- 1ICAR-Central Inland Fisheries Research Institute, Regional Centre, Guwahati, Assam, India
- 2ICAR-Central Inland Fisheries Research Institute, Kolkata, India
- 3ICAR-Central Institute of Fisheries Education, Mumbai, Maharashtra, India
Introduction: The Brahmaputra, a major transboundary river of the Himalayas flowing predominantly through Northeast India, particularly Assam, is increasingly endangered by contamination due to rapid urbanization and anthropogenic pressures. These environmental changes pose significant risks at the microbial level, affecting nutrient cycling and productivity, and thereby impacting river ecosystem health. The next-generation sequencing technology using a metagenomics approach has revolutionized our understanding of the microbiome and its critical role in various aquatic environments.
Methods: The present study aimed to investigate the structure of the bacterial community and its functional potentials within the sediments of the Brahmaputra River, India, using high-throughput shotgun metagenomics. Additionally, this study sought to explore the presence of antimicrobial resistance genes in the river’s sediment.
Results and discussion: Shotgun metagenomics revealed a diverse bacterial community comprising 31 phyla, 52 classes, 291 families, 1,016 genera, and 3,630 species. Dominant phyla included Pseudomonadota (62.47–83.48%), Actinobacteria (11.10–24.89%), Bacteroidetes (0.97–3.82%), Firmicutes (0.54–3.94%), Cyanobacteria (0.14–1.70%), and Planctomycetes (0.30–0.78%). Functional profiling highlighted significant involvement in energy metabolism, amino acid and central carbon metabolism, stress response, and degradation pathways, emphasizing the microbial community’s role in ecosystem functioning and resilience. Notably, 50 types of antibiotic resistance genes (ARGs) were detected, with resistance profiles spanning multidrug, aminoglycoside, β-lactam, fluoroquinolone, rifampicin, sulfonamide, and tetracycline classes. Network analysis underscored the intricate relationships among ARG subtypes, suggesting potential mechanisms of resistance propagation. Furthermore, plasmid-related genes and 185 virulence factor genes (VFGs) were identified, indicating additional layers of microbial adaptation and potential pathogenicity within the river sediments. This comprehensive microbial and functional profiling of the Brahmaputra’s sediment metagenome provides crucial insights into microbial diversity, resistance potential, and ecological functions, offering a foundation for informed management and mitigation strategies to preserve river health and mitigate pollution impacts.
Introduction
The Himalayan Brahmaputra River is one of the world’s largest transboundary river systems, flowing through India (33.6%), China (50.5%), Bangladesh (8.1%), and Bhutan (7.8%) originating from the massive glacier mass in the southern Tibetan Kailash range (Singh et al., 2004). In India, the river flows for 916 km, entering through Northeast India. A major portion, approximately 640 km, flows through Assam, where the river gets the name Brahmaputra, flowing along the southern and northern banks (Datta and Singh, 2004). Large water volumes, considerable silt, rapid bed aggradations, continuous changes in channel morphology, and bank line erosion are some of the characteristics that define the mighty river (NDMA, 2012). The river is the fifth largest in terms of flow and serves as the primary water source for over 130 million people, providing essential space and resources (Mahanta et al., 2014; Ray et al., 2015). The river system is experiencing increased stress as a result of rapid industrialization and urbanization occurring in and around the river (Bhattacharjya et al., 2017). The river flowing through urban areas are severely endangered and contaminated due to anthropogenic activities and inadequate waste management. In recent years, pollution levels in the Brahmaputra River have increased due to population growth and increased water utility demands. With an average annual discharge of 591 km3/year, the river produces approximately 179 million liters of sewage per day. Between 2006 and 2019, water turbidity increased significantly, and microplastic pollution reached extremely high levels during 2018–2019. These trends signal serious concerns regarding the impacts of pollution on both the environment and human health (Bora and Goswami, 2017; Rakhecha, 2020; Barbulescu et al., 2021).
Microbes in river ecosystems are crucial for recycling nutrients, mineralizing organic matter, maintaining the ecosystem quality, facilitating energy flow in the food chain, degrading heavy metals, and many other beneficial properties (Findlay, 2010; Madsen, 2011; Liu et al., 2012). River sediments are the primary site where both pollutants and microbes adhere and accumulate; hence, the abundance, diversity, and stability of microbes are greatly impacted by numerous anthropogenic pollutants that are present (Devarajan et al., 2015; Saxena et al., 2015; Beattie et al., 2020). The impact of anthropogenic pollution on the microbial population composition can help predict the health and functionality of the ecosystem (Galand et al., 2016; Santillan et al., 2019). However, it is challenging to completely comprehend the structure, function, and interactions in such complex aquatic systems using culture-based techniques. Metagenomics has emerged as the ideal technique for the comprehensive study of the taxonomic and functional profile of microbial communities. Whole-genome metagenomic sequencing has revealed an understanding of the taxonomic diversity of freshwater and marine microbial species worldwide (Vogel et al., 2009; Toyama et al., 2016).
Metagenomics sequencing has emerged as a handy tool for exploring the antibiotic resistance profiles of microbes in the aquatic ecosystem (Xu et al., 2018; Li et al., 2020; Das et al., 2020). The aquatic environment represents a reservoir of resistance bacteria or antibiotic resistance genes (ARGs) that could be transmissible to animals and humans (Wang et al., 2020; Zhuang et al., 2021). Aquatic systems significantly impact the accumulation and spread of antibiotic resistance bacteria and ARGs in sediments and freshwater (Pham et al., 2018; Zhao et al., 2018). Mobile genetic elements (MGEs) such as plasmids, which function as a vector of ARG transfer and shape ARG patterns in microbial communities, mediate the horizontal gene transfer of ARGs (Zhang et al., 2011; Mencía-Ares et al., 2020). In recent years, the presence of antimicrobial resistance and ARGs has been reported in various aquatic ecosystems, posing a risk to human health (Taylor et al., 2011; Reddy and Dubey, 2019). Similarly, virulence factor genes (VFGs) of pathogenic bacteria can be transferred to other bacteria through plasmids or phages, helping pathogens to cause infectious diseases (Wagner and Waldor, 2002; Chen et al., 2021; Kim et al., 2022). The metagenomics technique has advantages in exploring the presence of ARGs, MGEs, VFGs, and resistomes from various environments (Segata et al., 2012; Ma et al., 2014; Bashiardes et al., 2016; Sukumar et al., 2016). The investigation of ARGs and VFGs in such environments helps to understand the possible human health risks associated with genes that exist in these environments and have the ability to spread to other regions.
Furthermore, metagenomics studies have facilitated researchers to identify many beneficial microbes in river sediment for bioremediation properties, plastic-degrading microbes, and classes of ARGs (Iyer and Damania, 2020; Cabral et al., 2019; Behera et al., 2021; Das et al., 2020). However, there has been limited exploration of the metagenomics of microbial communities, their functions, and potential ARGs in the Brahmaputra River, India. Therefore, the present research aimed to investigate the bacterial communities in the Brahmaputra River and explore the relationship between bacterial composition and their functional potential across different stretches by screening functional genes associated with ARGs. The study also explored MGEs, mainly plasmid-related genes and virulence factor genes (VFGs), derived from the sediment metagenome.
Materials and methods
Study area and collection of samples
Sediment samples were collected from six locations of the Brahmaputra River, India, covering the major landing centers of its upper (Sadiya and Dibrugarh), middle (Tezpur and Morigaon), and lower (Guwahati and Dhubri) stretches between September and October 2021 (Figure 1). The sampling locations were as follows: Sadiya (Code: BRS-1; 27° 49.14” N 95° 40.52″ E), Dibrugarh (Code: BRS-2; 27° 29.9” N 94° 54.13″ E), Tezpur (Code: BRS-3; 26° 36.58” N 92° 47.27″ E), Morigaon (Code: BRS-4; 26° 17.20” N 92° 06.40″ E), Guwahati (Code: BRS-5; 26° 11.43” N 91° 45.20″ E), and Dhubri (Code: BRS-6; 26°1.20” N 89° 59.41″ E). At each sampling location, sediment samples were collected from five distinct points at a depth of 15–20 cm, resulting in a single composite sample of approximately 500 g. This approach aimed to accurately represent the microbial diversity present at each specific sampling location. The samples were transported in sterile containers within an icebox and stored at −80°C for further laboratory analysis.
Physicochemical parameters analysis
Water samples were collected from all six sampling sites of the Brahmaputra River using clean 1,000 ml polypropylene bottles for the determination of various physicochemical parameters. The river water samples were analyzed for temperature (°C), dissolved oxygen (DO), pH, total dissolved solids (TDS), salinity (%), specific conductivity (μS/cm), biological oxygen demand (BOD), and chemical oxygen demand (COD) as per the standard methods of American Public Health Association (APHA) (APHA AWWA WEF, 2012). To collect sediment samples, an Ekman dredger was used. Three samples were collected from each sampling site. To obtain uniform composite samples, the residue was mixed thoroughly. The samples were transported in plastic pouch bags to the laboratory for further examination. The sediment samples were analyzed as per APHA standard methods for texture, pH, specific conductivity, organic carbon, available phosphate, and total nitrogen (APHA AWWA WEF, 2012). For heavy metal analysis, the sediment samples were preserved with concentrated HNO3 and analyzed using inductively coupled plasma mass spectrometry (ICP-MS) (NexION 1,000, PerkinElmer, Waltham, United States).
Extraction of DNA and high-throughput sequencing
Total genomic DNA was isolated separately from six sediment samples using Power soil DNA isolation kits (Qiagen, Germany) as per the manufacturer’s protocol. The purity and integrity of isolated DNA were assessed using agarose gel electrophoresis (0.8%) and by absorbance (260/280 ratio) in a Nanodrop-2000 spectrophotometer (Thermo Scientific, Burladingen, Germany). Six DNA libraries, each representing a different sampling location, were prepared using the Truseq Nano library preparation kit (Illumina #20015964). The libraries were quantified using a Qubit fluorometer (Thermofisher, Life Technologies, CA, United States) and a DNA HS assay kit (Thermofisher #Q32851, United States), following the manufacturer’s protocol. The insert size of each library was estimated using Tapestation 4150 (Agilent, Waldbronn, Germany) (Agilent # 5067-5582) according to the manufacturer’s protocol. Subsequently, the libraries were indexed using barcodes and sequenced using the Illumina NovaSeq 6,000 platform to generate paired-end reads.
Data submission
The raw metagenomic sequence data, generated in the study was submitted to the National Center for Biotechnology Information (NCBI) Sequence Read Archive (SRA) with BioProject identification number PRJNA944918. Each sediment metagenome BRS (1–6) can be assessed through SRA accession numbers (SAMN33764823, SAMN33764824, SAMN33764825, SAMN33764826, SAMN33764827, and SAMN33849149), respectively.
Sequence analysis and taxonomical binning
The sequence reads underwent preprocessing to remove the adaptors, poor-quality bases (quality value [QV] < 20 Phred score), and short reads using the fastP tool (version 0.23.2) (Chen et al., 2018). Later, the high-quality reads were assembled using MEGAHIT version 1.2.9 (Li et al., 2015) for de novo metagenome assembly. The resulting assembled contigs were subjected to the taxonomic assignment using the Kraken2 tool (version 2.1.1) with background database prebuilt reference sequence (RefSeq) indices: PlusPF (version 2022.06.07). Kraken2 assigns the taxonomic labels to the contigs on their k-mer content, comparing them to the reference genome sequences. The Kraken-mpa-report tools (version 1.2.4) were utilized to summarize read counts across taxonomic ranks for multiple samples (Wood and Salzberg, 2014).
Functional annotation
The bioinformatics database Kyoto Encyclopedia of Genes and Genomes (KEGG) was used to annotate and interpret functional genes and metabolic pathways in metagenomic data using best hit with a known reference sequence (Kanehisa et al., 2017). With over 13,000 nodes, the KEGG classification is represented as a rooted tree—the leaves of which stand for several roots. Clusters of Orthologous Groups (COG) uses orthology to categorize proteins into functional groups, which helps with metagenomic investigations’ functional annotation of genes. The SEED database facilitates the discovery of metabolic pathways and functional potential in metagenomic datasets by offering functional annotations and subsystem classifications for microbial genomes. The analysis has been carried out using MEGAN 6 (Huson et al., 2007).
Detection of ARGs, MGEs-plasmid type, and VFGs from sediment metagenome of Brahmaputra River
The tool Abricate (version 1.0.1) (https://github.com/tseemann/abricate) was used to detect antibiotic resistance genes (ARGs), MGEs-plasmid type, and virulence factor genes (VFGs) in the metagenome by mass screening the assembled contigs against the Comprehensive Antibiotic Resistance Database (CARD) with minimum DNA percentage identity and percentage coverage of 80 and 70, respectively, for ARGs, PlasmidFinder (Carattoli et al., 2014) for MGEs-plasmid type and the virulence factor database (VFDB) for VFGs. For ARGs heatmap generation, the R ggplot2 package was used (Wickham, 2009). The input values for the heatmap were derived from antibiotic resistance genes (ARGs) identified using the ABRicate tool with the CARD. For each sample, we considered the top percentage identity value for each detected ARG. This means that the highest percentage identity match between the sample and the reference gene in the CARD database was used as the input for the heatmap. The color scale represents percentage identity values, ranging from green (lower identity) to red (higher identity), highlighting the strength of the match for each gene. This approach allows clear visualization of the strongest matches for each ARG in the different sample groups Brahmaputra River station 1 to station 6 based on their top percentage identity.
