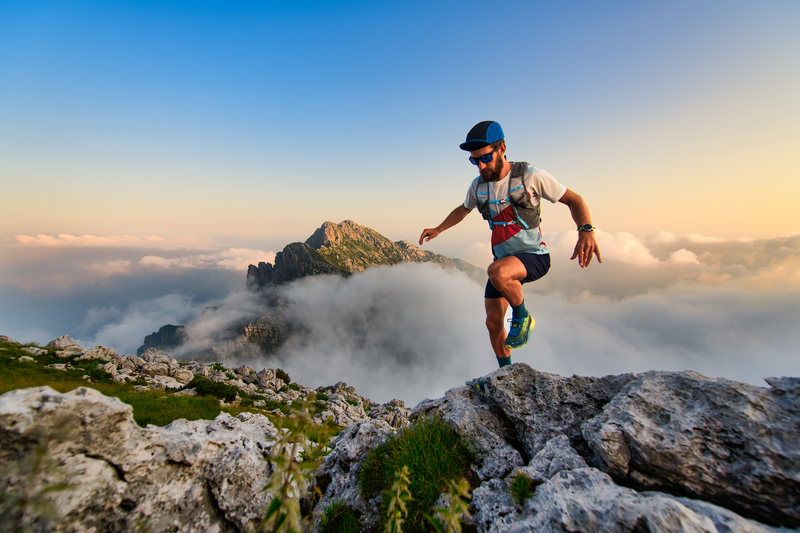
95% of researchers rate our articles as excellent or good
Learn more about the work of our research integrity team to safeguard the quality of each article we publish.
Find out more
BRIEF RESEARCH REPORT article
Front. Microbiomes , 22 October 2024
Sec. Nutrition, Metabolism and the Microbiome
Volume 3 - 2024 | https://doi.org/10.3389/frmbi.2024.1426150
Chronic Obstructive Pulmonary Disease (COPD) affects 30 million Americans. Previous epidemiologic work has shown that diet can impact pulmonary function in those with and without COPD. Diet is also a major driver of gut microbiome composition and function. Importantly, the gut microbiome has also been associated with lung health (i.e., the gut-lung axis) in both preclinical and clinical studies. Despite this growing body of evidence, many questions remain regarding the gut-lung axis. Specifically, how the microbiome impacts the relationship between diet factors and spirometry or stage of disease in people with COPD is little understood. We hypothesize that there are taxonomic differences in the gut microbiome among the different stages of COPD and that diet microbiome interactions influence pulmonary function. This study aimed to identify how the GI microbiota correlated with the severity of respiratory disease in COPD patients and how the microbiome may mediate the relationship between diet, including fiber and omega-3 fatty acids, and lung function outcomes.
Chronic Obstructive Pulmonary Disease (COPD) affects 30 million Americans (Prevention CfDCa, 2022) and was the sixth overall cause of death in 2021 (Prevention CfDCa, 2023). However, prior to the COVID-19 pandemic, it was the third leading cause of death worldwide (Organization WH). In addition to excess mortality, COPD increases morbidity, often leading to disability. COPD disproportionately affects women compared to men (Prevention CfDCa, 2022), as well as those who are socioeconomically disadvantaged (Buttery et al., 2021). The leading cause of COPD is tobacco smoke, with cigarette smoking being responsible for 8 out of 10 COPD-related deaths (Prevention CfDCa; Services USDoHaH, 2014). Despite smoking rates in the United States being cut in half since 1964, between 2011 and 2020, the prevalence of COPD remained unchanged. Likewise, the overall age-adjusted death rate has not changed from 1999-2019. These data emphasize smoking cessation alone may not be sufficient to optimize lung function outcomes in smokers, creating a need to examine additional modifiable lifestyle factors, such as dietary intake, as a complementary strategy for improving lung health.
Previous epidemiologic work has shown that diet can impact pulmonary function in those with COPD (Hanson et al., 2014). A “Western” diet pattern, generally categorized as high in refined grains, cured and red meats, added sugars, and fat, has also been identified as a risk factor for COPD (Young and Hopkins, 2018; Scoditti et al., 2019), impacting both men (Varraso et al., 2007b) and women (Varraso et al., 2007a). However, individual dietary factors, such as fiber and omega-3 fatty acids, have previously been identified as protective against COPD (Kan et al., 2008; Varraso et al., 2010; Hanson et al., 2016; Young et al., 2016; Saeed et al., 2020; Ding et al., 2021; Qu et al., 2022) and are potentially associated with reduced respiratory symptoms (Lemoine et al., 2019). One possible mechanism for the positive impact of fiber and omega-3 fatty acids on COPD is through supporting the gut microbiome. The gut microbiome is known to have a profound impact on the immune system, metabolism, and homeostasis. Through its metabolites, the gut microbiota impacts tissues far beyond the intestinal mucosa (Moloney et al., 2014; Albillos et al., 2020; Zhao et al., 2021; Ashique et al., 2022). Increasingly, the gut microbiome is being associated with lung health. This is becoming termed the “gut-lung axis” as an encompassing term for the cross-communication between intestinal and lung microbiota and resultant co-regulation of local and systemic response to environmental and intrinsic exposures (Ding et al., 2021; Zhao et al., 2021; Ashique et al., 2022; Qu et al., 2022). Through this association, we now know that noxious pulmonary exposures (Buttery et al., 2021), including cigarette smoke (Ding et al., 2021), can induce intestinal dysbiosis. However, dietary factors, including fiber and omega-3 fatty acids, are also key modifiers of the gut microbial composition, including microbes that produce anti-inflammatory short-chain fatty acids (So et al., 2018; Watson et al., 2018; Wagenaar et al., 2021).
Despite this growing body of evidence, many questions remain regarding the gut-lung axis. Spirometry measures the maximum volume and duration a participant can inspire and then forcefully and completely expire. These tests are considered the gold standard for the measurement of lung function, allowing for the classification of COPD severity. Little is known about whether microbiome diversity correlates with the Global Initiative for Obstructive Lung Disease (GOLD) severity stages of COPD and how nutrient intake might influence the microbiome and mediate lung function and stage of disease in people with COPD. We hypothesize that there are taxonomic differences in the gut microbiome among the different stages of COPD. This study aimed to identify the associations between nutrient intakes and respiratory disease stage in COPD patients, as well as how the microbiome may mediate the relationship between diet, including fiber and omega-3 fatty acids, and lung function outcomes.
