- 1Key Laboratory of Sichuan Province for Bamboo Pests Control and Resource Development, Leshan Normal University, Leshan, China
- 2College of Life Science, China West Normal University, Nanchong, China
- 3Department of Agricultural Engineering, Khwaja Fareed University of Engineering and Information Technology, Rahim Yar Khan, Pakistan
Global climate warming has led to changes in the suitable habitats for fungi. Colletotrichum acutatum, a common fungus causing anthracnose disease, is widely distributed in southern China. Currently, research on the relationship between C. acutatum and environmental warming was limited. In this study, MaxEnt and ArcGIS software were used to predict the suitable habitats of C. acutatum under current and future climate conditions based on its occurrence records and environmental factors. The optimal MaxEnt model parameters were set as feature combination (FC) = lp and regularization multiplier (RM) = 2.6. Bio15, Bio12, Bio09, and Bio19 were identified as the main environmental factors influencing the distribution of C. acutatum. Under current climate conditions, C. acutatum was distributed across all continents globally, except Antarctica. In China, C. acutatum was primarily distributed south of the Qinling-Huaihe Line, with a total suitable area of 259.52 × 104 km2. Under future climate conditions, the potential suitable habitat area for C. acutatum was expected to increase and spread towards inland China. The results of this study provided timely risk assessment for the distribution and spread of C. acutatum in China and offer scientific guidance for monitoring and timely controlled of its distribution areas.
1 Introduction
Anthracnose is a significant fungal disease that commonly occurs in plants and is mainly caused by species of the genus Colletotrichum (Cannon et al., 2012). Anthracnose often occurs on the leaves, stems, flowers, and fruits of plants. In the early stages of the disease, small spots typically appear on the infected areas, which later expand into dark brown circular lesions. In severe cases, the disease can cause the plant’s crown and stems to rot, leading to wilting and death of seedlings (Cannon et al., 2012). Colletotrichum acutatum is a common species within the genus Colletotrichum that can parasitize many plants and cause anthracnose. Initially, this fungus was isolated by researchers from diseased tissues of papaya, chili peppers, and delphiniums in Australia (Baroncelli et al., 2015). Nowadays, it is commonly found on tropical and subtropical crops such as strawberries, mangoes, citrus fruits, peach trees, avocados, bananas, coffee, and cereals (Thao et al., 2023). The conidia produced by C. acutatum are primarily spread through rainfall and wind (Dowling and Schnabel, 2020). When the conidia land on injured host plant tissue, they can cause secondary infections in the plant (Tan et al., 2022). The optimal conditions for C. acutatum to infect plants include high humidity, warm temperatures, wounds or natural openings on the plants, and susceptible or weakened plant varieties. The pathogen is most active in warm environments between 20°C and 30°C, where its spores rapidly germinate under high humidity or in the presence of water droplets on leaves. The spores then enter the plant tissue through wounds or stomata, leading to infection. When these conditions are present simultaneously, plants are more susceptible to infection by C. acutatum, resulting in severe disease outbreaks (Ruvishika Shehali et al., 2021). The high transmissibility and infectivity of C. acutatum make it a significant threat to the growth and yield of many fruit trees and crops, leading to decreased agricultural production and impacting the healthy development of the agricultural economy. Research has shown that there are both dominant and recessive resistance genes in C. acutatum. For example, the resistance of green pepper to the disease is controlled by a pair of recessive genes, while the resistance of mature fruit pepper is controlled by a pair of recessive genes (Mahasuk et al., 2009). In strawberry species, resistance to this fungus is a quantitative trait, with a dominant gene controlling high levels of resistance and a micro-effective gene controlling moderate levels of resistance (Mahasuk et al., 2009). Given that C. acutatum is sensitive to climate, global climate change could potentially expand its distribution. Therefore, understanding the current and future potential distribution of C. acutatum is crucial for preventing further infections and protecting crop growth.
Climate is a major factor influencing species distribution patterns. The Fifth Assessment Report (AR5) of the Intergovernmental Panel on Climate Change (IPCC) indicated that the annual mean temperature of the Earth’s surface had increased by 0.85°C over the past 130 years (1880–2012). While the mean temperature of the past 60 years (1951–2012) had increased by 0.72°C (IPCC, 2013). According to researchers’ projections of greenhouse gas emission scenarios, by the end of the 21st century, the global average surface temperature will increase by 0.3 to 4.8°C compared to current levels (Li et al., 2020). Climate change will lead to changes in ecosystems, directly impacting the survival of certain species and affecting the food chains they belong to, thereby further altering the composition of ecosystem patterns. Overall, global warming May accelerate the reproduction of heat-loving species and lead to the decline of cold-loving species (Parmesan and Yohe, 2003). Therefore, quantitative and visual analysis of climate factors affecting species distribution and the current and future potential distribution of species has become a focal point of biological research.
With the rise of statistical techniques and GIS tools, predictive models of species habitat distribution have rapidly emerged in biology and are widely used to forecast the potential distribution of invasive species, endangered species, harmful insects, and more (Gao et al., 2023). These models statistically correlate the geographical distribution of species with current environmental conditions, essentially being static and probabilistic in nature (Guisan and Zimmermann, 2000). Species distribution models (SDMs) encompass a wide range of disciplines, including ecology, biogeography, environmental science, conservation biology, species management, and more (Benavides Rios et al., 2024). Currently, many niche models are used to predict the potential distribution of species, including the bioclimate analysis and prediction system (BIOCLIM) (Ajene et al., 2024), the genetic algorithm for rule set production (GARP) (Townsend Peterson et al., 2007), the ecological niche factor analysis (ENFA) (Cushman et al., 2024), and the Maximum Entropy Model (Maxent) (Zhao et al., 2024). Species distribution models (SDMs) are widely used, but there are still some shortcomings. For example, the sample data for species occurrence May not always be accurate due to inaccuracies in recording or uncertainties in species classification, leading to potential biases in the samples (Elith and Leathwick, 2009). These niche models only consider the interaction between species distribution and environmental factors, lacking consideration for factors such as interactions between species, evolutionary processes within species, and disturbances from extreme conditions (Li et al., 2020). However, niche models remain a common tool for predicting species distributions because they can quickly infer the potential range of a species based on the presence of partial samples, thereby reducing the need for manual field surveys and re-recording processes.
The MaxEnt model, based on the principle of maximum entropy, is an effective and accurate tool for predicting species distributions. Maximum entropy principle serves as a criterion for learning probability models, where the model’s quality is assessed based on the magnitude of entropy; higher entropy indicates a better model (Steven et al., 2006). In general, the maximum entropy principle involves selecting the model with the highest entropy under specified constraints. The MaxEnt model utilizes current species occurrence data and various environmental factors such as soil, climate, and microbial factors to simulate both current and future potential distributions of species. It is known for its effective predictive capabilities, verifiable predictions, fast computation speed, and operational flexibility (Hirzel et al., 2006; Hou et al., 2023). In many studies, this model often operated using default parameters and all available environmental variables that can be collected. These operations increased the complexity of the model, reduced efficiency, and were not suitable for predicting suitable habitats for all species. Research had found that over-parameterized models tended to underestimate the availability of suitable habitats when transferring to new time periods, while under-parameterized models often overestimated it (Dan and Stephanie, 2011). In machine learning algorithms, complex models often led to overfitting, resulting in poor performance when predicting the spatiotemporal changes of species habitats (Zhao et al., 2022). Therefore, adjusting model parameters to reduce complexity and selecting appropriate environmental factors to enhance prediction accuracy was a necessary consideration.