Statistical analysis
The difference in bacterial composition between samples was calculated using principal component analysis (PCA). The diversity indices (Fisher, Simpson, Chao1, and Shannon) were used to calculate the α-diversity across all the samples. The variation in species between bacterial composition was calculated using β-diversity indices. The multivariate statistical tool canonical correspondence analysis (CCA) was used to quantify the relationship between the relative abundance of microbes with water and sediment parameters. The pattern of co-occurrence among ARG subtypes was investigated using a network inference approach based on strong correlations (ρ > 0.8) and high statistical significance (p < 0.01), following the methodology outlined by Junker and Schreiber (2008).
Results
Metagenomics sequencing and assembly
The number of reads varied from 31.28 million (BRS-2; an upper stretch of the river) to 80.53 million (BRS-4; a middle stretch of the river), with an average read of 54.98 million reads per sample. The maximum number of scaffolds was found in BRS-6, and the minimum number in BRS-2. The high-quality reads (30 > Q) were assembled into contigs, and a total of 0.37 million contigs were generated, including all samples. The minimum and maximum lengths of the contig are 200 and 123,389, respectively, with a mean value of 61,397 (Table 1).
Microbial community structure, diversity, and richness
The number of taxon abundance values varied from 2,451(BRS-3) to 3,148 (BRS-1) with a mean value of 2,744. Of these, 1,841 taxon abundance values were found to be common among all the samples. A large number of unique taxon abundance values were observed in the sample BRS-1 (186 numbers), followed by BRS-6 (79 numbers), BRS-3 (37 numbers), BRS-5 (30 numbers), BRS-4 (26 numbers), and BRS-2 (22 numbers), respectively (Figure 2). Accordingly, the Shannon’s H index was relatively high in BRS-1 (upper stretch) followed by BRS-6 (lower stretch of the river). The non-parametric diversity indices, that is, Fisher’s α and Chao1, were found maximum in the origin of the upper stretch of the river (BRS-1) and lowest in the middle stretch (BRS-3) (Table 2; Figure 3). Taxonomic cluster analysis showed the similarities in microbial communities among the sampling sites. BRS-1 and BRS-6 clusters under the same clade show similar groups of microbes than the other clade formed by BRS-2, BRS-3, BRS-4, and BRS-5 (Figure 4).
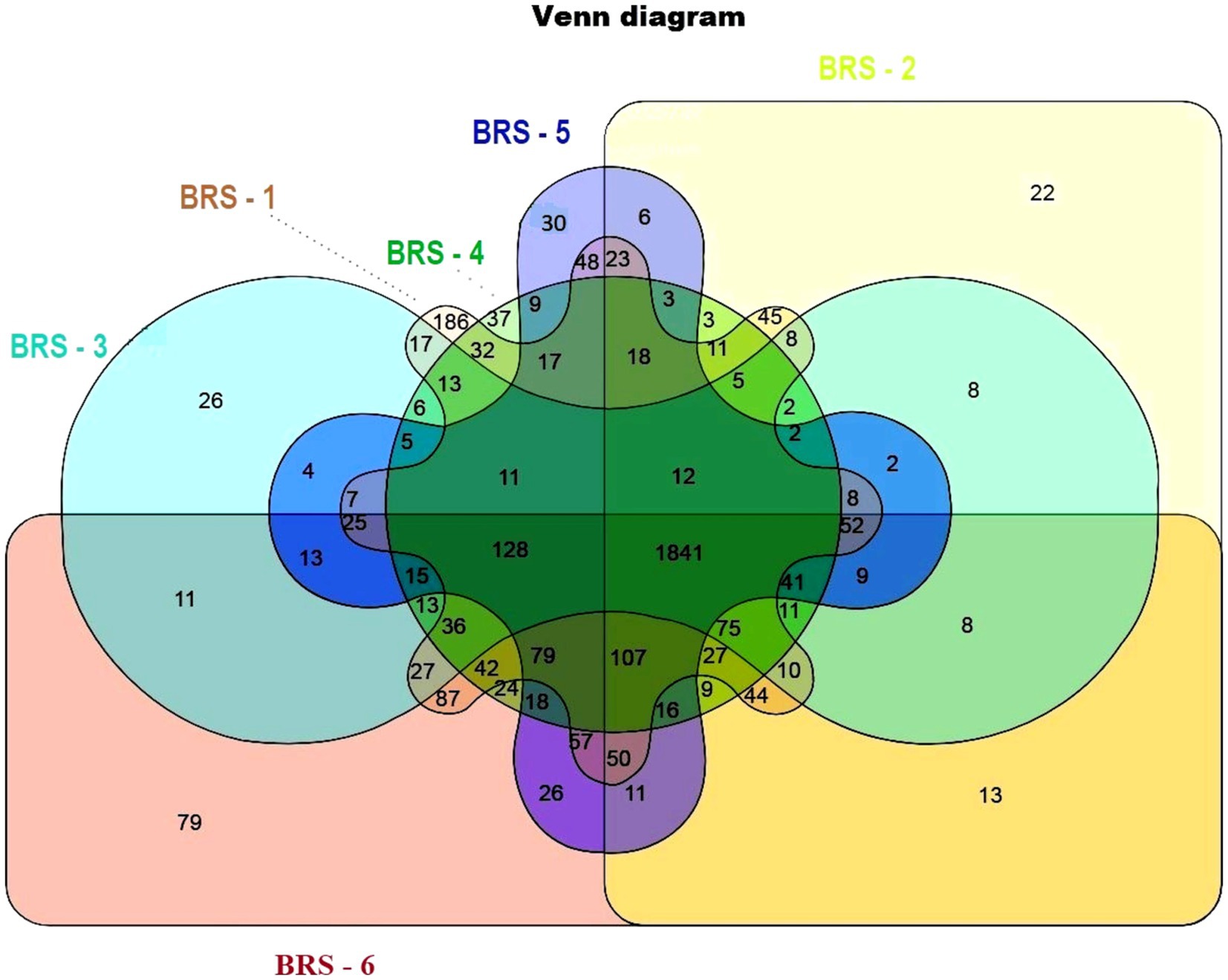
Figure 2. Venn diagram showing unique microbes in the six sampling sites. Different colors indicate different sampling sites; light brown color indicates BRS-1; light green color indicates BRS-2; light blue indicates BRS-3; dark green indicates BRS-4; dark blue indicates BRS-5; and dark red indicates BRS-6. A combination of colors indicates the union of two or more sites.
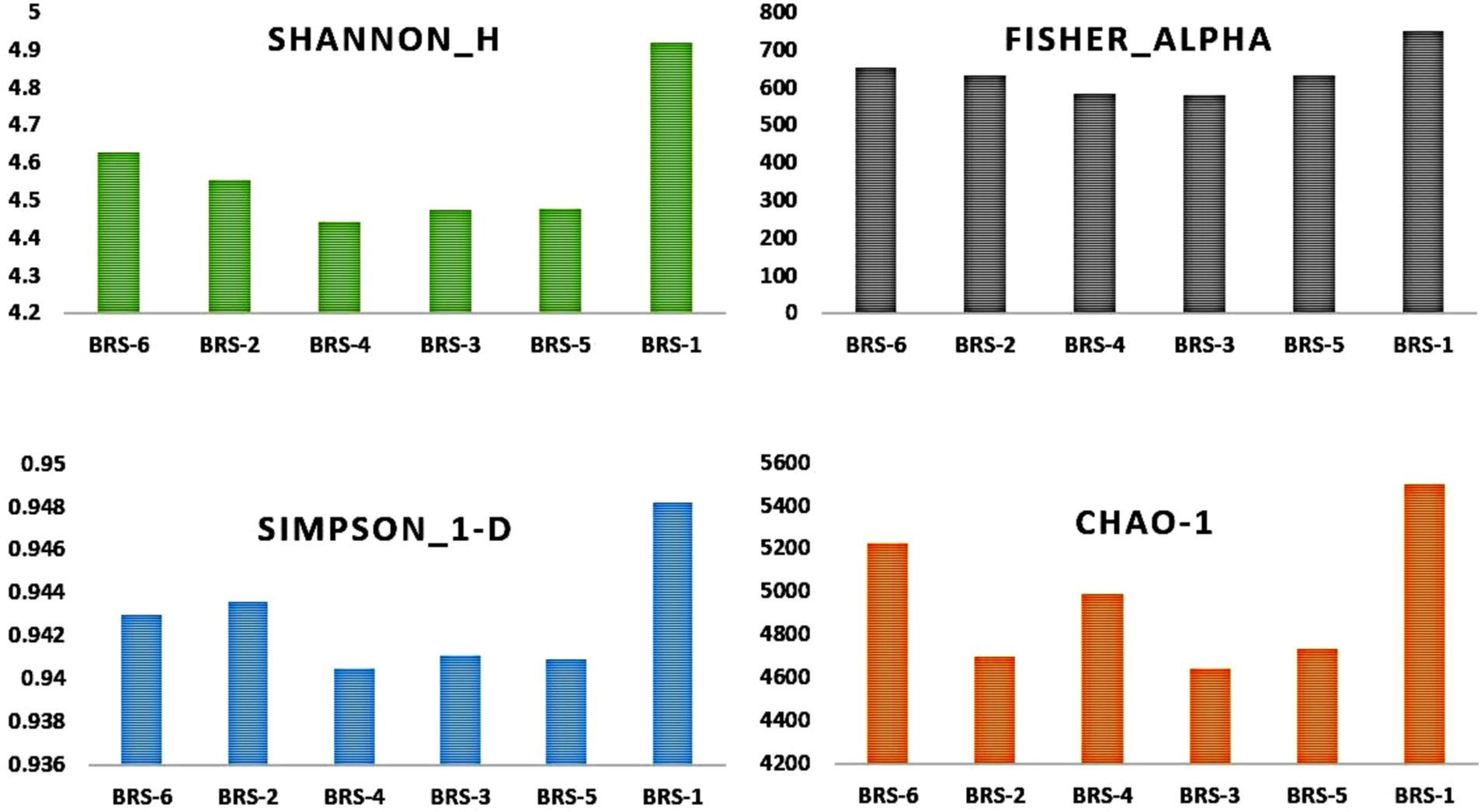
Figure 3. α-Diversity of sediment metagenome of Brahmaputra River, India. α-Diversity indices: parametric indices (Shannon and Simpson) and non-parametric indices (Fisher and Chao1) are shown in the bar diagram.
Comparison of microbial community across the samples
Among the microbial communities, the bacterial population dominates, contributing 99.22%, followed by archaea (0.40%), eukaryota (0.35%), and viruses (0.02%), respectively. A total of 31 phyla of bacteria dominated by Pseudomonadota, Actinobacteria, Bacteroidetes, Firmicutes, Cyanobacteria, and Planctomycetes were observed in all the samples. The relative abundance of the phyla varied from 62.47 to 83.48% (Pseudomonadota), 11.10 to 24.89% (Actinobacteria), 0.97 to 3.82% (Bacteroidetes), 0.54 to 3.94% (Firmicutes), 0.14 to 1.70% (Cyanobacteria), and 0.30 to 0.78% (Planctomycetes) across the samples (Figure 3). In Archaea, Euryarchaeota (from 91.68 to 97.05%) dominates, followed by Thaumarchaeota (from 0.59 to 5.91%) and Crenarchaeota (from 2.36 to 5.05%), respectively (Figure 5A). At the class level, the five bacterial classes β-proteobacteria, Gammaproteobacteria, α-proteobacteria, Actinobacteria, and Deltaproteobacteria are the most dominating categories in all the samples. In addition, in the Archaean class, Methanomicrobia and Halobacteria dominate among all the samples.
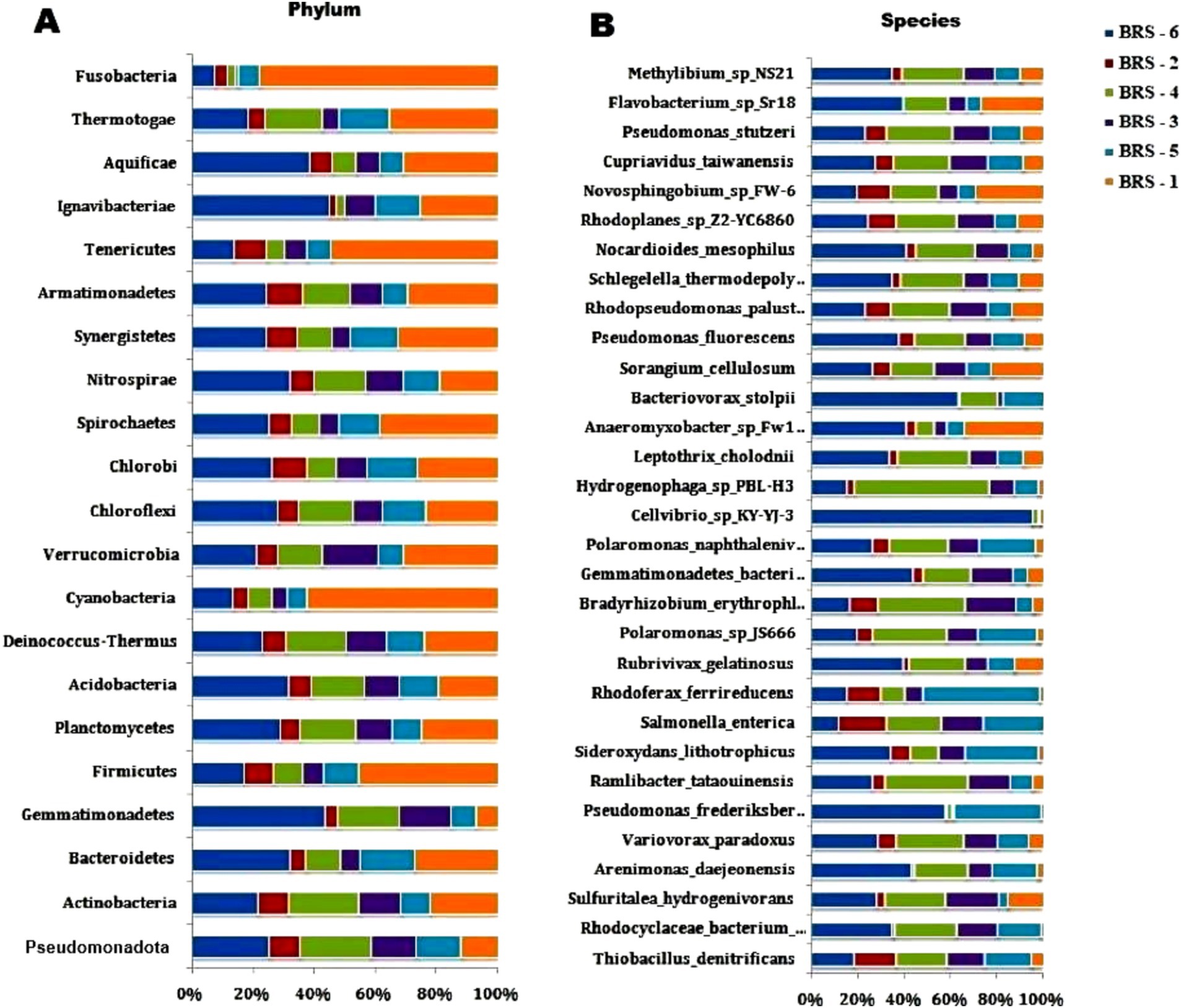
Figure 5. The figure shows two stacked bar charts depicting the relative abundances of bacterial taxa at the phylum and species levels across different sample groups (labeled BRS-1 to BRS-6). In both charts, the percentage scale along the x-axis shows the relative abundance (%) of each taxon per sample.
