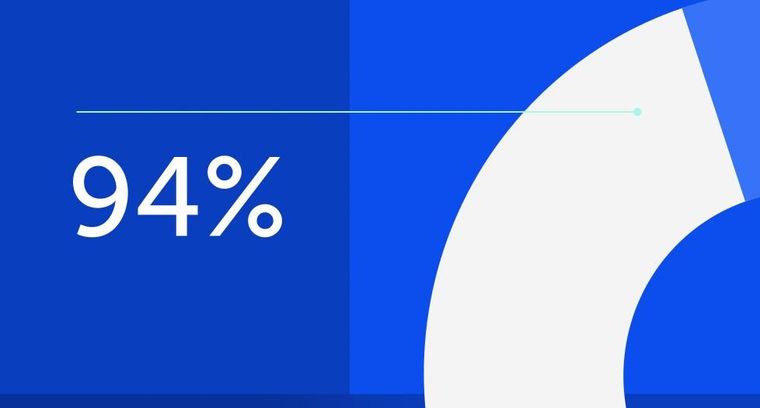
94% of researchers rate our articles as excellent or good
Learn more about the work of our research integrity team to safeguard the quality of each article we publish.
Find out more
REVIEW article
Front. Microbiol., 13 February 2025
Sec. Antimicrobials, Resistance and Chemotherapy
Volume 16 - 2025 | https://doi.org/10.3389/fmicb.2025.1536131
Developing new antibiotics poses a significant challenge in the fight against antimicrobial resistance (AMR), a critical global health threat responsible for approximately 5 million deaths annually. Finding new classes of antibiotics that are safe, have acceptable pharmacokinetic properties, and are appropriately active against pathogens is a lengthy and expensive process. Therefore, high-throughput platforms are needed to screen large libraries of synthetic and natural compounds. In this review, we present bacterial cytological profiling (BCP) as a rapid, scalable, and cost-effective method for identifying antibiotic mechanisms of action. Notably, BCP has proven its potential in drug discovery, demonstrated by the identification of the cellular target of spirohexenolide A against methicillin-resistant Staphylococcus aureus. We present the application of BCP for different bacterial organisms and different classes of antibiotics and discuss BCP’s advantages, limitations, and potential improvements. Furthermore, we highlight the studies that have utilized BCP to investigate pathogens listed in the Bacterial Priority Pathogens List 2024 and we identify the pathogens whose cytological profiles are missing. We also explore the most recent artificial intelligence and deep learning techniques that could enhance the analysis of data generated by BCP, potentially advancing our understanding of antibiotic resistance mechanisms and the discovery of novel druggable pathways.
The World Health Organization (WHO) has declared antimicrobial resistance (AMR) as one of the most severe global health threats facing humanity. AMR is the ability of a microbe to survive and grow in the presence of a chemical thought to prevent this effectively. It has been estimated that in 2019 alone, antimicrobial resistance killed at least 1.27 million people globally, more deaths than HIV/AIDS or malaria, with 4.95 million deaths associated with AMR (Murray et al., 2022). According to the Centers for Disease Control and Prevention’s Antibiotic Resistance Threats Report (Centers for Disease Control and Prevention (U.S.), 2019), in the United States, over 2.8 million antibiotic-resistant infections appear every year, leading to over 35,000 deaths. Furthermore, AMR has been predicted to lead to a total loss of up to $100 trillion for the global economy by 2050 (O’Neill, 2016). These alarming statistics underscore the urgent need to develop effective therapeutics to combat antimicrobial resistance.
The efforts undertaken in the field of AMR until now have not been enough despite the enormous research effort and inventive therapeutic approaches. Since 1940, antimicrobials have been used widely (Gardner, 1940; Gardner, 1945) and beyond treating infections, antibiotics enabled many modern medical procedures, such as open-heart surgeries, organ transplants, and cancer therapies (Hutchings et al., 2019). Even before 1940 and for about 60 years after, most antibiotics were discovered by culturing microbial samples from soil for compounds already expressed by microbes (Walsh, 2000; Hutchings et al., 2019). However, over the last 20 years, the lipopeptides and the oxazolidinones have been the only two new antibiotic classes created and have been effective only against Gram-positive bacteria (Luepke et al., 2017). The last novel antibiotic class introduced to kill Gram-negative bacteria was the quinolones when nalidixic acid was synthesized in 1962 (Tacconelli et al., 2018). Although recent developments have shown potential against Gram-negative bacteria, such as Zosurabalpin, a new antibiotic that disrupts bacterial lipopolysaccharide (LPS) transport from the inner membrane to the outer membrane (Zampaloni et al., 2024), further advancements in antibiotic discovery are needed. To facilitate the discovery of novel druggable pathways, new high-throughput screens based on Bacterial Cytological Profiling have been developed.
This review emphasizes the use of bacterial cytological profiling (BCP) as a highly effective method for discovering novel antibiotics and rapidly identifying antibiotic targets in a cost-effective manner. BCP initially creates a library that captures the overall profile of bacterial morphological and physiological changes at a single-cell level induced by antibiotics with known mechanisms of action. This profile includes details on bacterial cell shapes and sizes, fluorescent intensities and spatial distribution of DNA, and fluorescent distribution of membrane dyes (Nonejuie et al., 2013; Quach et al., 2016; Samernate et al., 2023). The library is then utilized to classify existing antibiotics based on the specific components of bacterial cells they target and to discover new antibiotics. In this review, we also highlight how BCP is used to expand our quantitative understanding of antibiotic pharmacodynamics and bacterial stress responses, as well as how BCP enhances the development of non-traditional antibacterial strategies such as phage therapies (Deep et al., 2024; Tsunemoto et al., 2023; Birkholz et al., 2024; Thammatinna et al., 2020; Soonthonsrima et al., 2023; Naknaen et al., 2023; Naknaen et al., 2024).
AMR arises from either genetic alterations or phenotypic changes in pathogens (Corona and Martinez, 2013; Davies and Davies, 2010; Jago et al., 2025). To effectively tackle antibiotic-resistant bacteria, it is essential to understand how antibiotics work, which is known as their mechanism of action (MOA), see Table 1. Understanding MOA involves studying how antibiotics affect bacterial physiology and molecular interaction with bacterial targets (Figure 1). However, identifying the MOA presents a significant limitation in drug discovery. In some cases, the exact MOA is determined years after a drug’s approval, as seen with daptomycin (Grein et al., 2020).
Figure 1. Antibiotic targets in bacteria. Antibiotics typically kill bacteria by targeting at least one of the five major cell components: cell wall, cell membrane, ribosomes, DNA, and RNA. Antibiotics interfere with the synthesis of or the direct damage of cellular structures or components resulting in the inhibition of bacterial growth or irreversible reduction of bacterial cell integrity. Some antibiotics inhibit the synthesis of essential cell components, such as folate synthesis, a precursor for DNA synthesis. Additionally, bacteria have evolved mechanisms such as efflux pumps, which actively transport antibiotics out from the cell, reducing their efficacy and contributing to antibiotic resistance. Figure is created using BioRender.
Table 1. *General classification of antibiotics based on their target and chemical structure, including their mechanism of action.
Traditionally, the pathway inhibited by a compound has been identified mainly through macromolecular synthesis (MMS) assays. These assays use radioactively labeled precursors for peptidoglycan, lipid, protein, RNA, or DNA synthesis (Cotsonas King and Wu, 2009), enabling the identification of whether one or more pathways are targeted. However, compounds that act on different stages of the same pathway cannot be identified. Despite being an important technique, MMS assays are limited by low accuracy, low resolution, low throughput and time-consuming (Nonejuie et al., 2013).
To address the limitations associated with MMS assays, diverse alternative techniques to identify the MOA have been developed (Silver, 2011; Da Cunha et al., 2021). These include biochemical approaches, that can start with molecular docking—a computational technique used to predict how a small molecule, such as an antibiotic, binds to its target (Fan et al., 2019). A complementary approach is affinity chromatography, which identifies direct biophysical interactions between antimicrobials and their targets where the antibiotic interacts with protein from whole-cell extracts (Hudson and Lockless, 2022; Hage et al., 2012; Franken et al., 2015). However, this requires a large amount of the test compound, which is often unavailable, particularly during the early stages of drug discovery (Nonejuie et al., 2013).
Identification of the molecular target can be achieved by employing genetic approaches (Cacace et al., 2017), such as resistance selection (Hudson and Lockless, 2022), testing specifically designed indicator strains or genetically modified mutant strains (Freiberg et al., 2005; Zlitni et al., 2013; Donald et al., 2009) and using pattern recognition techniques based on metabolomics (Vincent et al., 2016; Ribeiro da Cunha et al., 2020), such as Nuclear Magnetic Resonance Spectroscopy (Dörries et al., 2014; Hoerr et al., 2016) or Mass Spectrometry (Zampieri et al., 2018), as well as transcriptomics (Boshoff et al., 2004) through methods like hybridization assays (Boshoff et al., 2004) or next-generation sequencing (O’Rourke et al., 2020) and proteomics (Bandow and Hecker, 2007; Bandow et al., 2003). These approaches could be used independently or in conjunction with BCP to provide insights into antibiotic targets.
High-throughput screening platforms have been compared (Zampieri et al., 2018; Ayon, 2023) including some alternative discovery strategies (Quinn and Dyson, 2024). While these additional methods offer several benefits, they face significant limitations, particularly their time-consuming nature, which impacts their overall effectiveness. High cost and technical expertise are shared drawbacks of metabolomics, proteomics, and transcriptomics, as these methods rely on sophisticated equipment and complex data analysis. Similarly, transcriptional profiling and genetic approaches share the limitation of being unable to directly pinpoint molecular targets (Nonejuie et al., 2013). Apart from all limitations in determining MOA, discovering novel compounds that are active against Gram-positive and Gram-negative bacteria remains challenging.
The process of discovering and developing new classes of antibiotics is particularly challenging, as they must exhibit acceptable pharmacokinetic properties, demonstrate safety, and efficacy (Tacconelli et al., 2018). Moreover, producing antibiotics offers limited profit margins due to the high production costs and the extended process of research, testing, and approval (Tacconelli et al., 2018). Therefore, new, high-throughput screening platforms are needed for the fast and inexpensive screening of large libraries of synthetic and natural compounds that are highly effective against human pathogens (Lewis, 2013; Lewis et al., 2024). The following section reviews the quick and scalable bacterial cytological profiling methods (BCPs) and discusses their availability for some of the most important human pathogens as outlined in the latest WHO 2024 report.