In this study, after optimizing the parameters of the MaxEnt model and selecting environmental variables, the distribution changes of C. acutatum under different climatic conditions were demonstrated. The main objectives of this study were as follows: (1) Explore the suitable MaxEnt parameters and environmental factors for C. acutatum. (2) Investigate the environmental factors that significantly impact the distribution of C. acutatum. (3) Compare the distribution and changes of suitable habitats for C. acutatum under different time periods and economic emission scenarios. The significance of this study lay in providing scientific basis to prevent further spread of C. acutatum, manage anthracnose infections effectively, and enable relevant authorities to implement targeted measures, thereby reducing its impact on agricultural economies.
2 Materials and methods
2.1 Species occurrence data
The current distribution data of C. acutatum mainly came from the Global Biodiversity Information Facility (GBIF, https://www.gbif.org), supplemented with analysis of literature sources. Using Google Maps, latitude and longitude data were collected for 234 distribution points of C. acutatum where coordinates were not initially available. To reduce spatial autocorrelation (Marie-Josée, 1999), each grid (5 km × 5 km) was used as a standard, and ENM Tools 1.4 was employed to prune redundant data points, ensuring that each grid cell contains only one distribution point. The spatial resolution used was 2.5 arc-minutes (approximately 4.5 kilometers) (Gao et al., 2023). After pruning the coordinates using ENM Tools 1.4, the reduced dataset of coordinate points was imported into ArcGIS v10.8. In ArcGIS, data points that did not meet the criteria were removed, resulting in a final dataset of 164 distribution points.
2.2 Screening and processing of the environmental variables
Nineteen bioclimatic variables and 3 terrain variables were used to predict the current and future potential distribution of C. acutatum (Table 1). These variables were sourced from the World Climate Database1 and covered the time period from 1970 to 2000. They included spatial resolutions of 10 arc-minutes, 5 arc-minutes, 2.5 arc-minutes, and 30 arc-seconds, with modeling conducted at a resolution of 2.5 arc-minutes. Future climate data were obtained from the CMIP 6 (Coupled Model Intercomparison Project Phase 6) under the Beijing Climate Center Climate System Model (BCC-CSM 2-MR) climate model (Gao et al., 2023). In the study, scenarios from the 2050s (average from 2041 to 2060) and 2070s (average from 2061 to 2080) under SSP1-2.6, SSP3-7.0, and SSP5-8.5 were used for modeling. These scenarios represented different socioeconomic pathways and greenhouse gas emission levels that influenced the distribution of C. acutatum. Due to high correlations among these variables, directly using them in MaxEnt modeling could lead to overfitting. Initially, the environmental variables surrounding the distribution points of C. acutatum were analyzed using the sampling function in ArcGIS 10.8 software. Using SPSS software, a multicollinearity analysis was conducted to calculate the Variance Inflation Factor (VIF) (Ab Lah et al., 2021). This analysis preliminarily filtered out environmental factors with VIF values less than 100. VIF, also known as the reciprocal of tolerance, indicates severe multicollinearity when VIF > 100. Subsequently, ENMTools software was used to compute Pearson correlation coefficients (Figure 1), identifying and removing climate variables with correlation values greater than 0.8 to enhance the model’s accuracy (Wang et al., 2021). Nine environmental variables were retained: Slope, Elev (Elevation), Bio02, Bio03, Bio08, Bio09, Bio12, Bio15, and Bio19 (Table 2).
2.3 MaxEnt model setting and selection
The MaxEnt model calculates the maximum entropy of species distribution by inputting current species occurrence data and environmental variables. This estimation helps to predict the suitable habitat for the species (Steven et al., 2006). The prediction results of the MaxEnt model are related to feature combinations (FC), regularization multiplier (RM), and maximum background points (BC). The model includes five feature types: linear (linear-L), quadratic (quadratic-Q), hinge (hinge-H), product (product-P), and threshold (threshold-T) (Wan et al., 2020). Combining these types of factors results in a total of 31 combinations. The regularization multiplier varies from 0.1 to 4 in increments of 0.1, yielding 40 values. Therefore, combining the feature combinations with the regularization multiplier results in 1240 parameter combinations. The default parameters of the MaxEnt model May not necessarily be suitable for predicting the potential distribution of all species. Muscarella et al. developed an R package called ENMeval to evaluate the combination of model parameters (Muscarella et al., 2014). Using R Studio, based on statistical significance (ROC with 500 iterations), predictive ability (omission rate, OR), and model complexity (AICc), the performance of these 1,240 candidate models was evaluated (Shi et al., 2023). The difference in Akaike Information Criterion corrected for small sample sizes (delta AICc) between the calibrated model and the current model is less than 2, and the omission rates are less than 5% (Zhao et al., 2022). Therefore, this calibrated model parameter combination is considered the optimal parameter combination. Finally, an optimized MaxEnt model was established. 75% of the presence data was randomly allocated as training data, while the remaining 25% was used as testing data. The feature combination included linear and product features, with a regularization multiplier of 2.6 and a maximum of 10,000 background points. The sampling method chosen was non-replacement subsampling, repeated ten times. The final MaxEnt prediction result was obtained by averaging the results of these ten runs. Model performance was evaluated using the Area Under the Receiver Operating Characteristic Curve (AUC and ROC). AUC values range from 0 to 1, with values closer to 1 indicating better predictive performance (Wang et al., 2020).
2.4 MaxEnt model analysis
The prediction results of the MaxEnt model are output in logistic format, where the species habitat suitability is quantitatively assessed on a scale from 0 to 1. Subsequently, these MaxEnt model predictions were imported into ArcGIS 10.8 software for reclassification and visualization. Using the Jenks’ natural break method, the habitat suitability of C. acutatum was classified into four categories: unsuitable (<0.08), low suitability (0.08–0.27), medium suitability (0.27–0.49), and high suitability (0.49–1) (Li et al., 2022). Finally, the ranges of each suitability zone were determined, and the areas of each suitability zone were calculated.
3 Result
3.1 MaxEnt model optimization and accuracy evaluation
In this study, the optimized MaxEnt model showed excellent performance. The default parameters with FC = Auto feature and RM = 1 yielded an AUC value of 0.974 for the output results (Figure 2). After optimization, setting the MaxEnt model parameters to FC = lp and RM = 2.6 resulted in an AUC value of 0.950. The omission rate was 0.073%, and delta AICc was 0. The optimized MaxEnt model demonstrated high predictive performance, indicating that these optimized parameters can be used to predict the distribution of C. acutatum suitable habitats.
3.2 Relationships between the distribution of Colletotrichum acutatum and bioclimatic variables
In these nine environmental variables, Bio15, Bio09, and Bio12 contributed significantly to the MaxEnt model predictions, with a cumulative contribution of 73.9% (Table 3). Among them, Bio15 had the highest contribution at 33.1%. The jackknife test (Figure 3) indicated that Bio15, Bio12, Bio09, and Bio19 had a substantial impact on the distribution of C. acutatum. Therefore, among the environmental factors, precipitation emerged as the primary factor influencing the survival of C. acutatum, with significant contributions from Bio15, Bio12, and Bio19.
