- 1Grupo de Investigación en Ingeniería Electrónica, Industrial, Ambiental, Metrología GIEIAM, Universidad Santiago de Cali, Cali, Colombia
- 2Grupo de Investigación en Modelado, Análisis y Simulación de Procesos Ambientales e Industriales PAI+, Universidad Autónoma de Occidente, Cali, Colombia
- 3Centro de Investigación de la Caña de Azúcar, CENICAÑA, Cali, Colombia
The increasing reliance on fossil fuels and the growing accumulation of organic waste necessitates the exploration of sustainable energy alternatives. Anaerobic digestion (AD) presents one such solution by utilizing secondary biomass to produce biogas while reducing greenhouse gas emissions. Given the crucial role of microbial activity in anaerobic digestion, a deeper understanding of the microbial community is essential for optimizing biogas production. While metagenomics has emerged as a valuable tool for unravelling microbial composition and providing insights into the functional potential in biodigestion, it falls short of interpreting the functional and metabolic interactions, limiting a comprehensive understanding of individual roles in the community. This emphasizes the significance of expanding the scope of metagenomics through innovative tools that highlight the often-overlooked, yet crucial, role of microbiota in biomass digestion. These tools can more accurately elucidate microbial ecological fitness, shared metabolic pathways, and interspecies interactions. By addressing current limitations and integrating metagenomics with other omics approaches, more accurate predictive techniques can be developed, facilitating informed decision-making to optimize AD processes and enhance biogas yields, thereby contributing to a more sustainable future.
1 Introduction
Fossil fuels are the primary global energy source, substantially contributing to global warming while lacking replenishable capacity (Alengebawy et al., 2022). The rapid increase in residual biomass due to population growth, coupled with the urgent need to transition to renewable energy, has positioned anaerobic digestion (AD) for biogas production as a reliable technology for converting waste into energy (Subbarao et al., 2023; Piadeh et al., 2024).
Compared to solar and wind power, AD provides key advantages: (1) reliable baseload power, as it is not weather-dependent like solar and wind (Götz et al., 2016; Shah et al., 2024); (2) potentially lower investment and operation costs (Thi et al., 2016); (3) higher energy output per area once AD is stabilized (Araoye et al., 2018; Emrani and Berrada, 2024); and (4) flexibility in producing various products and byproducts (thermal and electrical power, vehicular fuel, biomethane, hydromethane, and green hydrogen; Villante and Genovese, 2012; Subbarao et al., 2023).
AD is a successful waste treatment method, reducing greenhouse gas emissions through microbial decomposition. The microbial community (MC) plays a key role in AD’s stability and biogas production, but limited knowledge of their roles and interactions hampers optimization efforts (Zhang et al., 2019b).
This review highlights metagenomics as a game-changer for AD, highlighting the importance of studying MC’s intricate composition, dynamics, and functions (Ghiotto et al., 2024b) to attain more efficient AD operations (Zhao et al., 2024).
This study emphasizes the advantages of metagenomics in understanding microorganisms’ taxonomy and function through innovative tools, showcasing microbiota’s often unnoticed yet crucial role during AD. Metagenomic enhancements allow for more accurate identification and comprehension of microbial ecological fitness, shared metabolic pathways, and interspecies interactions within AD, improving system knowledge (Schwan et al., 2020; Zhao et al., 2024). This review consolidates recent advances in AD research using metagenomics tools, highlighting key findings, addressing challenges, and outlining future research directions. It provides researchers insights to leverage metagenomics advancements, extending AD’s potential for biogas production and guiding future research toward more efficient AD approaches. Deepening the understanding of MC and their responses to reactor operational factors through metagenomics is expected to facilitate decision-making for higher CH4 content (Blades et al., 2017), contributing to the demystification of the AD black box.
2 Biogas composition and importance of the microbial community during AD
Before understanding microbial interactions, it is crucial to comprehend biogas composition and the roles of microorganisms in its production. As shown in Table 1, biogas primarily consists of methane (CH4) and carbon dioxide (CO2), with composition varying based on the substrate used. Substrate-specific levels of fats, carbohydrates, and proteins impact the biogas’ chemical nature and its impurity levels. These impurities include nitrogen (N₂), which dilutes CH4; ammonia (NH₃), inhibits methanogens and corrodes equipment; hydrogen sulfide (H₂S), a toxic compound that degrades biogas quality; oxygen (O₂), which can inhibit methanogens and create explosive mixtures with methane; hydrogen (H₂), an indicator of system imbalances; and carbon monoxide (CO), a toxic gas that can inhibit methanogenic activity at high concentrations.
To fully comprehend the AD process and achieve biogas production, it is also essential to understand the roles of microorganisms and substrates in the four main phases: hydrolysis, acidogenesis, acetogenesis, and methanogenesis (Fedailaine et al., 2015; Xu et al., 2018b). Each phase is facilitated by specialized microbiota (Tijani et al., 2018; Xu et al., 2018b; Table 2).
The initial phase, hydrolysis, can limit the overall process rate (Krishna and Kalamdhad, 2014). During this stage, hydrolytic microbes (Table 2) enzymatically break down complex polymers such as lipids, proteins, and carbohydrates into smaller molecules such as amino acids, sugars, and fatty acids (Bharathiraja et al., 2018; Khanh Nguyen et al., 2021). Cellulose is degraded by organisms such as Clostridium, Cellulomonas, Bacillus, Thermomonospora, Ruminococcus, Bacteroides, Erwinia, Acetovibrio, and Microbispora (Chukwuma et al., 2021), while hemicellulose is targeted by Bacteroides, Ruminococcus (Krishna and Kalamdhad, 2014), and Streptomyces genus, associated with high ratios of metabolite production and biotransformation, even capable of degrading lignocellulose (de Lima Procópio et al., 2012). Lignin degradation is performed by Streptomyces, Sphingomonas, Pseudomonas, Rhodococcus, and Nocardia with Clostridium thermocellum and Caldicellulosiruptor bescii, also breaking lignin down while utilizing sugars(Chukwuma et al., 2021). Organisms such as Acinetobacter sp., K. variicola, Bacillus sp., P. mirabilis, and S. maltophilia are considered lignocellulose degraders (Chukwuma et al., 2021).
Later, hydrolysis products are ingested by acidogenic bacteria to produce short-chain volatile fatty acids (VFAs) and alcohols (Table 2; Ali Shah et al., 2014; Tabatabaei et al., 2020; Zhang X. et al., 2023). In acidogenesis, NH₃ and H₂S have a significant impact since they produce an obnoxious odor associated with system inhibition and gear corrosion (Ali Shah et al., 2014).
During acetogenesis, acids are converted into H2, CO2, and acetic acid through acetogenic bacteria (Table 2; Mehariya et al., 2018; Tabatabaei et al., 2020). Acetic acid is an important product as it can be used by methanogenic organisms to obtain CH4. In this phase, Methanobacterium spp. can break down valeric acid to propionic acid, to be later turned into acetic acid by archaea such as Methanobacterium propionicum (Ali Shah et al., 2014). Moreover, released H2 from acetogenesis can be exploited through a symbiotic relationship (syntrophy) between acetogenic bacteria and autotrophic methane bacteria (Li et al., 2018; Tijani et al., 2018). This stage is accountable for producing approximately 70% of methane, 25% of acetates, and 11% of hydrogen, making acetates a crucial intermediate for AD mechanisms (Ali Shah et al., 2014; Jain et al., 2015).
During methanogenesis, the final stage of conventional AD, acetate, and H2 are converted into CH4 and CO2 by methanogenic, hydrogenotrophic, and acetoclastic archaea (Table 2; Manjusha and Beevi, 2016; Tabatabaei et al., 2020). Approximately 30% of CH4 comes from CO2 reduction by autotrophic methane bacteria, resulting in H2 depletion (Ali Shah et al., 2014). Low pH values from VFAs and significant production of H2S could inhibit methanogenesis (Izumi et al., 2010).
Optimizing biogas production in AD requires a deep understanding of MC structures due to their enormous role in the process. Research should focus on substrate composition, MC dynamics, metabolic networks, and their impact on performance. Metagenomic analysis offers a powerful method for quantifying and identifying ecological niches in complex communities (Zhang Y. et al., 2019; Zhu et al., 2019). Despite its importance, replication is often overlooked owing to cost and time constraints, impacting the robustness of findings in microbial ecology (Prosser, 2010).
3 Metagenomic analysis
Metagenomics is a revolutionary method for exploring microbial ecosystems and uncovering complex microbiological interactions (Taş et al., 2021). Shotgun sequencing typically allows for the analysis of total DNA from a microbiome (Lindner et al., 2024). In AD research, metagenomics focuses on studying genetic material from feedstocks or digestates to identify and examine MCs’ structure, abundance, functionality, and interactions using phylogenetic analysis and DNA sequencing (Bedoya et al., 2019; Zhang et al., 2019a). This information can be correlated with operational factors to reveal insights into biogas production and the essential role of microbiota in AD processes.
Figure 1 illustrates a streamlined metagenomic data analysis workflow, from sample collection to downstream analysis. Sequencing options include short-read (second generation), long-read (3rd generation), or hybrid approaches for improved accuracy and completeness (Chen et al., 2020; Eisenhofer et al., 2024). After sequencing, processing, and quality control, ensure data quality. Taxonomic and functional analysis follows, with amplicon sequencing typically identifying MCs at the genus level, while advanced metagenomics can achieve strain-level resolution in some cases. This broadens perceptions of microbial interactions and ecological dynamics. Advanced metagenomics also reveals novel functions and interactions, surpassing traditional approaches limited to DNA amplification. Downstream analysis includes diversity assessment, differential abundance, and network analysis, resulting in deeper insights into the AD microbiome. These findings can input ML predictive models to increase biogas yields.
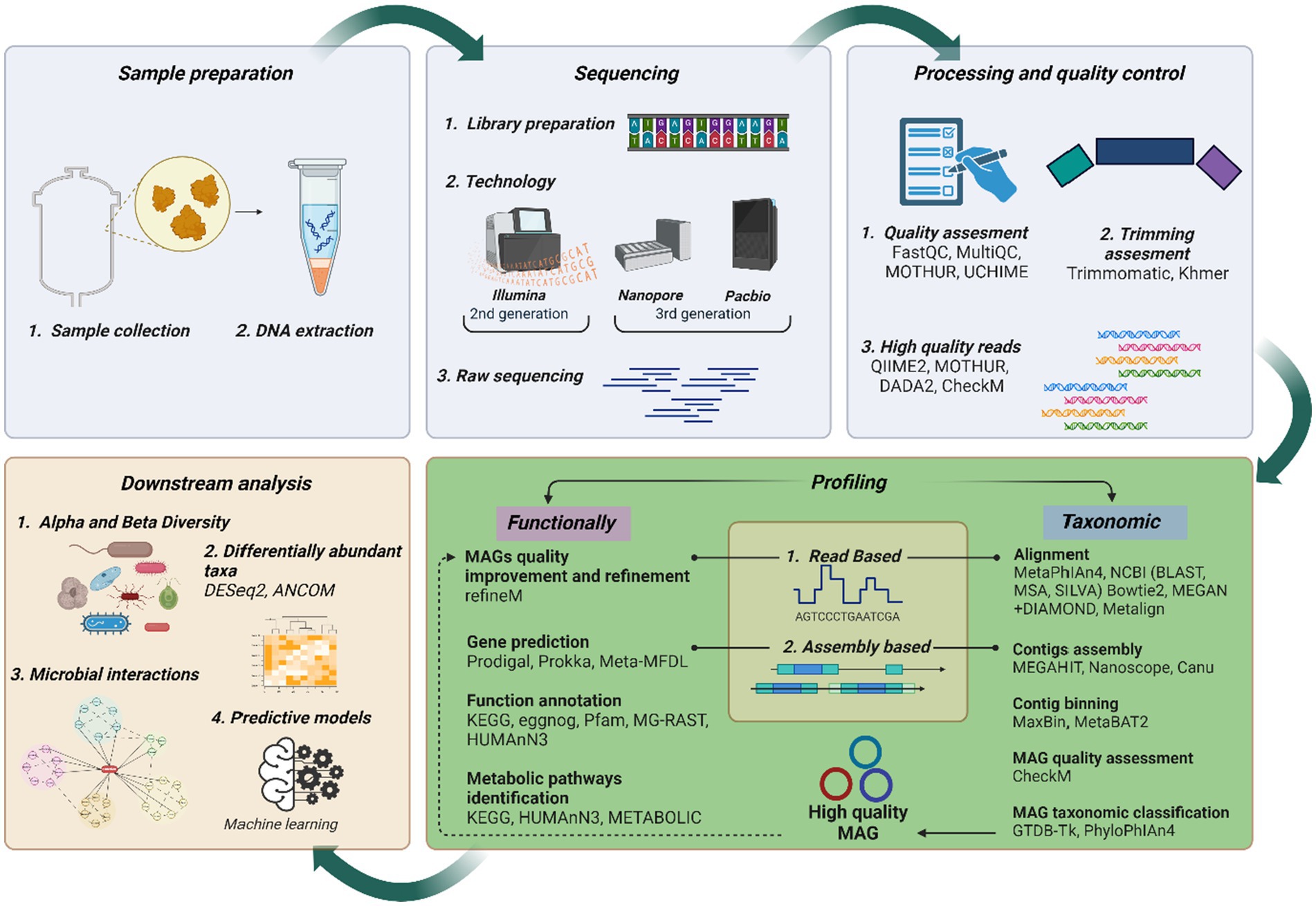
Figure 1. Current metagenomics data analysis flow chart. Created with BioRender.com.
3.1 Sample preparation
This early quality control step aims to minimize false positives. Meticulous sampling and cleaning are crucial to prevent interference during collection. Repeated sampling helps establish consistency and minimizes experimental error.
While no single formula guarantees successful DNA extraction, proper cleaning, lysing, extraction, purification, and quality assessment are needed before metagenomics analysis. Errors in these steps can result in unreliable sequencing. In AD, the MP Biomedicals FastDNA SPIN kit for soil is preferred for its high-quality DNA yield and effective lysis, ensuring accurate microbiome representation. Other commonly used kits include QIAGEN DNeasy PowerSoil Pro, Omega Bio-Tek E.Z.N.A. Soil DNA, and ZymoBIOMICS DNA Miniprep.
Library preparation is essential for DNA sequencing and analysis, permitting the identification and characterization of MCs. Illumina MiSeq and Nextera are widely used systems for this purpose.
3.2 Sequencing technologies
3.2.1 Second generation
Next-generation sequencing (NGS), or second-generation sequencing, works with short DNA reads between 50 and 400 base pairs, simultaneously enabling high-throughput, parallel sequencing of multiple DNA strands.
3.2.1.1 16S rRNA-based metagenomics or metataxonomics
The 16S rRNA gene is a fundamental tool in microbial analysis, traditionally grouped into operational taxonomic units but now clustered into amplicon sequence variants for greater accuracy. As a conserved phylogenetic marker across diverse microbial lineages, it allows for organism clustering, with conservation levels determining taxonomic resolution (Case et al., 2007; Yang et al., 2016). Its conserved nature and cost-effective sequencing make it popular in AD research (Zhang et al., 2019a). Nonetheless, the 16S rRNA gene cannot differentiate closely related species and does not provide functional or metabolic comprehension (Golub et al., 2011). Therefore, advanced metagenomics, transcriptomic (gene expression), proteomics (protein production), and metabolomic (metabolite profiles) are necessary to understand AD microbiome function and metabolism more deeply (Tabatabaei et al., 2020).
Shotgun metagenomic sequencing, which sequences the entire genomic DNA in a sample, provides a comprehensive view of the metagenome and insights into the microbiota’s functional potential (Portik et al., 2022). In contrast, amplifying and sequencing taxonomic marker genes, like the 16S rRNA gene, is better termed “metataxonomic” since it focuses on specific regions rather than the entire genome (Marchesi and Ravel, 2015; Portik et al., 2022; Lindner et al., 2024).
DNA polymerases, which replicate DNA by adding nucleotides to the 3′-end of an existing strand, require primers, short single-stranded nucleic acids, to anneal to complementary sequences on the DNA template, granting starting points (Michael et al., 2015; Abellan-Schneyder et al., 2021). Table 3 lists commercial primers for 16S rRNA gene amplification used in AD microbiota studies, highlighting their strengths and weaknesses. Most primers target the V3-V4 (Cattaneo et al., 2022; Alghashm et al., 2023; Zhao et al., 2023) and V4-V5 (Alghashm et al., 2023; Li et al., 2023; Chen et al., 2024) regions, which are informative for identifying bacteria and archaea.
The following are examples of frequently and recently used sets of primers regarding AD studies: 338F/806R (Li et al., 2022; Alghashm et al., 2023; Wei et al., 2023), 787F/1059R (Mao et al., 2021; Khanthong et al., 2023; Zhang et al., 2024), and 1369F/1492R (Mao et al., 2021; Trego et al., 2021; Zhang et al., 2024).
Primer selection is critical in AD research because potential primer bias can affect MC representation by preferentially amplifying certain sequences. The 338F/806R primer is widely used to capture the diverse microbial participants in AD. Other primers, like 787F/1059R and 1369F/1492R, are also employed but may have limitations, such as insufficient archaea coverage or taxonomic resolution, impacting MC analysis. Therefore, selecting primers should align with the specific research goals and AD systems through validation to ensure accuracy and reliability.
3.2.1.2 Shotgun sequencing
Shotgun sequencing is a leading metagenomic technique for characterizing the genetic composition of complex microbiomes, providing taxonomic and functional insights by analyzing the entire DNA content of an MC. Unlike amplicon sequencing, it allows detailed genome reconstruction through metagenomics assembly and binning, which is beneficial for understanding microbial dynamics.
Traditional reference-based assembly is effective for known organisms but struggles with novel or uncultured microbes common in AD environments. De novo assembly addresses this by reconstructing genomes directly from sequencing data without a reference genome, a significant advance in the field of identifying unknown species.
During assembly, short DNA sequences (reads) are combined into longer contiguous sequences (contigs) and then into scaffolds, often using paired-end reads or long-read data to enhance genome completeness. Binning groups these scaffolds into metagenome-assembled genomes (MAGs), providing detailed insights into microbial functions in AD (Lischer and Shimizu, 2017; Hauptfeld et al., 2024). However, short-read sequences can hinder genome assembly due to limitations in resolving repetitive sequences. Long-read technology inclusion improves genome assembly quality and resolution (Xie et al., 2020; Becker et al., 2023).