This study is a secondary analysis of data collected in the Comparing Urban and Rural Effects on COPD (CURE COPD, NIH grant #P50-ES026096) Center at Johns Hopkins University (JHU). The urban cohort of CURE COPD is an observational study of 99 former smokers with moderately severe COPD, followed longitudinally for six months. CURE COPD participants were evaluated at baseline, 3, and 6 months, with assessment of lung function, diet, and collection of stool samples. Inclusion criteria were 1) age ≥ 40 years, 2) physician diagnosis of COPD, 3) GOLD Stage I-IV disease with FEV1/FVC ≤70% and FEV1 (% predicted) < 80%, 4) tobacco exposure ≥ 10 pack-years, and 5) former smoker (identified those who report no current smoking in the past one year and have exhaled CO levels ≤ 6ppm. This threshold was chosen to maximize the chance of distinguishing true smokers and ex-smokers (> 95%). Exclusion criteria include; 1) use of chronic systemic corticosteroids (≥ 3 months continuous), 2) BMI less than 18.5, and 3) pregnancy or breastfeeding. The catchment area of the study represents a low socioeconomic status (SES) population from Baltimore and surrounding areas with high rates of Medicaid coverage (90%), 78% have a high school education or less, and 57% report less than $15,000 of yearly household income. All studies were approved by the JHU IRB.
Participants were given a fecal sampling kit with detailed instructions. Using an established protocol, the subject is asked to swab fresh feces from toilet paper and insert the swab into a sterile screw-cap tube with cell lysis and DNA stabilization buffer (American Gut Project). The subject is then given a postage-paid mailing pouch for return to the study coordinator. Samples collected for this study were obtained prior to the COVID-19 pandemic.
The primary outcomes of the study were lung function and COPD stage. Lung function was assessed as FEV1 and FEV1% predicted (FEV1, adjusted for age, height, and sex) according to ATS guidelines (Miller et al., 2005) using a KOKO® (Pulmonary Data Services, Inc., Louisville, CO) pneumotach. Predicted values for FEV1 were calculated by formulae of Hankinson et al. (1999). At each test, three sets of values were obtained, and the highest set of FEV1 and FVC measurements was used. Global Initiative for Obstructive Lung Disease (GOLD) criteria was used to classify COPD severity (Fromer and Cooper, 2008).
Analysis of the microbiome was performed on stool samples collected from patients. The DNA quality was checked using a Qubit 3.0 fluorometer before 16S Metagenomic Sequencing Library Preparation following Illumina MiSeq pair-end protocol. The protocol targeted the variable 16S V3 and V4 regions. After libraries were quantified and normalized, a 4nM pool of all samples was denatured and diluted to 8 pM. This pool was loaded onto the Illumina MiSeq for a 300bp paired-end run using the MiSeq v3 600 cycle kit.
Sequences were demultiplexed using the Illumina software, according to the manufacturer’s guidelines. Bioinformatics analyses were performed following the Bioconductor workflow for microbiome data analysis by Callahan et al. (Callahan et al., 2016) using the R software (version 4.2.1). For denoising, the R package DADA2 (Callahan et al., 2016) (version 1.18.0) was used following these conditions: the forward reads were truncated at position 290 while the reverse ones were truncated at position 260 to discard positions for which nucleotide median quality was Q25 or below. High-quality sequencing reads were clustered to infer amplicon sequence variants (ASV), and a final table of ASV counts per sample was generated after removing chimeras. A naïve Bayes taxonomy classifier (Wang et al., 2007) was used to classify each ASV against the SILVA 138.1 reference database, while MAFFT (Katoh and Standley, 2013) (version 7.407) and FASTTREE (Price et al., 2009) (version 2.1.11) programs were used to construct a phylogenetic tree. Taxa abundances were normalized with the total sum scaling normalization method, dividing each ASV count by the total library size to yield their relative proportion of counts for each sample. Alpha diversity was studied with several diversity indices with the R packages phyloseq (McMurdie and Holmes, 2013) (version 1.34.0) and picante (Kembel et al., 2010) (Version 1.8.2). Principal coordinates analysis (PCoA) via various distance matrices (Bray Curtis, Jaccard, weighted and unweighted UniFrac) was used to evaluate beta diversity and to plot patterns of microbiome community diversity. Analysis of variance of the distance matrices was performed with the nonparametric MANOVA test Adonis with 999 permutations (PERMANOVA) as implemented in the R package vegan (Oksanen, 2022) (version 2.5-7).
Nutrient intake was assessed using the Willett Food Frequency Questionnaire (FFQ). The FFQ provides information on the usual intake of many foods, food groups, and supplements. From responses to the questionnaire, individualized nutrient intake can be calculated based on the known nutrient content of foods. The FFQ was analyzed by trained personnel at the Harvard School of Public Health. Nutrients of interest for this analysis were based on previous associations with lung function in the literature and included total fiber intake, total omega (n)-3 fatty acid intake (FA) (including alpha-linolenic acid), intake of specific n-3 FAs [Docosahexaenoic acid (DHA) + eicosapentaenoic acid (EPA)], and total n-6 fatty acids. Using this data, a ratio of total n-6:total n-3 FAs was calculated.
Prior to analysis, all data was tested for normality using the D’Agostino & Pearson test method. All data not normally distributed was log-transformed prior to analysis. Differential abundance analyses were performed using the R package corncob (Martin et al., 2020) (version 0.2.0) to reveal statistically significantly changed taxa. Regularized Canonical Correlation Analysis (rCCA) was performed using R package mixOmics (Rohart et al., 2017) (version 6.14.1) to explore associations between nutrient intake, microbiome, and pulmonary outcomes. rCCA is a standard method for microbiome studies and accounts for the high dimensionality and/or high collinearities of the datasets, both of which are commonly seen in microbiome and biological studies. For taxa differential abundance analysis, the Benjamini-Hochberg (BH) procedure (Benjamini and Hochberg, 1995) was applied to correct for multiple hypothesis testing. Mediation analyses were performed using the MODIMA method by Hamidi et al. (Hamidi et al., 2019) and the CCMM method by Sohn et al. (Michael and Hongzhe, 2019) to evaluate the mediation effect of the microbiome as a community and individual taxa after agglomerating at the genus level, respectively, between dietary covariates as exposure and lung function outcomes. Prediction of metagenomics functions was performed using PICRUSt2 (Douglas et al., 2020) (version 2.4) and Pfam (Finn et al., 2007) database of protein families. Unless specified, an adjusted p-value of less than 0.05 was used for the significance level.
A total of 54 individuals who had completed fecal sample collection were included in the analysis. COPD GOLD stages were divided among the groups as follows: 7.4% (n = 4) GOLD stage I, 57.4% (n = 31) GOLD stage II, 27.8% (n = 15) stage III, and 7.4% (n = 4) stage IV. The mean age was 64 years, with an average BMI of 34.7. The study population was 67% female (n = 36) with 26 (48%) white, non-Hispanic patients and 28 (52%) black participants. Baseline characteristics of the study population are provided in Table 1.