At the genus level, significant variations in the bacterial composition across all sampling sites are observed. Some of the prominent genera are Pseudomonas, Salmonella, Bradyrhizobium, Variovorax, Hydrogenophaga, Burkholderia, Cupriavidus, Thiobacillus, Mycobacterium, and Streptomyces in all the sampling sites. While the majority of the dominant species are unknown (uncultured), Thiobacillus denitrificans is the most abundant in all the sites, Salmonella enterica dominates in all sites except in BRS-1. Rhodocyclaceae bacterium_PG1-Ca6 dominates in all except in BRS-1 and BRS-2; Pseudomonas frederiksbergensis and Arenimonas daejeonensis dominate in BRS-6 site; Bradyrhizobium erythrophlei dominates in BRS-2, BRS-3, and BRS-4; Ramlibacter tataouinensis dominates in BRS-3, BRS-4, and BRS-6; Polaromonas_sp_JS666 dominates in BRS-3, BRS-5, and BRS-6; Variovorax paradoxus dominates in BRS-4 and BRS-6 and Novosphingobium sp_FW-6 dominate only in BRS-1. The relative abundance of the most abundant bacterial species is shown in Figure 5B.
The difference in bacterial composition between samples was calculated using PCA. The findings highlighted significant differences between river sampling sites. The proportion of the total variability among the samples accounted for PC1 and PC2 is 90.79%. Two sediment samples obtained from BRS-2 and BRS-5 sites, which are the populated sites of the River, were positively correlated based on the bacterial community to component 2. However, the samples from the other four sites, BRS-1, BRS-3, BRS-4, and BRS-6, were clustered together and negatively correlated with the component 1 (Figure 6).
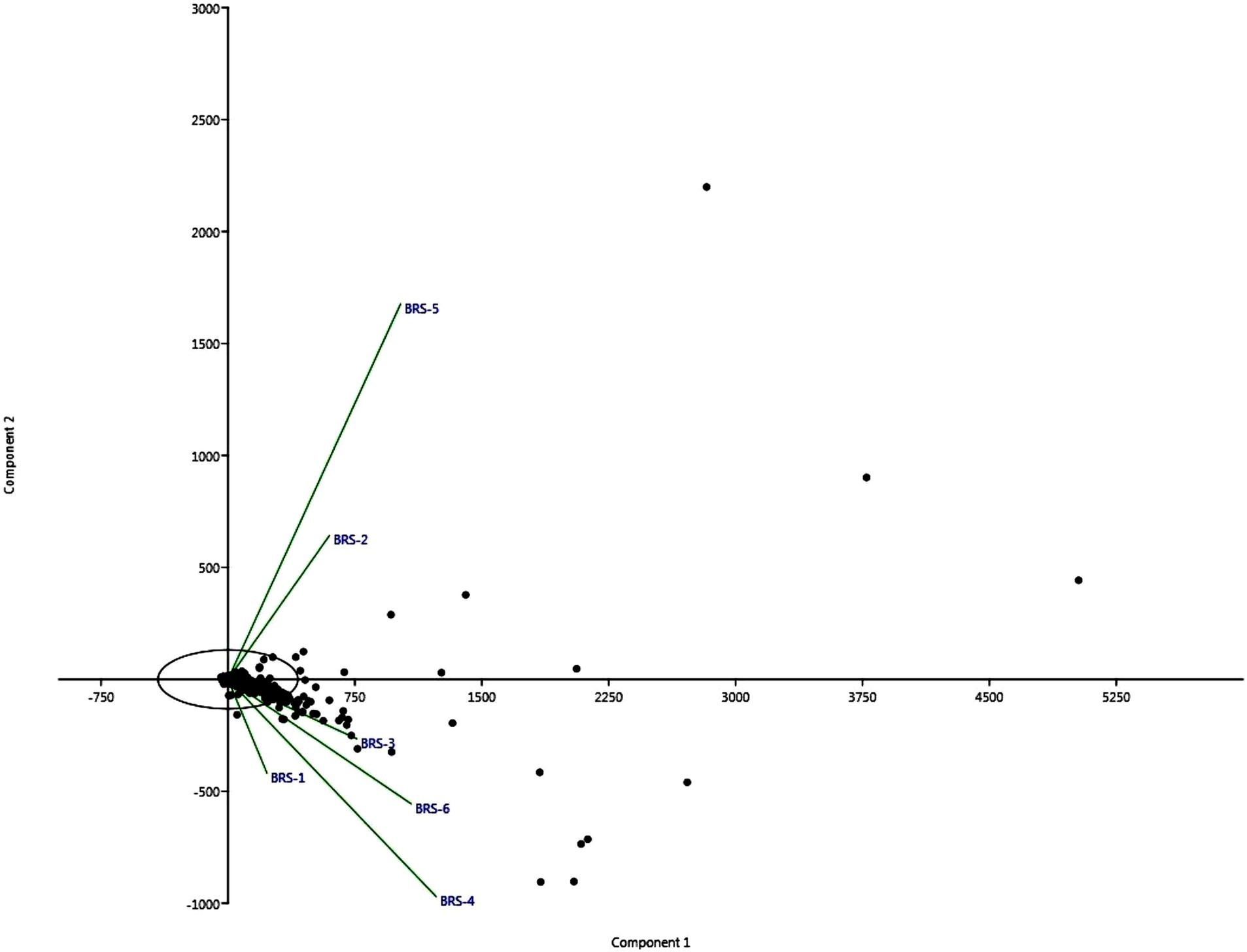
Figure 6. The figure shows two stacked bar charts depicting the relative abundances of bacterial taxa at the phylum (A) and species (B) levels across different sample groups (labeled BRS-1 to BRS-6). In both charts, the percentage scale along the x-axis shows the relative abundance (%) of each taxon per sample.
Correlation of microbial community and physicochemical variables
We used CCA to determine the relative abundance of the microbial community at the phylum level based on values of water and sediment parameters measured at the site, which allows us to identify quantifiable relationships between microbial communities and physiochemical parameters of sediment and water quality. The water and soil quality parameters were summarized in Supplementary Tables S1, S2. Physicochemical parameters were found to be acceptable for the survival and growth of aquatic organisms. At the phylum level, Verrucomicrobia, Deinococcus-Thermus, Crenarchaeota, Armatimonadetes, and Planctomycetes formed a major group and associated more with water parameters, such as dissolved oxygen, pH, water temperature, free CO2, turbidity, TDS, nitrate and nitrite and Gemmatimonadetes are shown to be associated with phosphate (Supplementary Figure S1). In sediment, the majority of the parameters, namely, available nitrogen, available phosphate, free CaCO3, heavy metals, pH, and organic carbon, are associated with microbial groups Deinococcus-Thermus, Crenarchaeota, Pseudomonadota, Nitrospirae, and Acidobacteria, whereas Verrucomicrobia is associated with Arsenic (Supplementary Figure S2).
Functional profiling of microbes
Functional annotation of sequences of all six metagenome samples through KEGG databases revealed several important functional features. A total of 407,034 (BRS-1), 291,345 (BRS-2), 414,113 (BRS-3), 673,709 (BRS-4), 410,221 (BRS-5), and 722,445 (BRS-6) numbers of genes were assigned to KEGG pathway. A total of eight major metabolic pathways were identified using KEGG analysis. Based on KEGG annotation, the relative abundance of the top six metabolic pathways were metabolism (from 44.02 to 33.85%), environmental information processing (from 2.73 to 1.62%), genetic information processing (1.30 to 0.73%), human diseases (0.59 to 0.20%), cellular processing (0.44 to 0.10%) and organismal systems (0.48 to 0.42%). The highest level of KEGG analysis showed that the major metabolic pathways involved are alcohol dehydrogenase (NADP+), malate dehydrogenase (oxaloacetate-decarboxylating) (NADP+), succinate dehydrogenase (ubiquinone) membrane anchor subunit pyrroline-5-carboxylate reductase, nitrate reductase gamma subunit, fucose-1-phosphate guanylyltransferase and cysteinyl-tRNA synthetase are highly prominent across all sampling sites highlighting their role in energy and amino acid metabolism, central carbon metabolism, stress response pathways and degradation (Figure 7). Overall, these findings provide a thorough overview of important biochemical regulatory networks that are active at the study sites, reflecting the organism’s metabolic diversity and environmental adaptability (Figure 7).
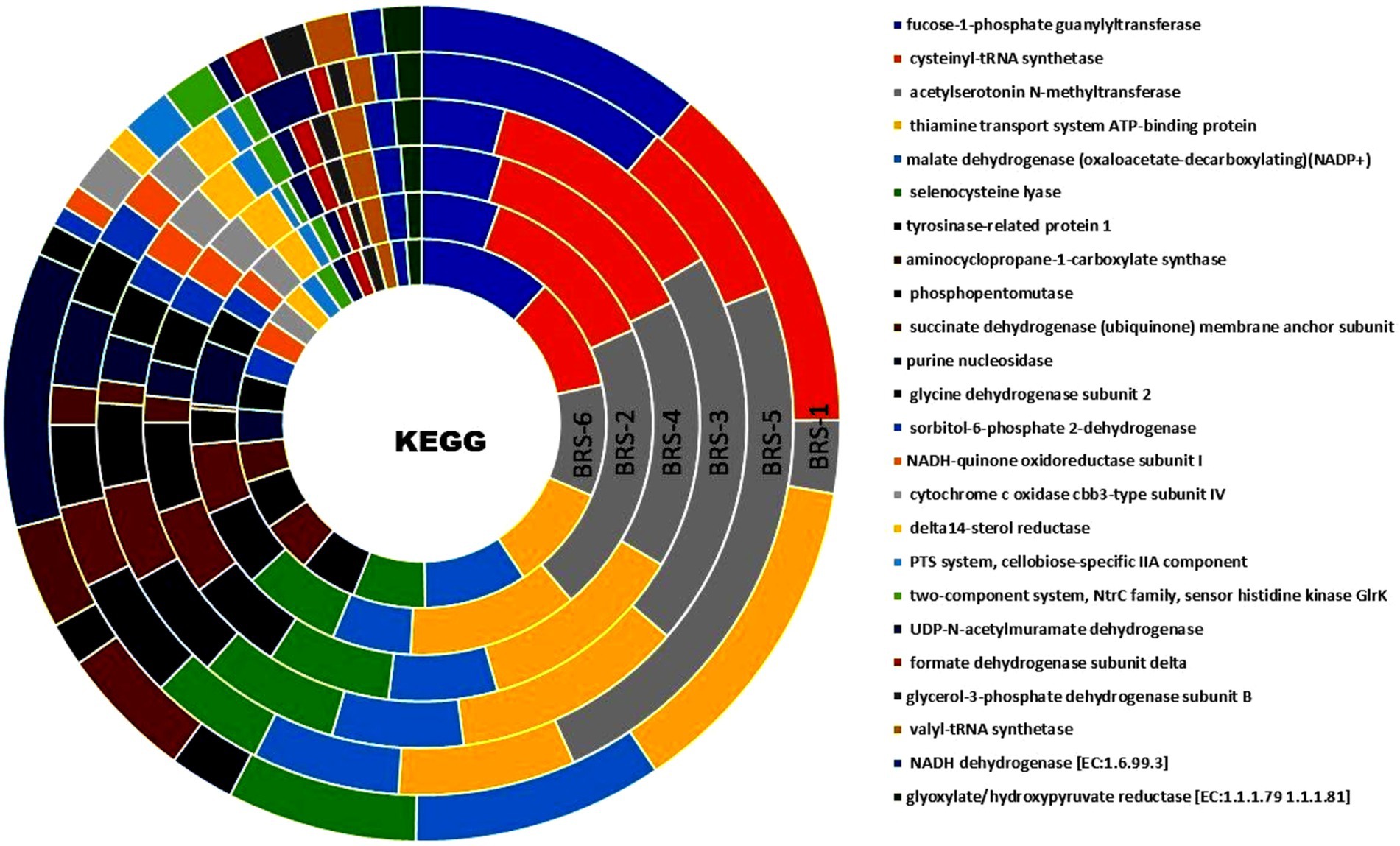
Figure 7. Functional genes from metagenome data enriched using KO terms from the stretches of the Brahmaputra River through KEGG orthologous pathways.