Despite its benefits, shotgun sequencing faces challenges such as short read length, missing data, and sequencing errors. Combining reference mapping with de novo assembly could enhance genome reconstruction by leveraging information from related genomes, offering a more robust understanding of microbial processes in AD (Lischer and Shimizu, 2017).
3.2.2 Third generation
Unlike short reads that usually cover a single gene portion, long reads can span multiple genes, making them more effective for sequence alignment and genome matching (Portik et al., 2022). Third-generation sequencing (TGS), such as those by Pacific Biosciences (PacBio) and Oxford Nanopore Technologies (ONT), represent significant advancements in sequencing, generating long reads without PCR amplification, thus reducing bias and improving genome coverage (Xiao and Zhou, 2020; Athanasopoulou et al., 2021). This gives TGS proven advantages over second-generation platforms in analyzing complex MCs.
For instance, in biogas research, TGS facilitates in-depth analysis of MCs within AD systems, which is essential for optimizing biogas production processes. TGS can provide a clear picture of microbial diversity and interactions, aiding in the identification of key microorganisms and their functional roles, which allows for targeted interventions to improve AD systems’ stability and efficiency (Xie et al., 2020).
Even though long TGS reads have accuracy issues, they may provide proper overall taxonomic classification because of the higher information content per read (Brandt et al., 2020). TGS requires careful sequencing library preparation to achieve optimal quantitative metagenomic analysis, enabling researchers to effectively correlate metagenomic data with biogas process operation.
PacBio’s single-molecule real-time technology produces highly accurate long reads, with the Sequel IIe system generating up to 4 million reads in a 30-h run, improving metagenomic assemblies and genome binning, thanks to its advanced data processing capacity, which reduces the computational cost and facilitates faster data transfer (Athanasopoulou et al., 2021). Comparative metagenomic analysis using short and long-read assemblers in AD systems indicates that PacBio long reads improve metagenomic assemblies, enhance gene catalog statistics, refine genome binning, and enhance the functional understanding of microbiomes (Xie et al., 2020).
ONT, which uses protein nanopores embedded in an electrically resistant membrane to sequence single-stranded DNA or RNA molecules (Cuber et al., 2023), has transformed sequencing by introducing pocket-sized devices capable of real-time sequencing in the field, facilitating immediate analysis of environmental samples and potential reactor monitoring (Athanasopoulou et al., 2021). The use of automated, biogas reactor-specific monitoring tools, such as those employing ML, could help predict operational problems (Brandt et al., 2020).
Tools such as LongQC assess TGS data quality, while Canu, Prowler, and Porechop are used for adapter trimming. Minimap2, GraphMap, and BWA-MEM are widely used aligners for TGS data, offering robust performance for aligning long reads (Wick et al., 2017a; Athanasopoulou et al., 2021). Additionally, Flye is effective for genome assembly, while tools such as Quiver, Nanopolish, and Racon are suitable for read corrections and polishing, enhancing the accuracy of TGS-based assemblies (Vaser et al., 2017; Kolmogorov et al., 2019).
The major drawback of these technologies is the high error rate, approximately 15%, observed during sequencing, making TGS less ideal for accurate detection (Athanasopoulou et al., 2021). However, improvements in sequencing chemistry are expected to reduce these errors and enhance accuracy. Despite this, the development of necessary bioinformatics tools and pipelines for TGS data analysis remains challenging, limiting the full exploitation of the vast amount of data produced.
3.2.3 Hybrid sequencing
Hybrid sequencing approaches, which combine short-read and long-read technologies, offer a cost-effective strategy for generating comprehensive genome assemblies, balancing the strengths and weaknesses of each method (Eisenhofer et al., 2024). For instance, the Unicycler pipeline uses Illumina short reads for initial assembly and ONT long reads to close gaps and resolve repeats, often producing complete genome assemblies. These hybrid assemblies are highly accurate and complete, consistent with reference genomes in size and GC content, and capable of producing high-quality MAGs that reveal the functional potential of AD microbiomes, including unknown bacteria and archaea (Chen et al., 2020).
A study by Singleton et al. (2021) combined long and short reads to assemble over 1,000 high-quality MAGs from anaerobic conditions, identifying key functional microbes, such as the novel genus Ca. methylophosphatis. This highlights the importance of raising standards for MAG quality to facilitate the identification and experimental validation of functional microbes while avoiding contamination with low-quality MAGs.
In AD microbiomes, hybrid approaches are becoming increasingly important for analyzing system stability and buffer capacity (Becker et al., 2023). These methods have enabled the identification of potentially new organisms critical to AD processes, such as Syntrophobacteraceae (involved in short-chain fatty acid oxidation), Syntrophomonadaceae (butyrate oxidation), and Methanoculleus (hydrogenotrophic methanogenesis; Becker et al., 2023).
In summary, hybrid sequencing is revolutionizing the field of AD metagenomics by generating high-quality MAGs and deepening the understanding of MC and their functions. As sequencing technologies and computational power continue to advance, these hybrid approaches will further enhance the investigation of AD microbiomes, leading to improved outcomes and expanding microbial knowledge.
3.3 Processing and quality control
After sequencing, data are subjected to rigorous QC and trimming. Tools such as MultiQC, Mothur, FastQc, and UCHIME assess sequencing quality and identify low-quality reads and contamination. Trimming tools such as Trimmomatic remove low-quality bases and adapter sequences, while K-mer-based tools (substrings of constant length k that capture consecutive bases, enabling efficient sequence comparison and analysis) like UCHIME identify and remove chimeric sequences. Trimming must be carefully balanced, as overly aggressive trimming can lead to data loss and less accurate assemblies (Yang et al., 2019; Taş et al., 2021; Häntze and Horton, 2023). The combination of QC and trimming is crucial to avoid biases in taxonomic profiling and functional annotation, as downstream accuracy relies on the input data quality.
Since QIIME2 performs initial QC and trimming, it plays a crucial role in downstream analysis, offering taxonomic and functional profiling tools, diversity analysis, and visualization. It integrates with QC and trimming outputs, allowing for further exploration, and includes plugins for state-of-the-art sequence quality control like DADA2 and Deblur (Bolyen et al., 2019).
Handling large metagenomic datasets can strain resources, but incorporating QIIME2 with cloud platforms like Microsoft Azure, AWS, and Google Cloud poses a scalable solution (Callaghan, 2023). This approach allows researchers to efficiently handle and analyze large datasets, making advanced metagenomic analysis more accessible and manageable.
3.4 Taxonomic profiling
Taxonomic profiling, which predicts the relative abundance of taxa in metagenomic samples, involves read alignment, taxonomic classification, and abundance estimation (Lapierre et al., 2020). In AD, Greengenes and SILVA databases commonly assign taxonomy to sequences after the sequencing run and QC. Although it can be computationally demanding, SILVA is widely used due to its high-quality curation, frequent updates, and comprehensive coverage. Greengenes, while less frequently updated, is also commonly used, particularly for its focus on bacteria and archaea. Alignment maps sequencing reads to reference genomes to identify microbial taxa, annotate functional genes, and ensure data quality. However, incomplete reference databases can limit accuracy, though regular updates like those in the Genome Taxonomy Database (GTDB) help (Parks et al., 2022). The high computational demand of processing large datasets can be optimized using efficient tools like Bowtie2 and BWA (Robinson et al., 2017). Handling divergent sequences is challenging, so tools like HISAT2, Bowtie2, or hybrid approaches are recommended for better accuracy. Metaling, a novel method, balances precision and speed by using containment min hash (a technique that can rapidly estimate dataset similarities via k-mers hashing and identifying overlaps) for pre-filtering, reducing computational cost and enhancing profiling accuracy (Lapierre et al., 2020).
In the following alignment, contig assembly reconstructs microbial genomes to understand their roles in biogas production. Tools like SPAdes (effective for assembling short reads; Bankevich et al., 2012), MEGAHIT (fast and efficient for large datasets; Li et al., 2015), and Canu (designed for long-read data but primarily for single-genome assembly; Koren et al., 2017) are commonly used in AD. For complex metagenomic datasets typically in AD studies, long-read assemblers like Flye and HiCanu may be more suitable (Liu et al., 2023). Assembly merges overlapping sequences into contigs, which are essential for generating high-quality MAGs. Challenges include repetitive sequences, coverage gaps, and assembly errors. Hybrid assembly, combining short-read accuracy with long-read connectivity, improves accuracy and completeness. This method balances high-depth, short-read sequencing with lower-depth, long-read sequencing (Sims et al., 2014; Eisenhofer et al., 2024). Emerging tools like Unicycler and MaSurCA, applying short and long reads, help resolve repetitive sequences and reduce chimeric contigs (Zimin et al., 2013; Wick et al., 2017b; Liu et al., 2023). Notably, MaSuRCA corrects long reads with short reads before assembly, while SPAdes fix errors within the assembly graph, further enhancing accuracy (Zimin et al., 2013).
Contig binning groups of DNA fragments from the same or related genomes is essential for reconstructing MAGs and identifying metabolic pathways. Moreover, it can lead to the discovery of novel species and enzymes (Campanaro et al., 2018; Wang et al., 2024). Without binning, uncultured microbiomes in AD systems would remain unknown, leaving significant gaps in understanding their metabolic capabilities and potential for optimizing biogas production. Binning methods include sequence composition, abundance, and hybrid approaches, with tools like MetaBAT2 and CONCOCT known for high accuracy but requiring significant computational resources (Alneberg et al., 2014; Kang et al., 2019). Maxbin2 is effective with multiple metagenomic datasets and faces computational intensity challenges, employing an Expectation–Maximization algorithm to assign contigs based on tetranucleotide frequency and coverage (Wu et al., 2016). Advanced methods like COMEBin, using contrastive multi-view learning and data augmentation, efficiently recover MAGs, providing deeper perceptions into AD microbiomes (Wang et al., 2024).
Reconstructed MAGs must be checked for completeness, contamination, and errors. CheckM is widely used for assessing MAG quality, balancing accuracy and computational efficiency (Parks et al., 2015). GUNC, a novel tool that utilizes an entropy-based measure of lineage homogeneity across contigs, can infer each gene across different levels, using taxonomy to approximate phylogenetic relationships. GUNC identifies chimerism caused by horizontal gene transfer, improving MAG accuracy in complex environments. However, it is computationally intensive and requires comprehensive reference databases (Orakov et al., 2021). Combining tools such as GUNC, GTDB-Tk, and CheckM refines MAG datasets. Tools like GTDB-Tk and PhyloPhlAn4 provide high-resolution taxonomic classification but demand significant computational resources. GUNC can further refine results, leading to more precise outcomes.
3.5 Functionality profiling
Before initiating functional profiling, additional MAG refinement is recommended to ensure accuracy and avoid the misinterpretation of key metabolic pathways. Refining MAGs increases functional annotation accuracy, improving system interpretability. Tools such as RefineM, which are used alongside CheckM, can redefine boundaries, remove contaminants, and correct binning errors.
High-quality MAGs are ideal for accurate gene prediction. Prodigal is efficient for fast gene prediction but may struggle with fragmented or low-quality MAGs. Alternatives like FragGeneScan, Glimmer-MG, and MetaGeneMark (which employs hidden Markov models) handle sequencing errors and diverse data, while Prokka offers a streamlined annotation pipeline, though less flexible for non-bacterial genomes designed for gene prediction from short reads, effectively accounting for sequencing errors and codon usage (Taş et al., 2021; Yang et al., 2021). Artificial intelligence (AI) applications like Meta-MFDL and CNN-MGP improve accuracy but are computationally intensive and require well-annotated training data, which can be scarce in AD environments (Yang et al., 2021).
Functional microbiomes, interspecies interactions, and versatile metabolic pathways are critical for the microbial degradation processes in AD, which are essential to achieving efficient and targeted operations. Although studies traditionally focused on cultivated microbial members, recent advances in 16S rRNA gene amplicon sequencing, metagenomics, and other omics technologies (metatranscriptomics, metaproteomics, and metabolomics) have expanded knowledge of uncultivated microbes, microbial black holes, niche differentiation, and previously unknown metabolic pathways (Zhang X. et al., 2023), providing a more holistic view of MC function and activity in AD.
Whereas 16S rRNA amplicon sequencing data is conventionally not directly linked to functional potential, improved methods such as PICRUSt2 and Tax4Fun2 can predict microbial function from 16S rRNA, enhancing the ability to infer functional profiles from taxonomic data. However, they still depend on the quality and completeness of reference genomes (Douglas et al., 2020; Wemheuer et al., 2020). Additionally, combining 16S rRNA amplicon sequencing with MAGs has proven beneficial in uncovering abundant, previously undescribed lineages within key functional groups (Singleton et al., 2021), facilitating the identification of novel metabolic pathways and understanding ecological roles.
High-quality MAGs are necessary for exploring complex MCs. Databases like MiDAS connect microbial function with process data, while tools like Prokka and Infernal aid gene identification and annotation (Singleton et al., 2021). KEGG is widely used for mapping metabolic pathways, yet its focus on well-characterized organisms can be limiting. Integrating KEGG with databases like Eggnog and Pfam expands functional annotation with varying specificity (Huerta-Cepas et al., 2019; Palù et al., 2022). Pfam effectively identifies protein families and domains but may miss novel proteins, which can be addressed alongside de novo prediction tools (Coggill et al., 2008).
A recently developed cutting-edge software called METABOLIC is noteworthy for advancing microbial ecology and biogeochemistry studies. It integrates protein annotation from KEGG, TIGRfam, Pfam, custom hidden Markov model databases, dbCAN2, and MEROPS and assesses metabolic pathways through KEGG modules. METABOLIC profiles metabolic and biogeochemical traits and functional networks in MCs based on MAGs. METABOLIC provides protein annotations and metabolic pathway analyses for inferring the contribution of microorganisms, metabolism, interactions, activity, and biogeochemistry at the community scale. This software facilitates the standardization and integration of genome-informed metabolism into models, enabling easier interpretation of system metabolism and biogeochemistry (Zhou et al., 2022).
A feasible choice to enhance the quality and accuracy of genome-scale metabolic models is the amalgamation of MAG data with KEMET, a novel tool that expands KEGG annotations by identifying missing orthologs through hidden Markov models. However, KEMET may be limited by its reliance on high-quality reference genomes, affecting its performance in poorly characterized environments (Palù et al., 2022).
HUMAnN3, an innovative approach for functional profiling and metabolic pathways analysis that combines the MetaCyc database (Beghini et al., 2021; Cortés et al., 2022), can also be used for KEGG orthology enrichment analysis (Chen et al., 2021). A disadvantage is that it is computationally intense and requires extensive reference data.
Identifying metabolic pathways aids the prediction of metabolic and biochemical functional trait profiles across datasets, including MAGs, single-cell amplified genomes, or pure culture genomes (Taş et al., 2021). This knowledge is crucial for optimizing microbial processes in AD operations. Despite these advancements, challenges regarding better computational resources, improved functional annotation methods, and more comprehensive databases remain significant barriers in metagenomic workflow analysis.
3.6 Downstream analysis
In microbiome analysis, alpha and beta diversity metrics assess variation within and between MC. Alpha diversity is measured by species richness (Chao1) and evenness (Shannon index), with the latter being less affected by sequencing depth (Zhang et al., 2022). Rarefaction or normalization ensures accurate sample comparison, determining MC coverage and saturation despite sequencing depth variations (Stolze et al., 2015). Beta-diversity evaluates dissimilarity between MCs using metrics like Bray-Curtis and weighted UniFrac, or qualitative metrics, with ordination techniques like PCoA and PCA for visualization. QIIME, Mothur, and the R package vegan are suitable for computing alpha and beta diversity (Knight et al., 2018).
Multivariate analyses surpass simple correlations by applying sophisticated techniques to capture complex relationships between MCs and environmental variables. Symmetric methods, like canonical correlation analysis, co-inertia analysis, and Procrustes analysis, treat variables equally without distinguishing between explanatory or response roles. Conversely, asymmetric approaches, like redundancy analysis and canonical correspondence analysis, differentiate between explanatory and response variables. Non-metric multidimensional scaling is an exploratory method mainly for visualizing sample similarities or dissimilarities. Methods like generalized linear models and permutation tests assess the significance of multivariate patterns. These analyses rely on detailed metadata, high-dimensional data, and well-structured experiments (Ramette, 2007; Paliy and Shankar, 2016).
Recent methods combine sequencing with microbial cell counts for accurate data, enabling ML approaches like random forest regression to distinguish samples based on metadata. SourceTracker, a Bayesian tool, identifies microbial sources and classifies samples. However, ML analysis demands large datasets and cross-validation to ensure robustness (Knight et al., 2018).
Analyzing alpha- and beta-diversity in AD studies is decisive for understanding MC structure and stability, impacting biogas production. While trained ML models improve data analysis efficiency, they require substantial training data and may struggle with adaptation, highlighting the need for more flexible models with less dependency on extensive data inputs.
3.7 Metagenomics resources used within the AD research field
Selecting appropriate tools for metagenomic analysis is vital, and researchers must match their selections to the specific requirements and resources of their projects. Comprehensive platforms such as MOTHUR, QIIME2, MG-RAST, and MEGAN can manage most of the metagenomic workflow, making them suitable for budgets with limited budgets or simple goals. Moreover, tools such as MOCAT2 and MetaWRAP efficiently cover processes from gene prediction to functional profiling (Taş et al., 2021).
However, these all-in-one solutions may lack the precision and adaptability of more specialized tools, such as SPAdes for genome assembly, Kraken2 for taxonomic classification, and MetaPhlAn for strain-level profiling. Advanced tools such as KEMET, METABOLIC, PICRUSt2, CNN-MPG, and GUNC provide greater accuracy and functionality but require significant computational resources and expertise. The future of metagenomic research may involve the integration of specialized tools into more user-friendly platforms that offer both precision with ease of use.
Table 4 presents a range of methods and tools, both common and novel, used for metagenomic analysis in AD research, spanning from sequencing type selection to functional analysis.
From Table 4, it is evident AD researchers predominantly favor short-read approaches targeting the V3, V4, and V5 regions, likely due to project scope, practical experience, or budget constraints. Short-read sequencing, often paired with Illumina NGS or Nextera XT kits, is cost-effective and scalable, though it may miss novel genomes. In contrast, while more expensive, long-read sequencing delivers high-fidelity genome recovery and deeper microbial insights. Hybrid approaches, which combine the strengths of both methods, balance cost with comprehensive analysis.