We examined the composition of the microbiota to determine whether there were differences in the dominant phyla of bacteria of the gut microbiota between the different stages of COPD, we measured the relative abundance of the major bacterial phylum in each GOLD stage for each participant. (Supplementary Figure 1). In each GOLD stage, the Firmicutes and Bacteroidetes phyla dominated the gut microbial communities. Analysis of α-diversity metrics were calculated for each sample using Observed species, Chao1, and Shannon diversity measures. No significant difference in α-diversity was observed between GOLD stages (Supplementary Figure 2A). Similarly, analysis of β-diversity showed no significant differences in β-diversity between any GOLD stages (Supplementary Figure 2B).
We then examined the data at the genus and order level. Despite the homogeneity in the phyla, there were clear changes in the relative abundance of specific microbial taxa between GOLD stages at both the genus (Figure 1A) and order (Figure 1B) levels. In this data, the dashed lines represent the level of expression in Gold Stage 1. Data to the left of the line shows decreases, while data to the right of the line shows increases. Changes that are significant do not cross the dashed line and are in blue. Compared to GOLD stage I, there were significant decreases in the abundance of three Ruminococcaceae (Acetanaerobacterium, UBA1819, and 5) (Figure 1A) while the abundance of Streptococcus increased as GOLD stage increased (Figure 1A). At the order level, Erysipelotrichales and Desulfovibrionales were significantly decreased in GOLD stages 2, 3, and 4 when compared to GOLD stage I (Figure 1B). Overall, as COPD severity increases, as measured by GOLD stage, there are more significant changes in the microbiome.
Figure 1. Differentially abundant taxa between different COPD Gold stages. Differentially abundant taxa at the (A) genus level and (B) order level in COPD Gold Stage 2 v 1 (left panel), COPD Gold Stage 3 v 1 (middle panel), and COPD Gold Stage 4 v 1 (right panel) using the corncob method with Benjamini-Hochberg (BH) correction for multiple comparisons. Blue lines indicate taxa that are significantly different between the compared GOLD Stages. Specifically, if the blue line is positive, then the indicated taxa is increased in GOLD Stage 2,3,4 compared to Stage 1; however, if the blue line is negative, then the indicated taxa is decreased in GOLD Stage 2,3,4 when compared to Stage 1.
Next, we wanted to better understand the potential functional impacts of these changes in bacterial taxa that were associated with COPD GOLD stage. To do this, we performed a PICRUSt2 analysis to predict the function of the metagenome (Douglas et al., 2020). In our dataset, PICRUSt2 analysis found that there were 161 upregulated pathways (Shown in red) and 20 downregulated pathways (Shown in blue) in COPD GOLD stage 2 compared to stage 1 (Supplementary Figure 3A), while there were 180 upregulated pathways and 154 downregulated pathways in COPD GOLD stage 3 compared to stage 1 (Supplementary Figure 3B). Finally, there were 117 upregulated pathways and 308 downregulated pathways in COPD GOLD stage 4 compared to stage 1 (Supplementary Figure 3C). A summary of the differential pathways via PICRUSt2 and DESeq2 analysis is provided in Supplementary Table 1.
Certainly, the stage of COPD is not the only relevant clinical characteristic that could alter the microbiome. Dietary intake can also dramatically affect the microbiome. Because previous studies have shown that dietary intake can influence the rate of lung function decline, we wanted to look at dietary factors and lung function together. To do this, we performed canonical correlation analysis to evaluate the correlation between the microbiome, dietary covariates (i.e., fiber, total omega, omega-3, omega-6, and n6:n3 ratio), and lung function (i.e., FEV1% predicted, FEV1 and FVC). A network plot and heatmap showed the strength of the association between the microbiome and these parameters (Supplementary Figure 4). When both lung function and dietary considerations were considered in the model, the lung function outcome FEV1% predicted, and FEV1 were negatively associated with Rikenella and Lachnospiraceae (NK4B4 group). Likewise, FEV1% predicted was also negatively associated with Lachnospiraceae (UCG.008), Angelakisella, and Merdibacter. Conversely, FEV1 and FVC were positively associated with Desulfovibrio (Supplementary Figure 4A). On the other hand, dietary parameters total omega and omega-3 were positively associated with Succinivibrio (Supplementary Figure 4B). In summary, both lung function parameters and dietary parameters are associated with significant alterations in the microbiota.
To understand if the relationship between diet and lung function in COPD subjects was mediated by the gut microbiota, we performed a mediation analysis. Specifically, mediation analysis was performed using dietary parameters as the exposure (fiber, total omega, omega-3, omega-6, and n6:n3 ratio) and lung function parameter (FEV1% predicted, FEV1 and FVC) as outcome, and microbiome diversity metric Bray-Curtis as the mediator using the MODIMA method. In Figure 2, the first column of data shows how each dietary component influences FEV1% predicted, FEV1, and FVC. The second column shows how each dietary component influences beta diversity (PCo1 and PCo2) of the microbiome. The third column depicts how the microbiome associated with the dietary component influences FEV1% predicted, FEV1, and FVC. Higher levels of total omega fatty acids (Figure 2A), Omega-3 (Figure 2B), and Omega-6 fatty acids (Figure 2C) were associated with higher FEV1% predicted. Similarly, higher levels of total omega fatty acids (Figure 2D), Omega-3 (Figure 2E), and Omega-6 fatty acids (Figure 2F) were associated with higher FEV1. Finally, levels of total fiber (Figure 2G), total omega fatty acids (Figure 2H), and Omega-3 fatty acids (Figure 2I) were associated with higher FVC. We identified lung function FEV1% predicted, FEV1 and FVC, at least partially, to be significantly mediated by the microbiome when considering either fiber, total omega, omega-3, or omega-6 as exposure (Figure 2). However, this analysis method only provides a global test of community-level mediation, not the effects of individual components.
Figure 2. The GI microbial community partially mediates diet and lung function interactions in COPD subjects. Mediation analysis was performed with dietary parameters as the exposure and lung function parameters FEV1, FVC, and FEV1 % predicted as the outcome, and community composition as the mediator using the MODIMA method. Mediation analysis was performed using FEV1 % predicted as the outcome and (A) Omega, (B) Omega-3, and (C) Omega-6 as the exposures. Mediation analysis was performed using FEV1 as the outcome and (D) Omega, (E) Omega-3, and (F) Omega-6 as the exposures. Finally, mediation analysis was performed using FVC as the outcome and (G) Fiber, (H) Omega, and (I) Omega-3 as the exposures.