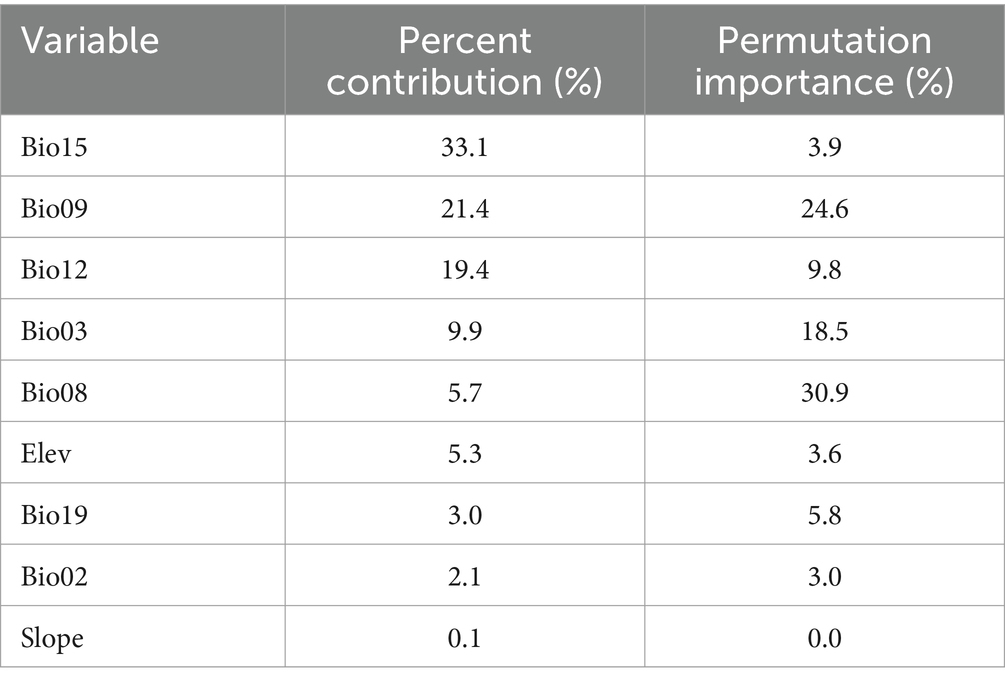
Table 3. Contribution and permutation importance estimation of climate variables in the MaxEnt model of Colletotrichum acutatum.
According to the response curves, areas suitable for C. acutatum survival exhibit seasonal variation in precipitation (Bio15) below 36.36 mm. Additionally, suitable environmental conditions for C. acutatum occurred when the precipitation of the coldest quarter (Bio19) exceeded 304.36 mm, annual precipitation (Bio12) exceeded 1283.76 mm, and the mean temperature of the driest quarter (Bio09) exceeded 11.12°C (Figure 4).
3.3 Potentially suitable distribution areas of Colletotrichum acutatum under current climate
Currently, potential suitable habitats for this fungus have been divided into four grades: high suitability, medium suitability, low suitability, and unsuitable areas. The Figure 5 illustrated the global potential distribution of C. acutatum based on current environmental variables. The results indicated that, except for Antarctica, suitable habitats for C. acutatum were distributed across all other continents. The species’ primary habitats were located in Western Europe, the terrestrial regions of Oceania, Central Africa, coastal areas of South America, the southeastern coast of North America, and Eastern Asia. In Europe, areas suitable for C. acutatum were mainly found in southwestern Germany, the western coastal regions of the UK, most of the western coastal regions of France, and the southwestern coastal regions of Italy. In Oceania, suitable habitats were primarily distributed along the southern coast of Australia and in New Zealand. In South America, the species’ suitable habitats were mainly in southern Brazil, Uruguay, southern Chile, the western coastal regions of Peru, as well as northwestern Ecuador and Colombia. In North America, C. acutatum was predominantly found along the western and eastern coasts of the southeastern United States. In Asia, its distribution was mainly in southern China, Japan, areas bordering India and Myanmar, and coastal regions of Indonesia.
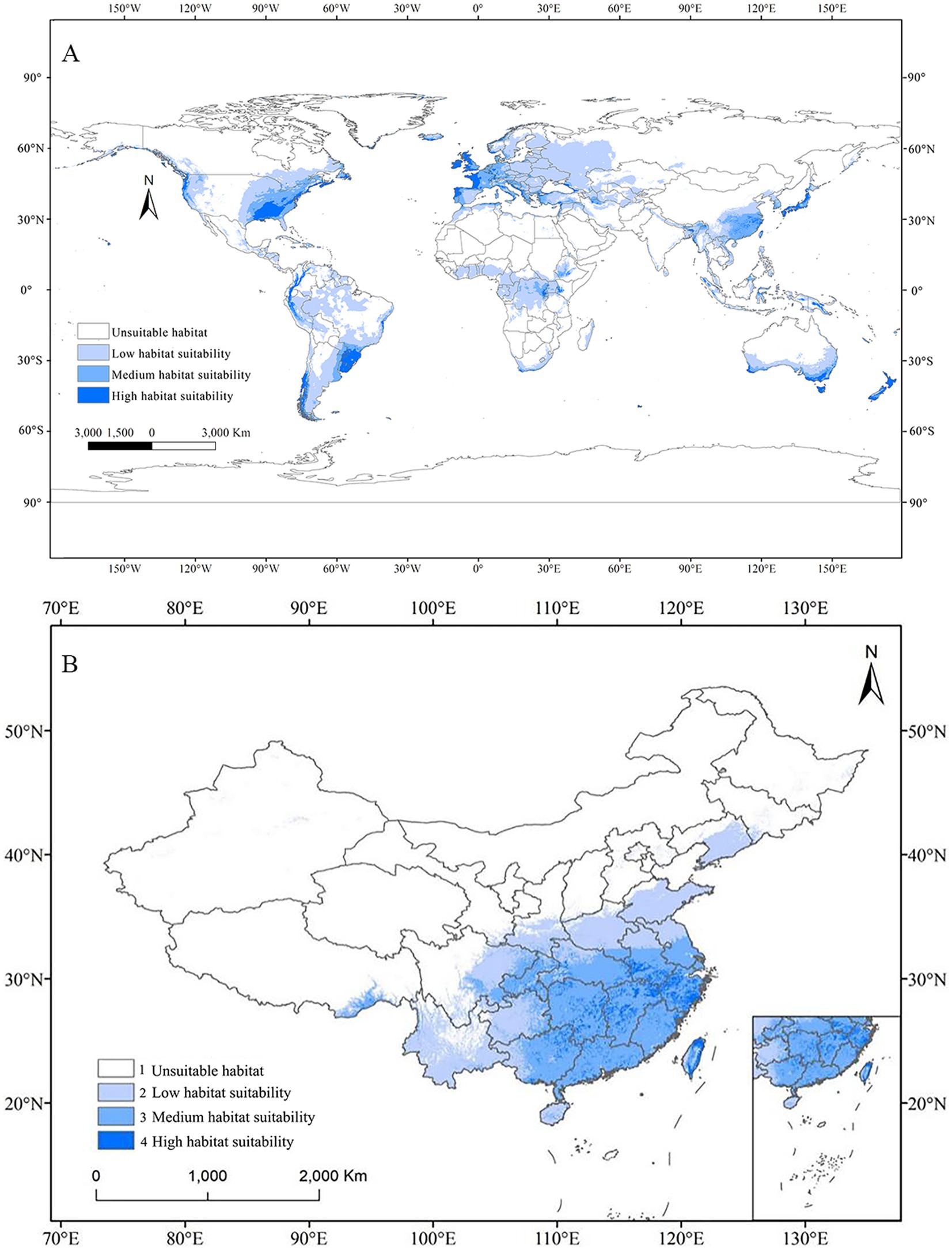
Figure 5. Potential distribution of C. acutatum under current climate scenario by MaxEnt. (A) Global habitat suitability map. (B) Potential habitat suitability map in China.
The total suitable habitat area was the sum of regions classified as highly suitable, moderately suitable, and lowly suitable. In Asia, the largest suitable habitat for C. acutatum was in China, covering 259.52 × 104 km2 (Table 4). Specifically, the highly suitability area covered 16.60 × 104 km2, the medium suitability area covered 121.77 × 104 km2, and the low suitability area covered 121.16 × 104 km2. The highly suitable areas were primarily distributed along the coastal regions south of 30°N, covering relatively small areas, including Zhejiang Province, northern Jiangxi Province, eastern coastal Fujian Province, southern Anhui Province, northeastern Hunan Province, southern coastal Guangdong Province, northeastern Taiwan, and other regions. The medium suitability area was larger and primarily situated south of the Qinling-Huaihe Line. This line in China represented the 800 mm annual precipitation line and the 0°C January isotherm.