Researchers should select sequencing strategies based on specific research needs, whether focusing on 16S rRNA gene regions for targeted studies or whole-genome sequencing for broader analysis. There is a growing interest in AD research in correlating MC, functional annotation, and metabolic pathway identification, aiming to improve biogas yields and produce valuable products such as biomethane, hydromethane, and hydrogen, along with byproducts like organic acids, alcohols, and biofertilizers.
Metagenomics and hybrid sequencing are increasingly preferred for exposing the microbial “black box” of AD systems, which is essential for enhancing biogas production. Tools like MOTHUR for versatile analysis stage and KEGG for functional annotation are popular in AD research. As costs decrease and technologies advance, integrating high-fidelity sequencing with robust bioinformatics is expected to enhance AD process understanding and efficiency. Additionally, metagenomics, along with other omics technologies, has proven effective for uncovering key functional associations and tracking gene expression, demonstrating the power of multi-omics in advancing AD research.
4 Applications of metagenomics in anaerobic digestion research
Operational factors in AD, such as the feeding regime (mono and co-digestion), temperature, reactor type, pH value, substrate choice, organic loading rate (OLR), and hydraulic retention time, can influence MC structure, dynamics, and interactions (Su et al., 2019; Pasalari et al., 2021). Understanding these variations is crucial for informed decisions that lead to more efficient AD systems, a task that is now more feasible thanks to metagenomics analysis.
Among factors influencing AD, the choice of substrate is crucial, as it significantly influences MC. Studies have shown a strong link between changes in MC and the substrate used in both mono-digestion and co-digestion processes. The composition of the feedstock plays a major role in shaping the active microbiota within AD systems (Tsapekos et al., 2017; Zhang et al., 2020a).
Furthermore, concerning reactor configurations, two of the most common setups in biogas are single-stage and two-stage continuous stirred tank reactors. Through metagenomic analysis, different microorganisms and their syntrophic relationships within a reactor can be unraveled, aiding in understanding how these factors can influence community structure, interactions, and overall process efficiency (Treu et al., 2016). For instance, using dairy wastes in a two-stage reactor, (Fontana et al., 2018) identified that certain microorganisms were predominant in the first (acidogenic) reactor, while others were more active in the second (methanogenic) reactor. This niche differentiation through metagenomics demonstrated that a two-stage reactor could benefit methane production and how MC can influence the AD process.
Moreover, temperature also plays a significant role in shaping microbial ecology. When comparing mesophilic and thermophilic biogas plants, the use of Genome Centric Metagenomics (GCM) was crucial because it allowed a more detailed examination of communities at the species level, providing insights into specific roles of individual microorganisms in a complex MC (Campanaro et al., 2018). In this study, thermophilic microorganisms were more efficient in breaking down complex organic compounds into methane than mesophilic ones. The Genome Centric Metagenomic (GCM) approach allowed us to understand how these temperature-dependent microorganisms contribute to the overall efficiency and stability of the AD process.
Previous examples demonstrate that the application of metagenomics in AD research has significantly enhanced the understanding of MC and their functional roles in the AD process. By providing exhaustive knowledge into the abundance, diversity, and interactions of microorganisms, as well as identifying keystone species and syntrophic relationships, metagenomics has paved the way for improved analysis and decision-making in AD systems. Likewise, evaluating the impact of varied inoculums on long-term anaerobic yield (Peces et al., 2018) suggested that achieving a stable process requires establishing and maintaining a desirable MC composition through careful planning and optimization of operational conditions. This knowledge can be leveraged to optimize process conditions and enhance microbial activity to increase biogas yield and process efficiency. Thus, in the following sections, the microbiota composition in relation to different substrates in the two feeding regimes, mono- and co-digestion in AD, will be explored.
4.1 Microbial community composition during mono-digestion
Understanding the MC is fundamental for optimizing AD. In mono-digestion, a single type of substrate is used as the sole feedstock for biogas production. Some common single substrates include pig manure, rice straw, corn stalk, or silage. These last two are differentiated, with the first consisting of plant residues that remain after harvest and silage referring to the fermented forage from the entire plant.
The substrate choice, carbon-to-nitrogen ratio (C/N), and total solids content are crucial factors affecting the OLR. High total solids content may require inconveniently large water additions, while a low C/N ratio might necessitate adjusted feeding strategies to avoid digester overload and process imbalances due to acidification or VFA accumulation (Pore et al., 2016). A feeding regime is essential to maintain a stable MC, ensuring they have sufficient time to adapt and function effectively.
An analysis was conducted using data from different studies under mesophilic conditions to understand the relative abundance of the MC using different substrates and their relative C/N ratio, TS%, CH4% content, and CH4 yield. Each study focused on a different substrate and described the MC abundance through a 16S rRNA gene metataxonomic, taking a sample once the system was stable. The resulting figures highlight the most common phyla (Figures 2A,B) and the relation to each substrate’s TS% and C/N ratio (Figure 2C).
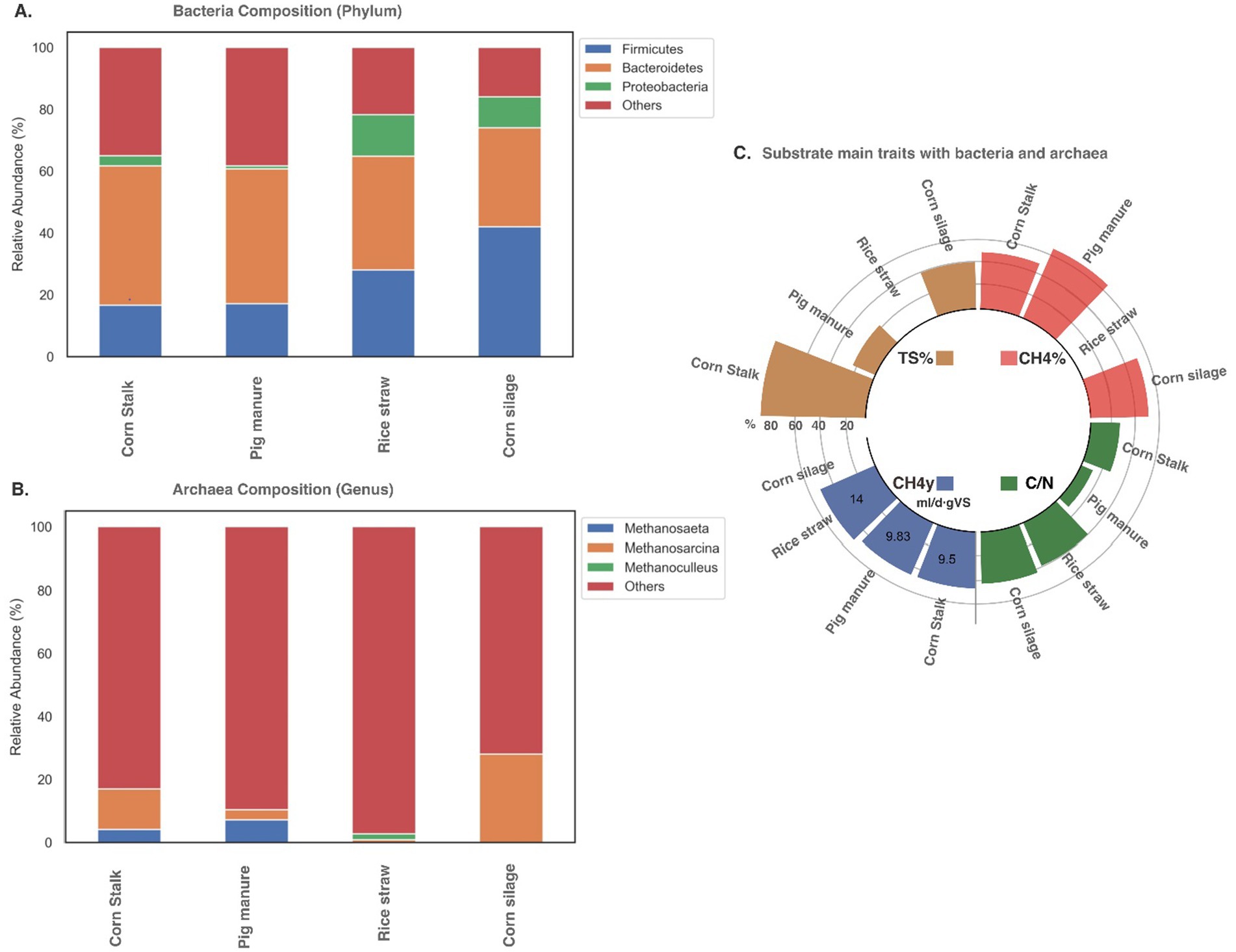
Figure 2. Microbial community composition during monodigestion: (A) bacteria and (B) Archaea composition and (C) substrate characteristics in terms of total solids (TS%), methane content (CH4), methane yield (CH4y) and carbon/nitrogen ratio (C/N) described in mesophilic digesters using average levels from different studies exemplifying corn stalk (Wang et al., 2017), pig manure (Wang et al., 2017), rice straw (Pore et al., 2016), and corn silage (Wirth et al., 2015).
This analysis reveals that Firmicutes, Bacteroidetes, and Proteobacteria are the most abundant bacteria across the four analyzed substrates (Figure 2A). Bacteroidetes, the dominant phylum, account for 23–36% of the MC and play a key role in breaking down cellulose and hemicellulose during acidogenesis into heteropolysaccharides like glucose and D-xylan, essential for lignocellulose degradation (Wirth et al., 2015; Heyer et al., 2016; Jia et al., 2016). Common hemicellulose-degrading species include Bacterioides fibrisolvens, Bacterioides ruminicola, Ruminococcus albus, and Ruminococcus flavenfaciens (Maspolim et al., 2015). Streptomyces is notable for its metabolite production and lignocellulose degradation, and it is frequently highlighted (de Lima Procópio et al., 2012; Zhang et al., 2019a). The consistent abundance of Bacteroidetes (Figure 2C), despite varying TS% and C/N ratios, highlights their adaptability and critical role in AD processes.
The second most abundant phylum, Firmicutes, involved in cellulose degradation (Wirth et al., 2015), accounted for 42% relative abundance in maize silage, with lower levels observed in corn stalk and pig manure. High TS% is believed to promote both Bacteroidetes and Firmicutes, though the lower C/N ratio in corn stalk compared to silage may have affected the abundance of Firmicutes.
The elevated TS% in corn stalks (Figure 2C) delays the initiation of microbial activity. Therefore, during hydrolysis and acidogenesis, key degradative bacteria from the Firmicutes and Bacteroidetes phyla can reach abundances of 60% (Pore et al., 2016; Wang et al., 2017) to 90% (Liu et al., 2019). Firmicutes is considered a versatile phylum capable of degrading lipids, carbohydrates, and proteins, reflecting the digester’s ability to metabolize cellulose, lignin, proteins, and sugars, which highlights the importance of this phylum in AD.
Furthermore, Proteobacteria were present to a lesser extent, with a relative abundance between 2 and 17% across samples (Figure 2A). Reduced Proteobacteria could be attributed to the high water content in materials like pig manure, which is associated with low TS%. This condition is believed to promote the proliferation of Bacteroidetes and Firmicutes while diminishing the population size of Proteobacteria (Xu et al., 2018b). Furthermore, the C/N ratio in pig manure is low compared to the optimal C/N value of 20–30 (Wang Y. et al., 2018; Xu et al., 2018b). This characteristic of pig manure as a single substrate is likely a limiting factor for microbial activity.
Archaea, single-celled prokaryotes, are found in a smaller proportion compared to bacteria, with ratios varying from 50 to 1,000 (Wang Y. et al., 2018) to 34.2 to 67.78% (Heyer et al., 2016). Some authors attribute these variations to methodological bias (Heyer et al., 2016). Despite their lower numbers, they are crucial in AD, serving as dominant methanogens, though many archaea contributing to methanogenesis remain unidentified (Bedoya et al., 2019; Pore et al., 2016). This study found many archaea that had not yet been described (Figure 2B). However, organisms such as Methanosarcina and Methanosaeta, known to play key roles in methanogenesis, were identified (Pore et al., 2016; Wang et al., 2017).
Methanoculleus, a hydrogenotrophic methanogen, was identified in rice straw as vital for biogas production (Xu et al., 2018b). In corn silage, Methanosarcina likely performed the entire methanogenesis phase, including hydrogenotrophic, acetoclastic, and methylotrophic reactions (Wang et al., 2017), while Methanothrix is related to methane production from acetate (Wirth et al., 2015).
In mono-digestion, pig manure exhibited the highest CH4% content, while rice straw achieved the highest CH4 yield. Bacteroidetes likely played an important role in degrading cellulose and hemicellulose in pig manure and corn stalks (Ren et al., 2014). The shared preference for acetate between Firmicutes and Methanosarcina may have enhanced acetoclastic methanogenesis (Xu et al., 2018b). The C/N ratio in single-substrate digestion is crucial, as imbalanced ratios can disrupt digester operation and diminish buffering capacity. Since single monodigestion substrates are rich in either carbon or nitrogen, careful selection of raw materials is essential to maintain a balanced C/N ratio, ensuring stable MC and system performance (Wirth et al., 2015; Wang P. et al., 2018).
4.2 Microbial community composition and associated syntrophic during codigestion
Co-digestion involves the simultaneous anaerobic digestion of two or more substrates, often developed to optimize biogas production and improve the stability of the digestion process. In this study, a comparative analysis of the MC in the codigestion system was analyzed by comparing two mesophilic digesters and three thermophilic digesters, each with different substrates. The mesophilic systems used a co-digestion base mixture of pig manure and corn stalk, with one system incorporating activated sludge as inoculum and the other adding cucumber residue. The thermophilic systems used a base mixture of food waste and activated sludge: one with a two-chamber configuration, another with a three-chamber configuration, with two adding horticultural waste, and a third with a single-chamber setup with the addition of biochar as a conductive material. Data from each digester was sourced from different studies, with samples taken for metagenomic analysis after system stabilization (Figure 3).
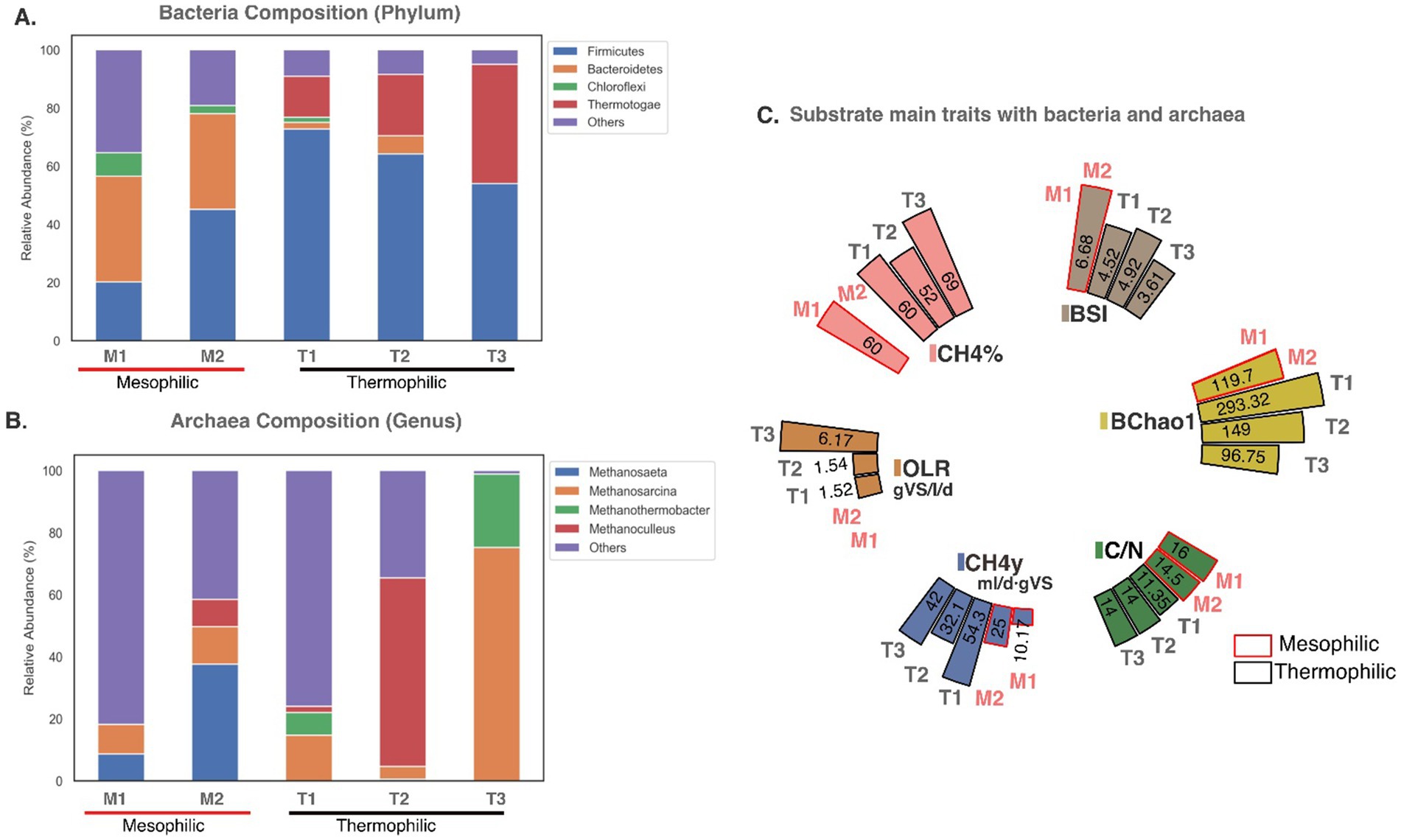
Figure 3. Microbial community composition during codigestion: (A) Bacteria and (B) Archaea composition described in mesophilic (M) and thermophilic digesters (T), M1 and M2 correspond to mesophilic digesters with one chamber configuration, M1 with substrates such as pig manure, corn stalk and activated sludge (Wang et al., 2017) and M2 with pig manure, corn stalk and cucumber residue (Wang Y. et al., 2018). In thermophilic digesters, T1 had a one-chamber configuration with substrates of food waste, activated sludge and biochar treatment (Zhang et al., 2020b), T2 had a two-chamber configuration with substrates like food waste, horticultural waste and activated sludge (Zhang et al., 2020a); and T3 had a three-chamber configuration with substrates as food waste, horticultural waste and activated sludge (Zhang et al., 2020a). (C) Represent main characteristics of the five treatments described above in terms of diversity: BSI (Diversity index Shannon-Wiener for bacteria), BChao1 (richness index for bacteria Chao1), substrate trait such as C/N ratio, OLR (organic loading rate) and associated CH4% (methane concentration) and CH4y (CH4 yield).