To better understand the effects of individual components of the microbiome, we performed a causal compositional mediation model (CCMM). Mediation analysis via CCMM showed indirect mediation effects of several taxa (Figure 3). Specifically, mediation analysis was performed using dietary parameters as the exposure (fiber, total omega fatty acid, omega-3, omega-6, and n6:n3 ratio) and lung function parameter FEV1% predicted, FEV1 and FVC as outcomes, and individual bacterial taxa as the mediator using the CCMM method. While mediation analysis did not reveal a significant direct effect (DE) or total indirect effect (TIDE) between diet (fiber, total omega, omega-3, omega-6, and the ratio of omega-6 to omega-3), the microbiome, and FEV1% predicted, FEV1 and FVC significant indirect component-wise mediation effects were observed. Specifically, for fiber, indirect component-wise mediation effects were seen for one bacterial taxon, CAG-56 (IDE of 0.044 with a CI of 0.009, 0.099), on FEV1% predicted. For total omega fatty acids, indirect component-wise mediation effects were seen for three bacterial taxa, Holdemanella (IDE of 0.024 with a CI of 0.004, 0.049), CAG-352 (IDE of 0.019 with a CI of 0.0003, 0.045), and Merdibacter (IDE of 0.012 with a CI of 0.002, 0.027), on FEV1% predicted. For omega-3 fatty acids, indirect component-wise mediation effects were seen for three bacterial taxa, CAG-56 (IDE of 0.032 with a CI of 0.002, 0.087), CAG-352 (IDE of 0.040 with a CI of 0.005, 0.086), and Merdibacter (IDE of 0.024 with a CI of 0.003, 0.052), on FEV1% predicted. For omega-6 fatty acids, indirect component-wise mediation effects were seen for one bacterial taxon, Merdibacter (IDE of 0.023 with a CI of 0.001, 0.054), on FEV1% predicted. For the ratio of omega-3 to omega-6 fatty acids, indirect component-wise mediation effects were seen for three bacterial taxa, Holdemanella (IDE of -0.106 with a CI of -0.217, -0.022), Paraprevotella (IDE of -0.054 with a CI of -0.131, -0.003), and Bacteroides (IDE of -0.060 with a CI of -0.201, -0.001), on FEV1% predicted. Similar results were seen for each dietary parameter, with either FEV1 or FVC as the outcome variable (Figure 3). A summary of the mediation analysis is shown in Supplementary Table 2.
Figure 3. Diet and lung function interaction in COPD subjects is partially mediated via specific bacterial taxa. Mediation analysis was performed with dietary parameters as the exposure and lung function parameters FEV1, FVC, and FEV1 % predicted as the outcome, and individual bacterial taxa as the mediator using the CCMM method. Mediation analysis was performed using Fiber, total omega, omega-3, omega-6, and n6:n3 ratio (from top to bottom) as the dietary exposure parameters.
COPD is a chronic lung disease principally caused by tobacco smoking. It is characterized by airway inflammation, obstruction on pulmonary function tests, chronic cough, and phlegm production. Because it is a lung disease, most microbiome studies have focused on the flora of the lungs (Leung et al., 2017; Dicker et al., 2021; Madapoosi et al., 2022) through sampling of bronchoalveolar lavage fluid, lung tissue, or sputum. These studies have firmly established that individuals who suffer from COPD have an altered lung microbiome compared to healthy individuals. While the lung microbiome is known to contribute to COPD progression (Qu et al., 2022), the function of the gut microbiome remains understudied. In this analysis, we sought to better understand how the gut microbiome changes in the different stages of COPD. Importantly, we also wanted to understand how dietary intake of key factors such as fiber and omega 3 and 6 fatty acids influence the gut microbiome in COPD.
We began our analysis by determining whether there were changes in the gut microbiome at the phylum level between the different stages of COPD (Supplementary Figure 1). While we did not identify dramatic changes between stages of COPD at this very high level, our data compares favorably to previous studies showing that Firmicutes and Bacteroidetes phyla dominated the gut microbial communities in those with COPD (Trompette et al., 2014; Chiu et al., 2021; Dicker et al., 2021). Likewise, other studies have not identified changes in gut microbiota in different stages of COPD (Qu et al., 2022). We went on to look at alpha diversity (Supplementary Figure 2A) and beta diversity (Supplementary Figure 2B) at the phylum level between the stages of COPD. We did not measure any changes at this level. This is similar to previously published studies (Qu et al., 2022). This indicates that our methods and patient selection compare favorably to those already published.
Despite the lack of changes at the phylum level, we did measure changes in the gut microbiome in both the order (Figure 1B) and Genus level (Figure 1A) with increasing COPD stage. One of the most consistent changes was increased Streptococcus species in stages II, III, and IV compared to stage I (Figure 1A). This data is consistent with previous reports of increases in Streptococcus species in the gut microbiome of COPD patients compared to control (Bowerman et al., 2020). Bowerman et al. report increased abundances of both Streptococcus sp000187445 and Streptococcus vestibularis. In that study, these strains were correlated with reduced lung function (Bowerman et al., 2020). Likewise, in a study of acute exacerbations of COPD, researchers found an increased abundance of both Streptococcus parasanguinis_B and Streptococcus salivarius in the fecal microbiome (Chan et al., 2015). Interestingly, streptococcus species are also increased in the upper GI tract microbiome of current smokers compared to never smokers (So et al., 2018).
We went on to examine what effect the differing microbiome could have. Compared to the microbiome of stage I COPD, Stages II, III, and IV had multiple proteins that are predicted to be differentially expressed. Many of these genes were upregulated up to nearly 30-fold, suggesting that this could make a dramatic difference in the microenvironment. This suggests that the changes in the composition of the microbiota have the potential to create physiologic differences. We observed changes in predicted functional protein families for lipid, xenobiotic, and amino acid metabolism. Like previous studies, we found significant correlations between lung function (FEV1% predicted, FEV1 and FVC) and the intestinal microbiota; FEV1% predicted and FEV1 were negatively associated Rikenella and Lachnospiraceae (NK4B4 group). Likewise, FEV1% predicted was also negatively associated Lachnospiraceae (UCG.008), Angelakisella, and Merdibacter. Conversely, FEV1 and FVC were positively associated with Desulfovibrio.
Diet is also an important factor influencing both COPD and the composition of the intestinal microbiota (Sorli-Aguilar et al., 2016; Jang et al., 2020; Lai et al., 2022; Kawashima et al., 2024). Dietary fiber content changes the composition of the GI microbiota by altering the ratio of Firmicutes to Bacteroidetes (Trompette et al., 2014; Chiu et al., 2021; Dicker et al., 2021), which can directly affect how the gut microbiota metabolizes fiber. The metabolism of fiber by the GI microbiota results in the production of short-chain fatty acids (SCFA), which have been shown to have anti-inflammatory properties. Intestinal microbiota-mediated production of various SCFAs is important for host systemic immunity (Meijer et al., 2010; den Besten et al., 2013; Trompette et al., 2014). SCFAs aid in the control of both asthma and allergic inflammation in the lungs (Trompette et al., 2014; Saeed et al., 2020). For example, mice fed a high-fiber diet had increased circulating levels of SCFAs and were protected against allergic inflammation in the lung, whereas a low-fiber diet decreased levels of SCFAs and increased allergic airway disease (Saeed et al., 2020). In addition, recently in mouse models of cigarette smoke induced COPD, it was found that fecal microbiota transfer of “healthy” microbiota mitigated inflammation, alveolar destruction, and impaired lung function associated with COPD. Furthermore, COPD markers correlated with the relative abundance of Muribaculaceae, Desulfovibrionaceae and Lachnospiraceae family members. Finally, glucose and starch metabolism were significantly downregulated in the COPD-associated microbiota, and supplementation of mice or human patients with complex carbohydrates improved disease outcomes (Budden et al., 2024).