In 2013, Poochit et al. designed Bacterial Cytological Profiling (BCP) analysis for E. coli cells using different classes of antibiotics (Nonejuie et al., 2013) (Figure 2). BCP data are obtained using fluorescent microscopy of E. coli cells stained with fluorescent membrane and DNA dyes as well as fluorescent reporter for membrane permeability. Using image analysis software, various bacterial cell parameters, such as cell length, width, solidity, and DNA content, are extracted (Nonejuie et al., 2013). Subsequently, complex multidimensional data are analyzed using the Principal Component Analysis (PCA) technique, to cluster cells based on their cytological profile to identify the MOA of known and unknown antibacterial compounds (Figure 2; Box 1).
Figure 2. Bacterial cytological profiling workflow. (A) Bacterial culture preparation: cells are treated with antibiotics at specific concentrations. After incubation, cells are stained with fluorescent dyes targeting cellular components, such as the membrane and DNA. (B) Microscopy slide preparation. (C) Fluorescence microscopy is used to image antibiotic-treated cells. (D) Bacterial segmentation and image analysis, the resulting micrographs are processed using image analysis software to extract quantitative cytological data. (E) Extracted data is traditionally analyzed using Principal Component Analysis (PCA), see Box 1, to identify phenotypic changes, providing insights into the antibiotics’ mechanism of action (MOA). Figure is created using BioRender.
BOX 1. Principal Component Analysis (PCA).
PCA is a popular statistical technique commonly used for identifying linear relationships in complex data, such as those generated in BCP, by identifying the fewest variables which contribute the most to variance in the data (Bailey, 2012). PCA creates a linear combination of the original variables to create a new set of principal components (Bailey, 2012). Firstly, PCA starts by calculating a matrix showing the relation each variable has for all others. Then, it finds their eigenvectors (direction of the relationship) and eigenvalues (contribution of relationship to variance). In PCA, the principal components with the most contribution to variance in the data are then plotted. PCA has diverse applications across almost all scientific fields, including biology, medicine, computer science, and geology. In the context of biomedical research, PCA has been utilized to analyze the human cell atlas and prostate cancer risk prediction (van et al., 2014; Regev et al., 2017). Whilst PCA captures linear relationships between variables exclusively, it has been the primary method for dimensional reduction in Bacterial Cytological Profiling (Figure 3B). There are also recent popular approaches capturing non-linear relationships such as UMAP (McInnes et al., 2020) (primarily local differences), or PaCMAP (Wang et al., 2021) (local and global relationships) which may provide other insights into local differences and non-linear relationships within and between antibiotic treatments. Additionally, plots of dimensionally reduced provide insightful comparisons between different treatments, however, identifying distinct profiles is not equivalent to identifying novel targets; a drug could have multiple known MOAs for example and still display a distinct profile (Samernate et al., 2023; Martin et al., 2020). Even so, there have been possible connections found between various BCP profiles and novel targets using PaCMAP (Takebayashi et al., 2024).
Since cytological profiling produces data at a single-cell level (Samernate et al., 2023), it uses morphological data such as bacterial chromosomal condensation, or cell shape changes in response to antimicrobials to differentiate between different targeted metabolic pathways (Nonejuie et al., 2013) (Figure 3). Furthermore, this approach can lead to the identification of antibiotics that are effective against multidrug-resistant bacteria (Quach et al., 2016).
Figure 3. Bacterial cytological profiling. (A) Antibiotics that target different bacterial cell components induce different cell morphologies. The drawings are based on the microscopy images from Nonejuie et al. (2013), where bacterial cells were treated with antibiotics targeting five major biosynthetic pathways (DNA, Ribosome, RNA, Cell Wall, Membrane), using fluorescent dyes FM4-64 (red) and DAPI (blue) to stain bacterial membranes and DNA, respectively. Scale bar, 1 μm. (B) Principal Component Analysis (PCA) is used to cluster different bacterial cell shapes based on the antibiotic MOA, allowing profiles to be distinguished qualitatively. Each point on the graph represents a single cell, with clusters forming distinct groups according to cell morphology induced by different antibiotic treatments. For example, green dots represent cells treated with a DNA-targeting antibiotic, but we can see that some green dots cluster with orange dots (untreated bacteria), this suggests no significant morphological changes, indicating potential antibiotic resistance or persistence (Balaban et al., 2019; Kussell et al., 2005). PCA highlights MOA differences by visualizing the morphological variability induced by different antibiotic treatments.
BCP takes advantage of the limited presence of cell-cycle checkpoints in bacteria (Nonejuie et al., 2013). When stressed by antibiotics, bacteria show phenotypical changes that are characteristic of the antibiotic target. For example, compounds that target the ribosome by stopping protein synthesis (e.g., tetracycline and chloramphenicol) produce circular chromosomes and wide cells (Nonejuie et al., 2013; Wu et al., 2019) (Figure 3).
During antibacterial treatment, rod-shaped bacteria (bacilli) can shrink and take on an oval form, known as ovoid cells (Spratt, 1975; Spratt and Pardee, 1975). There are no clearly defined names for these cells, however, as they have been referred to in literature as ‘round forms’ (Curtis et al., 1979; di et al., 1994); ‘round cells’ (Bernabeu-Wittel et al., 2004; Jackson and Kropp, 1996; de Pedro et al., 2001); ‘spherical forms’; ‘spherical cells’ (Sumita et al., 1990; Dalhoff et al., 2003; Horii et al., 1998); or ‘coccoid forms’ (Perumalsamy et al., 2013; Nickerson and Webb, 1956). Filamentation, or cell elongation, occurs when rod-shaped bacteria (or sometimes cocci) synthesize peptidoglycan for their side walls but not for their division walls, leading to abnormally elongated cells (Cushnie et al., 2016). This process results from the inhibition of septal peptidoglycan synthesis (Spratt, 1975). Filamentous cells can be also induced when DNA synthesis is inhibited (Elliott et al., 1987; Chen et al., 2005) or DNA is damaged (Uphoff et al., 2013; Jones and Uphoff, 2021; Jaramillo-Riveri et al., 2022) by a process known as the SOS response that inhibits cell division (Ojkic et al., 2020) (Figure 3).
Antibiotic treatments can drastically alter bacterial cell size, induce localized swelling, bulge formation, blebbing, and thicken peptidoglycan (Cushnie et al., 2016). Occasionally, antibiotic-treated cells can lose cell walls, turning bacterial cells into spheroplasts and protoplasts. Spheroplast are Gram-negative bacteria that lost their peptidoglycan layer, but kept their outer membrane, whereas protoplasts are formed from Gram-positive bacteria that lack the peptidoglycan layer (Gebicki and James, 1960; Ojkic et al., 2014). Bacterial variants that completely lack a cell wall, encompassing both Gram-negative and Gram-positive bacteria, are also known as L-forms (Errington, 2013; Allan et al., 2009; Mercier et al., 2014; Errington, 2017).
Phenotypical changes derived from the antibiotic-induced changes in bacterial subcellular architecture could confer an increase in fitness to bacteria in the presence of antibiotics (Banerjee et al., 2021). Resistance to antibiotics usually takes the form of reducing the concentration of intracellular antibiotic or by reducing the binding affinities of the cellular targets to the antibiotic (Ojkic et al., 2022; Baquero et al., 2023). By using available BCP data, recent studies have shown that by reducing the surface-to-volume ratio (S/V), bacteria can effectively reduce the antibiotic concentration inside a cell, thereby promoting cell growth by decreasing antibiotic influx (Ojkic et al., 2022). Similarly, an increase in S/V can benefit the cell in alternative ways such as increasing the antibiotic efflux rate or the rate of nutrient uptake (Ojkic et al., 2022; Ojkic et al., 2019; Ojkic and Banerjee, 2021). These studies explain how cell shape transformations promote bacterial survival under antibiotic treatments—pointing toward potential new druggable targets that control cell shape and size under stress.
BCP has been successfully employed to study the MOA of various antibacterial agents, including azithromycin (Lin et al., 2015), diphenylureas (Mohammad et al., 2017) and thailandamide (Wu and Seyedsayamdost, 2018). It has also been used to identify the cellular pathways targeted by anticancer metal complexes (Sun et al., 2018) and to study the response of bacteria to antibiotics in different growth conditions (Dillon et al., 2020). Additionally, BCP has been used to identify the cellular pathways targeted by antibacterial molecules affecting different cellular pathways (Araújo-Bazán et al., 2016; Andreu et al., 2022), making it a valuable tool not only for determining antibacterial targets but also to potentially identify novel MOA, i.e., ones that target new proteins or new pathways (Figure 4).
Figure 4. Representation of Principal Component Analysis (PCA) using bacterial morphologies to determine the MOA of a novel antibiotic. Arrow 1 indicates the antibiotic used against bacteria studied, which can change their shape depending on the antibiotic’s MOA. For example, if the bacteria exhibit a morphology as indicated by arrow 2, the antibiotic likely targets the membrane. Conversely, if the bacteria display a morphology as indicated by arrow 3, the antibiotic likely targets RNA. However, if the morphology is completely different from the known and clustered morphologies, as shown by arrow 4, it suggests that the antibiotic targets a novel pathway but lastly, if the bacteria do not show any change, it suggests that they are not susceptible to the tested antibiotic or, in the worst-case scenario, that they are resistant to said antibiotic.
BCP can also be used to determine the MOA of treatments beyond antibiotics, such as phage therapies, revealing how phages disrupt essential cellular pathways (Deep et al., 2024; Tsunemoto et al., 2023; Birkholz et al., 2024; Thammatinna et al., 2020; Soonthonsrima et al., 2023; Naknaen et al., 2023; Naknaen et al., 2024). BCP allows the visualization of bacterial chromosomal condensation, cell shape and overall cellular morphology changes within bacterial cells during phage infection. These changes not only reveal the pathways and cellular targets phages use to propagate their lifecycle but also highlight the role of bacterial defense mechanisms in combating phage infection (Tsunemoto et al., 2023; Thammatinna et al., 2020; Naknaen et al., 2024). BCP has demonstrated how the overexpression of phage-related proteins can induce specific phenotypic changes as a result of the activation of a bacterial defense system to suppress phage propagation (Deep et al., 2024). Additionally, BCP has been fundamental in assessing the impact of different antibiotics on phage replication, revealing that certain antibiotics can synergize with phages to enhance bacterial cell lysis. In contrast, others inhibit phage propagation by disrupting essential bacterial processes (Tsunemoto et al., 2023). This dual capability of BCP to show both the direct effects of phage infection and the influence of external agents such as antibiotics, makes it a high-throughput tool in studying phage-bacteria dynamics.
