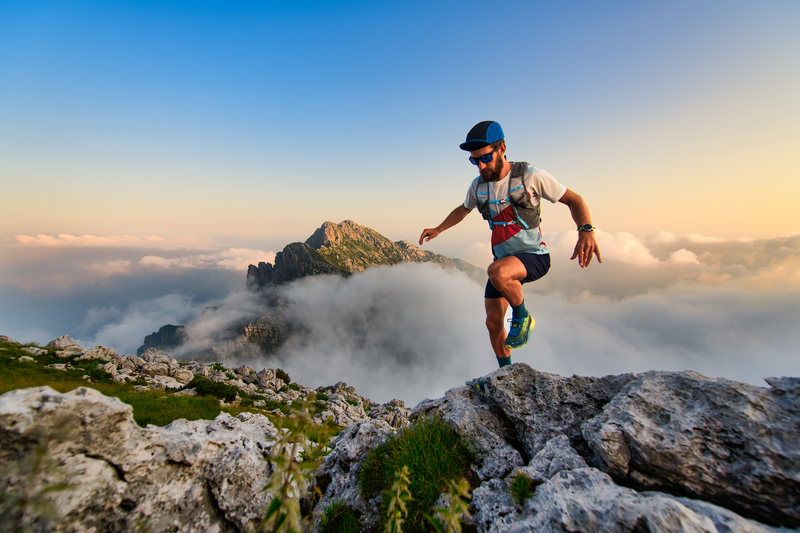
94% of researchers rate our articles as excellent or good
Learn more about the work of our research integrity team to safeguard the quality of each article we publish.
Find out more
ORIGINAL RESEARCH article
Front. Microbiol. , 28 September 2023
Sec. Terrestrial Microbiology
Volume 14 - 2023 | https://doi.org/10.3389/fmicb.2023.1229873
This article is part of the Research Topic Mineral Solubilizing Microorganisms (MSM) and their Applications in Nutrient Bioavailability, Bioweathering and Bioremediation, Volume II View all 7 articles
Introduction: Rhizosphere bacterial communities play a crucial role in promoting plant and soil ecosystem health and productivity. They also have great potential as key indicators of soil health in agroecosystems. Various environmental factors affect soil parameters, which have been demonstrated to influence soil microbial growth and activities. Thus, this study investigated how rhizosphere bacterial community structure and functions are affected by agronomic practices such as organic and conventional fertiliser application and plant species types.
Methods: Rhizosphere soil of vegetable crops cultivated under organic and conventional fertilisers in different farms was analysed using high-throughput sequencing of the 16S rRNA gene and co-occurrence network pattern among bacterial species. The functional structure was analysed with PICRUSt2 pipeline.
Results: Overall, rhizosphere bacterial communities varied in response to fertiliser type, with soil physicochemical parameters, including NH4, PO4, pH and moisture content largely driving the variations across the farms. Organic farms had a higher diversity richness and more unique amplicon sequence variants than conventional farms. Bacterial community structure in multivariate space was highly differentiated across the farms and between organic and conventional farms. Co-occurrence network patterns showed community segmentation for both farms, with keystone taxa more prevalent in organic than conventional farms.
Discussion: Module hub composition and identity varied, signifying differences in keystone taxa across the farms and positive correlations between changes in microbial composition and ecosystem functions. The organic farms comprised functionally versatile communities characterised by plant growth-promoting keystone genera, such as Agromyces, Bacillus and Nocardioides. The results revealed that organic fertilisers support high functional diversity and stronger interactions within the rhizosphere bacterial community. This study provided useful information about the overall changes in soil microbial dynamics and how the changes influence ecosystem functioning under different soil nutrient management and agronomic practices.
Soil microbes participate in nutrient cycling, organic matter decomposition and energy flow, which are key in sustaining soil ecosystem functions (Ling et al., 2022). However, soil nutrient management, soil properties and climatic conditions, which vary across geographic locations alter how soil ecosystem function through a possible shift in microbial diversity and functions (Ge et al., 2008; Ahkami et al., 2017; Yang et al., 2021). Substantial responses to changes in land use and soil nutrient build-up have been reported for both plant and soil microbial communities, with potential consequences for ecosystem functioning (Nielsen et al., 2015). Soil microbial diversity is vast and its interactions with other ecosystem components (e.g., vegetation and soil parameters) are complex, which significantly impacts the correlation between soil microbial diversity and ecosystem functions in response to disturbances (Garnica et al., 2020). Thus, insights into soil bacteriome structure and how it is affected by agronomic practices are key to making informed decisions that will promote agroecosystem sustainability.
Currently, organic and conventional fertilizers are the main agro-inputs for improving soil nutrient content (Musyoka et al., 2017). Improved soil nutrient content enhances overall plant health by altering the soil parameters and predisposing the selection of certain rhizosphere bacterial communities with unique ecosystem functions. Consequently, the plant and microbes interactions in the soil, along with the above-ground productivity, are affected by the type of fertilizer applied (Ahkami et al., 2017). Using phospholipid fatty acids, a study has reported that organically managed soil enhanced bacterial and fungal biomass, with an increase in total microbial metabolic activity and soil organic matter. Thus, soil nutrient management types could drastically impact soil ecosystem functioning, through the imbalances caused by fertilizer application and nutrient deposition (Martínez-García et al., 2018).