The phyla Bacteroidetes and Firmicutes, dominant acid-forming bacteria, were prevalent in mesophilic digesters. Chloroflexi, an acetic acid producer capable of degrading polysaccharides and monosaccharides (Wang et al., 2017), was prominent in mesophilic systems without cucumber residue and less abundant in thermophilic systems (Figure 3A). Bacteroidetes, known for strong adhesion to starch particles (Ren et al., 2014), were abundant in mesophilic digesters, likely facilitating the production of VFA, CO2, and H2 (Murillo-Roos et al., 2022). For mesophilic treatment (Wang Y. et al., 2018), pure cucumber residue led to acidification and low methane production during mono-digestion. However, a 5:2:3 mixture of pig manure, corn stalks, and cucumber residue improved microbial diversity and methane production, highlighting the benefits of co-digestion (Figure 3C).
Firmicutes were abundant in both mesophilic and thermophilic treatments, with a particularly high abundance in thermophilic systems. In thermophilic systems, Thermotogae was commonly observed, with its abundance increasing as the number of digestion stages grew. It is believed that Thermotogae, through syntrophic degradation of acetate with hydrogenotrophic methanogens, thrives in multi-stage digesters where acidogenesis and methanogenesis are separated. This separation allows for the proliferation of more specialized methanogenic bacteria (Xu et al., 2018b). This syntrophic relationship between Thermotogae and methanogens likely contributed to the higher methane content and yield observed in the three-chamber configuration system.
The use of biochar in thermophilic systems improved microbial diversity, methane content, and yield, demonstrating its potential to stabilize systems by promoting direct interspecies electron transfer (DIET) to accelerate microbial metabolism (Gahlot et al., 2021; Johnravindar et al., 2021; Su et al., 2023), and mitigate high OLR impacts (Ye et al., 2018; Zhang et al., 2020b). Biochar treatments yielded the highest CH4 at the lowest OLR (1.52gVS/l/d) and showed the greatest BSI and BChao1 (Wang Y. et al., 2018; Zhang et al., 2020b), indicating greater microbial diversity and richness (Xu et al., 2018a; Zhang et al., 2020a, 2020b).
Figure 3B shows that codigestion increased the abundance of dominant archaea and introduced new participants, such as Methanothermobacter, in thermophilic systems and Methanoculleus in mesophilic and thermophilic treatments. No single dominant archaea phylum was present across all digesters, though Methanosarcina appeared in all treatments, particularly dominating the three-chamber thermophilic system. This dominance may be due to its ability to utilize diverse substrates like acetate, methanol, and H2 and thrive across a wide temperature range (Ali Shah et al., 2014; Murillo-Roos et al., 2022).
Methanothrix dominated over Methanosarcina in mesophilic digesters, with the latter absent in thermophilic treatments. Methanothrix likely prevailed due to its higher acetate affinity, effectively lowering acetate concentration compared to Methanosarcina (Xu et al., 2018b; Oosterkamp et al., 2019). Methanoculleus and Methanothermobacter were identified in thermophilic digesters at different OLRs (Figure 3C), with low OLR favoring Methanoculleus in the two-chamber system, while high OLR advantaged Methanothermobacter in three-chamber systems (Zhang et al., 2020a). Both Methanothermobacter and Methanosarcina, key hydrogenotrophic microorganisms, were identified (Bedoya et al., 2019).
Phylum Chloroflexi such as Anaerolineae class is related to hydrogenotrophic methanogens (Xu et al., 2018b), Archaea Methanosaeta spp. (Bovio-Winkler et al., 2021), and acetoclastic methanogens like Methanosarcinaceae (Liang et al., 2015; Murillo-Roos et al., 2022), where Anaerolineae is an acetate or hydrogen contributor to methanogens (Bovio-Winkler et al., 2021; Murillo-Roos et al., 2022). This potential syntrophy could promote a flow of metabolites between the three, leading to higher methane yield.
Since syntrophy activity is an important aspect of AD system stoutness (Oosterkamp et al., 2019), Figure 4 displays common trophic microbial associations, identified through metagenomic studies, that have assisted biogas production.
To reiterate, Firmicutes stands out as a key player, linked to all archaea organisms Figure 4. It co-cultures acetate with Methanobacteriales, Methanobacterium, Methanococcus, Methanoculleus, Methanothrix, or Methanosarcina (Saha et al., 2019; Han et al., 2020; Tabatabaei et al., 2020); and butyrate with Methanobacterium to produce propionic acid, which is converted into biogas (Han et al., 2020). As shown in Figure 4, Proteobacteria also contribute to biogas production from propionic acid when associated with hydrogenotrophic methanogens such as Methanobacterium (Han et al., 2020). Similarly, syntrophy between Aminivibrio (Synergistetes) and Methanobacterium facilitated methanation via VFA from amino acid oxidation (Saha et al., 2019). Thermotogae was also observed to degrade acetate with hydrogenotrophic methanogens (Xu et al., 2018b).
In summary, the treatments shown in Figure 3 had an average C/N ratio of 14, which yielded optimal results, suggesting this to be a suitable C/N ratio for enhancing microbial biomass digestibility (Wirth et al., 2015). Codigestion consistently proved to be a more cost-effective method than monodigestion, offering improvements in buffering capacity, nutrient equilibrium, and overall process stabilization (Wang et al., 2017; Wang Y. et al., 2018). Achieving a balance of macronutrients and micronutrients while reducing inhibitory and toxic compounds, further supports system efficiency (Wang Y. et al., 2018). Then, it could be stated that codigestion induces the creation of favorable microenvironments that promote methanogen growth and system stabilization, leading to increased CH4y yield. These microenvironments are occupied by keystone microbial members, considered AD regulation markers, which are responsible for sustaining the AD process (Xu et al., 2018a). The absence of these key organisms could lead to AD failure.
Acidogenic bacteria from the Firmicutes phylum play a fundamental role in AD as a dominant, versatile, and functionally diverse organism (Figures 3, 4), capable of metabolizing complex organic residues like lipids, carbohydrates, cellulose, lignin, proteins, and sugars under various conditions (Ren et al., 2014; Venkiteshwaran et al., 2017; Zhao et al., 2018). Firmicutes are positively connected to biogas production (Xu et al., 2018b). Bacteroidetes, the second most dominant acidogenic bacteria, also play a significant role in AD (Wang P. et al., 2018). Both phyla are key in degrading substrates, producing acetic acid, and secreting lytic enzymes during acidogenesis, increasing soluble organic matter concentrations and CH4y yield (Liu et al., 2019).
Acetate concentration influences acetotrophic methanogens regarding growth, CH4 production, and metabolism. For instance, Methanothrix can grow and generate CH4 at low acetate concentrations. Firmicutes also appear to have a syntrophic relationship with Methanothrix and hydrogenotrophic methanogen Methanosarcina, where an increase in Methanosarcina correlates with higher acetate levels, potentially stabilizing the system and enhancing CH4 generation (Xu et al., 2018b; Zhou et al., 2023).
The three main methanogenesis pathways present in AD are acetoclastic, hydrogenotrophic, and methylotrophic (Ali Shah et al., 2014). Methanothrix, an acetoclastic methanogen, efficiently converts low-concentration acetate into CH4 and CO2, while Methanosarcina can generate CH4 through all three pathways, making it a cornerstone organism in co-digestion (Wang et al., 2017; Oosterkamp et al., 2019). Methanothrix dominated mesophilic treatments but decreased notably in thermophilic treatments, where Thermotogae and Methanothermobacter became more prevalent. Methanosarcina was present in both treatments.
Inoculum material like activated sludge is vital for system startup and stability, balancing populations, and promoting syntrophic metabolism (Ali Shah et al., 2014). Bioaugmentation with H2 producers has shown that it is a bottleneck, with its reduction leading to increased biogas production (Heyer et al., 2016). Moreover, methods such as DIET and conductive materials like biochar improve methanogenic bioreactor performance. With its functional groups acting as electron shuttles and high electrical conductivity, biochar is favored for DIET (Ye et al., 2018; Alghashm et al., 2023). Alternative conductive materials like magnetite boost AD performance by adding in situ H2S removal and elemental sulfur recovery. Metagenomics combined with DIET has uncovered new electric syntrophies between sulfide-oxidizing bacteria (SOB) and electrotrophic Methanothrix and new routes for anaerobic sulfur metabolism, stimulating electroactive microorganisms (Jung et al., 2023; Zhou et al., 2023). Syntrophic collaboration between hydrogenotrophic methanogens and acetogenic bacteria, known as interspecies hydrogen transfer, maintains H2 levels low enough to make secondary fermentation thermodynamically feasible, harmonizing H2 production and consumption in anaerobic reactors (Ali Shah et al., 2014; Gahlot et al., 2021).
5 Advanced metagenomic analyses
5.1 Genome-centric metagenomics in AD research
16S rRNA gene sequencing provides a broad overview of MC during AD but may lack the depth needed to interpret functional and metabolic interactions crucial to process efficiency (Asgarineshat et al., 2022). GCM tools have demonstrated significant advantages over PCR techniques for enhanced analysis of microbiota metagenomes without PCR bias, augments coverage of high-GC regions of the genome, reduces duplicate reads (Sims et al., 2014), and mitigates inaccuracies in the abundance and diversity of genes (Kanagawa, 2003). GCM is a rapidly advancing method that can recover high-quality MAGs through efficient binning procedures (Treu et al., 2018; Jiang et al., 2023). This technique facilitates the elucidation of key microbial players, their functions, shared metabolic pathways, interspecies hydrogen transfer, cofactor identification, nutrient competition, nutrient exchanges, and the metabolic reconstruction of MAGs (Figure 5) (Campanaro et al., 2019; Zhu et al., 2019; Sun M. et al., 2023; Zhang L. et al., 2023). As a result, more accurate predictive models can be developed, allowing better correlations between microbial physiology to ecological fitness, organic material selection, digester design, and operation to achieve higher performance and biogas generation (Turaev and Rattei, 2016; Palù et al., 2022; Greses et al., 2023; Heyer et al., 2024).
Recognizing the importance of compositional structures and functional connections in AD, recent studies have characterized and compared these aspects under different feeding treatments (continuous and discontinuous) and OLRs (high and low), using short-chain fatty acids such as acetate, propionate, and butyrate as the carbon sources. To address the limitations of existing technologies in analyzing complex MCs, a hybrid approach combining Illumina-based short-read and ONP long-read assemblies can be a feasible solution. This methodology is expected to enhance MAG completeness and proximity, leading to the discovery of potentially novel organisms such as Syntrophobacteraceae species, associated with syntrophic short-chain fatty acid oxidation; Syntrophomonadaceae species, implicated in butyrate oxidation; and Methanoculleus species, linked to hydrogenotrophic methanogenesis (Becker et al., 2023).
Additionally, it has been learned that MC responsible for fundamental processes may have a broader role in the food web, potentially leading to the discovery of unknown metabolite cross-feeding interactions and new participants like scavengers in biomass turnover. For instance, Syntrophobacter and Pelotomaculum have been identified as syntrophic oxidants for propionate, while pathway analysis might reveal common butyrate degradation gene patterns, probably caused by novel butyrate-degrading bacteria like Syntrophomonas. In the oxidation of propionic and butyric acid, Cloacimonetes, Cryptanaerobacter, and Desulfovibrio can be identified. Unclassified Bacteroidetes, Candidatus Cloacimonas, Mesotoga, Desulfovibrio, or Endomicrobium may not exhibit significant abundance unless butyrate degradation is completed. Desulfotomaculum, which utilizes acetate, butyrate, and propionate oxidation, can be considered a metabolically versatile genus.
Similarly, hydrogenotrophic and acetoclastic methanogenesis pathway genes identified in the archaeal genus Methanothrix are believed to generate CH4 through DIET (Zhang X. et al., 2023). Methanosarcina has been identified as a resilient and abundant archaeon, capable of overtaking Methanothrix at high OLRs. This indicates that stress tolerance can be alleviated if Methanosarcina is promoted in exchange for more vulnerable species, boosting system resilience during AD. The increase in methanosarcina abundance through discontinuous regimes is interesting, advocating that feeding regimes might influence temporal niches and provide optimal conditions for archaeal community motion. This type of study emphasizes the significance of syntrophic activity between bacteria and methanogenic archaea, which could enhance system buffering and stability.
Innovative research by Gaspari et al. (2024) used GCM to assess the impact of rising ammonia levels on the resilience and functional dynamics of AD systems, with a focus on microbial responses to ammonia stress, particularly key archaeal species and methanogenesis pathways. Ammonia is a well-known inhibitor of biogas production, with concentrations of approximately 3,000 mg/L, depending on pH levels, potentially affecting AD (Bi et al., 2021; Gaspari et al., 2024).
Concentrations above this threshold, irrespective of pH, significantly inhibit microbial activity and biogas production (Kalamaras et al., 2023; Gaspari et al., 2024). Gaspari et al. (2024) revealed that under high ammonia conditions, hydrogenotrophic methanogenesis pathways were favored over the acetoclastic pathway. Dominant species identified included Methanoculleus bourgensis MX4, Methanoculleus sp. MA7, Methano-thrix sp. MA6, and Methanosarcina flavescens MX5.
Furthermore, the findings suggest that certain archaeal species, such as M. flavescens MX5 and Methanothrix sp., MA6 may adapt their metabolic strategies to survive and continue methane production via CO2 reduction, potentially utilizing DIET, indicating ecological fitness. The presence of syntrophic acetate-oxidizing bacteria (SAOB), such as Syntrophomonadaceae sp. MX66, likely contributed to increased hydrogenotrophic activity by producing H2 and CO2 from acetate, which could then be reduced via the acetyl-CoA pathway to sustain methane production (Becker et al., 2023; Gaspari et al., 2024).
This research highlights the importance of expanding metagenomics approaches to achieve deep insights into microbial dynamics and their response to environmental stressors. Advanced metagenomics techniques, like GCM, allow detailed analysis recognizing microbial interactions and metabolic pathways, providing a valuable foundation for informed decision-making in AD process optimization. For example, understanding the MC’s tolerance to specific stress factors like ammonia can guide the selection of suitable feedstock compositions. Additionally, by modifying procedure parameters like pH and temperature based on metagenomic insights, it becomes possible to enhance biogas production and identify potential diagnostic biomarkers for monitoring and controlling AD.
In a significant step forward for AD research, Greses et al. (2023) demonstrated the power of GCM in deciphering the intricate microbial dynamics of open-mixed cultures for producing value-added biochemicals. By focusing on functional metabolic pathways, the study assessed the potential of food waste as a sustainable feedstock for generating these valuable compounds, with the aim of replacing traditional petrochemical-based products. The researchers employed an in-silico community-level simulation to elucidate single-species activities and essential interspecies interactions within the culture.
From 58 high-quality reconstructed MAGs, key players such as Bifidobacterium subtile IE007 and Eubacteriaceae IE027, associated with acetate, butyrate, and ethanol production, were favored at a pH of 6.5. However, a slight pH decreases to 6.1 shifted metabolic activity, promoting caproate and H2 production, primarily mediated by Eubacteriaceae IE037. The study also demonstrated the feasibility of achieving high ethanol titers (comparable to those from pure yeast cultures) using non-pretreated open-mixed cultures, highlighting the potential for cost-effective bioethanol production from waste. The research further revealed that microbial metabolisms can be modulated to obtain targeted products and that pH alteration can modify the AD process to help the production of valuable market intermediate compounds over biogas. This study underscored the importance of GCM in understanding MC interactions and dynamics, revealing how factor adjustment can shape microbial metabolism to generate added-value products, offering a promising approach to sustainable biorefinery development.
Table 5 presents a detailed comparison of the advanced metagenomics (GCM) tools employed in AD research. It organizes them by the specific processes they support and provides insights into their suitability, innovation, computational demand, and user-friendliness.
Recent studies in GCM applied to AD illustrate distinct approaches to the field, showing an evolution towards more refined and comprehensive tools. Becker et al. (2023) used well-established methods, Greses et al. (2023) joined traditional and cutting-edge techniques, while Gaspari et al. (2024) pushed the boundaries with innovative approaches. This progression reflects a pattern of evolution in metagenomics, with a trend toward increasingly sophisticated and computationally intensive tools that offer higher accuracy and detail.
Unlike 16S rRNA metataxonomics, generally limited to genus-level taxonomic resolution and lacking in functional detail, being confined to known organisms within reference databases, and often unable to detect novel microbes, these findings demonstrate the importance of furthering metagenomics through GCM. Using long, high-quality reads, GCM has confirmed the association of specific organisms with essential catabolic pathways, optimizing these routes under suboptimal conditions. For example, syntrophic interactions involving Syntrophobacter and Syntrophomonas are integral to the degradation of short-chain fatty acids and biogas production. Moreover, hydrogenotrophic methanogenesis becomes more prominent under ammonia stress, with Methanoculleus species contributing to system resilience.
GCM’s ability to uncover novel genomes missed by short-read studies and reveal new functional competencies refines the understanding of microbial metabolic diversity, challenging the conventional four-step AD scheme. As GCM techniques advance, they provide more accurate predictions of biogas production, transforming AD from a “black box” into a more manageable system. However, the increased accuracy and detail come at a higher cost, requiring a balance between precision and resources in sustainable biorefinery development. This overview helps researchers align established methods with cutting-edge techniques, highlighting the growing sophistication of metagenomics in unraveling the AD microbiome.
5.2 Strain-resolved metagenomics applied to biogas production
Although the recovery of high-quality MAGs denotes a substantial advancement in GCM within AD research, underexplored layers of microbial genome diversity remain. These hidden layers require more sophisticated methodologies to fully elucidate comprehensive microbial adaptive strategies, surpassing the limitations of species-level resolution (Greses et al., 2023; Ghiotto et al., 2024a). For instance, advanced microbiome evolutionary, functional, and metabolic tracking mechanisms are essential. Such a mechanism would enable the selection of microorganisms at a higher level of diversity through metagenome strain identification (Bucci et al., 2024; Ghiotto et al., 2024a), recognizing strains as the fundamental unit of microbiological diversity, a group of single-cell clonal descendants (Quince et al., 2021; Setubal, 2021). This approach allows for monitoring diversity beyond the species level, with the capacity to detect genetic changes even without microbial abundance fluctuations (Ghiotto et al., 2024b).