Most importantly, BCP has been successfully used to study some of the most important human pathogens from the WHO Bacterial Priority Pathogens List (Table 2). In 2017, the first Bacterial Priority Pathogen list was created by the WHO in collaboration with researchers from the Division of Infectious Diseases at the University of Tübingen, Germany which used a multicriteria analysis technique to inform research and development (R&D) for future antibacterial compounds (World Health Organization, 2017). Now, 7 years after the introduction of the list there have been novel antibiotics put onto the market either with effectiveness in vivo or in vitro against pathogens deemed critical priority, but unfortunately, resistance has been found in almost every one (di et al., 2021; Butler et al., 2022). This year the WHO updated this list to tackle new developments in antimicrobial resistance to give an updated and directions for policy makers and insight on future developments. The new Bacterial Priority Pathogens List 2024 includes 15 resistant pathogens, ordered at various levels of priority from medium; high; to critical (WHO Bacterial Priority Pathogens List, 2024) (Table 2). Out of 15 pathogen groups, bacterial cytological profiling is not available for 30% of them: Non-typhoidal Salmonella, Neisseria gonorrhoeae, Group A and B Streptococci, Haemophilus influenzae. Therefore, urgent BCPs regarding these severe pathogenic organisms are needed.
Table 2. BCP analysis studies checklist from the WHO Bacterial Priority Pathogens List (2024) separated by priority from 1 to 3.
BCP is used to scan new antibacterial components to identify their specific targets (Figure 4). As demonstrated, BCP effectively differentiates between various morphological changes induced by different antibiotics, thereby providing insights into the antibiotic’s MOA. If a novel antibiotic places bacteria in a distinct region of the PCA plot compared to known antibiotic targets, it could indicate a new pathway target or MOA previously uncharacterized (Figure 4). For example, if the PCA analysis shows that the morphology of bacteria treated with a new antibiotic clusters in a region associated with membrane or RNA targets (Arrows 2 and 3 in Figure 4), it directly indicates the antibiotic’s mode of action. Conversely, if the antibiotic’s effect causes a morphology change that places bacteria in a novel zone, as illustrated with Arrow 4, it may suggest the discovery of a new antibacterial pathway or target.
Together, BCP significantly enhances drug development by offering a precise, fast and systematic method for characterizing the effects of new antibacterial agents. Its ability to identify target-specific morphological changes provides a comprehensive tool for uncovering novel antibiotic targets and advancing our understanding of bacterial physiology.
Fluorescent microscopy has allowed us to visualize many cell components in great clarity, however, quantitative image analysis of both cellular and sub-cellular structures has been a continual challenge (Mistretta et al., 2024; Ma et al., 2024). The requirement for accurate tools is highlighted by the scale of the bacterial objects, with typically 100–300 pixels per typical E. coli cell (Cutler et al., 2022), while antibiotic-treated cells could have an order of magnitude larger sizes (Nonejuie et al., 2013). In addition to variations in size, bacteria exhibit a diverse array of shapes (Young, 2006). While most studied bacteria in BCP (see Tables 3, 4) are rod-shaped or spheroid, there is growing interest in bacteria with more complex shapes that emerge after exposure to antibiotics, such as seen in Caulobacter crescentus (Banerjee et al., 2021).
Table 3. Studies with bacterial profiles following the original BCP method, that being the use of segmentation, feature extraction, and dimensional reduction techniques to create a plot allowing the viewer to differentiate between different antibiotic treatments on the same bacterial cell strains.
Table 4. Studies using protocols similar to the original BCP method by merit of developing a profile or differentiating between bacterial cells which exhibit different phenotypes.
To precisely define cell boundaries and to segment cellular components, sub-pixel segmentation methods are required (Cutler et al., 2022; Bali and Singh, 2015). Classical image segmentation techniques have been used since the 1960s (Prewitt and Mendelsohn, 1966; Mendelsohn et al., 1965), laying the groundwork for the more advanced artificial intelligence methods used nowadays. Many segmentation solutions are currently available as user-friendly plugins such as MicrobeJ (Ducret et al., 2016) within easily accessible platforms such as ImageJ (Ma et al., 2024; Stringer et al., 2021; Sousa, 2020). MicrobJ is based on the classical segmentation method, but since December 2024, MicrobJ has implemented the deep neural network segmentation algorithm Omnipose. Stand-alone image analysis programs are also available but these can at times be less supported and less accessible (Paintdakhi et al., 2016).
In 2016 SuperSegger was created to improve upon flaws in segmentation through thresholding in bacterial phase-contrast images and it combines classical segmentation with Deep learning (Stylianidou et al., 2016). To correct common errors in segmentation from both the thresholding and watershed, SuperSegger uses a shallow neural network trained from the segmentation data (Chai et al., 2023). Recently, Deep neural networks (DNNs) which have now become the backbone of most Deep learning segmentation methods are now widely recognized as superior tools for cell segmentation (Jan et al., 2024). As showcased in Table 3, Deep learning is significantly underutilized in original BCP studies. However, some recent BCP-like profiles have been created using deep learning for segmentation and profiling (Zagajewski et al., 2023), and object detection (Spahn et al., 2022) for antibiotic susceptibility analysis (Table 4).
Among recent and easily available Deep learning segmentation algorithms, Pachitariu et al. demonstrated that Cellpose outperformed the popular programs Mask R-CNN and StarDist when applied to a varied dataset of different cell types and cell-like-objects, showcasing it as a powerful general solution for cell segmentation (Stringer et al., 2021). Cutler et al. assessed the performance of (at the time) state-of-the-art cell segmentation algorithms on a wide array of bioimages of bacteria with different morphologies. This led to the design of the algorithm, Omnipose, which outperformed all segmentation algorithms tested across a varied dataset of bacterial cell sizes, shapes, and optical characteristics and as such has been used extensively in research (Cutler et al., 2022). Recent benchmarks for bacterial segmentation algorithms have found that transformer based algorithms currently outperform other deep learning models such as CNNs, and classical segmentation techniques on a multitude of cell types when well implemented on a large datasets (Ma et al., 2024). The major advantages these algorithms have over their competing peers are threefold: They have a larger model capacity, allowing them to train for a more complex task than a model with lower capacity; are able to find patterns over the whole image due to self-attention mechanisms when CNNs are only able to do so in smaller regions of the image (Vaswani et al., 2023); less annotation is needed than competing algorithms for large datasets (Ma et al., 2024) as the model can pretrain using transfer-learning and fine tune with more limited annotations. The top three winning algorithms of the multimodality cell segmentation challenge have however not been used for BCP despite their recent inclusion in the widely accessible program, NAPARI (Sofroniew et al., 2025).
Segmented data availability (Tables 3, 4) is invaluable for scientific communities and accelerates new findings by increasing the reproducibility of datasets and enabling future meta-analyses. By using published BCP data and mathematical modeling, the researchers uncovered the robustness of scaling behavior between cell surface area and volume in E. coli (Ojkic et al., 2019) and B. subtilis (Ojkic and Banerjee, 2021), inferred cell physiological alterations upon antibiotic treatments (Cylke et al., 2022), and proposed a new antibiotic resistance pathway mediated by cell surface-to-volume ratio (S/V) transformations (Ojkic et al., 2022). Therefore, the availability of BCP data is good practice and should be considered as a benchmark for all future BCP platforms, especially for pathogenic bacteria (Table 2).
Even with all the advantages we mentioned about BCP, it has certain limitations. BCP can identify the general target of an antibiotic, but it cannot provide precise information about the exact site within the target that is affected. For instance, while BCP can indicate that an antibiotic targets the ribosome, it cannot specify which part of the ribosome is involved. Other high-throughput methods, such as affinity chromatography and omics-based approaches, can predict cellular targets more accurately by providing detailed insights into specific molecular interactions or pathway alterations. Combining these techniques with BCP could help overcome BCP’s limitation in pinpointing the exact stage of pathway inhibition.
BCP requires staining dyes to evaluate DNA content and cell size and shape, with fluorescent dye intensity being essential for determining the antibiotic MOA. A wide variety of dyes, protein fusions, and reporter strains have been used in BCP, facilitating both fast MOA detection, and discovery of new MOAs. However, despite the abundance of possible dyes, strains, and assays selecting the most appropriate ones for specific phenotypic experiments remains challenging, as more information is needed to understand cellular functions (Table 3). This is exacerbated by the complexity of bacterial physiology with many processes being overlapped by mechanisms such as metabolic flux or co-dependent regulation (Schäfer et al., 2024; Serbanescu et al., 2022). While numerous cytological profiles have been reported, neither antibiotic concentration nor time of antibiotic exposure has been standardized. Additionally, scaling BCP in low-resource settings may be challenging, particularly due to the reliance on specialized microscopes and fluorescent dyes.
As shown in Tables 3, 4, a variety of fluorescent dyes have been used to investigate the cytological profiles of different bacterial organisms. However, newly developed dyes have the potential to provide more detailed information that could help in building a more comprehensive response profile. Despite the prevalence of cell wall targeting antibiotics in BCP experiments, direct methods for visualizing cell wall synthesis and remodeling during antibiotic exposure have been lacking. In 2012, Kuru et al. discovered a groundbreaking method for bacterial cell wall staining using fluorescent amino acids (Kuru et al., 2012). The cell wall provides the shape and structural integrity of the cell. It is made of peptidoglycan (PG), which consists of glycan strands cross-linked by D-amino acid (DAA) (Vollmer et al., 2008). The team introduced HADA and NADA, two fluorescent D-amino acids (FDAAs) attached to a D-amino acid backbone (3-amino-d-alanine). This chemical biology approach aims to detect and visualize the exact location and amount of new peptidoglycan layer synthesis in bacteria. By using HADA or NADA as a fluorescent peptidoglycan label during cell wall synthesis, the technique also allows researchers to observe morphological changes in bacteria over time. This is relevant as HADA and NADA can be implemented in the methodology of BCP to investigate the growth modes of bacteria under antibiotic exposure as they exhibit a diverse growth pattern—that could confer selective advantages in their environments (Young, 2006; Ojkic et al., 2022).
Other fluorescent dyes are available to quantitatively probe bacterial physiological states: ThT and DiBAC4 for bacterial membrane potential (Stratford et al., 2019; Prindle et al., 2015; De Souza-Guerreiro et al., 2024; Wong et al., 2021), carboxy-H2DCFDA for reactive oxygen species (ROS) (Wong et al., 2021), and DAF-FM for reactive nitrogen species (RNS) (Wong et al., 2021). By integrating membrane potential, ROS, and RNS into cytological profiles could provide additional information regarding bacterial physiology and bacterial stress response during antibiotic treatment. However, adding additional dyes could be a challenge in low resource settings. With additional dyes also comes increasing potential problems with fluorescence crosstalk. Fortunately, there have been recent advances in AI image analysis which could circumvent this problem by predicting and generating overlays of fluorescent dyes onto unlabeled cells (Goldsborough et al., 2017; Osokin et al., 2017).