With regards to fatty acids, recent evidence has highlighted the fact that these compounds have the potential to remodel the gut microbiome, and that these changes may modulate lung metabolism (Li et al., 2023). While these findings have been mostly in animal experiments, our study provides important confirmation that the gut-lung axis can be modified by nutrition intake. Using two different mediation analyses, we found that the relationship between diet and lung function was partially mediated by the intestinal microbiota, at both a community level and individual taxa level. Specifically, fiber effects on FEV1% predicted via the gut microbiome were driven primarily by 1 bacterial taxon (e.g., CAG-56). Fiber effects on FEV1 via the gut microbiome were driven by five bacterial taxa (e.g., Fusicatenibacter). Likewise, fiber’s effects on FVC via the GI microbiome were driven by four bacterial taxa (e.g., Prevotella_2). In other words, our data suggest that as dietary fiber increases the abundance of CAG-56, Fusicatenibacter, Megasphaera, and Lachnospiraceae_NK4A136_group increase while decreasing the abundance of Odoribacter, which together improve lung function (FEV1% predicted, FEV1, and FVC). Similar results were observed when evaluating the relationship between total dietary omega-fatty acids and lung function, which was partially mediated by the intestinal microbiota at both the community level and individual taxa level. Total dietary omega-fatty acids effects on FEV1% predicted via the GI microbiome were driven by 3 bacterial taxa, Holdemanella, CAG-352, and Merdibacter. Total dietary omega-fatty acids effects on FEV1 were driven by four bacterial taxa (e.g., Fusicatenibacter). Likewise, total dietary omega-fatty acids effects on FVC via the gut microbiome were driven by two bacterial taxa (e.g., Odoribacter). In other words, as total dietary omega-fatty acids increase the abundance of Fusicatenibacter, Holdemanella, and Dorea increase while decreasing the abundance of Odoribacter, which together leads to improved lung function (FEV1% predicted, FEV1, and FVC). These results are supported by previously published data as Fusicatenibacter, Megasphaera, Lachnospiraceae_NK4A136_group, Holdemanella, and Dorea have all previously been shown to increase with the addition of dietary fiber (Burns et al., 2018; Myhrstad et al., 2020; Burakova et al., 2022; Huang et al., 2023; Zhao et al., 2024). Interestingly, the reduction in Odoribacter and associated improvements in lung function is in line with similar studies. For example, Nontuberculous mycobacterial infected mice showed decreased levels of L-arginine in sera, and oral administration of L-arginine or fecal microbiota transplantation from supplemented mice mitigated NTM infection and increased macrophage and Th1 responses. These changes were also associated with alteration to the gut microbiota, with an increased relative abundance of Bifidobacterium, Bilophila, and unclassified YS2 and a decreased relative abundance of Odoribacter, Prevotella, and Akkermansia (Kim et al., 2022). Similarly, in another study, Odoribacter was identified to have the strongest impact on the development of squamous cell lung carcinoma (Chen et al., 2024). Conversely, increases in Fusicatenibacter and associated improvements in lung function are similar to studies that identified the changes in the relative abundance of specific genera, which were associated with significant alterations in fecal metabolites of patients with tuberculosis (TB) (Wang et al., 2022). Specifically, Bacteroides, Parabacteroides, Fusobacterium, and Lachnoclostridium were enriched in TB patients while Blautia, Roseburia, Bifidobacterium, unidentified Ruminococcaceae, Fusicatenibacter, and Romboutsia were enriched in healthy people, all of which was associated with a decrease the production of SCFAs (Wang et al., 2022). However, additional experiments need to be performed to validate a functional mediation effect of the microbiota in relationship to all dietary and lung functional parameters. While our study focused on critical dietary exposures with biological plausibility of impacting the gut microbiome and lung function, future exploration of additional dietary components, such as more pro-inflammatory foods, and other potential confounders, such as sex, age, and race should be included in larger studies with adequate analysis power. In conclusion, our study strengthens established associations between the colonization of Streptococcus and COPD (Leung et al., 2017; Bowerman et al., 2020; Dicker et al., 2021; Madapoosi et al., 2022; Sim et al., 2022), as well as provides novel insight into the relationship between diet, the microbiome, and lung function in subjects with COPD.
The datasets presented in this study can be found in online repositories. The names of the repository/repositories and accession number(s) can be found in the article/Supplementary Material.
The studies involving humans were approved by Johns Hopkins School of Medicine Institutional Review Board. The studies were conducted in accordance with the local legislation and institutional requirements. The participants provided their written informed consent to participate in this study.
HQ: Data curation, Formal analysis, Methodology, Visualization, Writing – original draft, Writing – review & editing. RC: Data curation, Formal analysis, Writing – original draft, Writing – review & editing. MJ: Formal analysis, Writing – review & editing, Data curation. J-JR: Data curation, Formal analysis, Methodology, Writing – review & editing. NH: Funding acquisition, Project administration, Resources, Supervision, Writing – review & editing. KB: Conceptualization, Formal analysis, Supervision, Writing – review & editing. CH: Conceptualization, Data curation, Formal analysis, Methodology, Writing – review & editing. DS: Conceptualization, Data curation, Methodology, Resources, Supervision, Writing – original draft, Writing – review & editing.
The author(s) declare financial support was received for the research, authorship, and/or publication of this article. National Institutes of Health, National Institute of Environmental Health Sciences, Grants #P50-ES026096 (to NH, CH); National Institutes of Health, the National Institute on Alcohol Abuse and Alcoholism, Grants #R00-AA026336 (to DS); National Institutes of Health; the National Institute of Diabetes and Digestive and Kidney Diseases Grant #R01-DK131990-01 (to DS). The University of Nebraska DNA Sequencing Core receives partial support from the National Institute for General Medical Science (NIGMS) INBRE - P20GM103427-19 grant as well as The Fred & Pamela Buffett Cancer Center Support Grant - P30 CA036727.
We would like to especially thank all the study participants from CURE COPD, without their support this project would not have been possible. We would also like to thank The University of Nebraska DNA Sequencing Core.
The authors declare that the research was conducted in the absence of any commercial or financial relationships that could be construed as a potential conflict of interest.