In AD research, merely evaluating species dynamics often falls short of capturing the nuanced changes within species and the temporal succession of coexisting strains (Bucci et al., 2024; Ghiotto et al., 2024b). Single-nucleotide variants (SNVs) analysis emerges as a promising approach to bridge this gap. This method permits the detection of SNVs at specific genomic positions, whether within protein-coding sequences or intergenic regions (Zou et al., 2020). By revealing shared gene variations, such as insertions and deletions, SNV analysis makes it possible to track strains within a species based on allele pattern detection (Quince et al., 2021; Bucci et al., 2024; Ghiotto et al., 2024b).
A complementary strategy known as strain deconvolution uses shotgun metagenomics reads to concurrently determine strain genotypes and relative abundances across samples. This is accomplished via statistical deconvolution of allele frequencies, a process that separates mixed genetic signals into their parts, allowing the identification of multiple genotypes from pooled data (Smith et al., 2022). This procedure has proven effective in evaluating the genetic dynamics and ecological fluctuations of AD species, especially in tracking emerging mutations at species and strain levels (Bucci et al., 2024; Ghiotto et al., 2024b). These interrelated methods allow the tracking of microbial strains, assessment of their relative abundances, identification of dominant organism variations, and monitoring of evolutionary trends, thereby aiding in the identification of suitable environmental conditions for archaea and bacteria syntrophies during AD (Ghiotto et al., 2024b).
Bucci et al. (2024) explored the impact of escalating ammonia concentrations on MC within AD. The study sought to delve deeper into microbial evolution by applying SRM, focusing on monitoring SNVs over time. By gradually increasing ammonia levels across cultivation generations, the study aimed to unravel the dynamics of ammonia-tolerant methanogenic consortia at both species and strain levels. Strain deconvolution was employed to isolate genetic variants from shotgun metagenomics data, providing a granular view of allele occurrences. This approach enabled a detailed examination of how microbial consortia adapt to increasing ammonia stress at a finer genetic resolution.
The investigation identified 179 MAGs (172 bacterial and 7 archaeal), with Firmicutes as the predominant bacterial phylum. Among the archaeal species, Methanoculleus bourgensis vb3066 was notably abundant, likely due to its syntrophic interactions with bacteria involved in the Wood-Ljungdahl (WL) pathway, a carbon fixation process crucial for converting CO2 to acetate, and the glycine synthase reductase pathway. SAOBs like Keratinibaculum sp. ma44 and Acetomicrobium sp. ma133 were also key players. Over time, the relative abundance of Clostridium cochlearium ma73, Keratinibaculum sp. ma44, and M. bourgensis vb3066 increased, while Acetomicrobium sp. ma133 and Firmicutes sp. ma48 showed reduced relative abundance at later generations, possibly because of competition and ammonia tolerance thresholds. The study identified Acetomicrobium sp. ma133, C. cochlearium ma73, Firmicutes sp. ma48, Firmicutes sp. mb175, Keratinibaculum sp. ma44, Keratinibaculum sp. mb43, and M. bourgensis vb3066 as key organisms capable of thriving in high ammonia conditions. These findings accentuate the importance of specific microbes in maintaining metabolic balance under challenging conditions.
Notably, 148 non-synonymous SNVs were identified in enzymes associated with the WL and glycine synthase reductase pathways in Acetomicrobium sp. ma133148, indicating significant selective pressures that allowed these enzymes to maintain their functionality under elevated ammonia concentrations. The persistence and dominance of M. bourgensis vb3066 and Acetomicrobium sp. ma133 suggest that these strains have adapted to stabilize the system by mitigating inhibition and fostering robust syntrophic relationships. Genes associated with the WL and glycine synthase reductase pathways in C. cochlearium ma73, Keratinibaculum sp. ma44, and Tepidanaerobacteraceae sp. ma135 might imply that all or some of these organisms may have taken on roles as putative SAOB (Bucci et al., 2024). Strain deconvolution was thus proven to be an effective technique for identifying intraspecific diversity, displaying coexisting strains with diverse phenotypes as organisms respond uniquely to environmental pressures. This genetic evidence emphasizes the critical role of SNVs in supporting microbial adaptation and survival, reinforcing the importance of genetic diversity.
Recognizing the need to improve AD products, such as increasing CH4 content and reducing H2S concentration, Ghiotto et al. (2024a) studied the evolution of a mixed-methanogenic culture using a novel approach involving variant calling and strain deconvolution. The research subjected the methanogenic community to high H2S levels in a trickle bed reactor, a type of packed bed reactor that facilitates interaction between solid, liquid, and gas phases during chemical reactions driven by gravity-assisted flow (Malolan et al., 2022). The experiment was divided into three stages: S1 (artificial and sulfur-rich biogas), S2 (pure H2 with biogas from a lab-scale continuous stirred-tank reactor), and S3 (microaerophilic conditions with 0.002% v/v O2). These stages aimed to evaluate the combined biomethanation and desulfurization processes of anoxic and limited oxygen conditions while assessing the metabolic capabilities of AD microbiota to achieve biogas upgrading as a sustainable alternative to conventional chemical methods.
Throughout the study, 97 to 146 high-quality MAGs were identified, revealing 171,545 distinctive SNVs across six samples, 37% of which were nonsynonymous. In S1, the microbial landscape was prominently shaped by Methanobacterium sp. DTU45, a hydrogenotrophic methanogen that prevailed under sulfur-enriched conditions due to its capacity to utilize the elevated H2 levels. Its biofilm-forming ability could have offered protection against toxic agents like oxygen, further contributing to its resilience. Concurrently, Gammaproteobacteria sp. DTU53 began to proliferate, capitalizing on the controlled conditions. This initial phase provided critical insights into the early adaptive mechanisms of the methanogenic community when exposed to elevated H2S levels. During S2, putative SOB such as Gammaproteobacteria sp. DTU53 benefited from a gas injection, with an alteration in abundance indicating a possible electron redirection to the sulfate-sulfur receptor pathway. In S3, 81% of H2S was successfully removed, and Gammaproteobacteria sp. DTU53 reappeared, potentially exhibiting a metabolic modification from fermentation to aerobic respiration, utilizing sulfur compounds as electron donors. Facultative anaerobes like A. equifetale DTU58 also contributed to desulfurization as a potential oxygen-resistant species in this microaerophilic environment. Additionally, the identification of a molecular marker for potential SAOB, the formate-tetrahydrofolate ligase gene, in Limnochordia sp. DTU66, Proteinivoracia sp. DTU68, and Firmicutes sp. DTU69, participants in the WL pathway, could have encouraged facultative syntrophies with Methanobacterium species.
The study demonstrated that genomic variant growth persuades phenotypic changes, improving functional redundancy and resilience within the microbiome. Strain deconvolution effectively revealed intraspecific diversity, allowing for successful microaerophilic biomethanation (95% CH4) and hydrogen sulfide removal (81%), without hindering hydrogenotrophic archaea. However, while these findings are promising, the controlled experimental conditions may not fully reflect the complexities of industrial-scale systems. The scalability and wider applicability of these results required additional validation in more varied and realistic environments.
Seeking to promote sustainable practices within the Carbon Capture and Utilization concept, Ghiotto et al. (2024b) focused on achieving biological biogas upgrading through autotrophic approaches. The study highlighted the relevant role of hydrogenotrophic archaea in balancing H2 levels while cooperating with sulfate-reducers and SAOB to remove acetate and sulfur. Given the limited knowledge of SNVs’ impact on hydrogenotrophic archaea, this research analyzed how SNVs influence the functional properties of these organisms for biomethane production, aiming to uncover new genetic insights.
Two case studies were conducted: the first assessed various feedstock substrates, while the second evaluated the effect of continuous H2 addition on the microbiota. In the first experiment, 47 high-quality MAGs were identified, with Firmicutes dominating at 93% abundance. Methanothermobacter wolfeii MA_1 was notably abundant, primarily using the CO2 to CH4 reduction pathway and acetate, formate, and methanol when H2 was unavailable. Sphaerobacter thermophilus CO_9, Caldanaerobacter subterraneus MX_27, and Limnochordia sp. MA_37 were the predominant bacteria.
The second experiment yielded 50 high-quality MAGs, with Firmicutes as the most abundant bacteria. Methanosarcina thermophila MB_65 and Methanocullus thermophilus MA_62 were the dominant archaea, each with distinct metabolic preferences: acetate for M. thermophila and H2 for M. thermophilus, which thrived with H2 addition. Limnochordia sp. MB_100, Bacteroidales sp. VB_122, and Acetomicrobium sp. MX_67 showed the highest relative abundance.
It is likely that syntrophic relationships between acetate-oxidizing bacteria, M. wolfeii MA_1, and M. thermophilus MA_62 might have promoted the high abundance of both archaea. A total of 76,229 SNVs were identified and categorized as synonymous (56%), nonsynonymous (30%), and intergenic (14%) variations. Analysis of relative abundance and SNV accumulation over time indicated that species dominance was linked to a higher number of genetic variants. Defined environmental parameters facilitated cooperative coexistence within the MC during methanogenesis, involving conventional and alternative WL pathways. Notably, it is suggested that the efficiency of carbon capture and utilization within MC be prioritized over isolation experiments to enhance biogas production by identifying the most efficient and resilient strains (Ghiotto et al., 2024b).
Table 6 provides a clear and concise comparison of the field’s current state in strain-level analysis in AD research, showcasing key tools and their applications. This overview, grounded in insights from rigorous and recent studies, serves as a valuable guide for researchers in selecting suitable techniques for their specific research needs.
InStrain v1.6.3 is a widely used tool for SNV identification, known for its precision, though it requires moderate computational resources and expertise (Lindner et al., 2024). STRONG, integral to strain resolution, delivers high accuracy via its BayesPaths algorithm but is computationally intensive and complex to implement (Quince et al., 2021).
AlphaFold, used in one study for 3D protein modeling, provides cutting-edge insights but is also demanding in terms of computational power. Gene annotation relies on well-established tools, with Microbe-Annotator v2.0.4 being the most innovative, providing comprehensive analyses but with considerable computational requirements. For phylogenetic analysis, PhyloPhlAn v3.0 and iTOL are reliable options, requiring moderate computational power. The choice of tools varies in complexity, computational load, and user-friendliness, often demanding substantial expertise to achieve optimal results.
The exploration of advanced GCM and SRM in AD research represents a shift from species-level analysis to more refined strain-level insights. Studies by Ghiotto et al. (2024a), Bucci et al. (2024), and Ghiotto et al. (2024b) used SRM to reveal hidden layers of microbial genome diversity that traditional 16S rRNA gene-based metataxonomics or even GCM could not fully capture. SRM’s ability to track SNVs and analyze their influence on functional and metabolic traits provides a more comprehensive understanding of microbial adaptation to stressors such as high ammonia or H2S levels.
These studies consistently identified resilient strains of hydrogenotrophic archaea, which maintained metabolic balance through DIET and biofilm formation. A common theme was the discovery of numerous SNVs contributing to phenotypic variation, species dominance, increased functional redundancy, and overall microbial success under various conditions.
Implementing SRM and high-quality MAG recovery involves substantial computational and sequencing costs compared to 16S rRNA gene analysis. The need for deep sequencing, extensive computational resources for SNV analysis, and specialized software for strain deconvolution all contribute to higher expenses. Nevertheless, the added cost is justified by the richer, more actionable insights that SRM provides for optimizing AD operations.
Variant analysis grants critical perceptions into AD metabolic pathways by revealing how microbial evolution and strain dominance occur. Nonsynonymous SNVs can influence microbial metabolism and functional potential by altering protein structures, leading to gains or losses in function that can enhance biogas yields. The strain-level resolution provided by SRM is essential for knowing the impacts of selective pressures on MC structure and elucidating the adaptive mechanisms that support stable bioconversion and resistance development in inhibitory environments.
While GCM is valuable for understanding community compositions, it often lacks the precession needed to capture strain-level diversity and the functional implications of SNVs. In contrast, SRM offers novel insights into the evolution and interactions of AD microbiota, particularly under adverse conditions. Future efforts should prioritize identifying and enhancing the most robust and efficient strains within diverse MCs, ensuring that detailed genetic knowledge is applied with cost-effectiveness and wider practical implementation.
6 Challenges and perspectives of metagenomics in AD research
In AD research, replication is frequently neglected due to cost and time constraints, yet it is essential for ensuring statistical robustness and improving pattern detection in microbial ecology. This lack of replication is especially problematic in metagenomic studies, where sampling and storage biases can distort MC analysis. Additionally, the incomplete nature of metagenomics data hinders the identification of functional genes and the detection of uncharacterized microorganisms in biogas samples. Expanding gene databases is crucial to address these limitations, providing stronger evidence for functional predictions and capturing microbial diversity more effectively.
Combining GCM with SRM could offer a robust approach to refining functional predictions and exploring ecological interactions within complex MC (Kim et al., 2022). GCM reconstructs MAGs, providing insights into the genetic makeup and functional potential of different microbial populations in a community. However, it may not distinguish between closely related strains within a species. SRM addresses this limitation by resolving the strain level and uncovering the genetic diversity and specialized functions that dive into processes like organic matter degradation and methane production. Together, they might display a profound perspective on community-wide functions and the distinct contributions of individual strains.
Further integration of advanced metagenomics with multi-omics and molecular technologies would provide new depth in understanding MC functions (Kim et al., 2022; Li et al., 2022). This integration enhances the accuracy of functional predictions and allows for more precise manipulation of microbial consortia to achieve desired outcomes in AD processes, such as efficiently producing biochemicals and biofuels, supporting the shift toward sustainable production systems (Greses et al., 2023).
Molecular methods permit a detailed exploration of microbial ecological roles and metabolic capabilities through synergistic networks supported by catabolic complementarity, electron transfer balance, and energy conservation. In this context, the promising scientific discipline, ecogenomics, which studies the relationships between microbial functions and their environments, is valuable (Nobu et al., 2015; James and Richardson, 2020).
Concepts such as quorum sensing, a microbial cell-to-cell signal molecules communication system bridging biological processes between MC by regulating gene expression, population behavior, and density via extracellular autoinducers (Zhang et al., 2021; Wang et al., 2023), should be considered in microbial connectivity techniques in AD. This system can regulate AD metabolic processes such as chemical oxygen demand removal and methanogenesis (Lv et al., 2023). Similarly, metagenomics could become a useful tool for identifying microbiota with an affinity for conductive materials, thereby promoting DIET and enhancing CH4 production (Lei et al., 2019). A deeper understanding of microbiome interactions will lead to more efficient methodologies to sustain key metabolic routes in AD.
Metagenomic analysis through large-scale sequencing faces significant challenges, including substantial computing requirements, limited storage, extended processing times, and high sequencing costs. To address these, integrating supercomputing with data mining and ML algorithms on cloud platforms is essential. These algorithms should be specifically designed for metagenomics and linked to open-source databases to improve accuracy (Zhang et al., 2019a; Yang et al., 2021). However, AI methods in metagenomics are still constrained by high computational demands and the need for large, well-annotated datasets, which are often lacking in complex environments like AD. This shortage can lead to bias and generalization challenges. Therefore, enhancing data curation and optimizing models are essential for the wider adoption of advanced AI-driven approaches.
The future of AD research may involve developing specialized tools within more user-friendly platforms, combining precision with ease of use. This would make technologies accessible to a wider range of researchers and practitioners. Additionally, using real-time metagenomic data alongside operational controls could allow for dynamic adjustment to process parameters, enhancing system resilience and efficiency. This approach provides a comprehensive view of the functional potential within microbial populations, enabling more responsive and efficient management.
In summary, metagenomics has significantly advanced the analysis of microbial communities (MC) in anaerobic digestion (AD), but further progress in technology and methodology—particularly in real-time monitoring, multi-omics integration, and machine learning—will be crucial for optimizing these systems. Addressing these current challenges in sampling, data handling, computational demands, and data completeness while continuously expanding gene databases and providing direct evidence for predicted functionalities, will be key to fully unlocking the potential of metagenomics in AD research.
7 Conclusion
This review has thoroughly explored the transformative potential of metagenomics in revolutionizing AD processes. By providing a detailed understanding of the microbiome involved, metagenomics has clarified the crucial role of MCs in converting residual biomass into valuable products such as biogas, biomethane, biochemicals, and biofuels. The review also highlights how feedstock composition and operational conditions significantly shape MC structure and function.
Metagenomics is a powerful tool for identifying and characterizing entire microbiomes, including non-cultivable organisms while providing valuable insights into their metabolic pathways and interactions. The integration of advanced techniques, such as GCM and SRM, has further deepened the understanding of microbial dynamics, evolution, adaptations, and functional capabilities within AD systems.
This enhanced understanding of MC interactions paves the way for targeted interventions and process optimization, helping to unravel the complexity of the AD system. Identifying and isolating key MCs, combined with the development of user-friendly tools and real-time monitoring systems, holds immense promise for optimizing AD operations, achieving higher biogas yields, and ultimately developing more efficient and sustainable AD technologies. By empowering researchers and practitioners with critical insights, the application of metagenomics is poised to play a pivotal role in advancing the AD field.
Author contributions
IO: Conceptualization, Data curation, Formal analysis, Funding acquisition, Investigation, Methodology, Project administration, Resources, Supervision, Validation, Visualization, Writing – original draft, Writing – review & editing. LF-P: Conceptualization, Funding acquisition, Methodology, Project administration, Writing – original draft, Writing – review & editing. CC: Conceptualization, Formal analysis, Methodology, Validation, Visualization, Writing – original draft, Writing – review & editing.
Funding
The author(s) declare that financial support was received for the research, authorship, and/or publication of this article. This research has been funded by Dirección General de Investigaciones of Universidad Santiago de Cali under call No. 01-2024, and the Universidad Autónoma de Occidente.
Acknowledgments
The authors thank the Universidad Autónoma de Occidente and the Universidad Santiago de Cali for their invaluable support. This research has been partly funded by Dirección General de Investigaciones of Universidad Santiago de Cali under call No. 01-2024.
Conflict of interest
The authors declare that the research was conducted in the absence of any commercial or financial relationships that could be construed as a potential conflict of interest.
Publisher’s note
All claims expressed in this article are solely those of the authors and do not necessarily represent those of their affiliated organizations, or those of the publisher, the editors and the reviewers. Any product that may be evaluated in this article, or claim that may be made by its manufacturer, is not guaranteed or endorsed by the publisher.