Functional classification through SEED revealed 89,204 (BRS-1), 57,373 (BRS-2), 82,793 (BRS-3), 134,077 (BRS-4), 81,239 (BRS-5), and 147,565 (BRS-6) genes were assigned to six sediment metagenome of Brahmaputra River. SEED classification also revealed the presence of many functional genes in metagenome data from river sediments (Figure 8A). Among them, genes associated with metabolism, carbohydrates, secondary metabolism, stress response, defense, virulence, energy, and cellular process were found to be more significant than the other functions in all the sampling sites. Some of the functions were found to be less significant in a few of the sites; for example, nitrogen metabolism is found to be less in sites BRS-5 and BRS-1, and energy function is also found to be less significant in BRS-2, BRS-5, and BRS-6. COG categories assigned 2,69,246, 1,95,054, 275,570, 434,904, 2,65,255, and 4,67,374 number of genes in the metagenome of river sediment. A total of 10,57,976 (63.83 to 53.91%) genes are related to metabolism in all the samples, while 6,90,906 (from 41.59 to 27.06%) genes are related to information storage and processing, and 1,58,521 (from 9.09 to 7.24%) are associated with cellular processing and signaling. The deeper level of COG analysis revealed that several pathways, including N-acetylglutamate semialdehyde dehydrogenase, RuvB-like proteins, cleavage and polyadenylation, and the type-I restriction-modification system, were prominently represented across all sampling sites indicating diverse functional capabilities and adaptive strategies of the microbial communities. A comprehensive overview of the other major pathways can be found in Figure 8B.
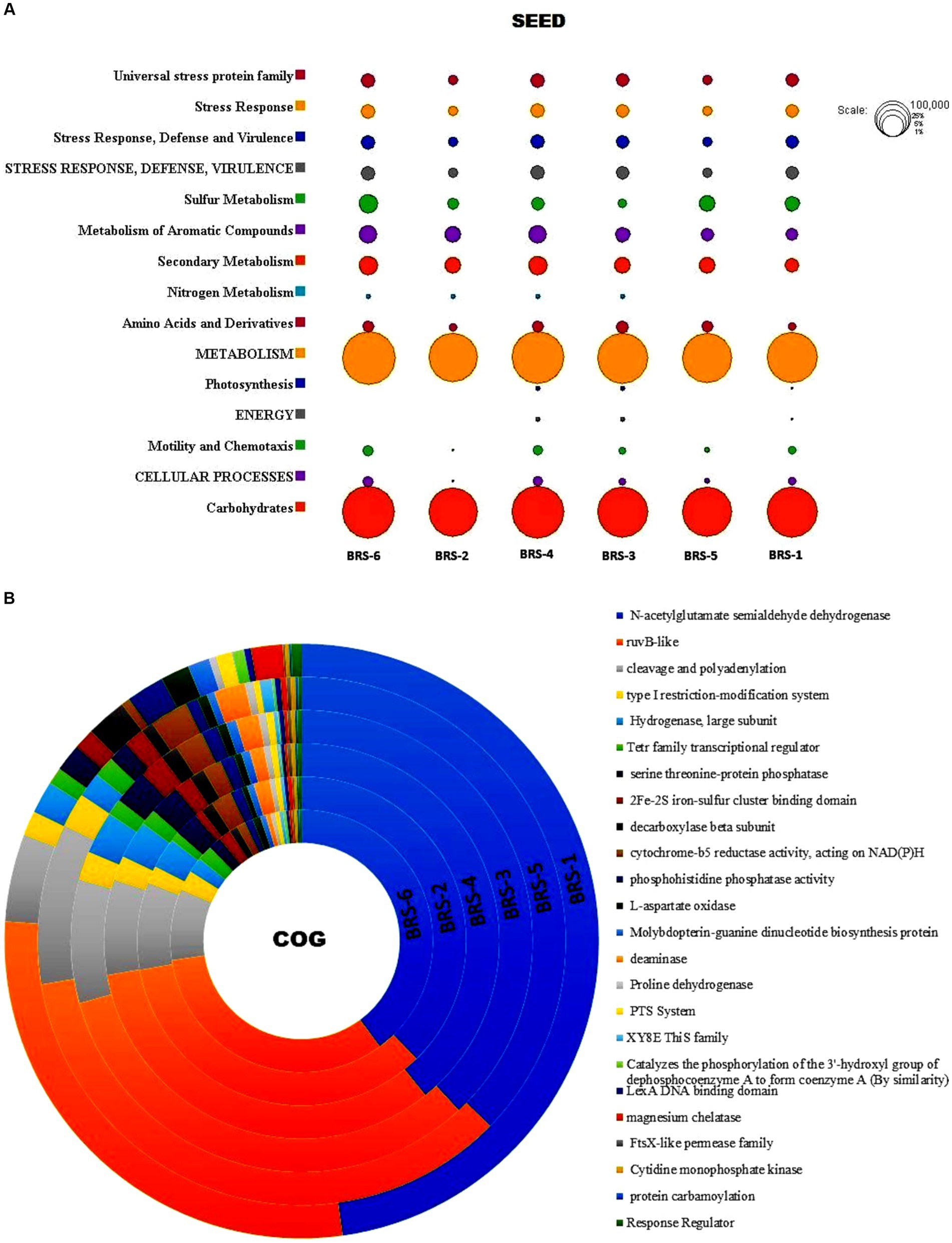
Figure 8. (A) Functional classification of microbes from Brahmaputra River through SEED tool. Circle size is based on the number of assigned values for particular functions; (B) Functional classification of microbes from Brahmaputra River through COG subsystem.
Mining of antibiotic resistance genes, plasmid-related genes, and virulence factor genes
A total of 50 diverse antibiotic resistance genes (ARGs) were identified, including all sampling locations. These genes include multidrug resistance genes (MDR), aminoglycoside resistance (AGR), β-lactam resistance (BLR), fluoroquinolone resistance (FR), phenicol resistance (PR), rifampicin resistance (RR), sulfonamide resistance (SR) and tetracycline resistance (TER) genes generally and ARGs, namely, AAC (6′)-ly, CRP, acrA, H-NS, TEM-116, emrB, emrR, golS and mdsA, mdsB. A list shows genes linked to significant antibiotic groups (Table 3). The heatmap shows the presence/absence of various antibiotic resistance genes across the six sample groups (BRS-1 to BRS-6) based on their percentage identity (Figure 9A). These sampling sites pass through the major city areas, whereas the start point (BRS-1) and endpoint (BRS-6) sampling sites contain very few ARGs. Approximately 44% of the ARGs were resistant to two or more drug classes. The gene TEM-116 was dominant in all the sites except in BRS-1. The genes acrA, AAC (6′)-ly, ampH, H-NS, emrB, golS, mdsA, and mdsB were predominant in BRS-2. The abundant ARGs at site BRS-4 were CRP, ramA, mexN, H-NS, emrR, and mdsA. The sampling site BRS-5 harbored more mdsC, golS, and H-NS. Genes such as arr-1, arr-4, and vatB were prevalent at BRS-1. The genes bacA, ramA were predominant in BRS-6 and genes sul1 and baeR were predominant in BRS-3. Supplementary Table S3 is provided regarding the information on the detection of potential ARGs (including contig name, start, end of hit, fasta sequences, similarity percentage, coverage percentage, etc.). Out of the different resistance microbes, six microbial species, namely, Escherichia coli, Enterobacter cloacae, Klebsiella pneumonia, Pseudomonas aeruginosa, Staphylococcus aureus, and S. enterica showed resistance against multiple drugs. The highest resistance microbes abundances were recorded for E. coli, followed by S. enterica > P. aeruginosa > K. pneumonia > Y. enterocolitica > S. aureus > V fluvialis > Mycolicibacterium smegmatis and E. cloacae. Using the plasmid finder part of Abricate, we have identified fewer plasmid type-MGEs. The plasmid-related genes observed were Col (BS512), Col440II, Col8282, ColRNAI, ColpVC, and IncFII, respectively (Figure 9B). The plasmid-related genes were found to be highest in BRS-2 and negligible in BRS-1 sampling sites. The virulence factor analysis showed the presence of a total of 185 virulence factor genes (VFGs) mostly present in the BRS-2 sampling site (135 numbers), followed by BRS-5 (92 numbers), BRS-4 (68 numbers), BRS-3 (56 numbers), BRS-6 (37 numbers) and BRS-1 (13 numbers), respectively (Figure 9C). A few of the VFGs present in all sampling sites were acpXl, algU, cheB, cheY, entB, flgC, flgG, fliA, pilG, and pilH, respectively. Detailed information about all the VFGs was provided in Supplementary Table S4.
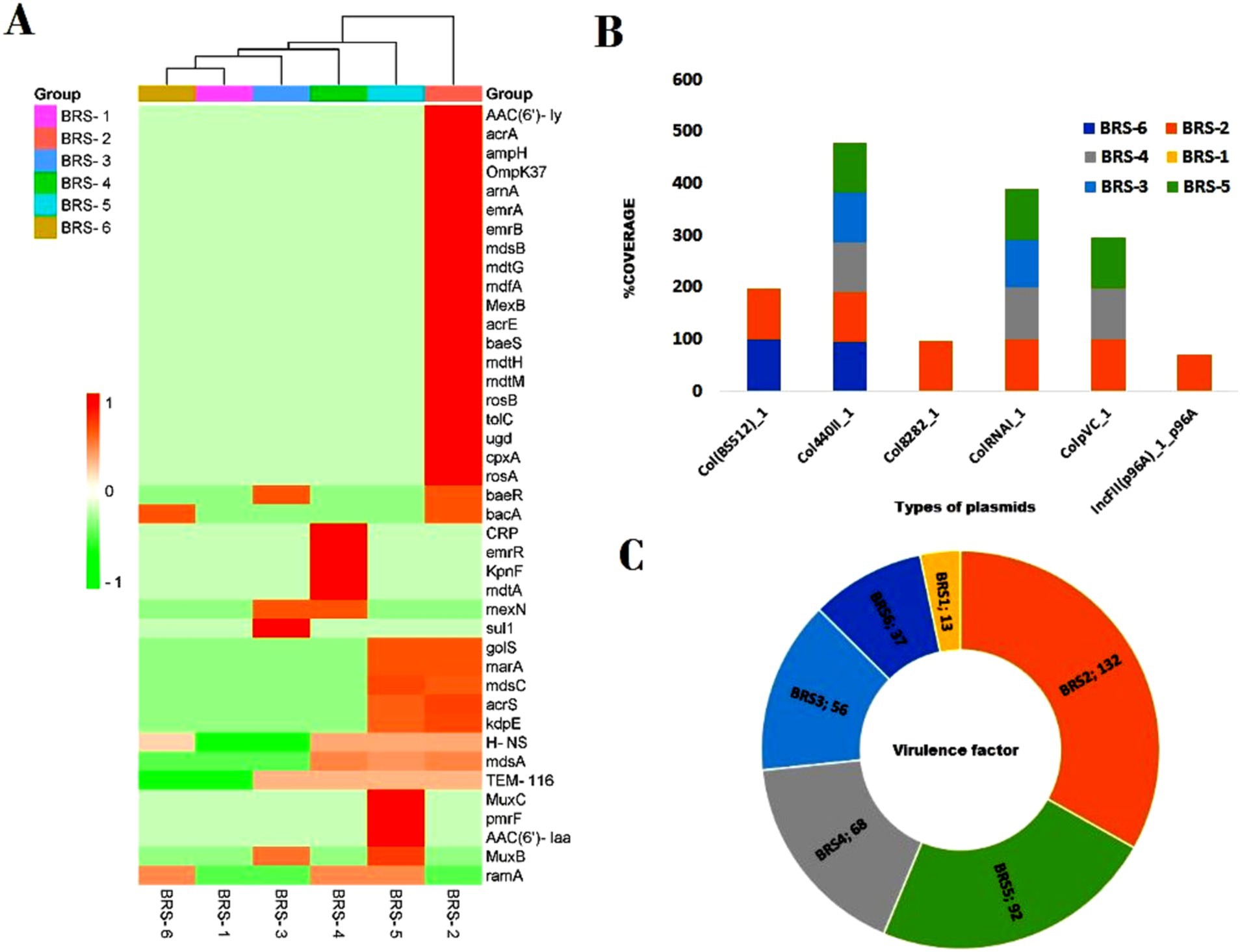
Figure 9. Abundance of ARGs, plasmid types, and virulence factors in all the six selected sites of Brahmaputra River, India. (A) The heatmap shows variability in antibiotic resistance genes (ARGs) profiles across samples, with some samples showing more pronounced resistance patterns. The color scale on the left indicates the presence of ARGs based on percentage identity, ranging from green (lower identity) to red (higher identity). The y-axis lists the resistance genes. The x-axis represents the sample groups (BRS-1 to BRS-6), with a dendrogram at the top, indicating clustering based on similarity in resistance gene profiles. The dark red coloring indicates samples showing a high abundance of specific genes are indicated by; (B) The diversity in plasmid types, with different plasmids dominating in different samples, indicating potential plasmid-mediated gene transfer; (C) The distribution of virulence factors (VFs), with some samples (BRS-2 and BRS-5) having significantly higher counts, suggesting a greater virulence potential.
Co-occurrence pattern between ARG subtypes
The pattern of co-occurrence among ARG subtypes was investigated using a network inference approach. The resulting network, depicted in Figure 10, comprises 25 nodes representing distinct ARG subtypes, connected by 66 edges indicating correlations. The average node degree, a measure of connectedness, is calculated at 5.28, suggesting that each ARG subtype is typically associated with around 5 other subtypes. Additionally, the average local clustering coefficient, indicating the prevalence of interconnected clusters, is found to be 0.653, signifying notable clustering within the network.