Despite significant advances in research and the development of new tools, combating antimicrobial resistance (AMR) requires a multifaceted approach. Continued investment in research and development, global collaboration, and the effective implementation of surveillance and prevention strategies are crucial. Bacterial Cytological Profiling (BCP) stands out as a rapid and cost-effective technique that facilitates drug discovery by revealing the mechanism of action of novel antibacterial agents through detailed physiological and morphological analysis. Furthermore, BCP could be used to identify phenotypic changes when multiple antibiotics are used, revealing unique or overlapping cell morphologies induced by these combinations (Samernate et al., 2023). However, systematic explorations of cytological profiles for drug combinations are still missing.
Apart from bacteria, cytological profiling methods are also widely used for other organisms such as yeast (Chessel and Carazo Salas, 2019; Chong et al., 2015), fungi (McMahon et al., 2023), and human cells (McDiarmid et al., 2024; Ren et al., 2021; Perlman et al., 2004). Deep learning techniques employed for yeast and human cells have been used without feature extraction, however, this method has only recently been used for bacteria (Spahn et al., 2022). Therefore, wider availability, applications and integration of machine learning tools across different scientific fields are needed.
Besides BCP being used to discover new antibiotics, BCP has been used to investigate complex interactions between bacteria and their predators—bacteriophages (Deep et al., 2024; Tsunemoto et al., 2023; Birkholz et al., 2024). BCP enables the identification of metabolic pathways and cellular processes targeted by phages and antibiotics, both individually and in combination. Therefore, BCP reveals molecular mechanisms governing the phage-bacteria interaction, ultimately paving the way for more effective phage-based antibacterial therapies (Thompson et al., 2024; Kunisch et al., 2024). While bacteriophages have been used in BCP studies, intracellular pathogens present a future challenge as these cytological profiles may strongly depend on host-specific interactions.
JS: Conceptualization, Data curation, Methodology, Visualization, Writing – original draft, Writing – review & editing. JR: Data curation, Methodology, Writing – original draft, Writing – review & editing. NO: Conceptualization, Funding acquisition, Writing – review & editing.
The author(s) declare that financial support was received for the research, authorship, and/or publication of this article. We gratefully acknowledge funding from BBSRC (BB/Y009002/1).
We gratefully acknowledge funding from BBSRC (BB/Y009002/1). We thank reviewers for insightful comments.
The authors declare that the research was conducted in the absence of any commercial or financial relationships that could be construed as a potential conflict of interest.
The authors declare that no Generative AI was used in the creation of this manuscript.
All claims expressed in this article are solely those of the authors and do not necessarily represent those of their affiliated organizations, or those of the publisher, the editors and the reviewers. Any product that may be evaluated in this article, or claim that may be made by its manufacturer, is not guaranteed or endorsed by the publisher.
Allan, E. J., Hoischen, C., and Gumpert, J. (2009). Bacterial L-forms. Adv. Appl. Microbiol. 68, 1–39. doi: 10.1016/S0065-2164(09)01201-5
Allen, R., Ames, L., Baldin, V. P., Butts, A., Henry, K. J., Durst, G., et al. (2024). An arylsulphonamide that targets cell wall biosynthesis in Mycobacterium tuberculosis. Antimicrob Agents Chemother 68: e01037–24. doi: 10.1128/aac.01037-24
Andreu, J. M., Huecas, S., Araújo-Bazán, L., Vázquez-Villa, H., and Martín-Fontecha, M. (2022). The search for antibacterial inhibitors targeting cell division protein FtsZ at its nucleotide and allosteric binding sites. Biomedicines 10:1825. doi: 10.3390/biomedicines10081825
Araújo-Bazán, L., Ruiz-Avila, L. B., Andreu, D., Huecas, S., and Andreu, J. M. (2016). Cytological profile of antibacterial FtsZ inhibitors and synthetic peptide MciZ. Front. Microbiol. 7:1558. doi: 10.3389/fmicb.2016.01558
Ayon, N. J. (2023). High-throughput screening of natural product and synthetic molecule libraries for antibacterial drug discovery. Meta 13:625. doi: 10.3390/metabo13050625
Bailey, S. (2012). Principal component analysis with noisy and/or missing data. Publ. Astron. Soc. Pac. 124, 1015–1023. doi: 10.1086/668105
Balaban, N. Q., Helaine, S., Lewis, K., Ackermann, M., Aldridge, B., Andersson, D. I., et al. (2019). Definitions and guidelines for research on antibiotic persistence. Nat. Rev. Microbiol. 17, 441–448. doi: 10.1038/s41579-019-0196-3
Bali, A., and Singh, S. N. (2015). “A review on the strategies and techniques of image segmentation” in 2015 fifth international conference on advanced computing & communication technologies, 113–120.
Bandow, J. E., Brötz, H., Leichert, L. I. O., Labischinski, H., and Hecker, M. (2003). Proteomic approach to understanding antibiotic action. Antimicrob. Agents Chemother. 47, 948–955. doi: 10.1128/AAC.47.3.948-955.2003
Bandow, J. E., and Hecker, M. (2007). Proteomic profiling of cellular stresses in Bacillus subtilis reveals cellular networks and assists in elucidating antibiotic mechanisms of action. Prog. Drug Res. Fortschritte Arzneimittelforschung Progres Rech. Pharm. 64, 81–101. doi: 10.1007/978-3-7643-7567-6_4
Banerjee, S., Lo, K., Ojkic, N., Stephens, R., Scherer, N. F., and Dinner, A. R. (2021). Mechanical feedback promotes bacterial adaptation to antibiotics. Nat. Phys. 17, 403–409. doi: 10.1038/s41567-020-01079-x
Baquero, F., and Levin, B. R. (2021). Proximate and ultimate causes of the bactericidal action of antibiotics. Nat. Rev. Microbiol. 19, 123–132. doi: 10.1038/s41579-020-00443-1
Baquero, F., Martínez, J. L., Sánchez, A., Fernández-de-Bobadilla, M. D., San-Millán, A., and Rodríguez-Beltrán, J. (2023). Bacterial subcellular architecture, structural epistasis, and antibiotic resistance. Biology 12:640. doi: 10.3390/biology12050640
Berg, S., Kutra, D., Kroeger, T., Straehle, C. N., Kausler, B. X., Haubold, C., et al. (2019). ilastik: interactive machine learning for (bio)image analysis. Nat. Methods 16, 1226–1232. doi: 10.1038/s41592-019-0582-9
Bernabeu-Wittel, M., García-Curiel, A., Pichardo, C., Pachon-Ibanez, M. E., Jimenez-Mejias, M. E., and Pachón, J. (2004). Morphological changes induced by imipenem and meropenem at sub-inhibitory concentrations in Acinetobacter baumannii. Clin. Microbiol. Infect. Off. Publ. Eur. Soc. Clin. Microbiol. Infect. Dis. 10, 931–934. doi: 10.1111/j.1469-0691.2004.00944.x
Birkholz, E. A., Morgan, C. J., Laughlin, T. G., Lau, R. K., Prichard, A., Rangarajan, S., et al. (2024). An intron endonuclease facilitates interference competition between coinfecting viruses. Science 385, 105–112. doi: 10.1126/science.adl1356
Blaskovich, M. A. T., Kavanagh, A. M., Elliott, A. G., Zhang, B., Ramu, S., Amado, M., et al. (2021). The antimicrobial potential of cannabidiol. Commun. Biol. 4, 1–18. doi: 10.1038/s42003-020-01530-y
Boshoff, H. I. M., Myers, T. G., Copp, B. R., McNeil, M. R., Wilson, M. A., and Barry, C. E. III. (2004). The transcriptional responses of Mycobacterium tuberculosis to inhibitors of metabolism: novel insights into drug mechanisms of action*. J. Biol. Chem. 279, 40174–40184. doi: 10.1074/jbc.M406796200
Butler, M. S., Gigante, V., Sati, H., Paulin, S., Al-Sulaiman, L., Rex, J. H., et al. (2022). Analysis of the clinical pipeline of treatments for drug-resistant bacterial infections: despite progress, more action is needed. Antimicrob. Agents Chemother. 66:e0199121. doi: 10.1128/AAC.01991-21
Cacace, E., Kritikos, G., and Typas, A. (2017). Chemical genetics in drug discovery. Curr. Opin. Syst. Biol. 4, 35–42. doi: 10.1016/j.coisb.2017.05.020
Centers for Disease Control and Prevention (U.S.). (2019). Antibiotic Resistance Threats in the United States, 2019. Available at: https://stacks.cdc.gov/view/cdc/82532
Chai, B., Efstathiou, C., Yue, H., and Draviam, V. M. (2023). Opportunities and challenges for deep learning in cell dynamics research. Trends Cell Biol. 34, 955–967. doi: 10.1016/j.tcb.2023.10.010
Chen, K., Sun, G. W., Chua, K. L., and Gan, Y.-H. (2005). Modified virulence of antibiotic-induced Burkholderia pseudomallei filaments. Antimicrob. Agents Chemother. 49, 1002–1009. doi: 10.1128/AAC.49.3.1002-1009.2005
Chessel, A., and Carazo Salas, R. E. (2019). From observing to predicting single-cell structure and function with high-throughput/high-content microscopy. Essays Biochem. 63, 197–208. doi: 10.1042/EBC20180044
Chong, Y. T., Koh, J. L. Y., Friesen, H., Kaluarachchi Duffy, S., Cox, M. J., Moses, A., et al. (2015). Yeast proteome dynamics from single cell imaging and automated analysis. Cell 161, 1413–1424. doi: 10.1016/j.cell.2015.04.051
Chopra, I., and Roberts, M. (2001). Tetracycline antibiotics: mode of action, applications, molecular biology, and epidemiology of bacterial resistance. Microbiol. Mol. Biol. Rev. 65, 232–260. doi: 10.1128/MMBR.65.2.232-260.2001
Coram, M. A., Wang, L., Godinez, W. J., Barkan, D. T., Armstrong, Z., Ando, D. M., et al. (2022). Morphological characterization of antibiotic combinations. ACS Infect. Dis. 8, 66–77. doi: 10.1021/acsinfecdis.1c00312
Corona, F., and Martinez, J. L. (2013). Phenotypic resistance to antibiotics. Antibiotics 2, 237–255. doi: 10.3390/antibiotics2020237
Correia, S., Poeta, P., Hébraud, M., Capelo, J. L., and Igrejas, G. (2017). Mechanisms of quinolone action and resistance: where do we stand? J. Med. Microbiol. 66, 551–559. doi: 10.1099/jmm.0.000475
Cotsonas King, A., and Wu, L. (2009). Macromolecular synthesis and membrane perturbation assays for mechanisms of action studies of antimicrobial agents. Curr. Protoc. Pharmacol. 47:13A-7. doi: 10.1002/0471141755.ph13a07s47
Curtis, N. A., Orr, D., Ross, G. W., and Boulton, M. G. (1979). Affinities of penicillins and cephalosporins for the penicillin-binding proteins of Escherichia coli K-12 and their antibacterial activity. Antimicrob. Agents Chemother. 16, 533–539. doi: 10.1128/AAC.16.5.533