Furthermore, plant species or genotypes influence the synthesis of unique exudates and extracellular enzymes, which impact microscale spatial patterns in soil bacterial communities and differentially stimulate the growth of specific microbial groups under different ecosystems across geographic locations (Hoch et al., 2019; Yan et al., 2021). Usually, plants exert a selective influence on their rhizo-microbiome to acquire key beneficial traits (Jaramillo et al., 2016), indicating rhizosphere microbial communities are a reflection of plant species and plant-beneficial genetic functions. For example, legumes harbor bacteria with nitrogenases that enhance their N-fixing ability (Lugtenberg, 2015). Similarly, plant-associated microbes, which perform functions such as nitrate reduction, denitrification, nutrient solubilization, and production of phytohormones such as indole acetic acid, ethylene and siderophore are a reflection of the host plant’s needs (Raimi et al., 2017). These microbial functions influence plant productivity and health, which in turn drive soil bacterial diversity and ecosystem functions (Mendes et al., 2013).
Soil parameters vary by geographic location and primarily provide similar conditions that affect the activities of plants and their associated microbiome to soil microbes (Mendes et al., 2013). Studies have shown that factors such as soil pH, carbon, organic matter and nutrient content drive spatial patterns of soil microbial diversity within an ecosystem (Bardgett and Caruso, 2020; Nan et al., 2020). Spatial patterns of soil microbial community due to plant species and fertilizer types and how it relates to bacterial taxonomic and functional profiles remain largely underexplored. Increase in land-use intensification is reported to alter soil microbial composition (Felipe-Lucia et al., 2020) and keystone taxa, which are crucial for microbial community structure and ecosystem functioning (Banerjee et al., 2018). Due to the importance and unique role of keystone taxa, their interactions or loss can trigger a shift in microbiome structure (Yang et al., 2021). Interactions between microbial community members have been widely assessed using microbial co-occurrence network analysis (Li et al., 2018; Xu et al., 2022). Thus, co-occurrence patterns and microbial networks are key to understanding how bacterial community interactions and keystone taxa distribution and functions change under different agronomic practices (Huang et al., 2019; Hernandez D. J. et al., 2021).
Consequently, this study assessed the effects of fertilizer type (organic vs. conventional) and plant species type on the microbial dynamics (including keystone taxa) and ecosystem functions of vegetable rhizosphere soil across different farms. It is hypothesized that fertilizer type in each of the farming systems predisposes the selection of certain rhizobacterial communities, which possess diverse but unique functional gene repertoire of agronomic importance. Results from this study could provide baseline information useful in predictive modeling for manipulating soil bacterial communities to improve agroecosystems. Similarly, the approach used in this study could unravel keystone taxa with unique biomarker potential for monitoring soil health and productivity in a given nutrient management system.
Rhizosphere soils were collected from four vegetable farms located in the North West (farms B and S) and Gauteng (farms J and T) provinces of South Africa. Farm B (26°42′55.0″S 27°04′59.6″E) and farm S (26°47′43.5″S 27°02′18.3″E) are situated in JB marks municipality, while farm J (26°11′40.1″S 28°03′58.3″E) and farm T (26°03′16.0″S 27°40′13.3″E) are in Johannesburg and West Rand District municipalities, respectively. The North West province is characterized as a semi-arid climate while the Gauteng province is classified as a mild climate that is neither humid nor too hot (www.sa-venues.com/weather/). Average annual temperature and rainfall are 22.36°C and 36.6 mm for North West province and 20.64°C and 56.11 mm for Gauteng province. The average annual relative humidity for North West and Gauteng provinces are 37.8 and 50.22%, respectively (Weather and Climate, 2023). Vegetable farming is a major agricultural production in the two provinces, with higher production in the North West compared with the Gauteng province (Macaskill, 2016). The farms have been cultivating various vegetables, including cabbage, lettuce, onions, and spinach for over 2 years on the same plot. The Gauteng province farms (farm J and T) practice conventional farming, using chemical fertilizers, pesticides and fungicides, whereas the farms in the North West province (farm B and S) practice mainly organic farming, utilizing plant and animal waste. The organic farms use compost, green waste, and animal manure (poultry, sheep, goat, and cow), while the conventional farms use chemical fertilizers including NPK fertilizers, urea, ammonium nitrate, diammonium phosphate, and pesticides such as carbamates, organophosphates and pyrethroid (Personal communication).
Sampling was conducted in the summer (October–February) of 2021. Based on visual inspection, rhizosphere soils of healthy vegetables of the same age and size including, Allium cepa (onion), Brassica oleracea (cabbage), Lactuca sativa (lettuce), and Spinacia oleracea (spinach), were sampled from each farm as described by Barillot et al. (2013), with some modifications. Briefly, vegetables were uprooted and shaken vigorously to remove bulk soil from the roots. Thereafter, the rhizosphere soil was collected by hand-shaking several undamaged roots to release the adhering soil into a sterile beaker. Five replicate samples were collected for each plant per farm and an equal quantity (50 g each) of the soils from the same vegetable for each farm were pooled to obtain a composite sample. An additional sample of the rhizosphere soil was collected from each farm; a different vegetable type was sampled at each farm, bringing the total number of study samples to 20. Samples were immediately placed in sterile zip-lock bags, transported to the laboratory on ice and stored for further analysis.
The soil pH was measured from a 1:2.5 soil-water suspension using a pre-calibrated pH meter (HQ40d Hach, United States), while the electrical conductivity (EC) was determined with an EC meter. The amounts of macronutrients (N, P, K, and Ca), micronutrients (Mn, Fe, Zn, and Cu), heavy metals (Pb, Cd, Cr, Hg, and As) and exchangeable cations (Ca2+, Mg2+, K+, and Na+) in the soil were determined using the inductively coupled plasma mass spectrometry (ICP-MS), following standard procedure (Bulska and Wagner, 2016). Other physicochemical properties, such as soil texture (sand, silt, and clay proportion), cation exchange capacity (CEC), moisture and organic matter (OM) content, and the soil enzyme activities, including acid and alkaline phosphatases, β-glucosidase, urease and dehydrogenase activity were analyzed at the Eco-Analytical, North-West University, South Africa, following standardized methods described by the Soil Science Society of South Africa (SSSSA, 1990).