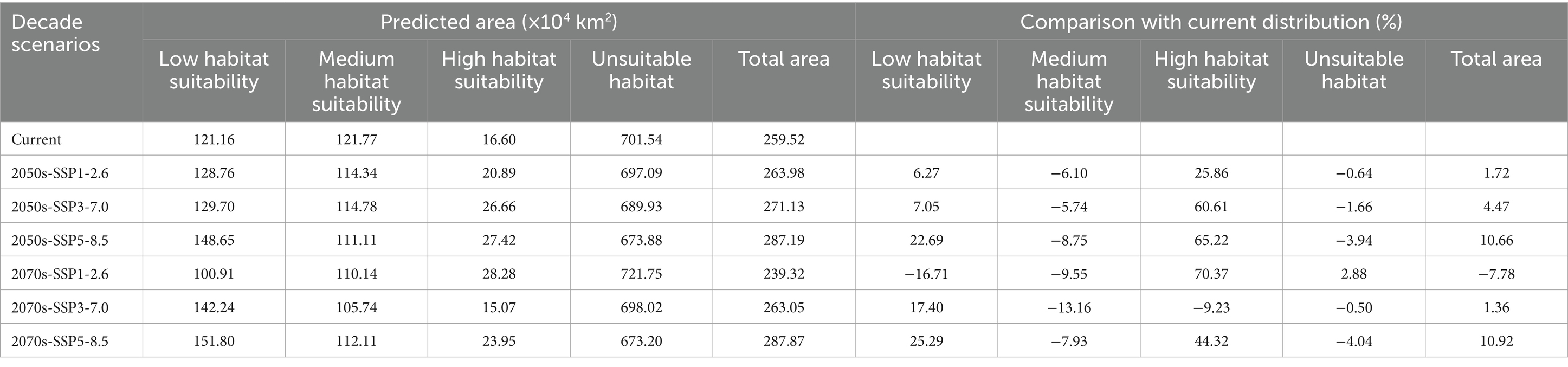
Table 4. Prediction of suitable areas for Colletotrichum acutatum under current and future climatic conditions.
3.4 Change in potentially suitable distribution areas of Colletotrichum acutatum under the future climate
In the future climate scenarios of SSP1-2.6, SSP3-7.0, and SSP5-8.5, the distribution of suitable habitats for C. acutatum in the 2050s and 2070s was predicted. Globally, the suitable habitat for C. acutatum was generally expanding inland. In North America, the suitable habitat in the southeastern United States was extending northwestward. In South America, low-suitability areas in the central part of the continent were becoming unsuitable, showing significant change. In Europe, the suitable habitat was gradually expanding towards the higher latitudes in the north. In Asia, the low-suitability areas in Russia were continuously expanding eastward (Figure 6).
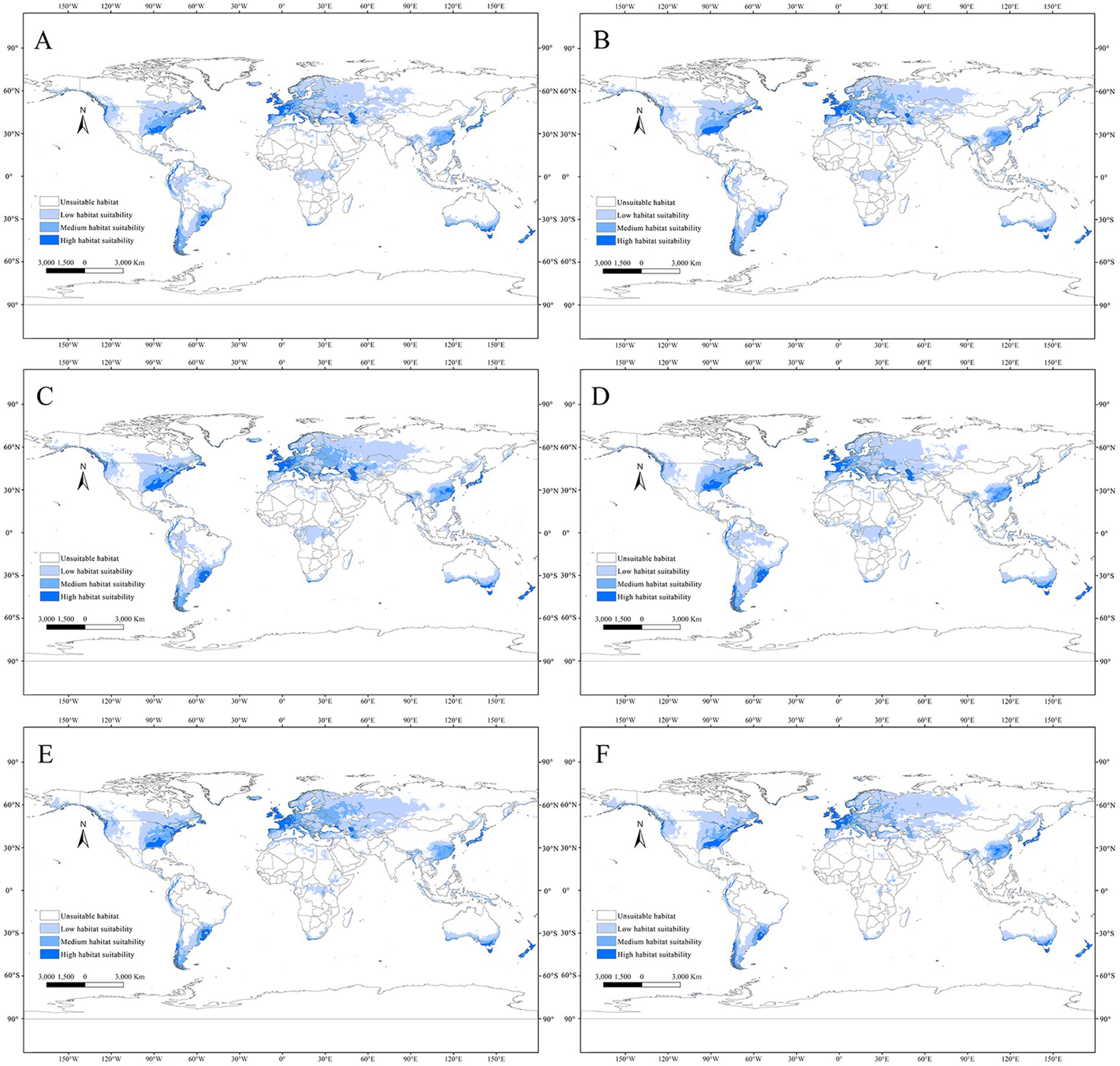
Figure 6. Global potential distribution map for C. acutatum under the future climate scenario. (A) ssp126 in the year 2050; (B) ssp370 in the year 2050; (C) ssp585 in the year 2050; (D) ssp126 in the year 2070; (E) ssp370 in the year 2070; (F) ssp585 in the year 2070.
In China, under most future climate emission scenarios, the area of suitable habitats for C. acutatum was expected to increase (Figure 7). Only in the SSP1-2.6 scenario for the 2070s did the total suitable habitat area decrease, although the high suitability habitat area increased from 16.60 × 104 km2 to 28.28 × 104 km2 (Table 4). Under the SSP5-8.5 emission scenario, this species’ total suitable habitat area increased the most. In the 2050s, the total suitable habitat area expanded to 287.19 × 104 km2, an increase of 10.66%. The high suitability habitat area and low suitability habitat area also increased significantly, by 65.22 and 22.69%, respectively. By the 2070s, the total suitable habitat area had expanded by 10.92%, with the high suitability habitat area expanding by 44.32%.