Cushnie, T. P. T., O’Driscoll, N. H., and Lamb, A. J. (2016). Morphological and ultrastructural changes in bacterial cells as an indicator of antibacterial mechanism of action. Cell. Mol. Life Sci. 73, 4471–4492. doi: 10.1007/s00018-016-2302-2
Cutler, K. J., Stringer, C., Lo, T. W., Rappez, L., Stroustrup, N., Brook Peterson, S., et al. (2022). Omnipose: a high-precision morphology-independent solution for bacterial cell segmentation. Nat. Methods 19, 1438–1448. doi: 10.1038/s41592-022-01639-4
Cylke, C., Si, F., and Banerjee, S. (2022). Effects of antibiotics on bacterial cell morphology and their physiological origins. Biochem. Soc. Trans. 50, 1269–1279. doi: 10.1042/BST20210894
Da Cunha, B. R., Zoio, P., Fonseca, L. P., and Calado, C. R. C. (2021). Technologies for high-throughput identification of antibiotic mechanism of action. Antibiotics 10:565. doi: 10.3390/antibiotics10050565
Dalhoff, A., Nasu, T., and Okamoto, K. (2003). Target affinities of faropenem to and its impact on the morphology of gram-positive and gram-negative bacteria. Chemotherapy 49, 172–183. doi: 10.1159/000071141
Davies, J., and Davies, D. (2010). Origins and evolution of antibiotic resistance. Microbiol. Mol. Biol. Rev. 74, 417–433. doi: 10.1128/MMBR.00016-10
Davis, B. D., Chen, L. L., and Tai, P. C. (1986). Misread protein creates membrane channels: an essential step in the bactericidal action of aminoglycosides. Proc. Natl. Acad. Sci. USA 83, 6164–6168. doi: 10.1073/pnas.83.16.6164
de Pedro, M. A., Donachie, W. D., Höltje, J.-V., and Schwarz, H. (2001). Constitutive septal murein synthesis in Escherichia coli with impaired activity of the morphogenetic proteins RodA and penicillin-binding protein 2. J. Bacteriol. 183, 4115–4126. doi: 10.1128/JB.183.14.4115-4126.2001
De Souza-Guerreiro, T. C., Huan Bacellar, L., da Costa, T. S., Edwards, C. L., Tasic, L., and Asally, M. (2024). Membrane potential dynamics unveil the promise of bioelectrical antimicrobial susceptibility testing (BeAST) for anti-fungal screening. MBio 15, e01302–e01324. doi: 10.1128/mbio.01302-24
de Wet, T. J., Winkler, K. R., Mhlanga, M., Mizrahi, V., and Warner, D. F. (2020). Arrayed CRISPRi and quantitative imaging describe the morphotypic landscape of essential mycobacterial genes. eLife 9:e60083. doi: 10.7554/eLife.60083
Deep, A., Liang, Q., Enustun, E., Pogliano, J., and Corbett, K. D. (2024). Architecture and activation mechanism of the bacterial PARIS defence system. Nature 634, 432–439. doi: 10.1038/s41586-024-07772-8
di, E., Erbetti, I., Ferrari, L., Galassi, G., Hammond, S., and Xerri, L. (1994). In vitro activity of the tribactam GV104326 against gram-positive, gram-negative, and anaerobic bacteria. Antimicrob. Agents Chemother. 38, 2362–2368. doi: 10.1128/AAC.38.10.2362
di, S., Giacobbe, D., Maraolo, A., Viaggi, V., Luzzati, R., Bassetti, M., et al. (2021). Resistance to ceftazidime/avibactam in infections and colonisations by KPC-producing Enterobacterales: a systematic review of observational clinical studies. J. Glob. Antimicrob. Resist. 25, 268–281. doi: 10.1016/j.jgar.2021.04.001
Dillon, N. A., Tsunemoto, H., Poudel, S., Meehan, M. J., Seif, Y., Szubin, R., et al. (2020). Characterizing the response of Acinetobacter baumannii ATCC 17978 to azithromycin in multiple in vitro growth conditions. BioRchiv [Preprint]. doi: 10.1101/2020.05.19.079962
Donald, R. G. K., Skwish, S., Forsyth, R. A., Anderson, J. W., Zhong, T., Burns, C., et al. (2009). A Staphylococcus aureus fitness test platform for mechanism-based profiling of antibacterial compounds. Chem. Biol. 16, 826–836. doi: 10.1016/j.chembiol.2009.07.004
Dörries, K., Schlueter, R., and Lalk, M. (2014). Impact of antibiotics with various target sites on the metabolome of Staphylococcus aureus. Antimicrob. Agents Chemother. 58, 7151–7163. doi: 10.1128/AAC.03104-14
Ducret, A., Quardokus, E. M., and Brun, Y. V. (2016). MicrobeJ, a tool for high throughput bacterial cell detection and quantitative analysis. Nat. Microbiol. 1:16077. doi: 10.1038/nmicrobiol.2016.77
Elliott, T. S. J., Shelton, A., and Greenwood, D. (1987). The response of Escherichia coli to ciprofloxacin and norfloxacin. J. Med. Microbiol. 23, 83–88. doi: 10.1099/00222615-23-1-83
El-sagheir, A. M. K., Abdelmesseh Nekhala, I., Abd El-Gaber, M. K., Aboraia, A. S., Persson, J., Schäfer, A. B., et al. (2023). Rational design, synthesis, molecular modeling, biological activity, and mechanism of action of polypharmacological norfloxacin hydroxamic acid derivatives. RSC Med. Chem. 14, 2593–2610. doi: 10.1039/D3MD00309D
Errington, J. (2013). L-form bacteria, cell walls and the origins of life. Open Biol. 3:120143. doi: 10.1098/rsob.120143
Errington, J. (2017). Cell wall-deficient, L-form bacteria in the 21st century: a personal perspective. Biochem. Soc. Trans. 45, 287–295. doi: 10.1042/BST20160435
Fan, J., Fu, A., and Zhang, L. (2019). Progress in molecular docking. Quant. Biol. 7, 83–89. doi: 10.1007/s40484-019-0172-y
Feng, L., Wu, K., Pei, Z., Weng, T., Han, Q., Meng, L., et al. (2024). MLU-Net: a multi-level lightweight U-net for medical image segmentation integrating frequency representation and MLP-based methods. IEEE Access 12, 20734–20751. doi: 10.1109/ACCESS.2024.3360889
Franken, H., Mathieson, T., Childs, D., Sweetman, G. M. A., Werner, T., Tögel, I., et al. (2015). Thermal proteome profiling for unbiased identification of direct and indirect drug targets using multiplexed quantitative mass spectrometry. Nat. Protoc. 10, 1567–1593. doi: 10.1038/nprot.2015.101
Freiberg, C., Fischer, H. P., and Brunner, N. A. (2005). Discovering the mechanism of action of novel antibacterial agents through transcriptional profiling of conditional mutants. Antimicrob. Agents Chemother. 49, 749–759. doi: 10.1128/AAC.49.2.749-759.2005
Gardner, A. (1945). Microscopical effect of penicillin on spores and vegetative cells of bacilli. Lancet 245, 658–659. doi: 10.1016/S0140-6736(45)90043-2
Gardner, A. D. (1940). Morphological effects of penicillin on bacteria. Nature 146, 837–838. doi: 10.1038/146837b0
Gebicki, J. M., and James, A. M. (1960). The preparation and properties of spheroplasts of aerobacter aerogenes. J. Gen. Microbiol. 23, 9–18. doi: 10.1099/00221287-23-1-9
Goldsborough, P., Pawlowski, N., Caicedo, J. C., Singh, S., and Carpenter, A. E. (2017). CytoGAN: generative modeling of cell images. BioRchiv [Preprint]. doi: 10.1101/227645
Grein, F., Müller, A., Scherer, K. M., Liu, X., Ludwig, K. C., Klöckner, A., et al. (2020). Ca2+-Daptomycin targets cell wall biosynthesis by forming a tripartite complex with undecaprenyl-coupled intermediates and membrane lipids. Nat. Commun. 11:1455. doi: 10.1038/s41467-020-15257-1
Hage, D. S., Anguizola, J. A., Bi, C., Li, R., Matsuda, R., Papastavros, E., et al. (2012). Pharmaceutical and biomedical applications of affinity chromatography: Recent trends and developments. J. Pharm. Biomed. Anal. 69, 93–105. doi: 10.1016/j.jpba.2012.01.004
Hausen, R., and Robertson, B. E. (2020). Morpheus: a deep learning framework for the pixel-level analysis of astronomical image data. Astrophys. J. Suppl. Ser. 248:20. doi: 10.3847/1538-4365/ab8868
Herschede, S. R., Salam, R., Gneid, H., and Busschaert, N. (2022). Bacterial cytological profiling identifies transmembrane anion transport as the mechanism of action for a urea-based antibiotic. Supramol. Chem. 34, 26–33. doi: 10.1080/10610278.2023.2178921
Hoerr, V., Duggan, G. E., Zbytnuik, L., Poon, K. K. H., Große, C., Neugebauer, U., et al. (2016). Characterization and prediction of the mechanism of action of antibiotics through NMR metabolomics. BMC Microbiol. 16:82. doi: 10.1186/s12866-016-0696-5
Horii, T., Kobayashi, M., Sato, K., Ichiyama, S., and Ohta, M. (1998). An in-vitro study of carbapenem-induced morphological changes and endotoxin release in clinical isolates of gram-negative bacilli. J. Antimicrob. Chemother. 41, 435–442. doi: 10.1093/jac/41.4.435
Htoo, H. H., Brumage, L., Chaikeeratisak, V., Tsunemoto, H., Sugie, J., Tribuddharat, C., et al. (2019). Bacterial cytological profiling as a tool to study mechanisms of action of antibiotics that are active against Acinetobacter baumannii. Antimicrob. Agents Chemother. 63:10-1128. doi: 10.1128/aac.02310-18
Hudson, M. A., and Lockless, S. W. (2022). Elucidating the mechanisms of action of antimicrobial agents. MBio 13:e0224021. doi: 10.1128/mbio.02240-21
Hutchings, M. I., Truman, A. W., and Wilkinson, B. (2019). Antibiotics: past, present and future. Curr. Opin. Microbiol. 51, 72–80. doi: 10.1016/j.mib.2019.10.008
Isola, P., Zhu, J.-Y., Zhou, T., and Efros, A. A. (2018). Image-to-image translation with conditional adversarial networks. arXiv [Preprint]. doi: 10.48550/arXiv.1611.07004
Jackson, J. J., and Kropp, H. (1996). Differences in mode of action of (β-lactam antibiotics influence morphology, LPS release and in vivo antibiotic efficacy. J. Endotoxin Res. 3, 201–218. doi: 10.1177/096805199600300306
Jago, M. J., Soley, J. K., Denisov, S., Walsh, C. J., Gifford, D. R., Howden, B. P., et al. (2025). High-throughput method characterizes hundreds of previously unknown antibiotic resistance mutations. Nat. Commun. 16:780. doi: 10.1038/s41467-025-56050-2
Jan, M., Spangaro, A., Lenartowicz, M., and Mattiazzi Usaj, M. (2024). From pixels to insights: machine learning and deep learning for bioimage analysis. BioEssays 46:e2300114. doi: 10.1002/bies.202300114
Jaramillo-Riveri, S., Broughton, J., McVey, A., Pilizota, T., Scott, M., and el Karoui, M. (2022). Growth-dependent heterogeneity in the DNA damage response in Escherichia coli. Mol. Syst. Biol. 18:e10441. doi: 10.15252/msb.202110441
Jerala, R. (2007). Synthetic lipopeptides: a novel class of anti-infectives. Expert Opin. Investig. Drugs 16, 1159–1169. doi: 10.1517/13543784.16.8.1159
Jones, E. C., and Uphoff, S. (2021). Single-molecule imaging of LexA degradation in Escherichia coli elucidates regulatory mechanisms and heterogeneity of the SOS response. Nat. Microbiol. 6, 981–990. doi: 10.1038/s41564-021-00930-y
Kalla, G. (2020). Using bacterial cytological profiling to study the interactions of bacteria and the defense systems of multicellular eukaryotes. USA: University of California San Diego.