Soil community DNA was extracted using Power Soil DNA extraction kits (Qiagen, Hilden, Germany) following the manufacturer’s instructions. The DNA concentration was measured with a Qubit fluorometer (Invitrogen, Carlsbad, CA, United States) and the integrity was checked using gel electrophoresis with a 1% agarose gel. Extracted DNA was normalized to equimolar concentrations (5 ng/μl) using 0.1 M Tris-HCl (pH 8.5) and the partial 16S rRNA gene (V3-V4 region), a universal barcode for the characterization of bacteria, was amplified in a polymerase chain reaction (PCR) using Illumina-barcoded 341F and 805R primers (Klindworth et al., 2013). The 16S rRNA gene library was prepared as described by van Wyk et al. (2017). Paired-end (2 × 300 bp) sequencing of the gene libraries was performed on the Illumina MiSeq sequencer using the Nextera v3 kit (Illumina Inc., San Diego, CA, United States). Sequencing was performed at the sequencing facility of the Unit for Environmental Sciences and Management, North-West University, Potchefstroom, South Africa.
The 16S rRNA gene sequence reads were demultiplexed and trimmed of barcodes using MiSeq Reporter software (Illumina Inc., San Diego, CA, United States) and then quality checked with FastQC (v. 0.11.5) (Babraham Bioinformatics, UK). Low-quality reads were trimmed with trimmomatic software (v. 0.38) (Bolger et al., 2014) and reads with mean nucleotide base quality score of less than 20 (Phred Q score) were removed. Reads were analyzed using the Quantitative Insight into Microbial Ecology v2 platform (QIIME 2, Release 2020.11; Bolyen et al., 2019). Quality-filtered reads were denoised and clustered into amplicon sequence variants (ASVs) using DADA2 denoiser (Callahan et al., 2016) with a pre-trained classifier of the SILVAngs rRNA gene reference (release 138) (Quast et al., 2013). After singleton removal, the ASV table was rarefied to an even depth before the taxonomic assignment and diversity analyzes in QIIME 2. Alpha diversity indices such as the Shannon-Wiener index, inverse Simpson index, Chao1 richness estimator and phylogenetic diversity were analyzed in R v 4.1.1 (R Development Core Team, 2018).
The functional metagenomic profile of the absolute abundance of microbial genera was predicted using the Tax4Fun2 package (Wemheuer et al., 2020) in R (R Development Core Team, 2018). Firstly, the ASVs were searched against the 16S rRNA gene reference sequences using the basic local alignment search tool (BLAST) via the runRefBlast function. Thereafter, the functional prediction was evaluated using the makeFunctionalPrediction, where the ASVs were summarized based on the results of the next neighbor search (Wemheuer et al., 2018). Predicted profiles were annotated based on the Kyoto Encyclopaedia of Genes and Genomes (KEGG) orthology (KO) pathways (Kanehisa et al., 2012). KO terms and metabolic pathways were generated for the ASVs and the relative abundance of the functional genes was calculated. To gain insights into the influence of agronomic practices on bacterial community functional genes, only a few of the KO terms contributing to major ecosystem functions (e.g., organic matter decomposition and biogeocycling), plant growth and nutrient metabolism were investigated.
Bacterial community co-occurrence patterns were analyzed by constructing ecological networks using the Random matrix theory (RMT)-based method in the molecular ecological network analysis (MENA) pipeline1 (Faust and Raes, 2016). To assess the impact of fertilizer type on soil bacterial structure, organic and conventional farm networks were constructed. Network complexity was reduced by using only ASVs present in at least 40% of the samples across the organic and conventional farms and the Pearson correlation coefficient was employed for data transformation. Using a multilevel modularity optimization algorithm, bacterial community groups were identified by clustering networks into modules (Blondel et al., 2008). Node connectivity was established based on among-module connectivity (Pi) and within-module connectivity (Zi); creating four sub-categories of nodes (a) peripheral (Zi < 2.5; Pi < 0.62), (b) connectors (Zi < 2.5; Pi > 0.62), (c) module hubs (Zi > 2.5; Pi < 0.62) and (d) network hubs (Zi > 2.5; Pi > 0.62) (Guimera and Amaral, 2005). The networks were visualized in Gephi v 0.9.2.
All statistical analyzes were performed in R software (v.4.1.1) unless otherwise stated. The significance for all tests was set at p < 0.05 and the data was tested across fixed factors: farms, fertilizer types (organic vs. conventional farms) and vegetable species. Data normality was tested using the Shapiro–Wilk test and non-normal data was transformed with log10, square root or sine to fit a normal distribution. Normal or non-normal data were analyzed using parametric or non-parametric tests, respectively. Correlation between soil physicochemical and enzyme activity data was tested using Pearson or Spearman rank correlation for normalized or non-normal datasets. The community structure across the farms, and between organic and conventional farm soil was visualized with a nonmetric multidimensional scaling (NMDS) plot and a cluster dendrogram using vegan and dendextend (v. 1.12.0) (Galili, 2015) packages in R studio. Differences in multivariate space across the fixed factors were performed with Bray-Curtis dissimilarity using permutational multivariate analysis of variance (PERMANOVA) and Permutational test for homogeneity of multivariate dispersion (PERMDISP). Linear Discriminant Analysis (LDA) Effect size (LEfSe) (Segata et al., 2011) was performed to detect differentially abundant genera (Mann–Whitney U test or Kruskal-Wallis test, p-value < 0.05; LDA score > 2) between the farms, organic and conventional farms and plant species using microeco package in R software (Liu et al., 2021). Subsequently, the LEfSe results were visualized as bar plots. An indicator species functional analysis was performed for predicted KO terms to detect the most discriminatory KOs between the fixed factors. KO terms with false discovery rate (FDR)-adjusted p < 0.05 and an indicator value of > 0.1 were taken to be discriminant for the fixed factors. Redundancy analysis (RDA) was performed in R software to show the physicochemical parameters that best explain the variations in the microbial community composition.