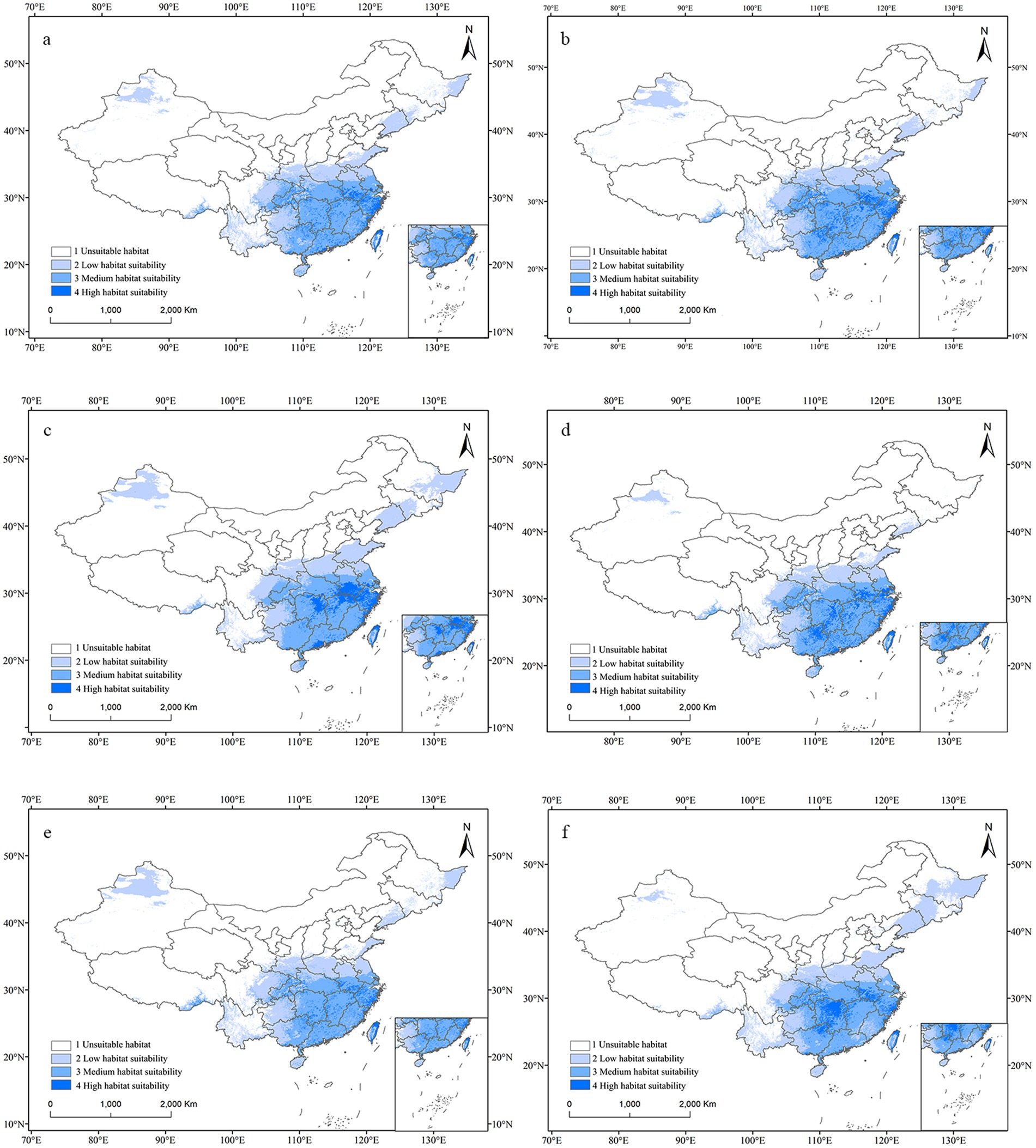
Figure 7. Potential distribution map for C. acutatum in China under the future climate scenario. (A) ssp126 in the year 2050; (B) ssp370 in the year 2050; (C) ssp585 in the year 2050; (D) ssp126 in the year 2070; (E) ssp370 in the year 2070; (F) ssp585 in the year 2070.
In future climate scenarios, C. acutatum showed a trend of shifting towards higher latitudes in northern regions. From the map, it can be observed that the Sanjiang Plain and the Junggar Basin will transition from unsuitable areas to low-suitability areas. The high suitability habitat for this species expanded from the southeast coastal areas inland. Northeastern Jiangxi Province, northeastern Hunan Province, southern Anhui Province, southern Guangdong Province, southeastern Guangxi Zhuang Autonomous Region, and western Zhejiang Province became high suitability habitats for C. acutatum. Inner Mongolia Autonomous Region, Qinghai Province, Hebei Province, Ningxia Hui Autonomous Region, Tianjin Municipality, and Beijing Municipality remained unsuitable areas for this species.
3.5 Centroid changes in potential distribution
In this study, the movement of the distribution centroid of the high suitability habitat of C. acutatum under different climate scenarios was illustrated (Figure 8). Currently, under present conditions, the distribution centroid of the high suitability habitat for C. acutatum was located in Chongren County, Fuzhou City, Jiangxi Province (116°10′46″E, 27°42′33″N). In future scenarios, the centroid of this high suitability habitat generally shifted southwestward, indicating the gradual inland expansion of C. acutatum’s distribution in China. Under SSP1-2.6 and SSP3-7.0 scenarios, the centroid of the high suitability habitat for C. acutatum initially shifted northwestward. Interestingly, for these two emission scenarios, there was a segment of the centroid’s shifting trajectory that overlaps. In the SSP1-2.6 scenario, by the 2070s, the centroid shifted southwestward and reaches Jishui County, Ji’an City, Jiangxi Province (115°9′42″E, 27°8′56″N). Under the SSP5-8.5 scenario, the centroid of the high suitability habitat for C. acutatum showed the greatest degree of displacement. Initially, it shifted northeastward to Guixi City, Yingtan City, Jiangxi Province (116°10′46″E, 27°42′33″N), and then southwestward to Liuyang City, Changsha City, Hunan Province (113°53′40″E, 28°5′58″N).