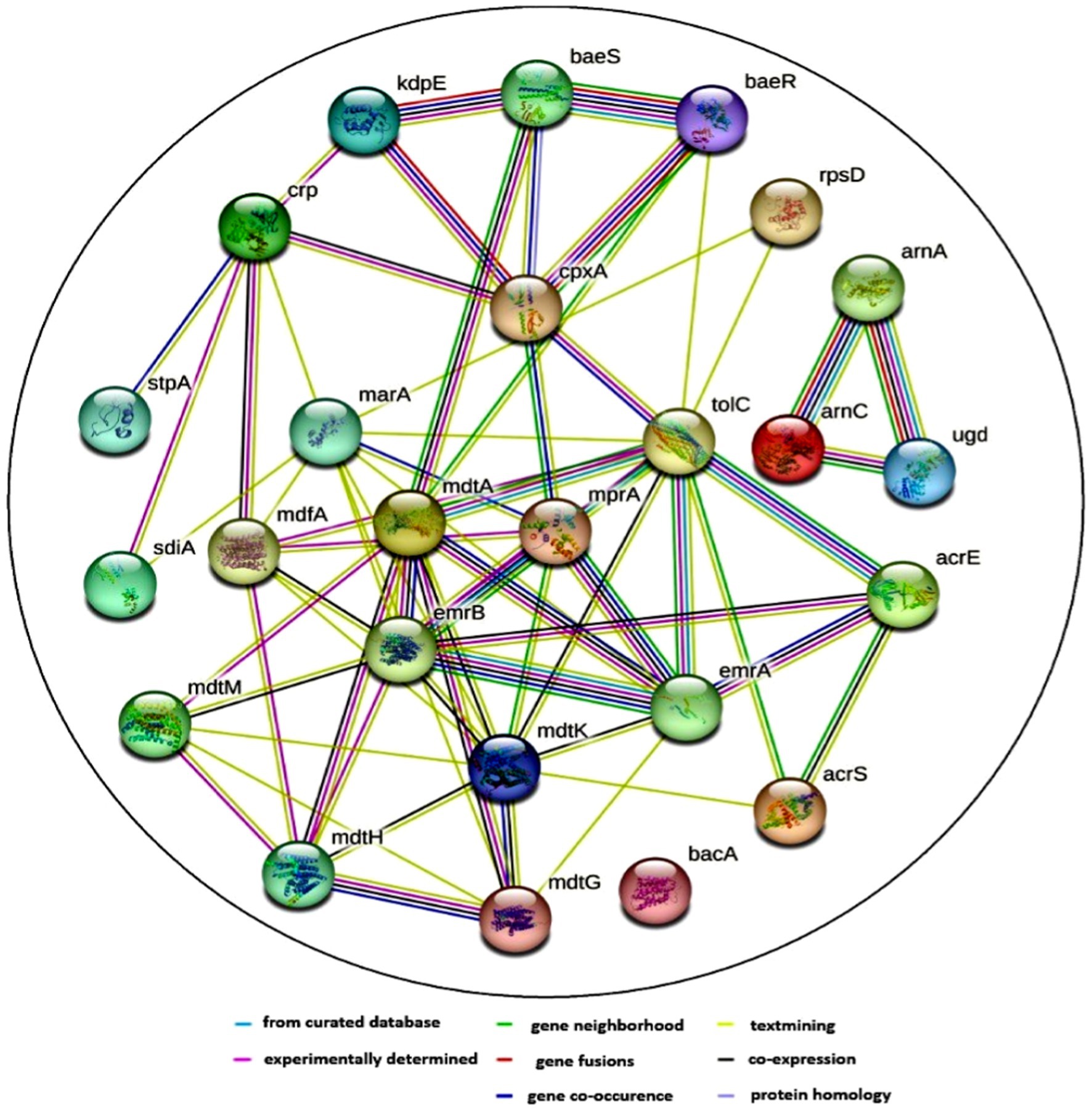
Figure 10. Protein–protein interaction (PPI) network of various antibiotic resistance genes (ARGs) and their co-occurrence patterns. The network was generated using the Search Tool for the Retrieval of Interacting Genes/Proteins (STRING) database, which predicts functional associations based on evidence from different sources. The nodes represent different microbial genes that are associated with antibiotic resistance mechanisms. The edges indicate different types of interactions. The color coding for the lines provides information about the nature of these interactions: Known interactions (blue) or experimentally determined (pink). Predicted interactions such as gene neighborhood (green), gene fusions (red), and gene co-occurrence (blue). Other interactions include text mining, co-expression, and protein homology (yellow, black, and cyan).
It was observed that ARG subtypes belonging to the same ARG type exhibit a tendency to co-occur. This phenomenon is exemplified by the correlation between emrA, emrB, mdtA, mdtG, mdtH, and mdtK, all associated with multidrug resistance. Furthermore, specific functional associations between ARGs become apparent; for instance, arnA, arnC, and ugd, conferring resistance to polymixin, are found to co-occur. Noteworthy interactions also involve baeS and baeR, which respond to envelope stress and activate various operons, including mdtABCD and potentially the CRISPR-Cas casABCDE-ygbT-ygbF operon. The ARGs’ subtypes revealed that the majority of them acted through multiple modes, such as antibiotic efflux, antibiotic inactivation, antibiotic target alteration, and replacement.
Discussion
Microbial communities play an important role in ecological processes and maintenance of the diverse environments of river ecosystems. The river system’s continuous anthropogenic activity may have an effect on the composition and dynamics of the microbiome as well as their biological roles. The present study evaluated the microbial diversity and its functional profiling in sediments of the Brahmaputra River using shotgun metagenomic sequencing. The study also identified ARGs in the river sediments. The sediments from the Brahmaputra River sampling sites revealed greater diversity and abundance of microbial communities. The Shannon diversity indices were higher than the average value of 4, showing greater diversity. Similarly, Rout et al. (2022) found a higher diversity Shannon index value of up to 4 for microbial communities in the sediment of River Ganga, and Feng et al. (2022) also observed a greater Shannon index of the Bahe River Basin, China, than the average value of 6, indicating that the microbial community diversity in the sediments of the Bahe River Basin to be high. Bacterial populations are the most dominating among microbial communities in the sediments of the Brahmaputra River, contributing about 99%. Similarly, Behera et al. (2021) observed the occurrence of bacteria to be higher than other groups of microbes in River Ganga sediment. Using paired-end Illumina HiSeq, Malakar et al. (2021) sequenced the V3–V4 region of the 16S rRNA gene amplicon, identifying 631 genera of bacteria from 22 phyla found in the gills of Brahmaputra River fish, including both pathogenic and non-harmful species. Taxonomic profiling of bacteria in the study revealed the presence of phyla Pseudomonadota, Actinobacteria, Bacteroidetes, Firmicutes, Cyanobacteria, and Planctomycetes. The microbial taxonomic profile obtained in this study was consistent with other studies of microbial distribution in freshwater rivers, where bacterial population dominates the river sediment (Wang et al., 2017; Abia et al., 2018; Behera et al., 2021; Rout et al., 2022).
The most prevalent phyla across all sampling sites were Pseudomonadota and Actinobacteria, which is consistent with findings from several previous studies on river ecosystems (Reddy et al., 2019; Behera et al., 2020a,b; Feng et al., 2022). Pseudomonadota is commonly the abundant phylum in sediments, having a wide range of functions in nitrogen, carbon, and sulfur metabolism and degradation of organic and inorganic compounds (Spain et al., 2009; Bai et al., 2012; Zainun and Simarani, 2018). Among the Pseudomonadota classes, β-proteobacteria, Gammaproteobacteria, α-proteobacteria, and Deltaproteobacteria are the most dominating categories in all the sampling sites. A higher abundance of these classes of bacteria was also reported from lakes and rivers (Medeiros et al., 2016; Tsagaraki et al., 2018; Samson et al., 2019; Parida et al., 2022). α-Proteobacteria and β-proteobacteria abundance have been related to pH and nutrients of the aquatic environment (Newton et al., 2011). The nitrogen and sulfur cycles are significantly influenced by deltaproteobacteria and gammaproteobacteria (Mori et al., 2019; Masuda et al., 2022). The high abundance of gammaproteobacteria and deltaproteobacteria may also be linked to anthropogenic activities, primarily from domestic and industrial waste (Parida et al., 2022). Freshwater actinobacteria plays an important ecological role in degrading complex polymers, producing bioactive molecules, and recycling compounds (Trujillo et al., 2015; Lewin et al., 2016; Zothanpuia et al., 2018; Mawang et al., 2021). Additionally, Bacteroidetes and Firmicutes play a significant part in organic decomposition and fermentation (Oni et al., 2015; Yi et al., 2021). In Archaean phyla, Euryarchaeota, a common group of Methanomicrobiales found in freshwater environments, are abundant and help in the anaerobic degradation of organic matter (Koizumi et al., 2003; Bomberg et al., 2008). Similar observations were reported by Parida et al., 2022 in the Yamuna River and by Rathour et al. (2020) in Pangong Lake.
The presence of genera Bradyrhizobium, Pseudomonas, and Variovorax, in the sediments of the Brahmaputra River indicates their role in denitrification and organic matter degradation (Lalucat et al., 2006; Horel et al., 2015; Liao et al., 2013). Burkholderia, Hydrogenophaga, and Cupriavidus have a role in biodegrading many kinds of environmental chemicals (Lambo and Patel, 2007; Han et al., 2015; Morya et al., 2020). At the species level, T. denitrificans was found to be abundant in all the sites, which has the capability for oxidation of sulfur, ferrous iron, and iron sulfides (Beller, 2005; Beller et al., 2006). T. denitrificans plays a key role in the nitrate-dependent oxidation of iron sulfide minerals in natural freshwater systems (Haaijer et al., 2006). The presence of Salmonella enterica, a foodborne pathogen, in all the sites except in BRS-1 (start of the river), indicates anthropogenic activities or polluted sites of the river as the river passes through the urban areas. Other abundant species, Rhodocyclaceae bacterium_PG1-Ca6 and P. frederiksbergensis degrade polycyclic aromatic hydrocarbons (Singleton et al., 2015; Ruiz et al., 2021), Polaromonas_sp_JS666 degrades cis-1,2-dichloroethene in subsurface and aerobic environments (Giddings et al., 2010).
The α-diversity assessment, such as Simpson index, Fisher’s α-index, Shannon index, and Chao1 index, did not differ significantly in all the samples, with its highest richness at the upstream and downstream side of the river, and least at midstream. The maximum number of taxa was also observed at the start of the upstream and end of downstream of the river, forming one cluster, and the river sites passing through the urban areas formed one cluster. The undisturbed water with rich nutrients flows into the river contributing to the enrichment of microbial communities. Furthermore, unique and shared microbes among the sites show that the upstream contributed higher microbial species to downstream and midstream. A similar observation was reported in the Danube River, where upstream contributes more microbial diversity due to the passive transportation of microbial communities in lotic water and large contact of small headwaters facilitates the contribution of allochthonous bacteria to the river community (Savio et al., 2015). Chen et al. (2018) observed α-diversity increased gradually from upstream to downstream than the midstream, as microbial dispersal or drift limitations are influenced mainly by spatial distance between the sites.
Exploiting the functional potential of the genes expressed by the microbes helps to gain insight into the metabolic contribution of microbes to the river ecosystem. The present study’s functional profiling through the KEGG database revealed that the majority of the microbes were involved in metabolism and environmental information processing, degradation, and disease pathways. Similar findings were reported in the Apies River, South Africa (Abia et al., 2018), where bacteria were involved in metabolic and environmental information pathways, and some were related to human diseases involving infectious diseases. According to Raiyani and Singh (2020) the metabolic capabilities of microbial communities in Arabian seawater revealed several functions such as metabolism, genetic information processing, cellular processes, and deeper analysis revealed amino acid, carbohydrate, nucleotide metabolism, etc. Srivastava and Verma (2023) also identified significant genes that play roles in metabolism, degradation, and certain human diseases in the Ganga River. Several studies on functional profiles of environmental bacteria have been linked to human diseases, as discussed by Sandifer et al. (2015). The transmission of infectious diseases and antimicrobial resistance spread across the environment via wind, agricultural and urban runoff, and biological agents such as humans and animals (Allen et al., 2010). Based on the SEED and COG analysis, the microbiomes of the Brahmaputra River exhibited considerable enrichment in major pathways, including metabolism, secondary metabolism, information storage, and processing, which can be correlated with the prevalence of Pseudomonadota, Actinobacter, and Bacteroidetes in the river and its lakes (Koo et al., 2017; Ahmad et al., 2021; Parida et al., 2022).