The 16S rRNA gene sequence data for this study are available in the sequence read archives (SRA) of the National Centre for Biotechnological Information under a BioProject with SRA accession no PRJNA904574 (https://www.ncbi.nlm.nih.gov/bioproject/904574).
The soil physicochemical parameters varied across the fixed factors with no particular trend. A near-neutral pH range was observed across the farms, with the conventional farms having a higher pH than the organic farms (Table 1). The moisture and OM content ranged from 1.06–1.42% and 4.98–5.89%, respectively. Organic farms largely had a higher CEC and OM content than conventional farms. Farms mostly had low quantities of microelements and heavy metals, except for Pb and As in farm J (Table 1). Soil texture was mainly classified as sandy-clay-loam for organic farms and sandy-loam for conventional farms. Some of the soil physicochemical properties significantly (Kruskal-Wallis test, p < 0.05) differed across the farms (Table 1), while only the differences in OM, clay proportion, CEC, NH4, P, and Cu were statistically significant (Mann–Whitney or t-test, p < 0.05) across the fertilizer types.
The soil enzyme activities were significantly (Kruskal-Wallis H test, p < 0.05) different across the farms and fertilizer type, except for alkaline phosphatase, which was not influenced by the fertilizer type. Mostly, the enzyme activities were higher for organic farms compared to conventional farms (Table 2). The alkaline and acid phosphatases had a very low range of activities across the farms. Comparing among farms, farm B had the highest dehydrogenase and β-glucosidase activities, while farm S had the highest activity for urease. Farm J had the lowest urease activity, which is approximately five, 12 and 23 folds lower compared to farms B, T, and S, respectively (Table 2). The main effect of crop type and the interactions between the fixed factors on soil enzyme activities were not statistically significant (Kruskal-Wallis H test, p > 0.05).
A total of 1,933,349 16S rRNA gene reads was obtained from all samples, with a mean read count of 96,667 per sample. Quality-filtered reads were clustered into 28,604 ASVs after pruning low count and low variance features. The ASVs were rarefied to an equal depth of 74,170 (rarefaction curve in Supplementary Figure S1) before examining the treatment effects. The distribution and richness of ASVs differed substantially across the farms, with farm S having the highest number of unique ASVs (Figure 1A). Observed ASVs were higher in organic compared to conventional farms and 276 ASVs were shared among all the farms (Figures 1A,B). Comparing the shared ASVs in the organic (Supplementary Figure S2A) with the conventional (Supplementary Figure S2B) farms, 1,222 and 313 ASVs were exclusive to the organic and conventional farms, respectively (Figure 1B). Similarly, ASV richness differed across the rhizosphere of each vegetable species. Unique ASVs were higher in cabbage, followed by lettuce and onion rhizosphere, while spinach and lettuce rhizosphere share more ASVs (Supplementary Figure S3).
Figure 1. Comparison of unique and shared amplicon sequence variants (ASVs) richness. (A) ASV richness across the farms, (B) unique ASVs between organic and conventional farms.
The alpha diversity indices measured were not significantly influenced by the main effect of fertilizer type and plant species, except for the inverse Simpson index, which was significant (ANOVA, F = 3.65, p = 0.035) across the farms, with the pairwise comparison (Tukey HSD, P-adjusted = 0.0218) showing differences between farms T and S. In addition, the combined effects of the fixed factors (fertilizer type and plant species) and their interactions had no significant effect (general linear model, p > 0.05) on the alpha diversity indices measured.
Bacterial community structure was highly differentiated across the farms and between the organic and conventional farms in multivariate space. Although the bacterial community structure in the organic farms (farms B and S) are somewhat differentiated, they are jointly less similar to conventional farms (farms J and T) (Figure 2A); the community structural pattern is also supported by the hierarchical clustering (Figure 2B). Moreover, the bacterial community composition was significantly different between the farms (PERMANOVA, p = 0.001) and across organic and conventional farms (PERMANOVA, p = 0.009), contributing about 39.01 and 11.74% of the variations in the models and a PERMDISP of 0.073 and 0.269, respectively. Significant variation was observed between farms B and T, J and T, and S and T. However, the plant species had no significant (PERMAOVA, p = 0.568) effect on the bacterial community structure, which was not clearly differentiated (Supplementary Figure S4). The non-significant and low dispersion rate in the bacterial community across the farms (Supplementary Figure S5A) suggests the results depicted by the NMDS plot in Figure 2A are reliable. The stress plot run for the NMDS plot is below 0.2 (Supplementary Figure S5B). The effect of fertilizer type and plant species interaction on the bacterial community structure was not significant (Supplementary Table S1).
Figure 2. Bray-Curtis dissimilarity between bacterial communities. (A) Comparison of observed ASVs between organic and conventional fertilization and across farms based on non-metric multidimensional scaling (NMDS). (B) Unweighted paired group mean arithmetic (UPGMA) hierarchical cluster dendrogram. The eclipses in the NMDS plot show 95% confidence intervals (standard error) in multivariate space within the group centroids, while the dotted lines indicate the distance of each sample to its group centroid in multivariate space. The stress plot (Supplementary Figure S5B) for the NMDS showed that the original dissimilarities are well preserved (stress = 0.165047) in the reduced number of dimensions.