Kohanski, M. A., Dwyer, D. J., and Collins, J. J. (2010). How antibiotics kill bacteria: from targets to networks. Nat. Rev. Microbiol. 8, 423–435. doi: 10.1038/nrmicro2333
Korsunsky, I., Millard, N., Fan, J., Slowikowski, K., Zhang, F., Wei, K., et al. (2019). Fast, sensitive and accurate integration of single-cell data with Harmony. Nat. Methods 16, 1289–1296. doi: 10.1038/s41592-019-0619-0
Kunisch, F., Campobasso, C., Wagemans, J., Yildirim, S., Chan, B. K., Schaudinn, C., et al. (2024). Targeting Pseudomonas aeruginosa biofilm with an evolutionary trained bacteriophage cocktail exploiting phage resistance trade-offs. Nat. Commun. 15:8572. doi: 10.1038/s41467-024-52595-w
Kuru, E., Hughes, H. V., Brown, P. J., Hall, E., Tekkam, S., Cava, F., et al. (2012). In situ probing of newly synthesized peptidoglycan in live bacteria with fluorescent D-amino acids. Angew. Chem. Int. Ed. 51, 12519–12523. doi: 10.1002/anie.201206749
Kussell, E., Kishony, R., Balaban, N. Q., and Leibler, S. (2005). Bacterial Persistence. Genetics 169, 1807–1814. doi: 10.1534/genetics.104.035352
Lamsa, A., Lopez-Garrido, J., Quach, D., Riley, E. P., Pogliano, J., and Pogliano, K. (2016). Rapid inhibition profiling in Bacillus subtilis to identify the mechanism of action of new antimicrobials. ACS Chem. Biol. 11, 2222–2231. doi: 10.1021/acschembio.5b01050
Lewis, K. (2013). Platforms for antibiotic discovery. Nat. Rev. Drug Discov. 12, 371–387. doi: 10.1038/nrd3975
Lewis, K., Lee, R. E., Brötz-Oesterhelt, H., Hiller, S., Rodnina, M. V., Schneider, T., et al. (2024). Sophisticated natural products as antibiotics. Nature 632, 39–49. doi: 10.1038/s41586-024-07530-w
Lima, L. M., Da Silva, B. N. M., Barbosa, G., and Barreiro, E. J. (2020). β-lactam antibiotics: an overview from a medicinal chemistry perspective. Eur. J. Med. Chem. 208:112829. doi: 10.1016/j.ejmech.2020.112829
Lin, L., Nonejuie, P., Munguia, J., Hollands, A., Olson, J., Dam, Q., et al. (2015). Azithromycin synergizes with cationic antimicrobial peptides to exert bactericidal and therapeutic activity against highly multidrug-resistant gram-negative bacterial pathogens. EBioMedicine 2, 690–698. doi: 10.1016/j.ebiom.2015.05.021
López-Jiménez, A. T., Brokatzky, D., Pillay, K., Williams, T., Güler, G. Ö., and Mostowy, S. (2024). High-content high-resolution microscopy and deep learning assisted analysis reveals host and bacterial heterogeneity during Shigella infection. eLife 13:RP97495. doi: 10.7554/eLife.97495.1
Luepke, K. H., Suda, K. J., Boucher, H., Russo, R. L., Bonney, M. W., Hunt, T. D., et al. (2017). Past, present, and future of antibacterial economics: increasing bacterial resistance, limited antibiotic pipeline, and societal implications. Pharmacother. J. Hum. Pharmacol. Drug Ther. 37, 71–84. doi: 10.1002/phar.1868
Ma, J., Xie, R., Ayyadhury, S., Ge, C., Gupta, A., Gupta, R., et al. (2024). The multimodality cell segmentation challenge: toward universal solutions. Nat. Methods 21, 1103–1113. doi: 10.1038/s41592-024-02233-6
Mandal, S., and Uhlmann, V. (2021). “Splinedist: automated cell segmentation with spline curves” in 2021 IEEE 18th international symposium on biomedical imaging (ISBI), 1082–1086.
Martin, J. K., Sheehan, J. P., Bratton, B. P., Moore, G. M., Mateus, A., Li, S. H., et al. (2020). A dual-mechanism antibiotic kills gram-negative bacteria and avoids drug resistance. Cell 181, 1518–1532.e14. doi: 10.1016/j.cell.2020.05.005
Mayer, B., Schwan, M., Thormann, K. M., and Graumann, P. L. (2022). Antibiotic Drug screening and Image Characterization Toolbox (A.D.I.C.T.): a robust imaging workflow to monitor antibiotic stress response in bacterial cells in vivo. F1000Research 10:277. doi: 10.12688/f1000research.51868.3
McDiarmid, A. H., Gospodinova, K. O., Elliott, R. J. R., Dawson, J. C., Graham, R. E., el-Daher, M. T., et al. (2024). Morphological profiling in human neural progenitor cells classifies hits in a pilot drug screen for Alzheimer’s disease. Brain Commun. 6:fcae101. doi: 10.1093/braincomms/fcae101
McInnes, L., Healy, J., and Melville, J. (2020). UMAP: uniform manifold approximation and projection for dimension reduction. Available at: http://arxiv.org/abs/1802.03426
McMahon, C. L., Esqueda, M., Yu, J. J., Wall, G., Romo, J. A., Vila, T., et al. (2023). Development of an imaging flow cytometry method for fungal cytological profiling and its potential application in antifungal drug development. J. Fungi 9:722. doi: 10.3390/jof9070722
McQuin, C., Goodman, A., Chernyshev, V., Kamentsky, L., Cimini, B. A., Karhohs, K. W., et al. (2018). CellProfiler 3.0: next-generation image processing for biology. PLoS Biol. 16:e2005970. doi: 10.1371/journal.pbio.2005970
Mendelsohn, M. L., Kolman, W. A., Perry, B., and Prewitt, J. M. S. (1965). Computer analysis of cell images. Postgrad. Med. 38, 567–573. doi: 10.1080/00325481.1965.11695692
Mercier, R., Kawai, Y., and Errington, J. (2014). General principles for the formation and proliferation of a wall-free (L-form) state in bacteria. eLife 3:e04629. doi: 10.7554/eLife.04629
Mistretta, M., Cimino, M., Campagne, P., Volant, S., Kornobis, E., Hebert, O., et al. (2024). Dynamic microfluidic single-cell screening identifies pheno-tuning compounds to potentiate tuberculosis therapy. Nat. Commun. 15:4175. doi: 10.1038/s41467-024-48269-2
Mohammad, H., Younis, W., Ezzat, H. G., Peters, C. E., AbdelKhalek, A., Cooper, B., et al. (2017). Bacteriological profiling of diphenylureas as a novel class of antibiotics against methicillin-resistant Staphylococcus aureus. PLoS One 12:e0182821. doi: 10.1371/journal.pone.0182821
Montaño, E. T., Nideffer, J. F., Sugie, J., Enustun, E., Shapiro, A. B., Tsunemoto, H., et al. (2021). Bacterial cytological profiling identifies rhodanine-containing PAINS analogs as specific inhibitors of Escherichia coli thymidylate kinase in vivo. J. Bacteriol. 203:e0010521, 10–128. doi: 10.1128/jb.00105-21
Murray, C. J. L., Ikuta, K. S., Sharara, F., Swetschinski, L., Robles Aguilar, G., Gray, A., et al. (2022). Global burden of bacterial antimicrobial resistance in 2019: a systematic analysis. Lancet 399, 629–655. doi: 10.1016/S0140-6736(21)02724-0
Naknaen, A., Samernate, T., Saeju, P., Nonejuie, P., and Chaikeeratisak, V. (2024). Nucleus-forming jumbophage PhiKZ therapeutically outcompetes non-nucleus-forming jumbophage Callisto. iScience 27:109790. doi: 10.1016/j.isci.2024.109790
Naknaen, A., Samernate, T., Wannasrichan, W., Surachat, K., Nonejuie, P., and Chaikeeratisak, V. (2023). Combination of genetically diverse Pseudomonas phages enhances the cocktail efficiency against bacteria. Sci. Rep. 13:8921. doi: 10.1038/s41598-023-36034-2
Nickerson, W. J., and Webb, M. (1956). Effect of folic acid analogues on growth and cell division of nonexacting microorganisms. J. Bacteriol. 71, 129–139. doi: 10.1128/jb.71.2.129-139.1956
Nonejuie, P., Burkart, M., Pogliano, K., and Pogliano, J. (2013). Bacterial cytological profiling rapidly identifies the cellular pathways targeted by antibacterial molecules. Proc. Natl. Acad. Sci. 110, 16169–16174. doi: 10.1073/pnas.1311066110
Nonejuie, P., Trial, R. M., Newton, G. L., Lamsa, A., Ranmali Perera, V., Aguilar, J., et al. (2016). Application of bacterial cytological profiling to crude natural product extracts reveals the antibacterial arsenal of Bacillus subtilis. J. Antibiot. (Tokyo) 69, 353–361. doi: 10.1038/ja.2015.116
O’Neill, J. (2016). Tackling drug-resistant infections globally: final report and recommendations. Available at: https://scholar.google.co.uk/scholar?q=Tackling+drug-resistant+infections+globally:+final+report+and+recommendations.&hl=es&as_sdt=0&as_vis=1&oi=scholart
O’Rourke, A., Beyhan, S., Choi, Y., Morales, P., Chan, A. P., Espinoza, J. L., et al. (2020). Mechanism-of-action classification of antibiotics by global transcriptome profiling. Antimicrob. Agents Chemother. 64, e01207–e01219. doi: 10.1128/AAC.01207-19
Ojkic, N., and Banerjee, S. (2021). Bacterial cell shape control by nutrient-dependent synthesis of cell division inhibitors. Biophys. J. 120, 2079–2084. doi: 10.1016/j.bpj.2021.04.001
Ojkic, N., Lilja, E., Direito, S., Dawson, A., Allen, R. J., and Waclaw, B. (2020). A roadblock-and-kill mechanism of action model for the DNA-targeting antibiotic ciprofloxacin. Antimicrob. Agents Chemother. 64, e02487–e02419. doi: 10.1128/AAC.02487-19
Ojkic, N., López-Garrido, J., Pogliano, K., and Endres, R. G. (2014). Bistable forespore engulfment in Bacillus subtilis by a zipper mechanism in absence of the cell wall. PLoS Comput. Biol. 10:e1003912. doi: 10.1371/journal.pcbi.1003912
Ojkic, N., Serbanescu, D., and Banerjee, S. (2019). Surface-to-volume scaling and aspect ratio preservation in rod-shaped bacteria. eLife 8:e47033. doi: 10.7554/eLife.47033
Ojkic, N., Serbanescu, D., and Banerjee, S. (2022). Antibiotic resistance via bacterial cell shape-shifting. MBio 13, e00659–e00622. doi: 10.1128/mbio.00659-22
Osokin, A., Chessel, A., Salas, R. E. C., and Vaggi, F. (2017). “GANs for biological image synthesis” in in 2017 IEEE international conference on computer vision (ICCV) (Venice: IEEE), 2252–2261.