References
Abedi, S., Nozarpour, A., and Tavakoli, O. (2023). Evaluation of biogas production rate and leachate treatment in landfill through a water-energy nexus framework for integrated waste management. Energy Nexus 11:100218. doi: 10.1016/j.nexus.2023.100218
Abellan-Schneyder, I., Matchado, M. S., Reitmeier, S., Sommer, A., Sewald, Z., Baumbach, J., et al. (2021). Primer, pipelines, parameters: issues in 16S rRNA gene sequencing. mSphere 6, e01202–e01220. doi: 10.1128/mSphere.01202-20
Adebayo, A., Jekayinfa, S., and Linke, B. (2015). Effects of organic loading rate on biogas yield in a continuously stirred tank reactor experiment at mesophilic temperature. Br. J. Appl. Sci. Technol. 11, 1–9. doi: 10.9734/BJAST/2015/18040
Akyol, Ç., Ince, O., Bozan, M., Ozbayram, E. G., and Ince, B. (2019). Biological pretreatment with Trametes versicolor to enhance methane production from lignocellulosic biomass: a metagenomic approach. Ind. Crop. Prod. 140:111659. doi: 10.1016/j.indcrop.2019.111659
Alarcón-Vivero, M., Moena, N. R. T., Gonzalez, F., Jopia-Contreras, P., Aspé, E., Briones, H. U., et al. (2022). Anaerobic biofilm enriched with an ammonia tolerant methanogenic consortium to improve wastewater treatment in the fishing industry. Biotechnol. Lett. 44, 239–251. doi: 10.1007/s10529-021-03213-y
Alengebawy, A., Mohamed, B. A., Ghimire, N., Jin, K., Liu, T., Samer, M., et al. (2022). Understanding the environmental impacts of biogas utilization for energy production through life cycle assessment: An action towards reducing emissions. Environ. Res. 213:113632. doi: 10.1016/j.envres.2022.113632
Alghashm, S., Song, L., Liu, L., Ouyang, C., Zhou, J. L., and Li, X. (2023). Improvement of biogas production using biochar from Digestate at different pyrolysis temperatures during OFMSW anaerobic digestion. Sustainability (Switzerland) 15, 1–15. doi: 10.3390/su151511917
Ali Shah, F., Mahmood, Q., Maroof Shah, M., Pervez, A., and Ahmad Asad, S. (2014). Microbial ecology of anaerobic digesters: the key players of Anaerobiosis. Sci. World J. 2014, 1–21. doi: 10.1155/2014/183752
Alneberg, J., Bjarnason, B. S., de Bruijn, I., Schirmer, M., Quick, J., Ijaz, U. Z., et al. (2014). Binning metagenomic contigs by coverage and composition. Nat. Methods 11, 1144–1146. doi: 10.1038/nmeth.3103
Araoye, T. O., Mgbachi, C., Ajenikoko, G. A., Oluwaseun, A. T., Mgbachi, C. A., and Okelola, M. O. (2018). A comparative analysis of renewable energy using biogas and solar photovoltaic systems: a case study of Ajaba, in Osun State.
Asgarineshat, S., Arumugam, K., Shankari Chandra Segaran, U., Sekar, P., and Anika, A., (2022). A genome-centric metagenomics approach to explain microbial community structure in anaerobic digesters. Available at: https://hdl.handle.net/10356/156928 (Accessed September 10, 2024).
Athanasopoulou, K., Boti, M. A., Adamopoulos, P. G., Skourou, P. C., and Scorilas, A. (2021). Third-generation sequencing: the spearhead towards the radical transformation of modern genomics. Life 12:30. doi: 10.3390/life12010030
Bankevich, A., Nurk, S., Antipov, D., Gurevich, A. A., Dvorkin, M., Kulikov, A. S., et al. (2012). SPAdes: a new genome assembly algorithm and its applications to single-cell sequencing. J. Comput. Biol. 19, 455–477. doi: 10.1089/cmb.2012.0021
Becker, D., Popp, D., Bonk, F., Kleinsteuber, S., Harms, H., and Centler, F. (2023). Metagenomic analysis of anaerobic microbial communities degrading short-chain fatty acids as sole carbon sources. Microorganisms 11:420. doi: 10.3390/microorganisms11020420
Bedoya, K., Coltell-Simon, O., Cabarcas, F., and Alzate, J. F. (2019). Metagenomic assessment of the microbial community and methanogenic pathways in biosolids from a municipal wastewater treatment plant in Medellín, Colombia. Sci. Total Environ. 648, 572–581. doi: 10.1016/j.scitotenv.2018.08.119
Beghini, F., McIver, L. J., Blanco-Míguez, A., Dubois, L., Asnicar, F., Maharjan, S., et al. (2021). Integrating taxonomic, functional, and strain-level profiling of diverse microbial communities with biobakery 3. eLife 10:e65088. doi: 10.7554/eLife.65088
Bharathiraja, B., Sudharsana, T., Jayamuthunagai, J., Praveenkumar, R., Chozhavendhan, S., and Iyyappan, J. (2018). Biogas production – a review on composition, fuel properties, feed stock and principles of anaerobic digestion. Renew. Sust. Energ. Rev. 90, 570–582. doi: 10.1016/j.rser.2018.03.093
Bi, S., Westerholm, M., Hu, W., Mahdy, A., Dong, T., Sun, Y., et al. (2021). The metabolic performance and microbial communities of anaerobic digestion of chicken manure under stressed ammonia condition: a case study of a 10-year successful biogas plant. Renew. Energy 167, 644–651. doi: 10.1016/j.renene.2020.11.133
Blades, L., Morgan, K., Douglas, R., Glover, S., De Rosa, M., Cromie, T., et al. (2017). Circular biogas-based economy in a rural agricultural setting. Energy Procedia 123, 89–96. doi: 10.1016/j.egypro.2017.07.255
Bolyen, E., Rideout, J. R., Dillon, M. R., Bokulich, N. A., Abnet, C. C., Al-Ghalith, G. A., et al. (2019). Reproducible, interactive, scalable and extensible microbiome data science using QIIME 2. Nat. Biotechnol. 37, 852–857. doi: 10.1038/s41587-019-0209-9
Bovio-Winkler, P., Cabezas, A., and Etchebehere, C. (2021). Database mining to unravel the ecology of the phylum Chloroflexi in methanogenic full scale bioreactors. Front. Microbiol. 11:603234. doi: 10.3389/fmicb.2020.603234
Brandt, C., Bongcam-Rudloff, E., and Müller, B. (2020). Abundance tracking by long-read Nanopore sequencing of complex microbial communities in samples from 20 different biogas/wastewater plants. Appl. Sci. 10:7518. doi: 10.3390/app10217518
Bucci, L., Ghiotto, G., Zampieri, G., Raga, R., Favaro, L., Treu, L., et al. (2024). Adaptation of anaerobic digestion microbial communities to high ammonium levels: insights from strain-resolved metagenomics. Environ. Sci. Technol. 58, 580–590. doi: 10.1021/acs.est.3c07737
Callaghan, M. (2023). Cloud computing for metagenomics: building a personalized computational platform for pipeline analyses. Methods Mol. Biol. 2649, 261–279. doi: 10.1007/978-1-0716-3072-3_13
Campanaro, S., Treu, L., Kougias, P. G., Luo, G., and Angelidaki, I. (2018). Metagenomic binning reveals the functional roles of core abundant microorganisms in twelve full-scale biogas plants. Water Res. 140, 123–134. doi: 10.1016/j.watres.2018.04.043
Campanaro, S., Treu, L., Rodriguez, L. M. R., Kovalovszki, A., Ziels, R. M., Maus, I., et al. (2019). The anaerobic digestion microbiome: a collection of 1600 metagenome-assembled genomes shows high species diversity related to methane production. bioRxiv. doi: 10.1101/680553
Case, R. J., Boucher, Y., Dahllöf, I., Holmström, C., Doolittle, W. F., and Kjelleberg, S. (2007). Use of 16S rRNA and rpoB genes as molecular markers for microbial ecology studies. Appl. Environ. Microbiol. 73, 278–288. doi: 10.1128/AEM.01177-06
Cattaneo, C. R., Rodríguez, Y., Rene, E. R., García-Depraect, O., and Muñoz, R. (2022). Biogas bioconversion into poly(3-hydroxybutyrate) by a mixed microbial culture in a novel Taylor flow bioreactor. Waste Manag. 150, 364–372. doi: 10.1016/j.wasman.2022.07.017
Chen, Z., Erickson, D. L., and Meng, J. (2020). Benchmarking hybrid assembly approaches for genomic analyses of bacterial pathogens using Illumina and Oxford Nanopore sequencing. BMC Genomics 21:631. doi: 10.1186/s12864-020-07041-8
Chen, P., Guo, Y., Jia, L., Wan, J., He, T., Fang, C., et al. (2021). Interaction between functionally activate endometrial microbiota and host gene regulation in endometrial Cancer. Front. Cell Dev. Biol. 9:727286. doi: 10.3389/fcell.2021.727286
Chen, Z., Ma, J., Ma, J., Ye, J., Yu, Q., Zou, P., et al. (2024). Long-term biogas slurry application increases microbial necromass but not plant lignin contribution to soil organic carbon in paddy soils as regulated by fungal community. Waste Manag. 175, 254–264. doi: 10.1016/j.wasman.2024.01.011
Chen, X. Y., Vinh-Thang, H., Ramirez, A. A., Rodrigue, D., and Kaliaguine, S. (2015). Membrane gas separation technologies for biogas upgrading. RSC Adv. 5, 24399–24448. doi: 10.1039/C5RA00666J
Cheng, H., Medina, J. S., Zhou, J., Pinho, E. M., Meng, R., Wang, L., et al. (2024). Predicting anaerobic membrane bioreactor performance using flow-cytometry-derived high and low nucleic acid content cells. Environ. Sci. Technol. 58, 2360–2372. doi: 10.1021/acs.est.3c07702
Chukwuma, O. B., Rafatullah, M., Tajarudine, H. A., and Ismail, N. (2021). A review on bacterial contribution to lignocellulose breakdown into useful bio-products. Int. J. Environ. Res. Public Health 18:6001. doi: 10.3390/ijerph18116001
Coggill, P., Finn, R. D., and Bateman, A. (2008). Identifying protein domains with the Pfam database. Curr. Protoc. Bioinformatics 23. doi: 10.1002/0471250953.bi0205s23 [Epub ahead of print].
Cortés, A., Martin, J., Rosa, B. A., Stark, K. A., Clare, S., McCarthy, C., et al. (2022). The gut microbial metabolic capacity of microbiome-humanized vs. wild type rodents reveals a likely dual role of intestinal bacteria in hepato-intestinal schistosomiasis. PLoS Negl. Trop. Dis. 16:e0010878. doi: 10.1371/journal.pntd.0010878
Cuber, P., Chooneea, D., Geeves, C., Salatino, S., Creedy, T. J., Griffin, C., et al. (2023). Comparing the accuracy and efficiency of third generation sequencing technologies, Oxford Nanopore technologies, and Pacific biosciences, for DNA barcode sequencing applications. Ecol. Genet. Genom. 28:100181. doi: 10.1016/j.egg.2023.100181
De Bernardini, N., Basile, A., Zampieri, G., Kovalovszki, A., De Diego Diaz, B., Offer, E., et al. (2022). Integrating metagenomic binning with flux balance analysis to unravel syntrophies in anaerobic CO2 methanation. Microbiome 10:117. doi: 10.1186/s40168-022-01311-1
de Lima Procópio, R. E., da Silva, I. R., Martins, M. K., de Azevedo, J. L., and de Araújo, J. M. (2012). Antibiotics produced by Streptomyces. Braz. J. Infect. Dis. 16, 466–471. doi: 10.1016/j.bjid.2012.08.014
Douglas, G. M., Maffei, V. J., Zaneveld, J. R., Yurgel, S. N., Brown, J. R., Taylor, C. M., et al. (2020). PICRUSt2 for prediction of metagenome functions. Nat. Biotechnol. 38, 685–688. doi: 10.1038/s41587-020-0548-6
Eisenhofer, R., Nesme, J., Santos-Bay, L., Koziol, A., Sørensen, S. J., Alberdi, A., et al. (2024). A comparison of short-read, HiFi long-read, and hybrid strategies for genome-resolved metagenomics. Microbiol. Spectr. 12:e0359023. doi: 10.1128/spectrum.03590-23
Emrani, A., and Berrada, A. (2024). Modeling and optimal capacity configuration of dry gravity energy storage integrated in off-grid hybrid PV/wind/biogas plant incorporating renewable power generation forecast. J. Energy Storage 97, 1–20. doi: 10.1016/j.est.2024.112698
Fedailaine, M., Moussi, K., Khitous, M., Abada, S., Saber, M., and Tirichine, N. (2015). Modeling of the anaerobic digestion of organic waste for biogas production. Proc. Comput. Sci. 52, 730–737. doi: 10.1016/j.procs.2015.05.086
Fontana, A., Campanaro, S., Treu, L., Kougias, P. G., Cappa, F., Morelli, L., et al. (2018). Performance and genome-centric metagenomics of thermophilic single and two-stage anaerobic digesters treating cheese wastes. Water Res. 134, 181–191. doi: 10.1016/j.watres.2018.02.001
Gahlot, P., Aboudi, K., Ahmed, B., Tawfik, A., Khan, A. A., Khursheed, A., et al. (2021). Direct interspecies electron transfer (DIET) via conductive materials in anaerobic digestion of organic wastes. Clean Energy Resourc. Recov. 1, 227–252. doi: 10.1016/B978-0-323-85223-4.00024-5
Gaspari, M., Ghiotto, G., Centurion, V. B., Kotsopoulos, T., Santinello, D., Campanaro, S., et al. (2024). Decoding microbial responses to ammonia shock loads in biogas reactors through metagenomics and metatranscriptomics. Environ. Sci. Technol. 58, 591–602. doi: 10.1021/acs.est.3c07840
Ghiotto, G., De Bernardini, N., Giangeri, G., Tsapekos, P., Gaspari, M., Kougias, P. G., et al. (2024a). From microbial heterogeneity to evolutionary insights: a strain-resolved metagenomic study of H2S-induced changes in anaerobic biofilms. Chem. Eng. J. 485:9824. doi: 10.1016/j.cej.2024.149824
Ghiotto, G., Zampieri, G., Campanaro, S., and Treu, L. (2024b). Strain-resolved metagenomics approaches applied to biogas upgrading. Environ. Res. 240:117414. doi: 10.1016/j.envres.2023.117414
Golub, K. W., Smith, A. D., Hollister, E. B., Gentry, T. J., and Holtzapple, M. T. (2011). Investigation of intermittent air exposure on four-stage and one-stage anaerobic semi-continuous mixed-acid fermentations. Bioresour. Technol. 102, 5066–5075. doi: 10.1016/j.biortech.2011.02.011
Götz, M., Lefebvre, J., Mörs, F., McDaniel Koch, A., Graf, F., Bajohr, S., et al. (2016). Renewable power-to-gas: a technological and economic review. Renew. Energy 85, 1371–1390. doi: 10.1016/j.renene.2015.07.066
Greses, S., De Bernardini, N., Treu, L., Campanaro, S., and González-Fernández, C. (2023). Genome-centric metagenomics revealed the effect of pH on the microbiome involved in short-chain fatty acids and ethanol production. Bioresour. Technol. 377:128920. doi: 10.1016/j.biortech.2023.128920
Han, Y., Green, H., and Tao, W. (2020). Reversibility of propionic acid inhibition to anaerobic digestion: inhibition kinetics and microbial mechanism. Chemosphere 255:126840. doi: 10.1016/j.chemosphere.2020.126840
Han, G., Shin, S. G., Lee, J., Shin, J., and Hwang, S. (2017). A comparative study on the process efficiencies and microbial community structures of six full-scale wet and semi-dry anaerobic digesters treating food wastes. Bioresour. Technol. 245, 869–875. doi: 10.1016/j.biortech.2017.08.167
Häntze, H., and Horton, P. (2023). Effects of spaced k-mers on alignment-free genotyping. Bioinformatics 39, i213–i221. doi: 10.1093/bioinformatics/btad202
Hauptfeld, E., Pappas, N., van Iwaarden, S., Snoek, B. L., Aldas-Vargas, A., Dutilh, B. E., et al. (2024). Integrating taxonomic signals from MAGs and contigs improves read annotation and taxonomic profiling of metagenomes. Nat. Commun. 15:3373. doi: 10.1038/s41467-024-47155-1
Heyer, R., Benndorf, D., Kohrs, F., De Vrieze, J., Boon, N., Hoffmann, M., et al. (2016). Proteotyping of biogas plant microbiomes separates biogas plants according to process temperature and reactor type. Biotechnol. Biofuels 9, 1–16. doi: 10.1186/s13068-016-0572-4
Heyer, R., Hellwig, P., Maus, I., Walke, D., Schlüter, A., Hassa, J., et al. (2024). Breakdown of hardly degradable carbohydrates (lignocellulose) in a two-stage anaerobic digestion plant is favored in the main fermenter. Water Res. 250:1020. doi: 10.1016/j.watres.2023.121020
Huerta-Cepas, J., Szklarczyk, D., Heller, D., Hernández-Plaza, A., Forslund, S. K., Cook, H., et al. (2019). eggNOG 5.0: a hierarchical, functionally and phylogenetically annotated orthology resource based on 5090 organisms and 2502 viruses. Nucleic Acids Res. 47, D309–D314. doi: 10.1093/nar/gky1085
Izumi, K., Okishio, Y., Nagao, N., Niwa, C., Yamamoto, S., and Toda, T. (2010). Effects of particle size on anaerobic digestion of food waste. Int. Biodeterior. Biodegradation 64, 601–608. doi: 10.1016/j.ibiod.2010.06.013
Jain, S., Jain, S., Wolf, I. T., Lee, J., and Tong, Y. W. (2015). A comprehensive review on operating parameters and different pretreatment methodologies for anaerobic digestion of municipal solid waste. Renew. Sust. Energ. Rev. 52, 142–154. doi: 10.1016/j.rser.2015.07.091
James, A. R. S., and Richardson, R. E. (2020). Ecogenomics reveals community interactions in a long-term methanogenic bioreactor and a rapid switch to sulfate-reducing conditions. FEMS Microbiol. Ecol. 96:50. doi: 10.1093/FEMSEC/FIAA050
Jia, Y., Wilkins, D., Lu, H., Cai, M., and Lee, P. K. H. (2016). Long-term enrichment on cellulose or xylan causes functional and taxonomic convergence of microbial communities from anaerobic digesters. Appl. Environ. Microbiol. 82, 1519–1529. doi: 10.1128/AEM.03360-15