Across all datasets, the relatively more abundant classifiable ASVs belonged to nine phyla and 19 major genera. Across the farms, the most relatively abundant (>5%) phyla were Actinobacteria (32.3%), Proteobacteria (25.4%), Acidobacteria (8.4%), Firmicutes (7.6%), Chloroflexi (7.6%), and Planctomyces (5.7%) (Figure 3A). The relatively more abundant (> 5%) genera across the farms are Bacillus, Rubrobacter, Gemmatimonas, Solirubrobacter, RB41, Nocardioides and Bryobacter (Figure 3B). Bacillus and Nocardioides were relatively more abundant in conventional farms, with their highest abundance found in farm J, while Rubrobacter and Solirubrobacter were more abundant in organic farms, mainly in farms B and S, respectively. Across the plant species, the bacterial community composition at the phylum and genus taxa levels were not particularly different; however, at the phylum level, Chloroflexi, Planctomycetes and Gemmatimonadetes were relatively most abundant in cabbage, whereas Actinobacteria, Proteobacteria and Firmicutes were the most abundant phyla in onion (Supplementary Figure S6A) compared to other vegetables. Cabbage had the highest relative abundance of Bacillus and Rubrobacter, whereas onion had the highest relative abundance of Nocardioides, Streptomyces and Solirubrobacter (Supplementary Figure S6B).
Figure 3. Average relative abundance (>5%) of dominant phylotypes across the farms. (A) Dominant Phyla (B) Dominant Genus taxa. The phylotypes with average relative abundance below 1% and the unculturable and unclassified at the genus taxa level were expunged from the plot. The bar plots were constructed based on the average relative abundance per farm site in R studio.
Linear discriminant analysis (LDA) effect size (LEFSe) showed that a total of 205 features were discriminant across the farms (Kruskal-Wallis H rank-sum test, p < 0.05; LDA score > 2.0; Supplementary Table S2) while only 18 features were discriminant for organic farms vs. conventional farms (LDA score > 2.4; Supplementary Table S3). No taxa was discriminant across the plant species after multiplicity adjustment (Kruskal-Wallis rank-sum test; p > 0.05). The genera Rubrobacter and Microlunatus were highly discriminant in organic farms (Figure 4A), while Nocardioides, Ilumatobacter, Lysobacter, and Marmoricola, were significantly discriminant in conventional farms (Figure 4A). Some of the bacterial communities that were significant and differentially abundant (FDR-adjusted p < 0.05, LDA score > 2.0) at the genus taxa across the farms are shown in Figure 4B. Rubrobacter and uncultured Conexibacteraceae were more discriminant in farm B, while Pira4lineage and uncultured bacterial Clone C112 were discriminant in farm T. In farm S, Uncultured Actinomycetales, Thermoactinomyces, and Tumebacillus were found to be discriminant. The discriminant features in conventional farms, Marmoricola and Lysobacter, were mainly from farm J. Before multiplicity adjustment of the LEFSe results for plant species type, Pseudomonas, Paracoccus and Candidatus Udaeobacter were discriminant (p > 0.05, LDA score > 2.0) and abundant in cabbage, onion, and spinach, respectively (Figure 4C), while lettuce rhizosphere soil showed no discriminant feature.
Figure 4. Differentially abundant genera of the rhizosphere bacterial communities. (A) Top 6 statistically significant discriminant (FDR-adjusted p-value < 0.1, LDA score > 2.4) genera between organic and conventional farms (B) Top 9 discriminant (FDR-adjusted p-value < 0.05, LDA score > 2.0) between the farms. (C) Differentially abundant genera (FDR-adjusted p-value < 0.1, LDA score > 2.0) between the plant species. The bar plot was generated using the Linear Discriminant Analysis (LDA) Effect size (LefSe) in the R using the microeco package. Uncul., uncultured.
The KO terms and pathways predicted for all the ASVs were 6,429 and 279, respectively. After multiplicity adjustment, 17 of the predicted pathways were significant for fertilizer type factor (Kruskal-Wallis rank sum test, p < 0.05; LDA > 0.181; Supplementary Table S4), while no significant pathway was observed for the plant species factor. Across the farms, a total of 161 functions were significant (Kruskal-Wallis rank sum test, p < 0.05; LDA > 0.274) after multiplicity adjustment (Supplementary Table S5). Seventeen abundant KO terms, contributing to important agroecological processes such as the synthesis or metabolism of N, P, C, S, and Fe compounds were compared across the farms (Figure 5). Predicted KO terms, including nitrogenase, acid and alkaline phosphatase, ferric chelate reductase and aminocyclopropane-1-carboxylate (ACC) deaminase, had low relative abundance in organic compared to conventional farms. Based on the Bray-Curtis distance, the predicted pathways in organic farms and conventional farms clustered separately as shown by the hierarchical cluster dendrogram plot in Figure 5.
Figure 5. Relative abundance of KEGG Orthology terms contributing to important agroecosystem functions. The hierarchical clustering dendrograms are based on the mean Bray-Curtis distance between the farms. The color range for the relative abundance is scaled across the farms. The superscripts by the enzyme commission (EC) number indicate (A); ACC, (B); N-fixation, (C); phosphorous mineralization, (D); sulfur mineralization (E); soil iron balance, (F); biocontrol, and (G); soil C breakdown. ICT, iron complex transport system.