The development of antibiotic resistance bacteria in aquatic ecosystems is caused by the direct discharge of hospital and domestic sewage, agricultural runoffs, and wastewater into open water bodies without adequate pre-treatment (Chandy, 2008; Prado et al., 2018). The development of multidrug-resistant strains, which enable the bacteria to adapt and survive, is a major health concern (Marathe et al., 2013). In the present study, through heatmap analysis, 50 numbers of ARGs’ subtypes were observed, and the majority of microbial groups are associated with multidrug resistance, aminoglycoside resistance, β-lactam resistance, fluoroquinolone resistance, phenicol resistance, sulfonamide resistance, rifampicin resistance, and tetracycline resistance. Similar observations were also reported by Das et al. (2020) where a high amount of ARGs were obtained from the Yamuna River, India. ARGs families in sediments of an urban river (Chen et al., 2019) and the European river (Kneis et al., 2022) reported resistance to aminoglycosides, macrolides, sulfonamide, or tetracyclines, possibly indicating the spread of ARGs in river environment because of selective pressure resulting from antibiotic use. In this study, six groups of plasmid-type MGEs were identified in the river sediment metagenome. Nguyen et al. (2022) also observed ARGs and MGEs in both Day River water samples and shrimp ponds in Vietnam, where 11 ARGs have a significant correlation with both total MGEs and individual MGE types. The Col plasmid groups are often found to be associated with E. coli and K. pneumonia, having been found to disseminate AMR genes, which confers resistance to quinolones (Rozwandowicz et al., 2018; Khezri et al., 2021). The IncF plasmid types have been reported to harbor a wide range of resistance genes to aminoglycosides, tetracyclines, β-lactams, quinolones, and macrolides, reported from various systems such as riverine systems, wastewater treatment plants, drinking water sources. The IncF plasmids often carry multiple replicons and harbor-reliant systems, which enable them to remain stable in bacterial host cells even under changing environmental conditions (Yang et al., 2015; Kesamang and Rahube, 2019; Ajayi et al., 2021). Additionally, the present study also identified 185 virulence factor genes (VFGs) from the river sediment metagenome, mostly from the BRS-2 sampling site. The metagenomic analysis uncovered ARGs along VFGs from different environments, such as river sediment, soil, animal fecal, etc. (Kim et al., 2022; Zou et al., 2023; Rout et al., 2024; Bai et al., 2024). Similar to the present study, Rout et al. (2024) investigated the prevalence of ARGs and VFGs within the sediment environment of the river Ganga, where a high diversity of VFGs includes mshM associated with adherence mechanisms; pilH, pilT, pilU, and pilZ, involved in twitching motility; such as cheY, fliJ, fliQ, and PA1464 genes were associated with chemotaxis and flagellar biosynthesis and acpXL, related to immune modulation. Bai et al. (2024) observed a total of 731 ARGs and 400 VFs common among humans, chickens, pigs, and soil environments, where a greater number of specific ARGs and VFGs were present in soil and chicken samples. The VFGs and plasmid-related genes were found to be highest in BRS-2 in sampling sites, which may be responsible for the observed higher ARG abundance (Jiang et al., 2022). Environmental variables, along with ARGs host influence the abundance of ARGs and VFGs by directly regulating MGEs and microbial community structure (Zou et al., 2023; Liu et al., 2024). The sites having high ARGs, MGEs-plasmid type, and VFGs are the sites along the Brahmaputra River passing through the major city areas, where human activities are involved, such as domestic waste, municipal waste, nearby hospital waste, transportation, etc.
In the network analysis, the co-occurrence pattern of ARGs was explored to illustrate the intricate relationships between various ARG subtypes. ARG subtypes assigned to the same ARG category (i.e., intratypes) usually co-occur. Li et al. (2015) noted the co-occurrence of environmental ARGs’ subtypes consisting of 46 nodes and 98 edges and highlighted the high prevalence of tetM and aminoglycoside resistance protein in different environments facilitating their applicability as ARG indicators. Gu et al. (2022) found a strong positive correlation among ARG subtypes, bacterial taxa, and mobile genetic elements, revealing a high ARG dissemination risk in drinking water treatment systems. Sang et al. (2023) described that the environmental factors greatly influenced the distribution of ARGs in the mangrove ecosystem, and the high abundance of ARGs was distributed in a modular manner. The presence of multidrug resistance genes in abundance and 100% occupancy of aminoglycoside-ARGs and β-lactam-ARGs in some of the sampling sites provides an overview of the role of human activities in accelerating spreading and proliferation of ARGs/VFGs in the urban river environment. It draws attention to the control of antibiotic use and emissions to protect public health. The presence of co-occurring ARGs suggests that these genes might be part of the same or closely related pathways, or they may be co-selected under antibiotic pressure. The co-occurrence of ARGs in environmental samples suggests that bacteria in these environments may be exposed to multiple selective pressures (e.g., antibiotic contamination, heavy metals, etc.). Co-occurrence patterns can enhance the horizontal gene transfer (HGT) potential among bacteria, increasing the spread of antibiotic resistance in the environment. These patterns are also of public health concern, as the spread of multidrug resistance could complicate the treatment of bacterial infections. This highlights the importance of monitoring and managing antibiotic usage and contamination in river environmental settings to mitigate the risk of resistance gene dissemination.
Conclusion
By employing a metagenomic approach, the present study provides insights into microbiome community structure, its function, and the abundance of antimicrobial resistance genes in the sediments of selected stretches of the Brahmaputra River, India. The microbial diversity reveals a high abundance of Pseudomonadota at the phylum level, with Bradyrhizobium, Pseudomonas, and Variovorax dominating at the genera level, while T. denitrificans emerges as the most abundant bacterial species across all sampling sites highlighting its crucial role in organic matter degradation and denitrification processes. The highest bacterial diversity and abundance were recorded upstream of the river, particularly at the initial point, in comparison to middle and downstream areas. Functional analysis revealed that the identified microbes exhibited diverse functional capabilities and adaptive strategies, with a significant portion involved in energy and amino acid metabolism, central carbon metabolism, stress response pathways, and degradation pathways. The presence of ARGs in the river indicated multiple drug-resistance genes linked to anthropogenic activities. The growing number of reports concerning antimicrobial resistance is alarming and posing challenges to global health initiatives. Since rivers such as the Brahmaputra are principal water sources for household, drinking, and personal hygiene, individuals using this water without prior treatment may experience serious health risks. To our knowledge, this is the first study to report the whole genome metagenomic sequencing of sediments in the Himalayan Brahmaputra River. Thus, it is essential to properly regulate the discharge of untreated sewage into the river. Consequently, the study emphasizes the need for further research on the important microbes and ARGs to better understand their interaction with the aquatic ecosystem and promote sustainable river health management.
Data availability statement
The datasets presented in this study can be found in online repositories. The names of the repository/repositories and accession number(s) can be found in the article/Supplementary material.
Author contributions
NS: Writing – original draft, Writing – review & editing. BD: Conceptualization, Supervision, Validation, Visualization, Writing – original draft, Writing – review & editing. BirB: Methodology, Supervision, Writing – review & editing. AC: Supervision, Validation, Visualization, Writing – review & editing. BijB: Methodology, Supervision, Validation, Writing – review & editing. AK: Supervision, Validation, Visualization, Writing – review & editing. HC: Formal analysis, Validation, Visualization, Writing – review & editing.
Funding
The author(s) declare that financial support was received for the research, authorship, and/or publication of this article. This research was funded by the Indian Council of Agricultural Research, New Delhi, and the ICAR-Central Inland Fisheries Research Institute, Barrackpore, India.
Acknowledgments
The authors would like to acknowledge DG, the Indian Council of Agricultural Research, New Delhi, and the ICAR-Central Inland Fisheries Research Institute, Barrackpore, for supporting the research. The study was conducted as part of the PhD research work of one of the authors.
Conflict of interest
The authors declare that the research was conducted in the absence of any commercial or financial relationships that could be construed as a potential conflict of interest.
Publisher’s note
All claims expressed in this article are solely those of the authors and do not necessarily represent those of their affiliated organizations, or those of the publisher, the editors and the reviewers. Any product that may be evaluated in this article, or claim that may be made by its manufacturer, is not guaranteed or endorsed by the publisher.
Supplementary material
The Supplementary material for this article can be found online at: https://www.frontiersin.org/articles/10.3389/fmicb.2024.1426463/full#supplementary-material
References
Abia, A. L. K., Alisoltani, A., Keshri, J., and Ubomba-Jaswa, E. (2018). Metagenomic analysis of the bacterial communities and their functional profiles in water and sediments of the Apies River, South Africa, as a function of land use. Sci. Total Environ. 616–617, 326–334. doi: 10.1016/j.scitotenv.2017.10.322
Ahmad, T., Gupta, G., Sharma, A., Kaur, B., El-Sheikh, M. A., and Alyemeni, M. N. (2021). Metagenomic analysis exploring taxonomic and functional diversity of bacterial communities of a Himalayan urban fresh water lake. PLoS One 16:e0248116. doi: 10.1371/journal.pone.0248116
Ajayi, A. O., Perry, B. J., Yost, C. K., Jamieson, R. C., Hansen, L. T., and Rahube, T. O. (2021). Comparative genomic analyses of β-lactamase (blaCMY-42)-encoding plasmids isolated from wastewater treatment plants in Canada. Can. J. Microbiol. 67, 737–748. doi: 10.1139/cjm-2021-0012
Allen, H. K., Donato, J., Wang, H. H., Cloud-Hansen, K. A., Davies, J., and Handelsman, J. (2010). Call of the wild: antibiotic resistance genes in natural environments. Nat. Rev. Microbiol. 8, 251–259. doi: 10.1038/nrmicro2312
APHA AWWA WEF , (2012). Standard methods for the examination of water and wastewater, 22nd Edn. American Journal of Public health and the Nations Health, 51, 940.
Bai, Y., Shi, Q., Wen, D., Li, Z., Jefferson, W. A., Feng, C., et al. (2012). Bacterial communities in the sediments of Dianchi Lake, a partitioned eutrophic waterbody in China. PLoS One 7:e37796. doi: 10.1371/journal.pone.0037796
Bai, H., He, L.-Y., Gao, F.-Z., Yao, K.-S., Zhang, M., Qiao, L.-K., et al. (2024). Airborne antibiotic resistome and microbiome in pharmaceutical factories. Environ. Int. 186: 108639. doi: 10.1016/j.envint.2024.108639
Barbulescu, A., Barbes, L., and Dumitriu, C. S. (2021). Assessing the water pollution of the Brahmaputra River using water quality indexes. Toxics 9:297. doi: 10.3390/toxics9110297
Bashiardes, S., Zilberman-Schapira, G., and Elinav, E. (2016). Use of Metatranscriptomics in microbiome research. Bioinform. Biol. Insights 10, 19–25. doi: 10.4137/BBI.S34610
Beattie, R. E., Bandla, A., Swarup, S., and Hristova, K. R. (2020). Freshwater sediment microbial communities are not resilient to disturbance from agricultural land runoff. Front. Microbiol. 11:539921. doi: 10.3389/fmicb.2020.539921
Behera, B. K., Chakraborty, H. J., Patra, B., Rout, K. A., Dehury, B., Das, B. K., et al. (2020a). Metagenomic analysis reveals bacterial and fungal diversity and their bioremediation potential from sediments of river ganga and Yamuna in India. Front. Microbiol. 11:556136. doi: 10.3389/fmicb.2020.556136
Behera, B. K., Patra, B., Chakraborty, H. J., Sahu, P., Rout, A. K., Sarkar, D. J., et al. (2020b). Metagenome analysis from the sediment of river ganga and Yamuna: in search of beneficial microbiome. PLoS One 15:e0239594. doi: 10.1371/journal.pone.0239594
Behera, B. K., Sahu, P., Rout, A. K., Parida, P. K., Sarkar, D. J., Kaushik, N. K., et al. (2021). Exploring microbiome from sediments of river ganga using a metagenomic approach. Aquat. Ecosyst. Health Manag. 24, 12–22. doi: 10.14321/aehm.024.04.04
Beller, H. R. (2005). Anaerobic, nitrate-dependent oxidation of U(IV) oxide minerals by the chemolithoautotrophic bacterium Thiobacillus denitrificans. Appl. Environ. Microbiol. 71, 2170–2174. doi: 10.1128/AEM.71.4.2170-2174.2005
Beller, H. R., Chain, P. S. G., Letain, T. E., Chakicherla, A., Larimer, F. W., Richardson, P. M., et al. (2006). The genome sequence of the obligately chemolithoautotrophic, facultatively anaerobic bacterium Thiobacillus denitrificans. J. Bacteriol. 188, 1473–1488. doi: 10.1128/JB.188.4.1473-1488.2006
Bhattacharjya, B. K., Bhaumik, U., and Sharma, A. P. (2017). Fish habitat and fisheries of Brahmaputra River in Assam, India. Aquat. Ecosyst. Health Manag. 20, 102–115. doi: 10.1080/14634988.2017.1297171
Bomberg, M., Montonen, L., Münster, U., and Jurgens, G. (2008). Diversity and function of archaea in freshwater habitats. Curr. Trends Microbiol. 4, 61–89.
Bora, M., and Goswami, D. C. (2017). Water quality assessment in terms of water quality index (WQI): case study of the Kolong River, Assam, India. Appl Water Sci 7, 3125–3135. doi: 10.1007/s13201-016-0451-y
Cabral, L., Noronha, M. F., de Sousa, S. T. P., Lacerda-Júnior, G. V., Richter, L., Fostier, A. H., et al. (2019). The metagenomic landscape of xenobiotics biodegradation in mangrove sediments. Ecotoxicol. Environ. Saf. 179, 232–240. doi: 10.1016/j.ecoenv.2019.04.044
Carattoli, A., Zankari, E., García-Fernández, A., Voldby, L. M., Lund, O., Villa, L., et al. (2014). In silico detection and typing of plasmids using plasmid finder and plasmid multilocus sequence typing. Antimicrob Agents Chemother., 58, 3895–903. doi: 10.1128/AAC.02412-14
Chandy, S. J. (2008). Consequences of irrational use of antibiotics. Indian J Med Ethics 5, 174–175. doi: 10.20529/IJME.2008.064
Chen, H., Bai, X., Jing, L., Chen, R., and Teng, Y. (2019). Characterization of antibiotic resistance genes in the sediments of an urban river revealed by comparative metagenomics analysis. Sci. Total Environ. 653, 1513–1521. doi: 10.1016/j.scitotenv.2018.11.052
Chen, H., Liu, C., Teng, Y., Zhang, Z., Chen, Y., and Yang, Y. (2021). Environmental risk characterization and ecological process determination of bacterial antibiotic resistome in lake sediments. Environ. Int. 147:106345. doi: 10.1016/j.envint.2020.106345
Chen, J., Wang, P., Wang, C., Wang, X., Miao, L., Liu, S., et al. (2018). Bacterial communities in riparian sediments: a large-scale longitudinal distribution pattern and response to dam construction. Front. Microbiol. 9:999. doi: 10.3389/fmicb.2018.00999
Chen, S., Zhou, Y., Chen, Y., and Gu, J. (2018). fastp: an ultra-fast all-in-one FASTQ preprocessor, Bioinform. 34, i884–i890. doi: 10.1093/bioinformatics/bty560
Das, B. K., Behera, B. K., Chakraborty, H. J., Paria, P., Gangopadhyay, A., Rout, A. K., et al. (2020). Metagenomic study focusing on antibiotic resistance genes from the sediments of river Yamuna. Gene 758:144951. doi: 10.1016/j.gene.2020.144951
Datta, B., and Singh, V. P. (2004). Hydrology, in the Brahmaputra Basin water resources, edited by Singh et al. Boston: Kluwer Academic Publishers, 139–195.