Ouyang, X., Hoeksma, J., Lubbers, R. J. M., Siersma, T. K., Hamoen, L. W., and den Hertog, J. (2022). Classification of antimicrobial mechanism of action using dynamic bacterial morphology imaging. Sci. Rep. 12:11162. doi: 10.1038/s41598-022-15405-1
Paintdakhi, A., Parry, B., Campos, M., Irnov, I., Elf, J., Surovtsev, I., et al. (2016). Oufti: An integrated software package for high-accuracy, high-throughput quantitative microscopy analysis. Mol. Microbiol. 99, 767–777. doi: 10.1111/mmi.13264
Perlman, Z. E., Slack, M. D., Feng, Y., Mitchison, T. J., Wu, L. F., and Altschuler, S. J. (2004). Multidimensional drug profiling by automated microscopy. Science 306, 1194–1198. doi: 10.1126/science.1100709
Perumalsamy, H., Jung, M. Y., Hong, S. M., and Ahn, Y.-J. (2013). Growth-Inhibiting and morphostructural effects of constituents identified in Asarum heterotropoides root on human intestinal bacteria. BMC Complement. Altern. Med. 13:245. doi: 10.1186/1472-6882-13-245
Prewitt, J. M. S., and Mendelsohn, M. L. (1966). The analysis of cell images*. Ann. New York Acad. Sci. 128, 1035–1053. doi: 10.1111/j.1749-6632.1965.tb11715.x
Prindle, A., Liu, J., Asally, M., Ly, S., Garcia-Ojalvo, J., and Süel, G. M. (2015). Ion channels enable electrical communication in bacterial communities. Nature 527, 59–63. doi: 10.1038/nature15709
PubChem. Cerulenin. Available at: https://pubchem.ncbi.nlm.nih.gov/compound/5282054 (Accessed October 22, 2024).
Quach, D. T., Sakoulas, G., Nizet, V., Pogliano, J., and Pogliano, K. (2016). Bacterial cytological profiling (BCP) as a rapid and accurate antimicrobial susceptibility testing method for Staphylococcus aureus. EBioMedicine 4, 95–103. doi: 10.1016/j.ebiom.2016.01.020
Quinn, G. A., and Dyson, P. J. (2024). Going to extremes: progress in exploring new environments for novel antibiotics. Npj Antimicrob. Resist. 2:8. doi: 10.1038/s44259-024-00025-8
Regev, A., Teichmann, S., Lander, E., Amit, I., Benoist, C., Birney, E., et al. (2017). The human cell atlas. eLife 6:e27041. doi: 10.7554/eLife.27041
Ren, E., Kim, S., Mohamad, S., Huguet, S. F., Shi, Y., Cohen, A. R., et al. (2021). Deep learning-enhanced morphological profiling predicts cell fate dynamics in real-time in hPSCs. doi: 10.1101/2021.07.31.454574
Reynolds, P. E. (1989). Structure, biochemistry and mechanism of action of glycopeptide antibiotics. Eur. J. Clin. Microbiol. Infect. Dis. 8, 943–950. doi: 10.1007/BF01967563
Ribeiro da Cunha, B., Fonseca, L. P., and Calado, C. R. C. (2020). Metabolic fingerprinting with Fourier-transform infrared (FTIR) spectroscopy: towards a high-throughput screening assay for antibiotic discovery and mechanism-of-action elucidation. Meta 10:145. doi: 10.3390/metabo10040145
Sakoulas, G., Nonejuie, P., Kullar, R., Pogliano, J., Rybak, M. J., and Nizet, V. (2015). Examining the use of ceftaroline in the treatment of Streptococcus pneumoniae meningitis with reference to human cathelicidin LL-37. Antimicrob. Agents Chemother. 59, 2428–2431. doi: 10.1128/AAC.04965-14
Samernate, T., Htoo, H. H., Sugie, J., Chavasiri, W., Pogliano, J., Chaikeeratisak, V., et al. (2023). High-resolution bacterial cytological profiling reveals intrapopulation morphological variations upon antibiotic exposure. Antimicrob. Agents Chemother. 67, e01307–e01322. doi: 10.1128/aac.01307-22
Santos, T. M. A., Lammers, M. G., Zhou, M., Sparks, I. L., Rajendran, M., Fang, D., et al. (2018). Small molecule chelators reveal that iron starvation inhibits late stages of bacterial cytokinesis. ACS Chem. Biol. 13, 235–246. doi: 10.1021/acschembio.7b00560
Schäfer, A.-B., Sidarta, M., Abdelmesseh Nekhala, I., Marinho Righetto, G., Arshad, A., and Wenzel, M. (2024). Dissecting antibiotic effects on the cell envelope using bacterial cytological profiling: a phenotypic analysis starter kit. Microbiol. Spectr. 12, e03275–e03223. doi: 10.1128/spectrum.03275-23
Schindelin, J., Arganda-Carreras, I., Frise, E., Kaynig, V., Longair, M., Pietzsch, T., et al. (2012). Fiji: an open-source platform for biological-image analysis. Nat. Methods 9, 676–682. doi: 10.1038/nmeth.2019
Serbanescu, D., Ojkic, N., and Banerjee, S. (2022). Cellular resource allocation strategies for cell size and shape control in bacteria. FEBS J. 289, 7891–7906. doi: 10.1111/febs.16234
Silver, L. L. (2011). Challenges of antibacterial discovery. Clin. Microbiol. Rev. 24, 71–109. doi: 10.1128/CMR.00030-10
Smith, T. C., Pullen, K. M., Olson, M. C., ME, M. N., Richardson, I., Hu, S., et al. (2020). Morphological profiling of tubercle bacilli identifies drug pathways of action. Proc. Natl. Acad. Sci. USA 117, 18744–18753. doi: 10.1073/pnas.2002738117
Sofroniew, N., Lambert, T., Bokota, G., Nunez-Iglesias, J., Sobolewski, P., Sweet, A., et al. (2025). napari: a multi-dimensional image viewer for Python. Zenodo. doi: 10.5281/zenodo.14719463
Soonthonsrima, T., Htoo, H. H., Thiennimitr, P., Srisuknimit, V., Nonejuie, P., and Chaikeeratisak, V. (2023). Phage-induced bacterial morphological changes reveal a phage-derived antimicrobial affecting cell wall integrity. Antimicrob. Agents Chemother. 67, e00764–e00723. doi: 10.1128/aac.00764-23
Sousa, M. (2020). StarDist: application of the deep-learning tool for phase-contrast cell images. arXiv [Preprint]. doi: 10.48550/arXiv.1908.03636
Spahn, C., Gómez-de-Mariscal, E., Laine, R. F., Pereira, P. M., von Chamier, L., Conduit, M., et al. (2022). DeepBacs for multi-task bacterial image analysis using open-source deep learning approaches. Commun. Biol. 5, 1–18. doi: 10.1038/s42003-022-03634-z
Spratt, B. G. (1975). Distinct penicillin binding proteins involved in the division, elongation, and shape of Escherichia coli K12. Proc. Natl. Acad. Sci. USA 72, 2999–3003. doi: 10.1073/pnas.72.8.2999
Spratt, B. G., and Pardee, A. B. (1975). Penicillin-binding proteins and cell shape in E. coli. Nature 254, 516–517. doi: 10.1038/254516a0
Sridhar, S., Forrest, S., Warne, B., Maes, M., Baker, S., Dougan, G., et al. (2021). High-content imaging to phenotype antimicrobial effects on individual bacteria at scale. mSystems 6:e00028-21. doi: 10.1128/msystems.00028-21
Stratford, J. P., Edwards, C. L. A., Ghanshyam, M. J., Malyshev, D., Delise, M. A., Hayashi, Y., et al. (2019). Electrically induced bacterial membrane-potential dynamics correspond to cellular proliferation capacity. Proc. Natl. Acad. Sci. 116, 9552–9557. doi: 10.1073/pnas.1901788116
Stringer, C., Wang, T., Michaelos, M., and Pachitariu, M. (2021). Cellpose: a generalist algorithm for cellular segmentation. Nat. Methods 18, 100–106. doi: 10.1038/s41592-020-01018-x
Stylianidou, S., Brennan, C., Nissen, S. B., Kuwada, N. J., and Wiggins, P. A. (2016). SuperSegger: robust image segmentation, analysis and lineage tracking of bacterial cells. Mol. Microbiol. 102, 690–700. doi: 10.1111/mmi.13486
Sumita, Y., Fukasawa, M., and Okuda, T. (1990). Comparison of two carbapenems, SM-7338 and imipenem: affinities for penicillin-binding proteins and morphological changes. J. Antibiot. (Tokyo) 43, 314–320. doi: 10.7164/antibiotics.43.314
Sun, Y., Heidary, D. K., Zhang, Z., Richards, C. I., and Glazer, E. C. (2018). Bacterial cytological profiling reveals the mechanism of action of anticancer metal complexes. Mol. Pharm. 15, 3404–3416. doi: 10.1021/acs.molpharmaceut.8b00407
Sun, D., Tsivkovski, R., Pogliano, J., Tsunemoto, H., Nelson, K., Rubio-Aparicio, D., et al. (2022). Intrinsic antibacterial activity of Xeruborbactam in vitro: assessing spectrum and mode of action. Antimicrob. Agents Chemother. 66:e00879-22. doi: 10.1128/aac.00879-22
Swaney, S. M., Aoki, H., Ganoza, M. C., and Shinabarger, D. L. (1998). The oxazolidinone linezolid inhibits initiation of protein synthesis in bacteria. Antimicrob. Agents Chemother. 42, 3251–3255. doi: 10.1128/AAC.42.12.3251
Tacconelli, E., Carrara, E., Savoldi, A., Harbarth, S., Mendelson, M., Monnet, D. L., et al. (2018). Discovery, research, and development of new antibiotics: the WHO priority list of antibiotic-resistant bacteria and tuberculosis. Lancet Infect. Dis. 18, 318–327. doi: 10.1016/S1473-3099(17)30753-3