A higher relative abundance of K01505, K02217, K07405, K01187, and K03332 was predicted in the rhizosphere of cabbage relative to other plant species. Similarly, K00368, K02585, K01183, K07406, and K02013 were higher for ASVs from spinach than in other plant species, while onion had the least abundance of predicted KOs (Supplementary Table S6). The major functional profile group of the bacterial communities at taxa rank level 1 is metabolism, followed by environmental information, and genetic and cellular processes. Using LEfSe, it was revealed there are differences in the abundances of key enriched pathways across the fixed factors (Kruskal-Wallis rank-sum test, p < 0.05, LDA > 0.2). Organic farms had more differentially abundant pathways compared to conventional farms. Amino acid, arginine, proline metabolism, C-fixation and citrate cycle pathways were discriminant in the organic farm (Supplementary Table S4), while sulfur, phosphonate and phosphinate metabolism were more abundant in the conventional farms. Farm T, followed by farm J had the highest number of important enriched pathways (Supplementary Table S5). Xenobiotic degradation and terpenoid and polyketide metabolism were differentially abundant in farm J while bacterial secretion, translation and genetic information system were more abundant in farm T (Supplementary Table S5).
Although there were no significant correlations between some of the soil enzyme activities and physicochemical parameters (Supplementary Table S7), beta-glucosidase had a significantly positive correlation with pH (r = 0.32, p = 0.001) and PO4 (r = 0.12, p = 0.045) but negative correlation with OM (r = −0.69, p = 0.015), NH4+ (r = −0.72, p = 0.005) and K (r = −0.30, p = 0.019). Both alkaline and acid phosphatase significantly (p < 0.05) correlated with TOC, positively and Cl, negatively. Moreover, alkaline phosphatase correlated positively with NO3 while acid phosphatase correlated positively with pH and EC. In addition, dehydrogenase had significant correlations that were negative with pH, PO4, NH4 and K but positive with OM and TOC. Although there exists either a positive or negative correlation between the soil enzymes and moisture content, Ca and sand, the correlations were not significant. Similarly, though not significant, there were correlations between the species function percentage of bacterial community with the soil parameters (Supplementary Figure S7).
The RDA plot showed that the soil physicochemical properties contributed 34.5% (R-squared adjusted value) of the variation in the bacterial community structure. Farms and fertilizer type-specific clustering were observed, especially for the organic farms (Figure 6). Among the physicochemical parameters in the RDA model, TOC, OM, EC, and CEC were statistically significant (ANOVA, p < 0.05) (Supplementary Table S8). Similarly, there were significant correlations between the environmental variables and the distance matrix (Mantel, p < 0.05) (Supplementary Table S8). Overall, the plot showed that soil physicochemical properties largely influenced the variations observed in the bacterial community (Figure 6). PO4, Na, and NH4 had more impact on the bacterial community structure in farm T, while moisture, TOC, sand, and pH, had more influence on bacterial community structure in the organic farms (farms B and S).
Figure 6. Redundancy analysis (RDA) triplot showing the correlation between bacterial community relative abundance (for 30 major genera) and explanatory variables (physicochemical factors). The first species axis (RDA) is significant (p < 0.05).
Some of the ASVs had significant (r = 0.70, p < 0.05) positive correlations with the environmental traits, which produced a significant (Mantel, p = 0.001) effect on the network connectivity. The significant ASVs and node connectivity were found to be significant for two major phyla: Actinobacteria (Mantel, r = 0.120, p = 0.022) and Acidobacteria (Mantel, r = 0.344, p = 0.009). From the topological metrics (Table 3), both organic and conventional farm networks were non-random as inferred from the significant power-law distribution for each network (R2organic = 0.88, p < 0.05; R2conventional = 0.84, p < 0.05) and the higher values of some of the structural features in the empirical compared to the random networks (Table 3). The empirical network consisted of 247 nodes and 704 edges (Figure 7A) for the organic farms and 284 nodes and 1,027 edges for the conventional farms (Figure 7B).
Table 3. Topological properties of empirical and random networks between bacterial communities of organic and conventional farm.
Figure 7. Co-occurrence networks of bacterial communities and classification of nodes for detection of keystone taxa within ecological networks in (A) organic and (B) conventional farm rhizosphere soil. The nodes represent bacterial species (round shape), colored according to the community modularity class. Node sizes are proportional to their degree of distribution, and nodes having less than two connections have been removed. Edges are network connections signifying significant (FDR-adjusted p < 0.01) associations between nodes. Positive associations are colored red, while negative associations are colored blue. Detected module hubs in the two farm networks are linked with Acidobacteria, Actinobacteria, Proteobacteria and Chloroflexi, while the connectors are affiliated with Actinobacteria for both organic and conventional farms. The co-occurrence network statistics are presented in Table 3.
A higher level of positive (~72%) associations occurred among the bacterial communities in the organic farms compared to conventional farms, which had a higher negative (~33%) association. Though community segmentation was apparent in both networks, the organic farms had a more profound fragmentation. Moreover, a higher network complexity was observed for organic farms compared to conventional farms, which had more numbers of smaller modules and edges than organic farms. The module hubs were not of similar parameters, with organic farms (Figure 7A) having more bacterial communities compared to conventional farms (Figure 7B). Actinobacteria, Acidobacteria, Proteobacteria and Chloroflexi were dominant in the keystone nodes. No network hub was observed for both networks. While Actinobacteria occurred as a major node that highly connects modules in both networks (Figure 7), Bacteroides, Proteobacteria, Acidobateria and Chloroflexi are other connectors in the organic farm network. The keystone nodes comprised the genera Bacillus, Nocardioides, Blastocatella, and Saccharomonsospora. A major connector in conventional farms is Agromyces while organic farms are dominated by the family Micrococcaceae, Roseiflexaceae, Blastocatellaceae, Xanthobacteraceae, and Chitinophagaceae (Figure 7).