Devarajan, N., Laffite, A., Graham, N. D., Meijer, M., Prabakar, K., Mubedi, J. I., et al. (2015). Accumulation of clinically relevant antibiotic-resistance genes, bacterial load, and metals in freshwater lake sediments in Central Europe. Environ. Sci. Technol. 49, 6528–6537. doi: 10.1021/acs.est.5b01031
Feng, W., Gao, J., Wei, Y., Liu, D., Yang, F., Zhang, Q., et al. (2022). Pattern changes of microbial communities in urban river affected by anthropogenic activities and their environmental driving mechanisms. Environ. Sci. Eur. 34:93. doi: 10.1186/s12302-022-00669-1
Findlay, S. (2010). Stream microbial ecology. J. North Am. Benthol. Soc. 29, 170–181. doi: 10.1899/09-023.1
Galand, P. E., Lucas, S., Fagervold, S. K., Peru, E., Pruski, A. M., Vétion, G., et al. (2016). Disturbance increases microbial community diversity and production in marine sediments. Front. Microbiol. 7:1950. doi: 10.3389/fmicb.2016.01950
Giddings, C. G. S., Liu, F., and Gossett, J. M. (2010). Microcosm assessment of Polaromonas sp. JS666 as a bioaugmentation agent for degradation of cis-1,2-dichloroethene in aerobic, subsurface environments. Ground Water Monit. Remediat. 30, 106–113. doi: 10.1111/j.1745-6592.2010.01283.x
Gu, Q., Sun, M., Lin, T., Zhang, Y., Wei, X., Wu, S., et al. (2022). Characteristics of antibiotic resistance genes and antibiotic-resistant bacteria in full-scale drinking water treatment system using metagenomics and culturing. Front. Microbiol. 12:798442. doi: 10.3389/fmicb.2021.798442
Haaijer, S. C. M., Van der Welle, M. E. W., Schmid, M. C., Lamers, L. P. M., Jetten, M. S. M., and Op den Camp, H. J. M. (2006). Evidence for the involvement of betaproteobacteria Thiobacilli in the nitrate-dependent oxidation of iron sulfide minerals. FEMS Microbiol. Ecol. 58, 439–448. doi: 10.1111/j.1574-6941.2006.00178.x
Han, L., Zhao, D., and Li, C. (2015). Isolation and 2, 4-D-degrading characteristics of Cupriavidus campinensis BJ71. Braz. J. Microbiol. 46, 433–441. doi: 10.1590/S1517-838246220140211
Horel, A., Mortazavi, B., and Sobecky, P. A. (2015). Input of organic matter enhances degradation of weathered diesel fuel in sub-tropical sediments. Sci. Total Environ. 533, 82–90. doi: 10.1016/j.scitotenv.2015.06.102
Huson, D. H., Auch, A. F., Qi, J., and Schuster, S. C. (2007). MEGAN analysis of metagenomic data. Genome Res. 17, 377–386. doi: 10.1101/gr.5969107
Iyer, R., and Damania, A. (2020). Shotgun metagenomics of indigenous bacteria collected from the banks of the San Jacinto River for biodegradation of aromatic waste. FEMS Microbiol. Lett. 367:113. doi: 10.1093/femsle/fnaa133
Jiang, C., Chen, H., Grossart, H. P., Zhang, Q., Stoks, R., Zhao, Y., et al. (2022). Frequency of occurrence and habitat selection shape the spatial variation in the antibiotic resistome in riverine ecosystems in eastern China. Environ. Microbiome 17:53. doi: 10.1186/s40793-022-00447-9
Junker, B. H., and Schreiber, F. (2008). Correlation networks. In. Analysis of biological networks. Wiley-Interscience.
Kanehisa, M., Furumichi, M., Tanabe, M., Sato, Y., and Morishima, K. (2017). KEGG: new perspectives on genomes, pathways, diseases and drugs. Nucleic Acids Res. 45, D353–D361. doi: 10.1093/nar/gkw1092
Kesamang, M., and Rahube, T. O. (2019). In silico detection and correlative analysis of antibiotic resistance plasmid-incompatibility (Inc/rep) groups from different environments. ISABB J. Biotechnol. Bioinform. 4, 1–13. doi: 10.5897/isabb-jbb2019.0017
Khezri, A., Avershina, E., and Ahmad, R. (2021). Plasmid identification and plasmid-mediated antimicrobial gene detection in Norwegian isolates. Microorganisms 9:52. doi: 10.3390/microorganisms9010052
Kim, H., Kim, M., Kim, S., Lee, Y. M., and Shin, S. C. (2022). Characterization of antimicrobial resistance genes and virulence factor genes in an Arctic permafrost region revealed by metagenomics. Environ. Pollut. 294:118634. doi: 10.1016/j.envpol.2021.118634
Kneis, D., Berendonk, T. U., Forslund, S. K., and Hess, S. (2022). Antibiotic resistance genes in river biofilms: a metagenomic approach toward the identification of sources and candidate hosts. Environ. Sci. Technol. 56, 14913–14922. doi: 10.1021/acs.est.2c00370
Koizumi, Y., Takii, S., Nishino, M., and Nakajima, T. (2003). Vertical distributions of sulfate-reducing bacteria and methane-producing archaea quantified by oligonucleotide probe hybridization in the profundal sediment of a mesotrophic lake. FEMS Microbiol. Ecol. 44, 101–108. doi: 10.1016/S0168-6496(02)00463-4
Koo, H., Mojib, N., Hakim, J. A., Hawes, I., Tanabe, Y., Andersen, D. T., et al. (2017). Microbial communities and their predicted metabolic functions in growth laminae of a unique large conical mat from Lake Untersee, East Antarctica. Front. Microbiol. 8:1347. doi: 10.3389/fmicb.2017.01347
Lalucat, J., Bennasar, A., Bosch, R., García-Valdés, E., and Palleroni, N. J. (2006). Biology of Pseudomonas stutzeri. Microbiol. Mol. Biol. Rev. 70, 510–547. doi: 10.1128/MMBR.00047-05
Lambo, A. J., and Patel, T. R. (2007). Biodegradation of polychlorinated biphenyls in Aroclor 1232 and production of metabolites from 2,4,4′-trichlorobiphenyl at low temperature by psychrotolerant Hydrogenophaga sp strain IA3-a. J. Appl. Microbiol. 102, 1318–1329. doi: 10.1111/j.1365-2672.2006.03268.x
Lewin, G. R., Carlos, C., Chevrette, M. G., Horn, H. A., McDonald, B. R., Stankey, R. K., et al. (2016). Evolution and ecology of Actinobacteria and their bioenergy applications. Ann. Rev. Microbiol. 70, 235–254. doi: 10.1146/annurev-micro-102215-095748
Li, B., Yang, Y., Ma, L., Ju, F., Guo, F., Tiedje, J. M., et al. (2015). Metagenomic and network analysis reveal wide distribution and co-occurrence of environmental antibiotic resistance genes. ISME J. 9, 2490–2502. doi: 10.1038/ismej.2015.59
Li, D., Liu, C.-M., Luo, R., Sadakane, K., and Lam, T.-W. (2015). MEGAHIT: An ultra-fast single-node solution for large and complex metagenomics assembly via succinct de Bruijn graph. Bioinform. doi: 10.1093/bioinformatics/btv033
Li, Y., Cao, W., Liang, S., Yamasaki, S., Chen, X., Shi, L., et al. (2020). Metagenomic characterization of bacterial community and antibiotic resistance genes in representative ready-to-eat food in southern China. Sci. Rep. 10:15175. doi: 10.1038/s41598-020-72620-4
Liao, X. B., Chen, C., Wang, Z., Wan, R., Chang, C. H., Zhang, X., et al. (2013). Changes of biomass and bacterial communities in biological activated carbon filters for drinking water treatment. Process Biochem. 48, 312–316. doi: 10.1016/j.procbio.2012.12.016
Liu, Z., Huang, S., Sun, G., Xu, Z., and Xu, M. (2012). Phylogenetic diversity, composition and distribution of bacterioplankton community in the Dongjiang River, China. FEMS Microbiol. Ecol. 80, 30–44. doi: 10.1111/j.1574-6941.2011.01268.x
Liu, K., Li, Y., Ge, Z., Huang, D., and Zhang, J. (2024). Microbial communities and mobile genetic elements determine the variations of antibiotic resistance genes for a continuous year in the urban river deciphered by metagenome assembly. Environ. Pollut. 362:125018. doi: 10.1016/j.envpol.2024.125018
Ma, L. P., Li, B., and Zhang, T. (2014). Abundant rifampin resistance genes and significant correlations of antibiotic resistance genes and plasmids in various environments revealed by metagenomic analysis. Appl. Microbiol. Biotechnol. 98, 5195–5204. doi: 10.1007/s00253-014-5511-3
Madsen, E. L. (2011). Microorganisms and their roles in fundamental biogeochemical cycles. Curr. Opin. Biotechnol. 22, 456–464. doi: 10.1016/j.copbio.2011.01.008
Mahanta, C., Zaman, A. M., Newaz, S. M. S., Rahman, S. M. M., Mazumdar, T. K., Choudhury, R., et al. (2014). Physical assessment of the Brahmaputra River. Dhaka, Bangladesh: IUCN, International Union for Conservation of Nature, xii + 74.