All claims expressed in this article are solely those of the authors and do not necessarily represent those of their affiliated organizations, or those of the publisher, the editors and the reviewers. Any product that may be evaluated in this article, or claim that may be made by its manufacturer, is not guaranteed or endorsed by the publisher.
This publication’s contents are the sole responsibility of the authors and do not necessarily represent the official views of the NIH or NIGMS.
The Supplementary Material for this article can be found online at: https://www.frontiersin.org/articles/10.3389/frmbi.2024.1426150/full#supplementary-material
Albillos A., de Gottardi A., Rescigno M. (2020). The gut-liver axis in liver disease: Pathophysiological basis for therapy. J. Hepatol. 72, 558–577. doi: 10.1016/j.jhep.2019.10.003
Ashique S., De Rubis G., Sirohi E., Mishra N., Rihan M., Garg A., et al. (2022). Short Chain Fatty Acids: Fundamental mediators of the gut-lung axis and their involvement in pulmonary diseases. Chem. Biol. Interact. 368, 110231. doi: 10.1016/j.cbi.2022.110231
Benjamini Y., Hochberg Y. (1995). Controlling the false discovery rate: A practical and powerful approach to multiple testing. J. R. Stat. Society: Ser. B (Methodological) 57, 289–300. doi: 10.1111/j.2517-6161.1995.tb02031.x
Bowerman K. L., Rehman S. F., Vaughan A., Lachner N., Budden K. F., Kim R. Y., et al. (2020). Disease-associated gut microbiome and metabolome changes in patients with chronic obstructive pulmonary disease. Nat. Commun. 11, 5886. doi: 10.1038/s41467-020-19701-0
Budden K. F., Shukla S. D., Bowerman K. L., Vaughan A., Gellatly S. L., Wood D. L. A., et al. (2024). Faecal microbial transfer and complex carbohydrates mediate protection against COPD. Gut. 73, 751–769. doi: 10.1136/gutjnl-2023-330521
Burakova I., Smirnova Y., Gryaznova M., Syromyatnikov M., Chizhkov P., Popov E., et al. (2022). The effect of short-term consumption of lactic acid bacteria on the gut microbiota in obese people. Nutrients 14. doi: 10.3390/nu14163384
Burns A. M., Solch R. J., Dennis-Wall J. C., Ukhanova M., Nieves C. Jr., Mai V., et al. (2018). In healthy adults, resistant maltodextrin produces a greater change in fecal bifidobacteria counts and increases stool wet weight: a double-blind, randomized, controlled crossover study. Nutr. Res. 60, 33–42. doi: 10.1016/j.nutres.2018.09.007
Buttery S. C., Zysman M., Vikjord S. A. A., Hopkinson N. S., Jenkins C., Vanfleteren L. (2021). Contemporary perspectives in COPD: Patient burden, the role of gender and trajectories of multimorbidity. Respirology. 26, 419–441. doi: 10.1111/resp.14032
Callahan B. J., McMurdie P. J., Rosen M. J., Han A. W., Johnson A. J., Holmes S. P. (2016). DADA2: High-resolution sample inference from Illumina amplicon data. Nat. Methods 13, 581–583. doi: 10.1038/nmeth.3869
Callahan B., Sankaran K., Fukuyama J., McMurdie P., Holmes S. (2016). Bioconductor Workflow for Microbiome Data Analysis: from raw reads to community analyses. F1000Research. 5. doi: 10.12688/f1000research
Chan K. G., Ng K. T., Pang Y. K., Chong T. M., Kamarulzaman A., Yin W. F., et al. (2015). Genome anatomy of streptococcus parasanguinis strain C1A, isolated from a patient with acute exacerbation of chronic obstructive pulmonary disease, reveals unusual genomic features. Genome Announc 3. doi: 10.1128/genomeA.00541-15
Chen Z., Wang Z., Ma H., Bao H., Jiang T., Yang T., et al. (2024). Immune cells mediated the causal relationship between the gut microbiota and lung cancer: a Mendelian randomization study. Front. Microbiol. 15, 1390722. doi: 10.3389/fmicb.2024.1390722
Chiu Y. C., Lee S. W., Liu C. W., Lin R. C., Huang Y. C., Lan T. Y., et al. (2021). Comprehensive profiling of the gut microbiota in patients with chronic obstructive pulmonary disease of varying severity. PloS One 16, e0249944. doi: 10.1371/journal.pone.0249944
den Besten G., Lange K., Havinga R., van Dijk T. H., Gerding A., van Eunen K., et al. (2013). Gut-derived short-chain fatty acids are vividly assimilated into host carbohydrates and lipids. Am. J. Physiol. Gastrointest Liver Physiol. 305, G900–G910. doi: 10.1152/ajpgi.00265.2013
Dicker A. J., Huang J. T. J., Lonergan M., Keir H. R., Fong C. J., Tan B., et al. (2021). The sputum microbiome, airway inflammation, and mortality in chronic obstructive pulmonary disease. J. Allergy Clin. Immunol. 147, 158–167. doi: 10.1016/j.jaci.2020.02.040
Ding K., Chen J., Zhan W., Zhang S., Chen Y., Long S., et al. (2021). Microbiome links cigarette smoke-induced chronic obstructive pulmonary disease and dietary fiber via the gut-lung axis: A narrative review. Copd. 19, 10–17. doi: 10.1080/15412555.2021.2019208
Douglas G. M., Maffei V. J., Zaneveld J. R., Yurgel S. N., Brown J. R., Taylor C. M., et al. (2020). PICRUSt2 for prediction of metagenome functions. Nat. Biotechnol. 38, 685–688. doi: 10.1038/s41587-020-0548-6
Finn R. D., Tate J., Mistry J., Coggill P. C., Sammut S. J., Hotz H.-R., et al. (2007). The Pfam protein families database. Nucleic Acids Res. 36, D281–D2D8. doi: 10.1093/nar/gkm960
Fromer L., Cooper C. B. (2008). A review of the GOLD guidelines for the diagnosis and treatment of patients with COPD. Int. J. Clin. Practice. 62, 1219–1236. doi: 10.1111/j.1742-1241.2008.01807.x
Hamidi B., Wallace K., Alekseyenko A. V. (2019). MODIMA, a method for multivariate omnibus distance mediation analysis, allows for integration of multivariate exposure-mediator-response relationships. Genes (Basel). 10. doi: 10.3390/genes10070524
Hankinson J. L., Odenkranz J. R., Fedan K. B. (1999). Spirometric reference values from a sample of the general US population. Am J Respir Crit Care Med 159 (1), 179–187. doi: 10.1164/ajrccm.159.1.9712108
Hanson C., Lyden E., Rennard S., Mannino D. M., Rutten E. P., Hopkins R., et al. (2016). The relationship between dietary fiber intake and lung function in the national health and nutrition examination surveys. Ann. Am. Thorac. Soc 13, 643–650. doi: 10.1513/AnnalsATS.201509-609OC
Hanson C., Rutten E. P., Wouters E. F., Rennard S. (2014). Influence of diet and obesity on COPD development and outcomes. Int. J. Chron. Obstruct. Pulmon. Dis. 9, 723–733. doi: 10.2147/COPD.S50111
Huang S., Chen J., Cui Z., Ma K., Wu D., Luo J., et al. (2023). Lachnospiraceae-derived butyrate mediates protection of high fermentable fiber against placental inflammation in gestational diabetes mellitus. Sci. Adv. 9, eadi7337. doi: 10.1126/sciadv.adi7337