Takebayashi, Y., Ramos-Soriano, J., Jiang, Y. J., Samphire, J., Belmonte-Reche, E., O’Hagan, M. P., et al. (2024). Small molecule G-quadruplex ligands are antibacterial candidates for Gram-negative bacteria. BioRchiv [Preprint]. doi: 10.1101/2022.09.01.506212
Tenson, T., Lovmar, M., and Ehrenberg, M. (2003). The mechanism of action of macrolides, lincosamides and streptogramin B reveals the nascent peptide exit path in the ribosome. J. Mol. Biol. 330, 1005–1014. doi: 10.1016/S0022-2836(03)00662-4
Thammatinna, K., Egan, M. K. E., Htoo, H. H., Khanna, K., Sugie, J., Nideffer, J. F., et al. (2020). A novel vibriophage exhibits inhibitory activity against host protein synthesis machinery. Sci. Rep. 10:2347. doi: 10.1038/s41598-020-59396-3
Thompson, D. L., Semersky, Z., Feinn, R., Huang, P., Turner, P. E., Chan, B. K., et al. (2024). Particle size distribution of viable nebulized bacteriophage for the treatment of multi-drug resistant Pseudomonas aeruginosa. Respir. Med. Res. 86:101133. doi: 10.1016/j.resmer.2024.101133
Tsunemoto, H., Sugie, J., Enustun, E., Pogliano, K., and Pogliano, J. (2023). Bacterial cytological profiling reveals interactions between jumbo phage φKZ infection and cell wall active antibiotics in Pseudomonas aeruginosa. PLoS One 18:e0280070. doi: 10.1371/journal.pone.0280070
Ulloa, E. R., Kousha, A., Tsunemoto, H., Pogliano, J., Licitra, C., LiPuma, J. J., et al. (2020). Azithromycin exerts bactericidal activity and enhances innate immune mediated killing of MDR Achromobacter xylosoxidans. Infect. Microbes Dis. 2, 10–17. doi: 10.1097/IM9.0000000000000014
Uphoff, S., Reyes-Lamothe, R., Garza de Leon, F., Sherratt, D. J., and Kapanidis, A. N. (2013). Single-molecule DNA repair in live bacteria. Proc. Natl. Acad. Sci. 110, 8063–8068. doi: 10.1073/pnas.1301804110
van, T., Joniau, S., Clinckemalie, L., Helsen, C., Prekovic, S., Spans, L., et al. (2014). The role of single nucleotide polymorphisms in predicting prostate cancer risk and therapeutic decision making. Biomed. Res. Int. 2014, 1–16. doi: 10.1155/2014/627510
Vaswani, A., Shazeer, N., Parmar, N., Uszkoreit, J., Jones, L., Gomez, A. N., et al. (2023). Attention is all you need. arXiv [Preprint]. doi: 10.48550/arXiv.1706.03762
Vázquez-Laslop, N., and Mankin, A. S. (2018). How macrolide antibiotics work. Trends Biochem. Sci. 43, 668–684. doi: 10.1016/j.tibs.2018.06.011
Vincent, I. M., Ehmann, D. E., Mills, S. D., Perros, M., and Barrett, M. P. (2016). Untargeted metabolomics to ascertain antibiotic modes of action. Antimicrob. Agents Chemother. 60, 2281–2291. doi: 10.1128/AAC.02109-15
Vollmer, W., Blanot, D., and de Pedro, M. A. (2008). Peptidoglycan structure and architecture. FEMS Microbiol. Rev. 32, 149–167. doi: 10.1111/j.1574-6976.2007.00094.x
Walsh, C. (2000). Molecular mechanisms that confer antibacterial drug resistance. Nature 406, 775–781. doi: 10.1038/35021219
Wang, Y., Huang, H., Rudin, C., and Shaposhnik, Y. (2021). Understanding how dimension reduction tools work: an empirical approach to deciphering t-SNE, UMAP, TriMAP, and PaCMAP for data visualization. Available at: http://arxiv.org/abs/2012.04456
Weigert, M., and Schmidt, U. (2022). Nuclei instance segmentation and classification in histopathology images with StarDist. arXiv [Preprint]. doi: 10.1109/ISBIC56247.2022.9854534
Weigert, M., Schmidt, U., Boothe, T., Müller, A., Dibrov, A., Jain, A., et al. (2018). Content-aware image restoration: pushing the limits of fluorescence microscopy. Nat. Methods 15, 1090–1097. doi: 10.1038/s41592-018-0216-7
Werth, B. J., Steed, M. E., Ireland, C. E., Tran, T. T., Nonejuie, P., Murray, B. E., et al. (2014). Defining daptomycin resistance prevention exposures in vancomycin-resistant enterococcus faecium and E. faecalis. Antimicrob. Agents Chemother. 58, 5253–5261. doi: 10.1128/AAC.00098-14
WHO. (2023). AWaRe classification of antibiotics for evaluation and monitoring of use, 2023. World Health Organization.
WHO Bacterial Priority Pathogens List (2024). WHO Bacterial Priority Pathogens List, 2024: bacterial pathogens of public health importance to guide research, development and strategies to prevent and control antimicrobial resistance. Geneva: World Health Organization.
Wong, W. R., Oliver, A. G., and Linington, R. G. (2012). Development of antibiotic activity profile screening for the classification and discovery of natural product antibiotics. Chem. Biol. 19, 1483–1495. doi: 10.1016/j.chembiol.2012.09.014
Wong, F., Stokes, J. M., Cervantes, B., Penkov, S., Friedrichs, J., Renner, L. D., et al. (2021). Cytoplasmic condensation induced by membrane damage is associated with antibiotic lethality. Nat. Commun. 12:2321. doi: 10.1038/s41467-021-22485-6
World Health Organization. (2017). Prioritization of pathogens to guide discovery, research and development of new antibiotics for drug-resistant bacterial infections, including tuberculosis (No. WHO/EMP/IAU/2017.12). World Health Organization.
Wu, F., Japaridze, A., Zheng, X., Wiktor, J., Kerssemakers, J. W. J., and Dekker, C. (2019). Direct imaging of the circular chromosome in a live bacterium. Nat. Commun. 10:2194. doi: 10.1038/s41467-019-10221-0
Wu, Y., and Seyedsayamdost, M. R. (2018). The polyene natural product thailandamide A inhibits fatty acid biosynthesis in gram-positive and gram-negative bacteria. Biochemistry 57, 4247–4251. doi: 10.1021/acs.biochem.8b00678
Young, K. D. (2006). The selective value of bacterial shape. Microbiol. Mol. Biol. Rev. 70, 660–703. doi: 10.1128/MMBR.00001-06
Zagajewski, A., Turner, P., Feehily, C., El Sayyed, H., Andersson, M., Barrett, L., et al. (2023). Deep learning and single-cell phenotyping for rapid antimicrobial susceptibility detection in Escherichia coli. Commun. Biol. 6, 1–12. doi: 10.1038/s42003-023-05524-4
Zampaloni, C., Mattei, P., Bleicher, K., Winther, L., Thäte, C., Bucher, C., et al. (2024). A novel antibiotic class targeting the lipopolysaccharide transporter. Nature 625, 566–571. doi: 10.1038/s41586-023-06873-0
Zampieri, M., Szappanos, B., Buchieri, M. V., Trauner, A., Piazza, I., Picotti, P., et al. (2018). High-throughput metabolomic analysis predicts mode of action of uncharacterized antimicrobial compounds. Sci. Transl. Med. 10:eaal3973. doi: 10.1126/scitranslmed.aal3973
Keywords: antibiotic resistance, bacterial cytological profiling, high-throughput screening, antibiotic mechanism of action, bacterial priority pathogen list, cell segmentation, machine learning, deep learning
Citation: Salgado J, Rayner J and Ojkic N (2025) Advancing antibiotic discovery with bacterial cytological profiling: a high-throughput solution to antimicrobial resistance. Front. Microbiol. 16:1536131. doi: 10.3389/fmicb.2025.1536131
Received: 29 November 2024; Accepted: 29 January 2025;
Published: 13 February 2025.
Edited by:
Fernando Baquero, Ramón y Cajal Institute for Health Research, SpainReviewed by:
Shahper Nazeer Khan, University of Manitoba, CanadaCopyright © 2025 Salgado, Rayner and Ojkic. This is an open-access article distributed under the terms of the Creative Commons Attribution License (CC BY). The use, distribution or reproduction in other forums is permitted, provided the original author(s) and the copyright owner(s) are credited and that the original publication in this journal is cited, in accordance with accepted academic practice. No use, distribution or reproduction is permitted which does not comply with these terms.
*Correspondence: Nikola Ojkic, bi5vamtpY0BxbXVsLmFjLnVr
Disclaimer: All claims expressed in this article are solely those of the authors and do not necessarily represent those of their affiliated organizations, or those of the publisher, the editors and the reviewers. Any product that may be evaluated in this article or claim that may be made by its manufacturer is not guaranteed or endorsed by the publisher.
Research integrity at Frontiers
Learn more about the work of our research integrity team to safeguard the quality of each article we publish.