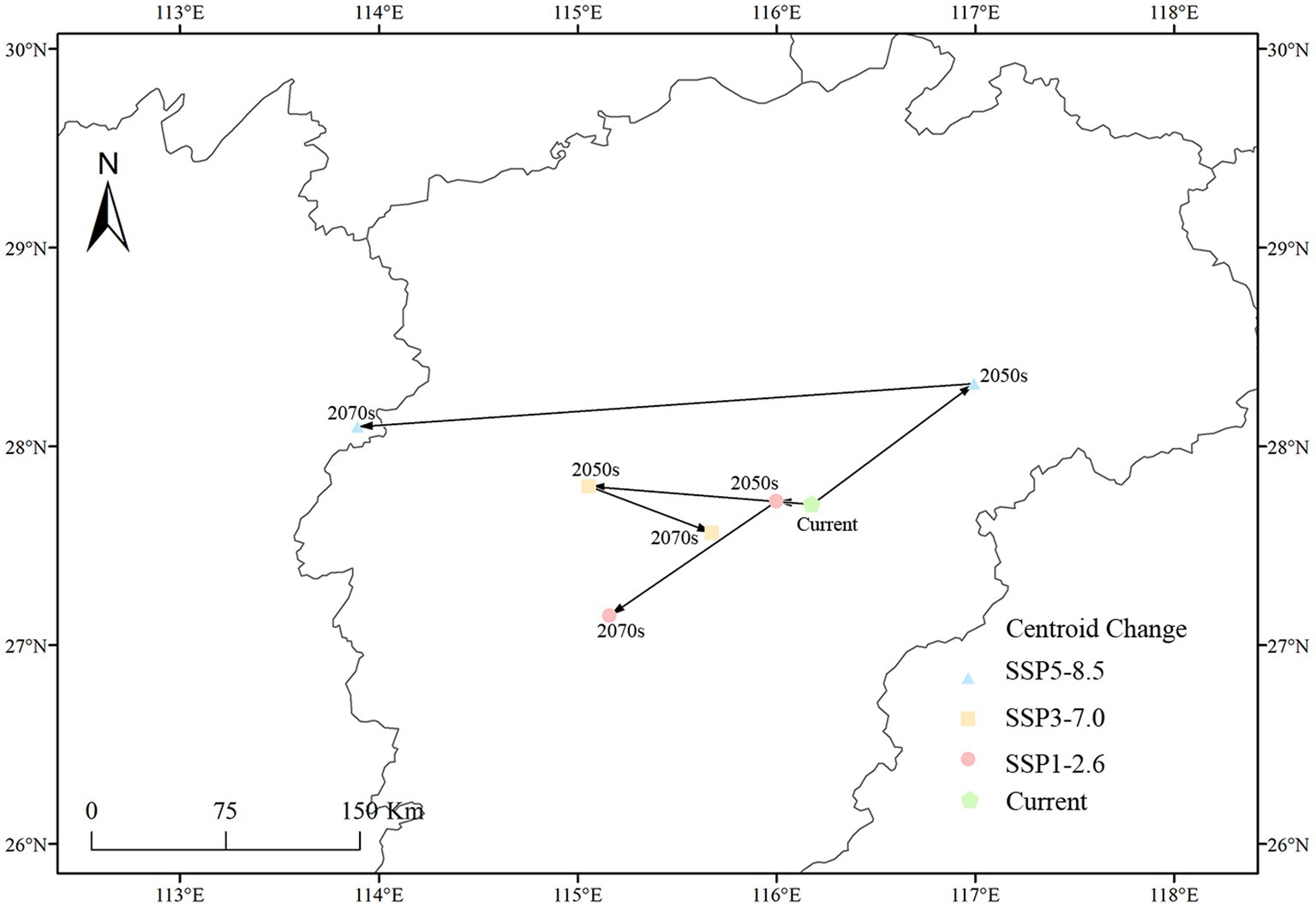
Figure 8. Changes in the centroid of the potential highly suitable distribution of C. acutatum in China.
4 Discussion
Species distribution modeling is a model tool based on species occurrence data and environmental variables to predict the potential geographic distribution of species under specific spatiotemporal conditions. It is a hot topic in current biological research. The relationship between species distribution and the environment is intricate and complex (Zhao et al., 2022). For different research objectives, ensuring the accuracy of results requires selecting appropriate models and parameters. The main goal of this study is to predict the current and future potential distribution of a species using known species occurrence points.
In recent years, the MaxEnt model has gained popularity as a robust ecological niche model capable of accurately predicting species distributions. When predicting the range of species distributions, this model assumes that species exist in all areas where environmental conditions are suitable and do not exist in unsuitable areas (Li et al., 2020). The research indicates that under known conditions, the larger the entropy of a species, the closer the predicted results are to reality (Steven et al., 2006). The default parameters of MaxEnt were initially derived by developers through testing known species distribution points and distribution areas (Steven and Miroslav, 2008). Researchers test optimal model parameters through extensive species distribution data and experimental designs to simplify the settings of the MaxEnt model to the greatest extent possible. The accuracy of MaxEnt’s default parameters can be evaluated by comparing the predicted results obtained through software computations with actual observations (Hirzel et al., 2006). However, when used to predict future species distribution ranges, these results cannot be verified. Moreover, using only default parameters for computation can lead to overfitting and reduce the accuracy of the model results (Aleksandar and Robert, 2014). Therefore, when using the MaxEnt model to predict species’ future distributions, it is necessary to reduce the model’s omission rate to improve prediction accuracy (Muscarella et al., 2014). In this study, based on occurrence data and environmental variables of C. acutatum, the parameters of the MaxEnt model were adjusted. After adjustment, the model’s omission rate was 0.073%, which is less than 5%, and the delta AICc was 0. These adjusted parameters are suitable for predicting the current and future distribution of C. acutatum.
The predictions of the MaxEnt model depend not only on its parameter settings but also on the selection of species distribution and environmental factors. Therefore, to ensure the accuracy of the MaxEnt model input data, species distribution data are collected as extensively as possible. This helps to avoid sample bias caused by insufficient species occurrence points. Generally, the selected species distribution points should adequately cover the species’ distribution range while avoiding biases caused by overfitting (Wisz et al., 2008). Therefore, in this study, the distribution data of this species were filtered to retain only one distribution point per grid. Using ArcGIS software, the distribution range of these points was validated, and only the valid distribution points were retained. Secondly, environmental factors were selected using VIF, Pearson correlation coefficients, and contribution rates, resulting in nine environmental factors being included in the MaxEnt model construction. In this research, AICc values were used to select the optimal combination of feature types and regularization multipliers, thereby reducing the model’s complexity. AUC is an important model evaluation metric in machine learning, widely used to assess the accuracy of MaxEnt model results. The AUC value in this MaxEnt model was 0.95, indicating reliable predictive results. Huercha et al. used the MaxEnt model to predict the distribution of Dermacentor marginatus and found the prediction results to be reliable (Huercha et al., 2020). In this study, relying on bioclimatic variables, the current and future potential distribution of C. acutatum was predicted. This research identifies potential risk areas for future invasion of C. acutatum using the MaxEnt model in the 2050s and 2070s.
C. acutatum is a globally widespread plant pathogenic fungus. It is primarily distributed in coastal areas between 20°N-70°N and 30°S-60°S. These regions are mainly characterized by temperate oceanic climates, subtropical monsoons, humid subtropical climates, and temperate continental climates. The abundant rainfall and relatively high temperatures in these areas create favorable conditions for the reproduction and survival of C. acutatum. The main regions in China where anthracnose disease occurs are concentrated in the middle and lower reaches of the Yangtze River Plain and the southeastern hills, which is consistent with the predictions of this study (Huanhuan, 2023). Our results indicated that precipitation played a crucial role in forming suitable habitats for C. acutatum, and the mean temperature of the driest quarter was also an important influencing factor. In China, the Qinling-Huaihe Line is a geographical boundary in China that distinguishes the northern and southern regions. There are significant differences in climate characteristics, geographical landscapes, and other aspects on both sides of this line. This line separates the humid and semi-humid areas, temperate and subtropical zones, and subtropical monsoon climate and temperate monsoon climate. In winter, south of the Qinling-Huaihe Line is mild and less rainy, while north of the line is cold and dry (Sunan and Chengyuan, 2024). According to the predictions, the suitable habitat of C. acutatum was distributed south of the Qinling-Huaihe Line, where the annual precipitation exceeds 800 mm, winter temperatures are relatively high, and there is minimal freezing. Research indicated that rainfall contributed to the reproduction and spread of C. acutatum, increasing both the infection rate and the amount of infection in plants (Mckay et al., 2014). Therefore, this MaxEnt model accurately predicted the environmental factors that affect the survival of C. acutatum.
