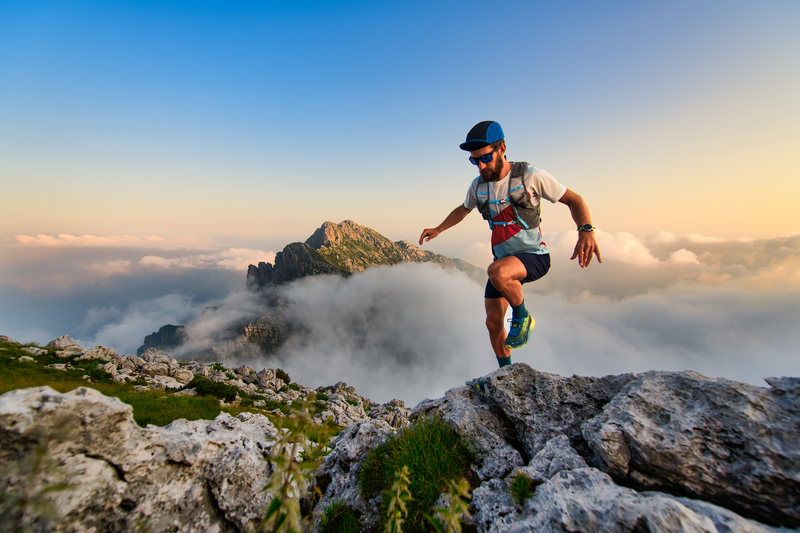
94% of researchers rate our articles as excellent or good
Learn more about the work of our research integrity team to safeguard the quality of each article we publish.
Find out more
REVIEW article
Front. Microbiol.
Sec. Antimicrobials, Resistance and Chemotherapy
Volume 16 - 2025 | doi: 10.3389/fmicb.2025.1528696
This article is part of the Research Topic Bioinformatics approaches to investigate antimicrobial resistance (AMR) in human, animal and environment View all 9 articles
The final, formatted version of the article will be published soon.
You have multiple emails registered with Frontiers:
Please enter your email address:
If you already have an account, please login
You don't have a Frontiers account ? You can register here
Antimicrobial resistance (AMR) presents a critical challenge in clinical settings, particularly among pediatric patients with life-threatening conditions such as sepsis, meningitis, and neonatal infections. The increasing prevalence of multi-and pan-resistant pathogens is strongly associated with adverse clinical outcomes. Recent technological advances in sequencing methods, including metagenomic next-generation sequencing (mNGS), Oxford Nanopore Technologies (ONT), and targeted sequencing (TS), have significantly enhanced the detection of both pathogens and their associated resistance genes. However, discrepancies between resistance gene detection and antimicrobial susceptibility testing (AST) often hinder the direct clinical application of sequencing results. These inconsistencies may arise from factors such as genetic mutations or variants in resistance genes, differences in the phenotypic expression of resistance, and the influence of environmental conditions on resistance levels, which can lead to variations in the observed resistance patterns. Machine learning (ML) provides a promising solution by integrating large-scale resistance data with sequencing outcomes, enabling more accurate predictions of pathogen drug susceptibility. This review explores the application of sequencing technologies and ML in the context of pediatric infections, with a focus on their potential to track the evolution of resistance genes and predict antibiotic susceptibility. The goal of this review is to promote the incorporation of ML-based predictions into clinical practice, thereby improving the management of AMR in pediatric populations.
Keywords: Next-generation sequencing, Oxford Nanopore Technologies, machine learning, antimicrobial resistance, Pediatrics
Received: 15 Nov 2024; Accepted: 21 Feb 2025.
Copyright: © 2025 Zou, Tang, Qiao, Wang and Zhang. This is an open-access article distributed under the terms of the Creative Commons Attribution License (CC BY). The use, distribution or reproduction in other forums is permitted, provided the original author(s) or licensor are credited and that the original publication in this journal is cited, in accordance with accepted academic practice. No use, distribution or reproduction is permitted which does not comply with these terms.
* Correspondence:
Haiyang Zhang, Department of Pediatrics, West China Second University Hospital, Sichuan University, Chengdu, China
Disclaimer: All claims expressed in this article are solely those of the authors and do not necessarily represent those of their affiliated organizations, or those of the publisher, the editors and the reviewers. Any product that may be evaluated in this article or claim that may be made by its manufacturer is not guaranteed or endorsed by the publisher.
Research integrity at Frontiers
Learn more about the work of our research integrity team to safeguard the quality of each article we publish.