Jiang, F., Li, Q., Wang, S., Shen, T., Wang, H., Wang, A., et al. (2023). Recovery of metagenome-assembled microbial genomes from a full-scale biogas plant of food waste by pacific biosciences high-fidelity sequencing. Front. Microbiol. 13:1095497. doi: 10.3389/fmicb.2022.1095497
Johnravindar, D., Patria, R. D., Lee, J. T. E., Zhang, L., Tong, Y. W., Wang, C.-H., et al. (2021). Syntrophic interactions in anaerobic digestion: how biochar properties affect them? Sustain. Environ. 7:1945282. doi: 10.1080/27658511.2021.1945282
Jung, H., Yu, H., and Lee, C. (2023). Direct interspecies electron transfer enables anaerobic oxidation of sulfide to elemental sulfur coupled with CO2-reducing methanogenesis. iScience 26:107504. doi: 10.1016/j.isci.2023.107504
Kalamaras, S. D., Christou, M. L., Tzenos, C. A., Vasileiadis, S., Karpouzas, D. G., and Kotsopoulos, T. A. (2023). Investigation of the critical biomass of acclimated microbial communities to high Ammonia concentrations for a successful bioaugmentation of biogas anaerobic reactors with Ammonia inhibition. Microorganisms 11:1710. doi: 10.3390/microorganisms11071710
Kanagawa, T. (2003). Bias and artifacts in multitemplate polymerase chain reactions (PCR). J. Biosci. Bioeng. 96, 317–323. doi: 10.1016/S1389-1723(03)90130-7
Kang, D. D., Li, F., Kirton, E., Thomas, A., Egan, R., An, H., et al. (2019). MetaBAT 2: an adaptive binning algorithm for robust and efficient genome reconstruction from metagenome assemblies. PeerJ 7:e7359. doi: 10.7717/peerj.7359
Khanh Nguyen, V., Kumar Chaudhary, D., Hari Dahal, R., Hoang Trinh, N., Kim, J., Chang, S. W., et al. (2021). Review on pretreatment techniques to improve anaerobic digestion of sewage sludge. Fuel 285:119105. doi: 10.1016/j.fuel.2020.119105
Khanthong, K., Kadam, R., Kim, T., and Park, J. (2023). Synergetic effects of anaerobic co-digestion of food waste and algae on biogas production. Bioresour. Technol. 382:129208. doi: 10.1016/j.biortech.2023.129208
Kim, N. K., Lee, S. H., Kim, Y., and Park, H. D. (2022). Current understanding and perspectives in anaerobic digestion based on genome-resolved metagenomic approaches. Bioresour. Technol. 344:126350. doi: 10.1016/j.biortech.2021.126350
Knight, R., Vrbanac, A., Taylor, B. C., Aksenov, A., Callewaert, C., Debelius, J., et al. (2018). Best practices for analysing microbiomes. Nat. Rev. Microbiol. 16, 410–422. doi: 10.1038/s41579-018-0029-9
Kolmogorov, M., Yuan, J., Lin, Y., and Pevzner, P. A. (2019). Assembly of long, error-prone reads using repeat graphs. Nat. Biotechnol. 37, 540–546. doi: 10.1038/s41587-019-0072-8
Koren, S., Walenz, B. P., Berlin, K., Miller, J. R., Bergman, N. H., and Phillippy, A. M. (2017). Canu: scalable and accurate long-read assembly via adaptive k-mer weighting and repeat separation. Genome Res. 27, 722–736. doi: 10.1101/gr.215087.116
Krishna, D., and Kalamdhad, A. S. (2014). Pre-treatment and anaerobic digestion of food waste for high rate methane production - a review. J. Environ. Chem. Eng. 2, 1821–1830. doi: 10.1016/j.jece.2014.07.024
Lapierre, N., Alser, M., Eskin, E., Koslicki, D., and Mangul, S. (2020). Metalign: efficient alignment-based metagenomic profiling via containment min hash. Genome Biol. 21:242. doi: 10.1186/s13059-020-02159-0
Lei, Y., Sun, D., Dang, Y., Feng, X., Huo, D., Liu, C., et al. (2019). Metagenomic analysis reveals that activated carbon aids anaerobic digestion of raw incineration leachate by promoting direct interspecies electron transfer. Water Res. 161, 570–580. doi: 10.1016/j.watres.2019.06.038
Li, Y., Jing, Z., Pan, J., Luo, G., Feng, L., Jiang, H., et al. (2022). Multi-omics joint analysis of the effect of temperature on microbial communities, metabolism, and genetics in full-scale biogas reactors with food waste. Renew. Sust. Energ. Rev. 160:112261. doi: 10.1016/j.rser.2022.112261
Li, D., Liu, C.-M., Luo, R., Sadakane, K., and Lam, T.-W. (2015). MEGAHIT: an ultra-fast single-node solution for large and complex metagenomics assembly via succinct de Bruijn graph. Bioinformatics 31, 1674–1676. doi: 10.1093/bioinformatics/btv033
Li, L., Peng, X., Wang, X., and Wu, D. (2018). Anaerobic digestion of food waste: a review focusing on process stability. Bioresour. Technol. 248, 20–28. doi: 10.1016/j.biortech.2017.07.012
Li, X., Tong, J., Yuan, M., Song, M., Gao, J., Zhu, J., et al. (2023). Demonstrating the application of batch anaerobic digestion recirculating slurry inoculation of food waste engineering from a microbiological perspective. Renew. Energy 217:9216. doi: 10.1016/j.renene.2023.119216
Liang, B., Wang, L. Y., Mbadinga, S. M., Liu, J. F., Yang, S. Z., Gu, J. D., et al. (2015). Anaerolineaceae and Methanosaeta turned to be the dominant microorganisms in alkanes-dependent methanogenic culture after long-term of incubation. AMB Express 5:117. doi: 10.1186/s13568-015-0117-4
Lindner, B. G., Gerhardt, K., Feistel, D. J., Rodriguez-R, L. M., Hatt, J. K., and Konstantinidis, K. T. (2024). A user’s guide to the bioinformatic analysis of shotgun metagenomic sequence data for bacterial pathogen detection. Int. J. Food Microbiol. 410:110488. doi: 10.1016/j.ijfoodmicro.2023.110488
Lischer, H. E. L., and Shimizu, K. K. (2017). Reference-guided de novo assembly approach improves genome reconstruction for related species. BMC Bioinform. 18:474. doi: 10.1186/s12859-017-1911-6
Liu, D., Cen, R., Yuan, A., Wu, M., Luo, C., Chen, M., et al. (2024). Effects of continuous low-speed biogas agitation on anaerobic digestion of high-solids pig manure: performance and microbial community. J. Environ. Manag. 354:120355. doi: 10.1016/j.jenvman.2024.120355
Liu, J., Liu, F., and Pan, W. (2023). Improving the completeness of chromosome-level assembly by recalling sequences from lost Contigs. Genes (Basel) 14:1926. doi: 10.3390/genes14101926
Liu, Y., Wachemo, A. C., Yuan, H. R., and Li, X. J. (2019). Anaerobic digestion performance and microbial community structure of corn Stover in three-stage continuously stirred tank reactors. Bioresour. Technol. 287:121339. doi: 10.1016/j.biortech.2019.121339
Lv, L., Chen, J., Liu, X., Gao, W., Sun, L., Wang, P., et al. (2023). Roles and regulation of quorum sensing in anaerobic granular sludge: research status, challenges, and perspectives. Bioresour. Technol. 387:129644. doi: 10.1016/j.biortech.2023.129644
Malolan, V. V., Shereen, M. F., Dharaneesh, A. B., Ponnusami, V., Arumugam, A., and Sudalai, S. (2022). “Pilot plant and industrial-scale operations for biodiesel production,” in Production of Biodiesel from Non-Edible Sources, (Elsevier), 179–210.
Manjusha, C., and Beevi, B. S. (2016). Mathematical modeling and simulation of anaerobic digestion of solid waste. Proc. Technol. 24, 654–660. doi: 10.1016/j.protcy.2016.05.174
Mao, L., Tsui, T. H., Zhang, J., Dai, Y., and Tong, Y. W. (2021). Mixing effects on decentralized high-solid digester for horticultural waste: startup, operation and sensitive microorganisms. Bioresour. Technol. 333:125216. doi: 10.1016/j.biortech.2021.125216
Marchesi, J. R., and Ravel, J. (2015). The vocabulary of microbiome research: a proposal. Microbiome 3:31. doi: 10.1186/s40168-015-0094-5
Maspolim, Y., Zhou, Y., Guo, C., Xiao, K., and Ng, W. J. (2015). Comparison of single-stage and two-phase anaerobic sludge digestion systems - performance and microbial community dynamics. Chemosphere 140, 54–62. doi: 10.1016/j.chemosphere.2014.07.028
McAteer, P. G., Christine Trego, A., Thorn, C., Mahony, T., Abram, F., and O’Flaherty, V. (2020). Reactor configuration influences microbial community structure during high-rate, low-temperature anaerobic treatment of dairy wastewater. Bioresour. Technol. 307:123221. doi: 10.1016/j.biortech.2020.123221
Mehariya, S., Patel, A. K., Obulisamy, P. K., Punniyakotti, E., and Wong, J. W. C. (2018). Co-digestion of food waste and sewage sludge for methane production: current status and perspective. Bioresour. Technol. 265, 519–531. doi: 10.1016/j.biortech.2018.04.030
Michael, C., Jennifer, D., and Michael, O. (2015). Molecular Biology. 2nd Edn. New York, NY: W. H. Freeman & Co Ltd.
Murillo-Roos, M., Uribe-Lorío, L., Fuentes-Schweizer, P., Vidaurre-Barahona, D., Brenes-Guillén, L., Jiménez, I., et al. (2022). Biogas production and microbial communities of mesophilic and thermophilic anaerobic co-digestion of animal manures and food wastes in Costa Rica. Energies (Basel) 15:3252. doi: 10.3390/en15093252
Ong, M., Williams, R., and Kaffka, S. (2014). Comparative Assessment of Technology Options for Biogas Clean‐up. Contractor Report to the California Energy Commission. California. Available at: https://ucdavis.app.box.com/s/iaog2diybkvoe5xxdjud97xsrx3ypwmq (Accessed September 7, 2024).
Nobu, M. K., Narihiro, T., Rinke, C., Kamagata, Y., Tringe, S. G., Woyke, T., et al. (2015). Microbial dark matter ecogenomics reveals complex synergistic networks in a methanogenic bioreactor. ISME J. 9, 1710–1722. doi: 10.1038/ismej.2014.256
Oosterkamp, M. J., Bauer, S., Ibáñez, A. B., Méndez-García, C., Hong, P. Y., Cann, I., et al. (2019). Identification of methanogenesis and syntrophy as important microbial metabolic processes for optimal thermophilic anaerobic digestion of energy cane thin stillage. Bioresour. Technol. Rep. 7:100254. doi: 10.1016/j.biteb.2019.100254
Orakov, A., Fullam, A., Coelho, L. P., Khedkar, S., Szklarczyk, D., Mende, D. R., et al. (2021). GUNC: detection of chimerism and contamination in prokaryotic genomes. Genome Biol. 22:178. doi: 10.1186/s13059-021-02393-0
Paliy, O., and Shankar, V. (2016). Application of multivariate statistical techniques in microbial ecology. Mol. Ecol. 25, 1032–1057. doi: 10.1111/mec.13536
Palù, M., Basile, A., Zampieri, G., Treu, L., Rossi, A., Morlino, M. S., et al. (2022). KEMET – a python tool for KEGG module evaluation and microbial genome annotation expansion. Comput. Struct. Biotechnol. J. 20, 1481–1486. doi: 10.1016/j.csbj.2022.03.015
Parks, D. H., Chuvochina, M., Rinke, C., Mussig, A. J., Chaumeil, P.-A., and Hugenholtz, P. (2022). GTDB: an ongoing census of bacterial and archaeal diversity through a phylogenetically consistent, rank normalized and complete genome-based taxonomy. Nucleic Acids Res. 50, D785–D794. doi: 10.1093/nar/gkab776
Parks, D. H., Imelfort, M., Skennerton, C. T., Hugenholtz, P., and Tyson, G. W. (2015). CheckM: assessing the quality of microbial genomes recovered from isolates, single cells, and metagenomes. Genome Res. 25, 1043–1055. doi: 10.1101/gr.186072.114
Pasalari, H., Gholami, M., Rezaee, A., Esrafili, A., and Farzadkia, M. (2021). Perspectives on microbial community in anaerobic digestion with emphasis on environmental parameters: a systematic review. Chemosphere 270:128618. doi: 10.1016/j.chemosphere.2020.128618
Peces, M., Astals, S., Jensen, P. D., and Clarke, W. P. (2018). Deterministic mechanisms define the long-term anaerobic digestion microbiome and its functionality regardless of the initial microbial community. Water Res. 141, 366–376. doi: 10.1016/j.watres.2018.05.028
Pei, Z., Liu, S., Jing, Z., Zhang, Y., Wang, J., Liu, J., et al. (2022). Understanding of the interrelationship between methane production and microorganisms in high-solid anaerobic co-digestion using microbial analysis and machine learning. J. Clean. Prod. 373:3848. doi: 10.1016/j.jclepro.2022.133848
Piadeh, F., Offie, I., Behzadian, K., Rizzuto, J. P., Bywater, A., Córdoba-Pachón, J. R., et al. (2024). A critical review for the impact of anaerobic digestion on the sustainable development goals. J. Environ. Manag. 349:119458. doi: 10.1016/j.jenvman.2023.119458
Pore, S. D., Shetty, D., Arora, P., Maheshwari, S., and Dhakephalkar, P. K. (2016). Metagenome changes in the biogas producing community during anaerobic digestion of rice straw. Bioresour. Technol. 213, 50–53. doi: 10.1016/j.biortech.2016.03.045
Portik, D. M., Brown, C. T., and Pierce-Ward, N. T. (2022). Evaluation of taxonomic classification and profiling methods for long-read shotgun metagenomic sequencing datasets. BMC Bioinform. 23:541. doi: 10.1186/s12859-022-05103-0
Prosser, J. I. (2010). Replicate or lie. Environ. Microbiol. 12, 1806–1810. doi: 10.1111/j.1462-2920.2010.02201.x
Quince, C., Nurk, S., Raguideau, S., James, R., Soyer, O. S., Summers, J. K., et al. (2021). STRONG: metagenomics strain resolution on assembly graphs. Genome Biol. 22, 1–34. doi: 10.1186/S13059-021-02419-7/FIGURES/9
Raboni, M., and Viotti, P. (2016). Formation and destruction of polycyclic aromatic hydrocarbons (PAHs) in the flaring of the biogas collected from an automotive shredded residues landfill. Ambien. Agua Interdiscip. J. Appl. Sci. 11, 1–9. doi: 10.4136/ambi-agua.1758
Ramette, A. (2007). Multivariate analyses in microbial ecology. FEMS Microbiol. Ecol. 62, 142–160. doi: 10.1111/j.1574-6941.2007.00375.x
Rao, M., Zou, X., Huang, Z., Ye, J., Huang, C., Zhang, M., et al. (2024). Purification of anaerobic fermentation digestate of dairy manure by Arthrospira biomass cultivated with biogas-CO2 fixation. Biochem. Eng. J. 203:109178. doi: 10.1016/j.bej.2023.109178
Ren, J., Yuan, X., Li, J., Ma, X., Zhao, Y., Zhu, W., et al. (2014). Performance and microbial community dynamics in a two-phase anaerobic co-digestion system using cassava dregs and pig manure. Bioresour. Technol. 155, 342–351. doi: 10.1016/j.biortech.2013.12.120
Rhee, C., Kim, D. W., Yu, S. I., Lee, M. E., Shin, J., Kim, H. W., et al. (2021). Biogas potential assessment and characterization of Korean slaughterhouse waste for anaerobic digestion. Environ. Technol. Innov. 24:1858. doi: 10.1016/j.eti.2021.101858
Robinson, K. M., Hawkins, A. S., Santana-Cruz, I., Adkins, R. S., Shetty, A. C., Nagaraj, S., et al. (2017). Aligner optimization increases accuracy and decreases compute times in multi-species sequence data. Microb. Genom. 3:122. doi: 10.1099/mgen.0.000122
Saha, S., Jeon, B. H., Kurade, M. B., Govindwar, S. P., Chatterjee, P. K., Oh, S. E., et al. (2019). Interspecies microbial nexus facilitated methanation of polysaccharidic wastes. Bioresour. Technol. 289:121638. doi: 10.1016/j.biortech.2019.121638
Samuel, P. O. (2015). Production of biogas from perennial and biennial crop wastes: peach palm and Banana’s wastes as alternative biomass in energy generation and environmental Susteinability. Am. J. Environ. Eng. 5, 79–89. doi: 10.5923/j.ajee.20150504.01
Schwan, B., Abendroth, C., Latorre-Pérez, A., Porcar, M., Vilanova, C., and Dornack, C. (2020). Chemically stressed bacterial communities in anaerobic digesters exhibit resilience and ecological flexibility. Front. Microbiol. 11:867. doi: 10.3389/fmicb.2020.00867
Sepúlveda, G., Jaimes, L. E., Pacheco, L., and Díaz, C. A. (2018). Simulation of a biogas cleaning process using different amines. Rev. Facult. Ingeniería 27, 51–60. doi: 10.19053/01211129.v27.n47.2018.7751
Setubal, J. C. (2021). Metagenome-assembled genomes: concepts, analogies, and challenges. Biophys. Rev. 13:905. doi: 10.1007/s12551-021-00865-y
Shah, S. V., Yadav Lamba, B., Tiwari, A. K., and Chen, W. H. (2024). Sustainable biogas production via anaerobic digestion with focus on CSTR technology: a review. J. Taiwan Inst. Chem. Eng. 162:105575. doi: 10.1016/j.jtice.2024.105575
Sims, D., Sudbery, I., Ilott, N. E., Heger, A., and Ponting, C. P. (2014). Sequencing depth and coverage: key considerations in genomic analyses. Nat. Rev. Genet. 15, 121–132. doi: 10.1038/nrg3642
Singleton, C. M., Petriglieri, F., Kristensen, J. M., Kirkegaard, R. H., Michaelsen, T. Y., Andersen, M. H., et al. (2021). Connecting structure to function with the recovery of over 1000 high-quality metagenome-assembled genomes from activated sludge using long-read sequencing. Nat. Commun. 12:2009. doi: 10.1038/s41467-021-22203-2