This study demonstrated that future climate change conditions favored the survival of C. acutatum. The spread of C. acutatum in its distribution areas will pose extensive risks to crops and fruit trees. This study predicted the distribution of C. acutatum under three socioeconomic pathways until the 2070s, providing more scenarios than previous research. In China, C. acutatum was mainly distributed in southeastern coastal regions such as Zhejiang, Jiangxi, Guangdong, Taiwan, Anhui, and others. These provinces are characterized by flat terrain, proximity to the ocean, high summer temperatures with humidity, significant precipitation, and short duration of low winter temperatures, which meet the environmental requirements for C. acutatum habitat. Under future environmental conditions, there was a trend for C. acutatum habitat to shift inland in China, with the greatest movement observed under the SSP5-8.5 scenario. This indicated a significant impact of high carbon emissions on C. acutatum. Within a certain range, higher future carbon dioxide emissions will increasingly favor the survival of this species. Studies have shown that as the global climate warms, the atmosphere’s ability to hold water increases, leading to a continued strengthening of the global water cycle, primarily expressed in the increase in total precipitation and the intensification of precipitation extremes (Zhang et al., 2021). These extreme weather changes will lead to an increase in plant disease rates and facilitate the spread of C. acutatum.
The southern regions of China are generally suitable for the survival of C. acutatum, so enhancing its prevention and control is of utmost importance. First and foremost, it is crucial to strengthen the prevention and control system, increase publicity on the damage caused by anthracnose and control measures, raise awareness among relevant personnel, and promptly detect and treat the disease. Secondly, optimize cultivation management by selecting suitable sites and trees, and timely prune dead branches and leaves to maintain good ventilation between plants (de los Santos Garcı́a de Paredes and Romero Muñoz, 2002). Choosing disease-resistant varieties can help reduce the risk of fungal infection to some extent. Upon discovering C. acutatum infection in plants, immediately prune and burn the infected parts to prevent the spread and dissemination of the pathogen. Lastly, when the disease becomes severe, chemical control is often effective. When using chemical control methods, it is advisable to alternate the use of fungicidal pesticides to prevent the development of resistance in the pathogens (Peres et al., 2002).
5 Conclusion
In summary, globally, under future climate conditions, the suitable habitat for C. acutatum expanded further inland, with rainfall and temperature being the main environmental factors limiting its distribution. In China, according to the prediction results, areas south of the Qinling Mountains and east of the Huai River were likely to become suitable habitats for this fungus. Given its wide distribution and ease of spread, C. acutatum poses significant challenges to the control of anthracnose. Therefore, relevant authorities should increase their attention to the prevention and control of C. acutatum, enhance monitoring efforts, and implement measures to mitigate its impact on agricultural development.
Data availability statement
The datasets presented in this study can be found in online repositories. The names of the repository/repositories and accession number(s) can be found at: https://doi.org/10.6084/m9.figshare.26175430.v1 and https://doi.org/10.15468/dl.6a554m (GBIF).
Ethics statement
The manuscript presents research on animals that do not require ethical approval for their study.
Author contributions
CF: Data curation, Methodology, Software, Writing – review & editing. YP: Formal analysis, Software, Writing – original draft. FY: Formal analysis, Writing – original draft. ZH: Investigation, Data curation. HA: Data curation, Formal analysis, Writing – review & editing. DX: Conceptualization, Data curation, Methodology, Supervision, Writing – review & editing.
Funding
The author(s) declare that financial support was received for the research, authorship, and/or publication of this article. This research was supported by the Fundamental Research Funds of China West Normal University (20A007, 20E051, 21E040, and 22kA011).
Conflict of interest
The authors declare that the research was conducted in the absence of any commercial or financial relationships that could be construed as a potential conflict of interest.
Publisher’s note
All claims expressed in this article are solely those of the authors and do not necessarily represent those of their affiliated organizations, or those of the publisher, the editors and the reviewers. Any product that may be evaluated in this article, or claim that may be made by its manufacturer, is not guaranteed or endorsed by the publisher.
Footnotes
References
Ab Lah, N. Z., Yusop, Z., Hashim, M., Mohd Salim, J., and Numata, S. (2021). Predicting the habitat suitability of Melaleuca cajuputi based on the MaxEnt species distribution model. Forests 12:1449. doi: 10.3390/f12111449
Ajene, I. J., Heya, H., and Khamis, F. M. (2024). Evaluating the mitochondrial genomic diversity, global distribution and niche overlap of two invasive Phthorimaea species. Heliyon 10:e29010. doi: 10.1016/j.heliyon.2024.e29010
Aleksandar, R., and Robert, A. (2014). Making better Maxent models of species distributions: complexity, overfitting and evaluation. J. Biogeogr. 41, 629–643. doi: 10.1111/jbi.12227
Baroncelli, R., Sarrocco, S., Zapparata, A., Tavarini, S., Angelini, L. G., and Vannacci, G. (2015). Characterization and epidemiology of Colletotrichum acutatum sensu lato (C. Chrysanthemi) causing Carthamus tinctorius anthracnose. Plant Pathol. 64, 375–384. doi: 10.1111/ppa.12268
Benavides Rios, E., Sadler, J., Graham, L., and Matthews, T. J. (2024). Species distribution models and island biogeography: challenges and prospects. Glob. Ecol. Conserv. 51:e02943. doi: 10.1016/j.gecco.2024.e02943
Cannon, P. F., Damm, U., Johnston, P. R., and Weir, B. S. (2012). Colletotrichum – current status and future directions. Stud. Mycol. 73, 181–213. doi: 10.3114/sim0014
Cushman, S. A., Kilshaw, K., Campbell, R. D., Kaszta, Z., Gaywood, M., and Macdonald, D. W. (2024). Comparing the performance of global, geographically weighted and ecologically weighted species distribution models for Scottish wildcats using GLM and random Forest predictive modeling. Ecol. Model. 492:110691. doi: 10.1016/j.ecolmodel.2024.110691
Dan, L. W., and Stephanie, N. S. (2011). Ecological niche modeling in Maxent: the importance of model complexity and the performance of model selection criteria. Ecol. Appl. 21, 335–342. doi: 10.1890/10-1171.1
de los Santos Garcı́a de Paredes, B., and Romero Muñoz, F. (2002). Effect of different fungicides in the control of Colletotrichum acutatum, causal agent of anthracnose crown rot in strawberry plants. Crop Prot. 21, 11–15. doi: 10.1016/S0261-2194(01)00054-0
Dowling, M., and Schnabel, G. (2020). Understanding plant diseases using art and technology. Int. J. Fruit Sci. 20, 959–966. doi: 10.1080/15538362.2020.1755767
Elith, J., and Leathwick, J. R. (2009). Species distribution models: ecological explanation and prediction across space and time. Annu. Rev. Ecol. Evol. Syst. 40, 677–697. doi: 10.1146/annurev.ecolsys.110308.120159
Gao, R., Liu, L., Zhao, L., and Cui, S. (2023). Potentially suitable geographical area for Monochamous alternatus under current and future climatic scenarios based on optimized MaxEnt model. Insects 14:182. doi: 10.3390/insects14020182
Guisan, A., and Zimmermann, N. E. Z. (2000). Predictive habitat distribution models in ecology. Ecol. Model. 135, 147–186. doi: 10.1016/S0304-3800(00)00354-9
Hirzel, A. H., le Lay, G., Helfer, V., Randin, C., and Guisan, A. (2006). Evaluating the ability of habitat suitability models to predict species presences. Ecol. Model. 199, 142–152. doi: 10.1016/j.ecolmodel.2006.05.017
Hou, Z., Sun, Z., du, G., Shao, D., Zhong, Q., and Yang, S. (2023). Assessment of suitable cultivation region for Pepino (Solanum muricatum) under different climatic conditions using the MaxEnt model and adaptability in the Qinghai–Tibet plateau. Heliyon 9:e18974. doi: 10.1016/j.heliyon.2023.e18974
Huanhuan, L. (2023). Identification and population genetic diversity analyses of Colletotrichum species in Camellia oleifera in Jiangxi Province, China : Jiangxi Agricultural University. doi: 10.27177/d.cnki.gjxnu.2023.000147
Huercha,, Song, R., Ma, Y., Hu, Z., Li, Y., Li, M., et al. (2020). MaxEnt modeling of Dermacentor marginatus (Acari: Ixodidae) distribution in Xinjiang, China. J. Med. Entomol. 57, 1659–1667. doi: 10.1093/jme/tjaa063
IPCC (2013). Climate Change 2013 the physical science basis: contribution of Working group I to the fifth assessment report of the intergovernmental panel on climate change. Cambridge, UK: Cambridge University Press.