Soil microbes significantly contribute to ecosystem functions through organic matter decomposition, nutrient cycling, and mineralization. These functions and plant ecosystems are influenced by agronomic practices such as soil nutrient management and cropping systems. Thus, this study provided insights into the rhizosphere soil bacterial community structure and functions of vegetable crops cultivated under organically and conventionally managed soil in different farms. Functional profiling and differentially abundant taxa of rhizosphere bacteria allowed for the identification of rare microbial communities with unique potentials in agroecosystems, emphasizing the benefits of understanding soil microbiome dynamics to reveal specific patterns, functions and strategies used by microbes under different agronomic conditions.
The fact that soil nutrients and structure drive plant growth and soil microbial diversity is well established (Bach et al., 2010; Xu et al., 2022). Some of the soil parameters, including pH, EC, moisture content, NH4+ and NO3− were not significant across the farms, However, except for pH and P, the measured values were relatively not within the optimal range required for the productivity of most crops, including vegetables (Rosen and Eliason, 2005; Warncke et al., 2009; Liu and Hanlon, 2018). Contrary to our results, low moisture content has been reported in chemically fertilized soils (Rachwał et al., 2021) and a higher pH in organically managed soil (Diacono and Montemurro, 2010; Aziz et al., 2017). Higher levels of organic matter increase soil water holding capacity through soil aggregation, signifying soil organic matter and moisture content are strongly correlated (Rajkai et al., 2015). Although organic farms typically have higher organic matter content and soil texture type that support high moisture content, our results showed that conventional farms had higher moisture content, possibly due to other factors such as time of sampling and irrigation system. Compared to conventional farms, soils in the organic farms largely had higher clay content, CEC and OM content; this is in agreement with the observations from other studies (Han et al., 2016; Rigane et al., 2020). Among the farms, farm S, which is organically managed, had the highest particle size (Table 1). Soil particle sizes impact soil aeration and structure, while high clay content and CEC strongly drive the fixation of key soil nutrients (Ramos et al., 2018; Kome et al., 2019). Being negatively charged, clay particles adsorb positive ions such as Ca2+, Fe3+, K+, and NH4+ which are key indicators of soil nutrient richness (Tomašić et al., 2021). In contrast, our results show that in the organic farms (Farm B and S), which had higher clay content, some of the positive ions, including NH4+, K+, and Ca2+ were relatively lower suggesting other factors, including irrigation, could have influenced the soil nutrient levels.
High activity of soil enzymes, including dehydrogenase, β-glucosidase and urease activities have been reported in organic farms (Hernandez T. et al., 2021; Pittarello et al., 2021). Extracellular enzyme production has been positively correlated with high microbial biomass and diversity in the community. Such reports are consistent with our results that showed a high activity of β-glucosidase across organic farms, validating the comparatively higher C mineralization in organic farms compared to conventional farms; compost and manure applied in organic farms are rich in carbon substrate (Zang et al., 2018; Cordero et al., 2019). Acid and alkaline phosphatase activities are optimal at pH 3.0–5.5 and 8.5–11.5, suggesting the low activities across the farms may be linked to the soil neutral pH (Neina, 2019). Soil enzyme activities dynamically respond to soil nutrient management, which influences soil parameters, particularly pH, OM, moisture content and nutrient content (Pan et al., 2013; Koishi et al., 2020). A significant correlation between soil pH and dehydrogenase, β-glucosidase and acid phosphatase suggests pH is a key factor driving enzyme activities (Pan et al., 2013). The observed positive correlation of β-glucosidase with OM indicates the specificity of the enzyme to soil C, which is a fraction of the total soil OM (Meena and Rao, 2021). Corroborating with our result of reduced enzyme activities in the conventional farm, excessive use of chemical fertilizers has been reported to hinder soil enzyme activities (Pittarello et al., 2021; Rachwał et al., 2021). Thus, the types of fertilizers used in plant cultivation affect changes in soil conditions, such as enzyme activities which may cause variations in the dynamics of the associated soil microbial community (Liu and Hanlon, 2018; Ullah et al., 2019).
Soil properties, especially organic matter drive bacterial growth and metabolism, suggesting the bacterial richness across the farms is related to soil conditions (Peltoniemi et al., 2021; Rachwał et al., 2021). This was evidenced in the RDA triplot (Figure 6), which revealed a significant correlation between the soil parameters and some bacterial communities. Soil physical and biological parameters, including OM, moisture and nutrient content, pH, dehydrogenase, β-glucosidase, and urease, support improved soil and plant health, which in turn influence the rhizosphere bacteria composition (Yan et al., 2021). Rhizosphere microbiome has some analogous traits with its associated plants, suggesting plant selective impact on rhizosphere microbes and consequently shaping rhizobacterial assemblage (Ling et al., 2022). Similar to other studies, though only the inverse Simpson diversity index was significant, the differences in alpha diversity across the farms suggest fertilizer types influence bacterial community richness (Hu et al., 2011; Acharya et al., 2021). According to Hermans et al. (2017) and Kamaa et al. (2011), bacterial communities respond to prevailing soil nutrient management types, which vary across landscapes; this is in agreement with our results which revealed differences in bacterial communities across the different farms, as depicted by the NMDS plot (Figure 2A). However, the bacterial community structure of soils from similar fertilizer types showed some differences, an indication that other site-specific factors, such as the source and type of seeds, fertilizers, and irrigation systems not considered in this study, may be responsible.