Jang Y. O., Lee S. H., Choi J. J., Kim D. H., Choi J. M., Kang M. J., et al. (2020). Fecal microbial transplantation and a high fiber diet attenuates emphysema development by suppressing inflammation and apoptosis. Exp. Mol. Med. 52, 1128–1139. doi: 10.1038/s12276-020-0469-y
Kan H., Stevens J., Heiss G., Rose K. M., London S. J. (2008). Dietary fiber, lung function, and chronic obstructive pulmonary disease in the atherosclerosis risk in communities study. Am. J. Epidemiol. 167, 570–578. doi: 10.1093/aje/kwm343
Katoh K., Standley D. M. (2013). MAFFT multiple sequence alignment software version 7: improvements in performance and usability. Mol. Biol. Evol. 30, 772–780. doi: 10.1093/molbev/mst010
Kawashima S., Kinose D., Arima H., Kondo K., Yamazaki A., Uchida Y., et al. (2024). Association of gut microbiome with COPD in Japanese male residents: the SESSA study. ERJ Open Res. 10. doi: 10.1183/23120541.00788-2023
Kembel S. W., Cowan P. D., Helmus M. R., Cornwell W. K., Morlon H., Ackerly D. D., et al. (2010). Picante: R tools for integrating phylogenies and ecology. Bioinformatics. 26, 1463–1464. doi: 10.1093/bioinformatics/btq166
Kim Y. J., Lee J. Y., Lee J. J., Jeon S. M., Silwal P., Kim I. S., et al. (2022). Arginine-mediated gut microbiome remodeling promotes host pulmonary immune defense against nontuberculous mycobacterial infection. Gut Microbes 14, 2073132. doi: 10.1080/19490976.2022.2073132
Lai H. C., Lin T. L., Chen T. W., Kuo Y. L., Chang C. J., Wu T. R., et al. (2022). Gut microbiota modulates COPD pathogenesis: role of anti-inflammatory Parabacteroides goldsteinii lipopolysaccharide. Gut. 71, 309–321. doi: 10.1136/gutjnl-2020-322599
Lemoine S. C., Brigham E. P., Woo H., Hanson C. K., McCormack M. C., Koch A., et al. (2019). Omega-3 fatty acid intake and prevalent respiratory symptoms among U.S. adults with COPD. BMC Pulm Med. 19, 97. doi: 10.1186/s12890-019-0852-4
Leung J. M., Tiew P. Y., Mac Aogáin M., Budden K. F., Yong V. F., Thomas S. S., et al. (2017). The role of acute and chronic respiratory colonization and infections in the pathogenesis of COPD. Respirology. 22, 634–650. doi: 10.1111/resp.2017.22.issue-4
Li J., Chen Y., Shi Q., Sun J., Zhang C., Liu L. (2023). Omega-3 polyunsaturated fatty acids ameliorate PM2.5 exposure induced lung injury in mice through remodeling the gut microbiota and modulating the lung metabolism. Environ. Sci. pollut. Res. Int. 30, 40490–40506. doi: 10.1007/s11356-022-25111-0
Madapoosi S. S., Cruickshank-Quinn C., Opron K., Erb-Downward J. R., Begley L. A., Li G., et al. (2022). Lung microbiota and metabolites collectively associate with clinical outcomes in milder stage chronic obstructive pulmonary disease. Am. J. Respir. Crit. Care Med. 206, 427–439. doi: 10.1164/rccm.202110-2241OC
Martin B. D., Witten D., Willis A. D. (2020). Modeling microbial abundances and dysbiosis with beta-binomial regression. Ann. Appl. Stat. 14, 94–115. doi: 10.1214/19-AOAS1283
McMurdie P. J., Holmes S. (2013). phyloseq: an R package for reproducible interactive analysis and graphics of microbiome census data. PloS One 8, e61217. doi: 10.1371/journal.pone.0061217
Meijer K., de Vos P., Priebe M. G. (2010). Butyrate and other short-chain fatty acids as modulators of immunity: what relevance for health? Curr. Opin. Clin. Nutr. Metab. Care 13, 715–721. doi: 10.1097/MCO.0b013e32833eebe5
Michael B. S., Hongzhe L. (2019). Compositional mediation analysis for microbiome studies. Ann. Appl. Statistics. 13, 661–681. doi: 10.1214/18-AOAS1210
Miller M. R., Hankinson J., Brusasco V., Burgos F., Casaburi R., Coates A., et al. (2005). Standardisation of spirometry. Eur. Respir. J. 26, 319–338. doi: 10.1183/09031936.05.00034805
Moloney R. D., Desbonnet L., Clarke G., Dinan T. G., Cryan J. F. (2014). The microbiome: stress, health and disease. Mamm Genome 25, 49–74. doi: 10.1007/s00335-013-9488-5
Myhrstad M. C. W., Tunsjo H., Charnock C., Telle-Hansen V. H. (2020). Dietary fiber, gut microbiota, and metabolic regulation-current status in human randomized trials. Nutrients. 12. doi: 10.3390/nu12030859
Oksanen J. (2022). vegan: Community Ecology Package. R package version 2.5-7. Available at: https://CRAN.R-project.org/package=vegan
Organization WH. Available online at: https://www.who.int/data/gho/data/themes/mortality-and-global-health-estimates. (accessed September 03, 2024)
Prevention CfDCa Basics about COPD. Available online at: https://www.cdc.gov/copd/basics-about.html. (accessed September 03, 2024)
Prevention CfDCa (2022). Age-adjusted prevalence of COPD among US adults aged ≥ 18 years—2011–2020 cdc.gov2020. Available online at: https://www.cdc.gov/copd/data-and-statistics/national-trends.html#data-table-COPD_prevalence_trends.csv. (accessed September 03, 2024)
Prevention CfDCa (2023). Leading Causes of Death. Available online at: https://www.cdc.gov/nchs/fastats/leading-causes-of-death.htm. (accessed September 03, 2024)
Price M. N., Dehal P. S., Arkin A. P. (2009). FastTree: computing large minimum evolution trees with profiles instead of a distance matrix. Mol. Biol. Evol. 26, 1641–1650. doi: 10.1093/molbev/msp077
Qu L., Cheng Q., Wang Y., Mu H., Zhang Y. (2022). COPD and gut-lung axis: how microbiota and host inflammasome influence COPD and related therapeutics. Front. Microbiol. 13, 868086. doi: 10.3389/fmicb.2022.868086
Rohart F., Gautier B., Singh A., Lê Cao K.-A. (2017). mixOmics: An R package for ‘omics feature selection and multiple data integration. PloS Comput. Biol. 13, e1005752. doi: 10.1371/journal.pcbi.1005752
Saeed M. A., Gribben K. C., Alam M., Lyden E. R., Hanson C. K., LeVan T. D. (2020). Association of dietary fiber on asthma, respiratory symptoms, and inflammation in the adult national health and nutrition examination survey population. Ann. Am. Thorac. Soc 17, 1062–1068. doi: 10.1513/AnnalsATS.201910-776OC
Scoditti E., Massaro M., Garbarino S., Toraldo D. M. (2019). Role of diet in chronic obstructive pulmonary disease prevention and treatment. Nutrients. 11, 1357. doi: 10.3390/nu11061357
Services USDoHaH (2014). National Center for Chronic Disease Prevention and Health Promotion (US) Office on Smoking and Health. The health consequences of smoking—50 years of progress: A report of the surgeon general. (U.S. Department of Health and Human Services: Centers for Disease Control and Prevention, Atlanta).