Li, Y., Li, M., Li, C., and Liu, Z. (2020). Optimized Maxent model predictions of climate change impacts on the suitable distribution of Cunninghamia lanceolata in China. Forests 11:302. doi: 10.3390/f11030302
Li, Y., Shao, W., Huang, S., Zhang, Y., Fang, H., and Jiang, J. (2022). Prediction of suitable habitats for Sapindus delavayi based on the MaxEnt model. Forests 13:1611. doi: 10.3390/f13101611
Mahasuk, P., Taylor, P. W. J., and Mongkolporn, O. (2009). Identification of two new genes conferring resistance to Colletotrichum acutatum in Capsicum baccatum. Phytopathology 99, 1100–1104. doi: 10.1094/PHYTO-99-9-1100
Marie-Josée, F. (1999). Effects of sampling unit resolution on the estimation of spatial autocorrelation. Écoscience 6, 636–641. doi: 10.1080/11956860.1999.11682547
Mckay, S. F., Shtienberg, D., and Sedgle, M. (2014). Anthracnose on almond in Australia: disease progress and inoculum sources of Colletotrichum acutatum. Eur. J. Plant Pathol. 139, 773–783. doi: 10.1007/s10658-014-0431-8
Muscarella, R., Galante, P. J., Soley-Guardia, M., Boria, R. A., Kass, J. M., Uriarte, M., et al. (2014). ENMeval: an R package for conducting spatially independent evaluations and estimating optimal model complexity forMaxentecological niche models. Methods Ecol. Evol. 5, 1198–1205. doi: 10.1111/2041-210X.12261
Parmesan, C., and Yohe, G. (2003). A globally coherent fingerprint of climate change impacts across natural systems. Nature 421, 37–42. doi: 10.1038/nature01286
Peres, N. A. R., Souza, N. L., Zitko, S. E., and Timmer, L. W. (2002). Activity of Benomyl for control of Postbloom fruit drop of Citrus caused by Colletotrichum acutatum. Plant Dis. 86, 620–624. doi: 10.1094/PDIS.2002.86.6.620
Ruvishika Shehali, J., Chitrabhanu, S. B., and Eleni, G. (2021). Colletotrichum: lifestyles, biology, morpho-species, species complexes and accepted species. Mycosphere 12, 519–669. doi: 10.5943/mycosphere/12/1/7
Shi, X., Wang, J., Zhang, L., Chen, S., Zhao, A., Ning, X., et al. (2023). Prediction of the potentially suitable areas of Litsea cubeba in China based on future climate change using the optimized MaxEnt model. Ecol. Indic. 148:110093. doi: 10.1016/j.ecolind.2023.110093
Steven, J. P., and Miroslav, D. (2008). Modeling of species distributions with Maxent: new extensions and a comprehensive evaluation. Ecography 31, 161–175. doi: 10.1111/j.2007.0906-7590.05203.x
Steven, J. P., Robert, P. A., and Robert, E. S. (2006). Maximum entropy modeling of species geographic distributions. Ecol. Model. 190, 231–259. doi: 10.1016/j.ecolmodel.2005.03.026
Sunan, H., and Chengyuan, H. (2024). Analysis on spatial-temporal variation characteristics of climate in Qinling-Huaihe demarcation zone since 1961. Ecol. Indic. 158:111345. doi: 10.1016/j.ecolind.2023.111345
Tan, Q., Schnabel, G., Chaisiri, C., Yin, L. F., Yin, W. X., and Luo, C. X. (2022). Colletotrichum species associated with peaches in China. J. Fungi 8:313. doi: 10.3390/jof8030313
Thao, L. D., Choi, H., Choi, Y., Mageswari, A., Lee, D., and Hong, S. B. (2023). Re-identification of Colletotrichum acutatum species complex in Korea and their host plants. Plant Pathol. J. 39, 384–396. doi: 10.5423/PPJ.OA.05.2023.0078
Townsend Peterson, A., Papeş, M., and Eaton, M. (2007). Transferability and model evaluation in ecological niche modeling: a comparison of GARP and Maxent. Ecography 30, 550–560. doi: 10.1111/j.2007.0906-7590.05102.x
Wan, J., Wang, R., Ren, Y., and McKirdy, S. (2020). Potential distribution and the risks of Bactericera cockerelli and its associated plant pathogen Candidatus Liberibacter Solanacearum for global potato production. Insects 11:298. doi: 10.3390/insects11050298
Wang, Y., Chao, B., Dong, P., Zhang, D., Yu, W., Hu, W., et al. (2021). Simulating spatial change of mangrove habitat under the impact of coastal land use: coupling MaxEnt and dyna-CLUE models. Sci. Total Environ. 788:147914. doi: 10.1016/j.scitotenv.2021.147914
Wang, G., Wang, C., Guo, Z., Dai, L., Wu, Y., Liu, H., et al. (2020). Integrating Maxent model and landscape ecology theory for studying spatiotemporal dynamics of habitat: suggestions for conservation of endangered red-crowned crane. Ecol. Indic. 116:106472. doi: 10.1016/j.ecolind.2020.106472
Wisz, M. S., Hijmans, R. J., Li, J., Peterson, A. T., Graham, C. H., and Guisan, A. (2008). Effects of sample size on the performance of species distribution models. Divers. Distrib. 14, 763–773. doi: 10.1111/j.1472-4642.2008.00482.x
Zhang, W., Furtado, K., Wu, P., Zhou, T., Chadwick, R., Marzin, C., et al. (2021). Increasing precipitation variability on daily-to-multiyear timescales in a warmer world. Sci. Adv. 7:eabf8021. doi: 10.1126/sciadv.abf8021
Zhao, Y., O’Neill, G. A., Coops, N. C., and Wang, T. (2024). Predicting the site productivity of forest tree species using climate niche models. For. Ecol. Manag. 562:121936. doi: 10.1016/j.foreco.2024.121936
Keywords: species distribution, habitat suitability, climate change, Colletotrichum acutatum , MaxEnt
Citation: Fu C, Peng Y, Yang F, He Z, Ali H and Xu D (2024) Potentially suitable geographical area for Colletotrichum acutatum under current and future climatic scenarios based on optimized MaxEnt model. Front. Microbiol. 15:1463070. doi: 10.3389/fmicb.2024.1463070
Edited by:
George Newcombe, University of Idaho, United StatesReviewed by:
Aurel Maxim, University of Agricultural Sciences and Veterinary Medicine of Cluj-Napoca, RomaniaNedeljko Latinovic, University of Montenegro, Montenegro
Copyright © 2024 Fu, Peng, Yang, He, Ali and Xu. This is an open-access article distributed under the terms of the Creative Commons Attribution License (CC BY). The use, distribution or reproduction in other forums is permitted, provided the original author(s) and the copyright owner(s) are credited and that the original publication in this journal is cited, in accordance with accepted academic practice. No use, distribution or reproduction is permitted which does not comply with these terms.
*Correspondence: Danping Xu, ZGFucGluZ3h1QGZveG1haWwuY29t
†These authors have contributed equally to this work and share first authorship