Malakar, D., Sarathbabu, S., Borah, P., and Kumar, N. S. (2021). Fish gill microbiome from India’s largest Brahmaputra River- a trans-border biodiversity hotspot region. Environ. Monit. Assess. 193:56. doi: 10.1007/s10661-021-08847-z
Marathe, N. P., Regina, V. R., Walujkar, S. A., Charan, S. S., Moore, E. R., Larsson, D. J., et al. (2013). A treatment plant receiving waste water from multiple bulk drug manufacturers is a reservoir for highly multi-drug resistant integrin-bearing bacteria. PLoS One 8:e77310. doi: 10.1371/journal.pone.0077310
Masuda, Y., Mise, K., Xu, Z., Zhang, Z., Shiratori, Y., Senoo, K., et al. (2022). Global soil metagenomics reveals ubiquitous yet previously-hidden predominance of 3 Deltaproteobacteria in nitrogen-fixing microbiome. bioRxiv. doi: 10.1101/2022.12.09.519847
Mawang, C. I., Azman, A. S., Fuad, A. M., and Ahamad, M. (2021). Actinobacteria: an eco-friendly and promising technology for the bioaugmentation of contaminants. Biotechnol. Rep. 32:e00679. doi: 10.1016/j.btre.2021.e00679
Medeiros, J. D., Cantão, M. E., Cesar, D. E., Nicolás, M. F., Diniz, C. G., Silva, V. L., et al. (2016). Comparative metagenome of a stream impacted by the urbanization phenomenon. Braz. J. Microbiol. 47, 835–845. doi: 10.1016/j.bjm.2016.06.011
Mencía-Ares, O., Cabrera-Rubio, R., Cobo-Díaz, J. F., Álvarez-Ordóñez, A., Gómez-García, M., Puente, H., et al. (2020). Antimicrobial use and production system shape the fecal, environmental, and slurry resistomes of pig farms. Microbiome 8:164. doi: 10.1186/s40168-020-00941-7
Mori, J. F., Chen, L.-X., Jessen, G. L., Rudderham, S. B., McBeth, J. M., Lindsay, M. B. J., et al. (2019). Putative Mixotrophic nitrifying-denitrifying Gammaproteobacteria implicated in nitrogen cycling within the Ammonia/oxygen transition zone of an Oil Sands pit Lake. Front. Microbiol. 10:2435. doi: 10.3389/fmicb.2019.02435
Morya, R., Salvachua, D., and Thakur, I. S. (2020). Burkholderia: an untapped, promising bacterial genus for the conversion of aromatic compounds. Trends Biotechnol. 38, 963–975. doi: 10.1016/j.tibtech.2020.02.008
NDMA (2012). Final report on study of Brahmaputra River erosion and its control, 337. Available at: https://ndma.gov.in/sites/default/files/PDF/Technical%20Documents/NDMA%20Final%20Report%20Brahmaputra%20River.pdf
Newton, R. J., Jones, S. E., Eiler, A., McMahon, K. D., and Bertilsson, S. (2011). A guide to the natural history of freshwater lake bacteria. Microbiol. Mol. Biol. Rev. 75, 14–49. doi: 10.1128/MMBR.00028-10
Nguyen, S. G., Raza, S., Ta, L. T., Le, L.-A. T., Ho, C. T., and Unno, T. (2022). Metagenomic investigation of the seasonal distribution of bacterial community and antibiotic-resistant genes in Day River downstream, Ninh Binh, Vietnam. Appl. Biol. Chem. 65:26. doi: 10.1186/s13765-022-00687-w
Oni, O. E., Schmidt, F., Miyatake, T., Kasten, S., Witt, M., Hinrichs, K.-U., et al. (2015). Microbial communities and organic matter composition in surface and subsurface sediments of the Helgoland mud area, North Sea. Front. Microbiol. 6:1290. doi: 10.3389/fmicb.2015.01290
Parida, P. K., Behera, B. K., Dehury, B., Rout, A. K., Rai, A., Das, B. K., et al. (2022). Community structure and function of microbiomes in polluted stretches of river Yamuna in New Delhi, India, using shotgun metagenomics. Environ. Sci. Pollut. Res. 29, 71311–71325. doi: 10.1007/s11356-022-20766-1
Pham, T. T. H., Rossi, P., Dinh, H. D. K., Pham, N. T. A., Tran, P. A., Ho, T., et al. (2018). Analysis of antibiotic multi-resistant bacteria and resistance genes in the effluent of an intensive shrimp farm (long an, Vietnam). J. Environ. Manag. 214, 149–156. doi: 10.1016/j.jenvman.2018.02.089
Prado, T., Bruni, A. D. C., Barbosa, M. R. F., Bonanno, V. M. S., Garcia, S. C., and Sato, M. I. Z. (2018). Distribution of human fecal marker GB-124 bacteriophages in urban sewage and reclaimed water of São Paulo city, Brazil. J. Water Health 16, 289–299. doi: 10.2166/wh.2017.011
Raiyani, N. M., and Singh, S. P. (2020). Taxonomic and functional profiling of the microbial communities of Arabian Sea: a metagenomics approach. Genomics 112, 4361–4369. doi: 10.1016/j.ygeno.2020.07.024
Rakhecha, P. R. (2020). Water environment pollution with its impact on human diseases in India. Int. J. Hydrol. 4, 152–158. doi: 10.15406/ijh.2020.04.00240
Rathour, R., Gupta, J., Mishra, A., Rajeev, A. C., Dupont, C. L., and Thakur, I. S. (2020). A comparative metagenomic study reveals microbial diversity and their role in the biogeochemical cycling of Pangong lake. Sci. Total Environ. 731:139074. doi: 10.1016/j.scitotenv.2020.139074
Ray, P. A., Yang, Y.-C. E., Wi, S., Khalil, A., Chatikavanij, V., and Brown, C. (2015). Room for improvement: Hydroclimatic challenges to poverty-reducing development of the Brahmaputra River basin. Environ. Sci. Pol. 54, 64–80. doi: 10.1016/j.envsci.2015.06.015
Reddy, B., and Dubey, S. K. (2019). River Ganges water as reservoir of microbes with antibiotic and metal ion resistance genes: high throughput metagenomic approach. Environ. Pollut. 246, 443–451. doi: 10.1016/j.envpol.2018.12.022
Reddy, B., Pandey, J., and Dubey, S. K. (2019). Assessment of environmental gene tags linked with carbohydrate metabolism and chemolithotrophy associated microbial community in river ganga. Gene 704, 31–41. doi: 10.1016/j.gene.2019.04.004
Rout, A. K., Dehury, B., Parida, P. K., Sarkar, D. J., Das, B. K., Rai, A., et al. (2022). Taxonomic profling and functional gene annotation of microbial communities in sediment of river ganga at Kanpur, India: insights from whole-genome metagenomics study. Environ. Sci. Pollut. Res. 29, 82309–82323. doi: 10.1007/s11356-022-21644-6
Rout, A. K., Tripathy, P. S., Dixit, S., Behera, D. U., Behera, B., Das, B. K., et al. (2024). Metagenomics analysis of sediments of river ganga, India for bacterial diversity, functional genomics, antibiotic resistant genes and virulence factors. Curr. Res. Biotechnol. 7:100187. doi: 10.1016/j.crbiot.2024.100187
Rozwandowicz, M., Brouwer, M. S. M., Fischer, J., Wagenaar, J. A., Gonzalez-Zorn, B., Guerra, B., et al. (2018). Plasmids carrying antimicrobial resistance genes in Enterobacteriaceae. J. Antimicrob. Chemother. 73, 1121–1137. doi: 10.1093/jac/dkx488
Ruiz, O. N., Radwan, O., and Striebich, R. C. (2021). GC–MS hydrocarbon degradation profile data of Pseudomonas frederiksbergensis SI8, a bacterium capable of degrading aromatics at low temperatures. Data Brief 35:106864. doi: 10.1016/j.dib.2021.106864
Samson, R., Shah, M., Yadav, R., Sarode, P., Rajput, V., Dastager, S. G., et al. (2019). Metagenomic insights to understand transient infuence of Yamuna River on taxonomic and functional aspects of bacterial and archaeal communities of river Ganges. Sci. Total Environ. 674, 288–299. doi: 10.1016/j.scitotenv.2019.04.166
Sandifer, P. A., Sutton-Grier, A. E., and Ward, B. P. (2015). Exploring connections among nature, biodiversity, ecosystem services, and human health and well-being: opportunities to enhance health and biodiversity conservation. Ecosyst. Serv. 12, 1–15. doi: 10.1016/j.ecoser.2014.12.007
Sang, Y., Mo, S., Zeng, S., Wu, X., Kashif, M., Song, J., et al. (2023). Model of shrimp pond-mediated spatiotemporal dynamic distribution of antibiotic resistance genes in the mangrove habitat of a subtropical gulf. Sci. Total Environ. 905:167199. doi: 10.1016/j.scitotenv.2023.167199
Santillan, E., Seshan, H., Constancias, F., Drautz-Moses, D. I., and Wuertz, S. (2019). Frequency of disturbance alters diversity, function, and underlying assembly mechanisms of complex bacterial communities. NPJ Biofilms Microbiomes 5:8. doi: 10.1038/s41522-019-0079-4
Savio, D., Sinclair, L., Ijaz, U. Z., Parajka, J., Reischer, G. H., Stadler, P., et al. (2015). Bacterial diversity along a 2600 km river continuum. Environ. Microbiol. 17, 4994–5007. doi: 10.1111/1462-2920.12886
Saxena, G., Marzinelli, E. M., Naing, N. N., He, Z., Liang, Y., Tom, L., et al. (2015). Ecogenomics reveals metals and land-use pressures on microbial communities in the waterways of a megacity. Environ. Sci. Technol. 49, 1462–1471. doi: 10.1021/es504531s
Segata, N., Waldron, L., Ballarini, A., Narasimhan, V., Jousson, O., and Huttenhower, C. (2012). Metagenomic microbial community profiling using unique clade-specific marker genes. Nat. Methods. 9, 811–814. doi: 10.1038/nmeth.2066
Singh, V. P., Sharma, N., Shekhar, C., and Ojha, P. (2004). The Brahmaputra Basin water resources. Dordrecht, Netherlands: Kluwer Academic Publishers.
Singleton, D. R., Dickey, A. N., Scholl, E. H., Wrigh, F. A., and Aitken, M. D. (2015). Complete genome sequence of a novel bacterium within the family Rhodocyclaceae that degrades polycyclin aromatic hydrocarbons. Genome Announc. 3, e00251–e00215. doi: 10.1128/genomeA.00251-15
Spain, A., Krumholz, L., and Elshahed, M. (2009). Abundance, composition, diversity and novelty of soil Proteobacteria. ISME J. 3, 992–1000. doi: 10.1038/ismej.2009.43
Srivastava, A., and Verma, D. (2023). Ganga River sediments of India predominate with aerobic and chemo-heterotrophic bacteria majorly engaged in the degradation of xenobiotic compounds. Environ. Sci. Pollut. Res. 30, 752–772. doi: 10.1007/s11356-022-22198-3
Sukumar, S., Roberts, A. P., Martin, F. E., and Adler, C. J. (2016). Metagenomic insights into transferable antibiotic resistance in Oral Bacteria. J. Dent. Res. 95, 969–976. doi: 10.1177/0022034516648944
Taylor, N. G. H., Verner-Jeffreys, D. W., and Baker-Austin, C. (2011). Aquatic systems: maintaining, mixing and mobilising antimicrobial resistance? Trends Ecol. Evol. 26, 278–284. doi: 10.1016/j.tree.2011.03.004
Toyama, D., Kishi, L. T., Santos-Júnior, C. D., Soares-Costa, A., de Oliveira, T. C. S., de Miranda, F. P., et al. (2016). Metagenomics analysis of microorganisms in freshwater lakes of the Amazon Basin. Genome Announc. 4, e01440–e01416. doi: 10.1128/genomeA.01440-16
Trujillo, M. E., Riesco, R., Benito, P., and Carro, L. (2015). Endophytic Actinobacteria and the interaction of Micromonospora and nitrogen fixing plants. Front. Microbiol. 6:1341. doi: 10.3389/fmicb.2015.01341
Tsagaraki, T. M., Pree, B., Leiknes, Ø., Larsen, A., Bratbak, G., Øvreås, L., et al. (2018). Bacterial community composition responds to changes in copepod abundance and alters ecosystem function in an Arctic mesocosm study. ISME J. 12, 2694–2705. doi: 10.1038/s41396-018-0217-7
Vogel, T. M., Simonet, P., Jansson, J. K., Hirsch, P. R., Tiedje, J. M., Elsas, J. D., et al. (2009). TerraGenome: a consortium for the sequencing of a soil metagenome. Nat. Rev. Microbiol. 7:252. doi: 10.1038/nrmicro2119
Wagner, P. L., and Waldor, M. K. (2002). Bacteriophage control of bacterial virulence. Infect. Immun. 70, 3985–3993. doi: 10.1128/IAI.70.8.3985-3993.2002
Wang, X., Cao, A., Zhao, G., Zhou, C., and Xu, R. (2017). Microbial community structure and diversity in a municipal solid waste landfill. Waste Manag. 66, 79–87. doi: 10.1016/j.wasman.2017.04.023
Wang, J., Chu, L., Wojnarovits, L., and Takacs, E. (2020). Occurrence and fate of antibiotics, antibiotic resistance genes (ARGs) and antibiotic resistance bacteria (ARB) in municipal wastewater treatment plant. An overview. Sci. Total Environ. 744:140997. doi: 10.1016/j.scitotenv.2020.140997
Wood, D. E., and Salzberg, S. L. (2014). Kraken ultrafast metagenomic sequence classification using exact alignments. Genome Biol. 15–R46.
Xu, R., Yang, Z. H., Wang, Q. P., Bai, Y., Liu, J. B., Zheng, Y., et al. (2018). Rapid startup of thermophilic anaerobic digester to remove tetracycline and sulfonamides resistance genes from sewage sludge. Sci. Total Environ. 612, 788–798. doi: 10.1016/j.scitotenv.2017.08.295
Yang, Q. E., Sun, J., Li, L., Deng, H., Liu, B. T., Fang, L. X., et al. (2015). IncF plasmid diversity in multi-drug resistant Escherichia coli strains from animals in China. Front. Microbiol. 6:964. doi: 10.3389/fmicb.2015.00964
Yi, Y., Lin, C., Wang, W., and Song, J. (2021). Habitat and seasonal variations in bacterial community structure and diversity in sediments of a shallow lake. Ecol. Indic. 120:106959. doi: 10.1016/j.ecolind.2020.106959
Zainun, M. Y., and Simarani, K. (2018). Metagenomics profiling for assessing microbial diversity in both active and closed landfills. Sci. Total Environ. 616–617, 269–278. doi: 10.1016/j.scitotenv.2017.10.266
Zhang, T., Zhang, X. X., and Ye, L. (2011). Plasmid metagenome reveals high levels of antibiotic resistance genes and mobile genetic elements in activated sludge. PLoS One 6:e26041. doi: 10.1371/journal.pone.0026041
Zhao, Y., Zhang, X. X., Zhao, Z., Duan, C., Chen, H., Wang, M., et al. (2018). Metagenomic analysis revealed the prevalence of antibiotic resistance genes in the gut and living environment of freshwater shrimp. J. Hazard. Mater. 350, 10–18. doi: 10.1016/j.jhazmat.2018.02.004
Zhuang, M., Achmon, Y., Cao, Y., Liang, X., Chen, L., Wang, H., et al. (2021). Dsitribution of antibiotic resistance genes in the environment. Environ. Pollut. 285:117402. doi: 10.1016/j.envpol.2021.117402
Zothanpuia, P., Passari, A. K., Leo, V. V., Chandra, P., Kumar, B., Nayak, C., et al. (2018). Bioprospection of actinobacteria derived from freshwater sediments for their potential to produce antimicrobial compounds. Microb. Cell Factories 17:68. doi: 10.1186/s12934-018-0912-0
Keywords: river sediment, Brahmaputra River, metagenomics, microbial communities, antibiotic resistance genes, anthropogenic activities
Citation: Sharma N, Das BK, Bhattacharjya BK, Chaudhari A, Behera BK, Kumar AP and Chakraborty HJ (2024) Metagenomic insights into microbial community, functional annotation, and antibiotic resistance genes in Himalayan Brahmaputra River sediment, India. Front. Microbiol. 15:1426463. doi: 10.3389/fmicb.2024.1426463
Edited by:
Danny Ionescu, Technical University of Berlin, GermanyReviewed by:
Tanja Vojvoda Zeljko, Ruđer Bošković Institute, CroatiaSujata Dabolkar, Government College of Arts, Science and Commerce, Quepem, India
Copyright © 2024 Sharma, Das, Bhattacharjya, Chaudhari, Behera, Kumar and Chakraborty. This is an open-access article distributed under the terms of the Creative Commons Attribution License (CC BY). The use, distribution or reproduction in other forums is permitted, provided the original author(s) and the copyright owner(s) are credited and that the original publication in this journal is cited, in accordance with accepted academic practice. No use, distribution or reproduction is permitted which does not comply with these terms.
*Correspondence: Basanta Kumar Das, YmFzYW50YWt1bWFyZEBnbWFpbC5jb20=; QmFzYW50YS5kYXNAaWNhci5nb3YuaW4=