Sim Y. S., Lee J. H., Lee E. G., Choi J. Y., Lee C. H., An T. J., et al. (2022). COPD exacerbation-related pathogens and previous COPD treatment. J. Clin. Med. 12. doi: 10.3390/jcm12010111
So D., Whelan K., Rossi M., Morrison M., Holtmann G., Kelly J. T., et al. (2018). Dietary fiber intervention on gut microbiota composition in healthy adults: a systematic review and meta-analysis. Am. J. Clin. Nutr. 107, 965–983. doi: 10.1093/ajcn/nqy041
Sorli-Aguilar M., Martin-Lujan F., Flores-Mateo G., Arija-Val V., Basora-Gallisa J., Sola-Alberich R. (2016). Dietary patterns are associated with lung function among Spanish smokers without respiratory disease. BMC Pulm Med. 16, 162. doi: 10.1186/s12890-016-0326-x
Trompette A., Gollwitzer E. S., Yadava K., Sichelstiel A. K., Sprenger N., Ngom-Bru C., et al. (2014). Gut microbiota metabolism of dietary fiber influences allergic airway disease and hematopoiesis. Nat. Med. 20, 159–166. doi: 10.1038/nm.3444
Varraso R., Fung T. T., Barr R. G., Hu F. B., Willett W., Camargo C. A. Jr. (2007a). Prospective study of dietary patterns and chronic obstructive pulmonary disease among US women. Am. J. Clin. Nutr. 86, 488–495. doi: 10.1093/ajcn/86.2.488
Varraso R., Fung T. T., Hu F. B., Willett W., Camargo C. A. (2007b). Prospective study of dietary patterns and chronic obstructive pulmonary disease among US men. Thorax. 62, 786–791. doi: 10.1136/thx.2006.074534
Varraso R., Willett W. C., Camargo C. A. Jr. (2010). Prospective study of dietary fiber and risk of chronic obstructive pulmonary disease among US women and men. Am. J. Epidemiol. 171, 776–784. doi: 10.1093/aje/kwp455
Wagenaar C. A., van de Put M., Bisschops M., Walrabenstein W., de Jonge C. S., Herrema H., et al. (2021). The effect of dietary interventions on chronic inflammatory diseases in relation to the microbiome: A systematic review. Nutrients 13. doi: 10.3390/nu13093208
Wang Q., Garrity G. M., Tiedje J. M., Cole J. R. (2007). Naïve Bayesian Classifier for Rapid Assignment of rRNA Sequences into the New Bacterial Taxonomy. Appl. Environ. Microbiol. 73, 5261–5267. doi: 10.1128/AEM.00062-07
Wang S., Yang L., Hu H., Lv L., Ji Z., Zhao Y., et al. (2022). Characteristic gut microbiota and metabolic changes in patients with pulmonary tuberculosis. Microb. Biotechnol. 15, 262–275. doi: 10.1111/1751-7915.13761
Watson H., Mitra S., Croden F. C., Taylor M., Wood H. M., Perry S. L., et al. (2018). A randomised trial of the effect of omega-3 polyunsaturated fatty acid supplements on the human intestinal microbiota. Gut. 67, 1974–1983. doi: 10.1136/gutjnl-2017-314968
Young R. P., Hopkins R. J. (2018). Is the “Western diet” a new smoking gun for chronic obstructive pulmonary disease? Am. Thorac. Society; 15 (6), 662–663. doi: 10.1513/AnnalsATS.201802-131ED
Young R. P., Hopkins R. J., Hanson C. (2016). Connecting dietary fiber directly with good lung health. Ann. Am. Thorac. Soc 13, 1869–1870.
Zhao S., Lau R., Zhong Y., Chen M. H. (2024). Lactate cross-feeding between Bifidobacterium species and Megasphaera indica contributes to butyrate formation in the human colonic environment. Appl. Environ. Microbiol. 90, e0101923. doi: 10.1128/aem.01019-23
Keywords: COPD, diet, fiber, lungs, microbiome, omega-3 fatty acid
Citation: Qiu H, Checketts R, Jackson MK, Riethoven J-JM, Hansel NN, Bailey KL, Hanson C and Samuelson DR (2024) Diet-microbiome interactions influence lung function in chronic obstructive pulmonary disease. Front. Microbiomes 3:1426150. doi: 10.3389/frmbi.2024.1426150
Received: 30 April 2024; Accepted: 25 September 2024;
Published: 22 October 2024.
Edited by:
David Rios-Covian, MicroViable Therapeutics SL, SpainReviewed by:
Jean Debédat, University of California, Davis, United StatesCopyright © 2024 Qiu, Checketts, Jackson, Riethoven, Hansel, Bailey, Hanson and Samuelson. This is an open-access article distributed under the terms of the Creative Commons Attribution License (CC BY). The use, distribution or reproduction in other forums is permitted, provided the original author(s) and the copyright owner(s) are credited and that the original publication in this journal is cited, in accordance with accepted academic practice. No use, distribution or reproduction is permitted which does not comply with these terms.
*Correspondence: Derrick R. Samuelson, ZGVycmljay5zYW11ZWxzb25AdW5tYy5lZHU=
†These authors share first authorship
Disclaimer: All claims expressed in this article are solely those of the authors and do not necessarily represent those of their affiliated organizations, or those of the publisher, the editors and the reviewers. Any product that may be evaluated in this article or claim that may be made by its manufacturer is not guaranteed or endorsed by the publisher.
Research integrity at Frontiers
Learn more about the work of our research integrity team to safeguard the quality of each article we publish.