Smith, B. J., Li, X., Shi, Z. J., Abate, A., and Pollard, K. S. (2022). Scalable microbial strain inference in metagenomic data using StrainFacts. Front. Bioinform. 2:867386. doi: 10.3389/fbinf.2022.867386
Song, C., Li, W., Cai, F., Liu, G., and Chen, C. (2021). Anaerobic and microaerobic pretreatment for improving methane production from paper waste in anaerobic digestion. Front. Microbiol. 12:688290. doi: 10.3389/fmicb.2021.688290
Stolze, Y., Zakrzewski, M., Maus, I., Eikmeyer, F., Jaenicke, S., Rottmann, N., et al. (2015). Comparative metagenomics of biogas-producing microbial communities from production-scale biogas plants operating under wet or dry fermentation conditions. Biotechnol. Biofuels 8:14. doi: 10.1186/s13068-014-0193-8
Su, C., Deng, Q., Lu, Y., Qin, R., Chen, S., Wei, J., et al. (2019). Effects of hydraulic retention time on the performance and microbial community of an anaerobic baffled reactor-bioelectricity Fenton coupling reactor for treatment of traditional Chinese medicine wastewater. Bioresour. Technol. 288:121508. doi: 10.1016/j.biortech.2019.121508
Su, K., Li, L., Wang, Q., and Cao, R. (2023). A review on the interspecies electron transfer of methane production in anaerobic digestion system. Fermentation 9:467. doi: 10.3390/fermentation9050467
Subbarao, P. M. V., D’ Silva, T. C., Adlak, K., Kumar, S., Chandra, R., and Vijay, V. K. (2023). Anaerobic digestion as a sustainable technology for efficiently utilizing biomass in the context of carbon neutrality and circular economy. Environ. Res. 234:116286. doi: 10.1016/j.envres.2023.116286
Sun, M., Zhang, C., Shi, Z., Zhang, C., Zhang, S., and Luo, G. (2023). Genome-centric metagenomic analysis revealed the microbial shifts in response to long-chain fatty acids (LCFA) in anaerobic digestion with hydrochar. Chem. Eng. J. 466:3249. doi: 10.1016/j.cej.2023.143249
Sun, Z., Zhao, L., Wu, J. T., Wang, Z. H., Wu, K. K., Chen, C., et al. (2023). Exogenous hydrogen supply improves in-situ biogas upgrading of sewage sludge: performance and mechanisms. Chem. Eng. J. 477:7307. doi: 10.1016/j.cej.2023.147307
Tabatabaei, M., Aghbashlo, M., Valijanian, E., Kazemi Shariat Panahi, H., Nizami, A.-S., Ghanavati, H., et al. (2020). A comprehensive review on recent biological innovations to improve biogas production, part 1: upstream strategies. Renew. Energy 146, 1204–1220. doi: 10.1016/j.renene.2019.07.037
Taş, N., de Jong, A. E., Li, Y., Trubl, G., Xue, Y., and Dove, N. C. (2021). Metagenomic tools in microbial ecology research. Curr. Opin. Biotechnol. 67, 184–191. doi: 10.1016/j.copbio.2021.01.019
Thi, N. B. D., Lin, C. Y., and Kumar, G. (2016). Electricity generation comparison of food waste-based bioenergy with wind and solar powers: a mini review. Sustain. Environ. Res. 26, 197–202. doi: 10.1016/j.serj.2016.06.001
Tijani, H., Yuzir, A., and Abdullah, N. (2018). Producing desulfurized biogas using two-stage domesticated shear-loop anaerobic contact stabilization system. Waste Manag. 78, 770–780. doi: 10.1016/j.wasman.2018.06.045
Trego, A. C., McAteer, P. G., Nzeteu, C., Mahony, T., Abram, F., Ijaz, U. Z., et al. (2021). Combined stochastic and deterministic processes drive community assembly of anaerobic microbiomes during granule flotation. Front. Microbiol. 12:666584. doi: 10.3389/fmicb.2021.666584
Treu, L., Campanaro, S., Kougias, P. G., Sartori, C., Bassani, I., and Angelidaki, I. (2018). Hydrogen-fueled microbial pathways in biogas upgrading systems revealed by genome-centric metagenomics. Front. Microbiol. 9:1079. doi: 10.3389/fmicb.2018.01079
Treu, L., Kougias, P. G., Campanaro, S., Bassani, I., and Angelidaki, I. (2016). Deeper insight into the structure of the anaerobic digestion microbial community; the biogas microbiome database is expanded with 157 new genomes. Bioresour. Technol. 216, 260–266. doi: 10.1016/j.biortech.2016.05.081
Tsapekos, P., Kougias, P. G., Treu, L., Campanaro, S., and Angelidaki, I. (2017). Process performance and comparative metagenomic analysis during co-digestion of manure and lignocellulosic biomass for biogas production. Appl. Energy 185, 126–135. doi: 10.1016/j.apenergy.2016.10.081
Turaev, D., and Rattei, T. (2016). High definition for systems biology of microbial communities: metagenomics gets genome-centric and strain-resolved. Curr. Opin. Biotechnol. 39, 174–181. doi: 10.1016/j.copbio.2016.04.011
Vaser, R., Sović, I., Nagarajan, N., and Šikić, M. (2017). Fast and accurate de novo genome assembly from long uncorrected reads. Genome Res. 27, 737–746. doi: 10.1101/gr.214270.116
Vendruscolo, E. C. G., Mesa, D., Rissi, D. V., Meyer, B. H., de Oliveira Pedrosa, F., de Souza, E. M., et al. (2020). Microbial communities network analysis of anaerobic reactors fed with bovine and swine slurry. Sci Total Environ. 742. doi: 10.1016/j.scitotenv.2020.140314
Venkiteshwaran, K., Milferstedt, K., Hamelin, J., Fujimoto, M., Johnson, M., and Zitomer, D. H. (2017). Correlating methane production to microbiota in anaerobic digesters fed synthetic wastewater. Water Res. 110, 161–169. doi: 10.1016/j.watres.2016.12.010
Villante, C., and Genovese, A. (2012). Hydromethane: a bridge towards the hydrogen economy or an unsustainable promise? Int. J. Hydrog. Energy 37, 11541–11548. doi: 10.1016/j.ijhydene.2012.03.066
Wang, Y., Li, G., Chi, M., Sun, Y., Zhang, J., Jiang, S., et al. (2018). Effects of co-digestion of cucumber residues to corn Stover and pig manure ratio on methane production in solid state anaerobic digestion. Bioresour. Technol. 250, 328–336. doi: 10.1016/j.biortech.2017.11.055
Wang, P., Wang, H., Qiu, Y., Ren, L., and Jiang, B. (2018). Microbial characteristics in anaerobic digestion process of food waste for methane production–a review. Bioresour. Technol. 248, 29–36. doi: 10.1016/j.biortech.2017.06.152
Wang, Z., You, R., Han, H., Liu, W., Sun, F., and Zhu, S. (2024). Effective binning of metagenomic contigs using contrastive multi-view representation learning. Nat. Commun. 15:585. doi: 10.1038/s41467-023-44290-z
Wang, M., Zhou, J., Yuan, Y. X., Dai, Y. M., Li, D., Li, Z. D., et al. (2017). Methane production characteristics and microbial community dynamics of mono-digestion and co-digestion using corn stalk and pig manure. Int. J. Hydrog. Energy 42, 4893–4901. doi: 10.1016/j.ijhydene.2016.10.144
Wang, S., Zhuang, X., Dong, W., Xin, F., Jia, H., and Wu, X. (2023). Advances in the application of quorum sensing to regulate electrode biofilms in bioelectrochemical systems. Fermentation 9:625. doi: 10.3390/fermentation9070625
Wei, Y., Zhao, H., Qi, X., Yang, T., Zhang, J., Chen, W., et al. (2023). Direct interspecies electron transfer stimulated by coupling of modified anaerobic granular sludge with microbial electrolysis cell for biogas production enhancement. Appl. Energy 341:121100. doi: 10.1016/j.apenergy.2023.121100
Wemheuer, F., Taylor, J. A., Daniel, R., Johnston, E., Meinicke, P., Thomas, T., et al. (2020). Tax4Fun2: prediction of habitat-specific functional profiles and functional redundancy based on 16S rRNA gene sequences. Environ.l Microb. 15:11. doi: 10.1186/s40793-020-00358-7
Wick, R. R., Judd, L. M., Gorrie, C. L., and Holt, K. E. (2017a). Completing bacterial genome assemblies with multiplex MinION sequencing. Microb. Genom. 3:132. doi: 10.1099/mgen.0.000132
Wick, R. R., Judd, L. M., Gorrie, C. L., and Holt, K. E. (2017b). Unicycler: resolving bacterial genome assemblies from short and long sequencing reads. PLoS Comput. Biol. 13:e1005595. doi: 10.1371/journal.pcbi.1005595
Wirth, R., Lakatos, G., Böjti, T., Maróti, G., Bagi, Z., Kis, M., et al. (2015). Metagenome changes in the mesophilic biogas-producing community during fermentation of the green alga Scenedesmus obliquus. J. Biotechnol. 215, 52–61. doi: 10.1016/j.jbiotec.2015.06.396
Wu, Y.-W., Simmons, B. A., and Singer, S. W. (2016). MaxBin 2.0: an automated binning algorithm to recover genomes from multiple metagenomic datasets. Bioinformatics 32, 605–607. doi: 10.1093/bioinformatics/btv638
Xiao, T., and Zhou, W. (2020). The third generation sequencing: the advanced approach to genetic diseases. Transl. Pediatr. 9, 163–173. doi: 10.21037/tp.2020.03.06
Xie, H., Yang, C., Sun, Y., Igarashi, Y., Jin, T., and Luo, F. (2020). PacBio long reads improve metagenomic assemblies, gene catalogs, and genome binning. Front. Genet. 11:516269. doi: 10.3389/fgene.2020.516269
Xu, R., Yang, Z.-H., Zheng, Y., Liu, J.-B., Xiong, W.-P., Zhang, Y.-R., et al. (2018a). Organic loading rate and hydraulic retention time shape distinct ecological networks of anaerobic digestion related microbiome. Bioresour. Technol. 262, 184–193. doi: 10.1016/j.biortech.2018.04.083
Xu, R., Zhang, K., Liu, P., Khan, A., Xiong, J., Tian, F., et al. (2018b). A critical review on the interaction of substrate nutrient balance and microbial community structure and function in anaerobic co-digestion. Bioresour. Technol. 247, 1119–1127. doi: 10.1016/j.biortech.2017.09.095
Yang, C., Chowdhury, D., Zhang, Z., Cheung, W. K., Lu, A., Bian, Z., et al. (2021). A review of computational tools for generating metagenome-assembled genomes from metagenomic sequencing data. Comput. Struct. Biotechnol. J. 19, 6301–6314. doi: 10.1016/j.csbj.2021.11.028
Yang, S.-F., Lu, C.-W., Yao, C.-T., and Hung, C.-M. (2019). To trim or not to trim: effects of read trimming on the de novo genome assembly of a widespread east Asian passerine, the rufous-capped babbler (Cyanoderma ruficeps Blyth). Genes (Basel) 10:737. doi: 10.3390/genes10100737
Yang, B., Wang, Y., and Qian, P.-Y. (2016). Sensitivity and correlation of hypervariable regions in 16S rRNA genes in phylogenetic analysis. BMC Bioinform. 17:135. doi: 10.1186/s12859-016-0992-y
Ye, M., Liu, J., Ma, C., Li, Y. Y., Zou, L., Qian, G., et al. (2018). Improving the stability and efficiency of anaerobic digestion of food waste using additives: a critical review. J. Clean. Prod. 192, 316–326. doi: 10.1016/j.jclepro.2018.04.244
Zhang, Y., Chen, K., and Fu, X. (2019). Scientific effects of triple Helix interactions among research institutes, industries and universities. Technovation 86–87, 33–47. doi: 10.1016/j.technovation.2019.05.003
Zhang, Q., Fan, N. S., Fu, J. J., Huang, B. C., and Jin, R. C. (2021). Role and application of quorum sensing in anaerobic ammonium oxidation (anammox) process: a review. Crit. Rev. Environ. Sci. Technol. 51, 626–648. doi: 10.1080/10643389.2020.1738166
Zhang, L., Kuroki, A., Loh, K. C., Seok, J. K., Dai, Y., and Tong, Y. W. (2020a). Highly efficient anaerobic co-digestion of food waste and horticultural waste using a three-stage thermophilic bioreactor: performance evaluation, microbial community analysis, and energy balance assessment. Energy Convers. Manag. 223:113290. doi: 10.1016/j.enconman.2020.113290
Zhang, L., Lim, E. Y., Loh, K. C., Ok, Y. S., Lee, J. T. E., Shen, Y., et al. (2020b). Biochar enhanced thermophilic anaerobic digestion of food waste: focusing on biochar particle size, microbial community analysis and pilot-scale application. Energy Convers. Manag. 209:112654. doi: 10.1016/j.enconman.2020.112654
Zhang, L., Loh, K. C., Lim, J. W., and Zhang, J. (2019a). Bioinformatics analysis of metagenomics data of biogas-producing microbial communities in anaerobic digesters: a review. Renew. Sust. Energ. Rev. 100, 110–126. doi: 10.1016/j.rser.2018.10.021
Zhang, L., Loh, K. C., Lim, J. W., and Zhang, J. (2023). Uncovering microbiome adaptations in a full-scale biogas plant: insights from MAG-centric metagenomics and metaproteomics. Microorganisms 11:2412. doi: 10.3390/microorganisms11102412
Zhang, L., Loh, K. C., Zhang, J., Mao, L., Tong, Y. W., Wang, C. H., et al. (2019b). Three-stage anaerobic co-digestion of food waste and waste activated sludge: identifying bacterial and methanogenic archaeal communities and their correlations with performance parameters. Bioresour. Technol. 285:121333. doi: 10.1016/j.biortech.2019.121333
Zhang, L., Tsui, T. H., Tong, Y. W., Aggarangsi, P., and Liu, R. (2024). Applying current-carrying-coil-based magnetic field (CCC-MF) to promote anaerobic digestion of chicken manure: performance evaluation, mitigation of ammonia inhibition, microbial community analysis, and pilot-scale validation. Energy Convers. Manag. 300:7908. doi: 10.1016/j.enconman.2023.117908
Zhang, X., Wang, Y., Jiao, P., Zhang, M., Deng, Y., Jiang, C., et al. (2023). Microbiome-functionality in anaerobic digesters: a critical review. Water Res. 249:120891. doi: 10.1016/j.watres.2023.120891
Zhang, S., Xiao, M., Liang, C., Chui, C., Wang, N., Shi, J., et al. (2022). Multivariate insights into enhanced biogas production in thermophilic dry anaerobic co-digestion of food waste with kitchen waste or garden waste: process properties, microbial communities and metagenomic analyses. Bioresour. Technol. 361:127684. doi: 10.1016/j.biortech.2022.127684
Zhao, W., Chen, X., Ma, H., Li, D., Yang, H., Hu, T., et al. (2024). Impact of co-substrate molecular weight on methane production potential, microbial community dynamics, and metabolic pathways in waste activated sludge anaerobic co-digestion. Bioresour. Technol. 400:130678. doi: 10.1016/j.biortech.2024.130678
Zhao, Y., Hu, K., Yu, J., Khan, M. T. A., Cai, Y., Zhao, X., et al. (2023). Biogas residues improved microbial diversity and disease suppression function under extent indigenous soil microbial biomass. Life 13:774. doi: 10.3390/life13030774
Zhao, C., Mu, H., Zhao, Y., Wang, L., and Zuo, B. (2018). Microbial characteristics analysis and kinetic studies on substrate composition to methane after microbial and nutritional regulation of fruit and vegetable wastes anaerobic digestion. Bioresour. Technol. 249, 315–321. doi: 10.1016/j.biortech.2017.10.041
Zhou, J., Smith, J. A., Li, M., and Holmes, D. E. (2023). Methane production by Methanothrix thermoacetophila via direct interspecies electron transfer with Geobacter metallireducens. MBio 14:e0036023. doi: 10.1128/mbio.00360-23
Zhou, Z., Tran, P. Q., Breister, A. M., Liu, Y., Kieft, K., Cowley, E. S., et al. (2022). METABOLIC: high-throughput profiling of microbial genomes for functional traits, metabolism, biogeochemistry, and community-scale functional networks. Microbiome 10:33. doi: 10.1186/s40168-021-01213-8
Zhu, X., Campanaro, S., Treu, L., Kougias, P. G., and Angelidaki, I. (2019). Novel ecological insights and functional roles during anaerobic digestion of saccharides unveiled by genome-centric metagenomics. Water Res. 151, 271–279. doi: 10.1016/j.watres.2018.12.041
Zimin, A. V., Marçais, G., Puiu, D., Roberts, M., Salzberg, S. L., and Yorke, J. A. (2013). The MaSuRCA genome assembler. Bioinformatics 29, 2669–2677. doi: 10.1093/bioinformatics/btt476
Keywords: methane, metagenome, microbiota, syntrophy, sequencing, DIET, GCM, SRM
Citation: Ostos I, Flórez-Pardo LM and Camargo C (2024) A metagenomic approach to demystify the anaerobic digestion black box and achieve higher biogas yield: a review. Front. Microbiol. 15:1437098. doi: 10.3389/fmicb.2024.1437098
Edited by:
Claudia Etchebehere, Instituto de Investigaciones Biológicas Clemente Estable (IIBCE), UruguayReviewed by:
Marcelo Navarro-Díaz, University of Tübingen, GermanyDaniel De Los Cobos Vasconcelos, National Autonomous University of Mexico, Mexico
Copyright © 2024 Ostos, Flórez-Pardo and Camargo. This is an open-access article distributed under the terms of the Creative Commons Attribution License (CC BY). The use, distribution or reproduction in other forums is permitted, provided the original author(s) and the copyright owner(s) are credited and that the original publication in this journal is cited, in accordance with accepted academic practice. No use, distribution or reproduction is permitted which does not comply with these terms.
*Correspondence: Iván Ostos, aW9zdG9zODNAZ21haWwuY29t