Important bacterial phyla, including Actinobacteria, Proteobacteria, Acidobacteria, Firmicutes and Chloroflexi, which were relatively abundant across the farms have been reported in similar studies (Ezeokoli et al., 2020; Zhang et al., 2022). These phyla usually exist in C-rich niches, such as the rhizosphere, which supports high metabolic activities and fast microbial growth. The variation in taxa abundance across crop species may be due to differences in root architecture and exudates, which differentially stimulate the growth of rhizosphere microbial communities (Hoch et al., 2019; Yan et al., 2021). Similarly, this may have influenced the higher ASVs abundance observed in cabbage compared to other crop species, suggesting the need to further explore factors influencing rhizobacteria diversity variability in different crop species under similar conditions. Several studies have identified core bacterial microbiomes primarily using taxonomic assignment; however, for detailed knowledge of microbial dynamics, it has become imperative to further identify soil microbes using key functions that are commonly expressed (Ling et al., 2022). For instance, Gemmatimonadetes, a recently classified phylum found among the major phyla in the cabbage soil have species that contribute to C-fixation through chlorophototrophy (Thiel et al., 2018). In addition, predominant genera, such as Bacillus, Nocardioides, Pseudomonas, Rubrobacter, and Lysobacter, which are constantly enhanced in the rhizosphere, contribute to key microbial functions and processes, such as biogeochemical cycling, organic matter decomposition, mineralization and processes that are crucial to agroecosystem sustainability (Raimi et al., 2017; Adeleke et al., 2019).
The differentially abundant Rubrobacter in organic farms is underexplored despite its ecological restoration and engineering potential, which is attributed to its ability to survive in low-nutrient soil and under intense desiccation (Baubin et al., 2021). Similarly, Lysinibacillus and Pseudomonas predominant in organic farm soils are well-known plant growth-promoting rhizobacteria involved in N-fixation, phytohormone production and P solubilization (Li et al., 2017; Vignesh et al., 2021; Shahwar et al., 2023). Candidatus Udaeobacter, a differentially abundant genus in the spinach rhizosphere, exhibits multidrug resistance and the ability to evade the harmful effects of antimicrobials (Willms et al., 2020). The unique properties of these genera suggest they are suitable indicator species for assessing the soil nutrient status. In contrast to the organic farms, conventional farms had differentially abundant Norcardioides, Lysobacter, Ilumatobacter, and Marmoricola. Nocardioides participate in bioremediation, especially organochlorine degradation such as lindane pesticide and chloroaromatics, making them a crucial candidate for soil remediation strategy (Ito et al., 2019; Singh and Singh, 2019). Lysobacter is a well-known biocontrol agent (Lin et al., 2021) while Ilumatobacter and Marmoricola participate in C and N cycling, respectively (Edgmont et al., 2012; Liu et al., 2023). Although soil microbes have been extensively classified, the high proportion of unclassified sequences in this study gave credence to the fact that the majority of soil microbiomes are yet to be fully identified and characterized (Delgado-Baquerizo, 2019; Furtak et al., 2020). However, metagenomics, a leading and relatively recent technique for effective analysis of diverse environments has advanced microbial diversity knowledge, offering valuable strategies for optimizing the cultivation of yet uncultured species (Kulski, 2016; Mendes et al., 2017).
Nitrogenase and NifT play key roles in the N-cycle, while cellulases break down cellulose and polysaccharide, and chitinase degrades chitins, contributing to C and N levels in the ecosystem (Glick, 2012). Corroborating the report by Ling et al. (2022), alpha-glucosidase and beta-fructokinase were highly predicted in organic farms, possibly due to the high level of C supplied by compost and animal manure, suggesting a more sustainable soil microbial community may be achieved through organic amendments that increased these key enzymes. Bacterial communities such as Bacillus, Enterobacter, Citrobacter, and Pseudomonas detected have been previously reported with N-fixing ability due to their nitrogenases (Gomez-Garzon et al., 2017; Li et al., 2017). On the contrary, nitrite reductase genes driving the denitrification pathway in the N-cycle were significantly abundant in Farm T, which is a conventional farm, establishing the fact that these genes play a crucial role in preventing groundwater pollution caused by nitrate compounds due to excessive chemical fertilizer application (Liu et al., 2020). Arylsulfatase and choline-sulfatase drive the sulfur content in the soil and are usually activated under sulfur-deficient conditions (Cregut et al., 2013; Sánchez-Romero and Olguin, 2015). Surprisingly, these genes were highly prevalent in conventional farms (Farm T and J) (Figure 5), suggesting other factors, including available sulfur, forms of sulfur compounds and other nutrient complexes in the soil may have influenced the prevalence of these enzymes (Siwik-Ziomek et al., 2016). In addition, the high abundance of enzyme coding genes predicted in the rhizosphere of cabbage compared to other vegetables could enhance soil nutrient richness and in turn drive increased soil microbial composition and diversity (de Bruijn, 2015; Xu et al., 2022). While variations across geographic locations drive changes in soil physicochemical parameters (Moore et al., 2022), the changes may in turn influence soil microbial and enzyme activities (Meena and Rao, 2021), suggesting the reason for some of the variations observed across the farms. Thus, it may be recommended toward best practices to always understand agronomic soil parameters for appropriate selection of efficient soil nutrient management.
Microbial co-occurrence structure greatly impacts community assembly, abundance and diversity (Pan et al., 2021). Soil nutrient management (types of fertilizer applied) and plant species have been reported to influence the network structure of soil bacterial communities (Xue et al., 2018; Xu et al., 2022). The network analysis showed that conventionally managed soil had high numbers of bacterial communities with negative links, which may indicate weak connections among microbial associations. This observation was corroborated by Huang et al. (2019), who attributed the distinctly weakened ecological interactions between soil microbes to long-term chemical fertilization. In contrast, the higher level of positive interactions in the organic farms may signify greater ecological cooperation among the microbial communities for diverse ecosystem functions and consequently; a network structure with high stability and high-order level complexity (Xue et al., 2018; Gu et al., 2019). Profound community fragmentation was noticed for organic farm networks. According to Hernandez D. J. et al. (2021), ecological stress greatly influences the complexity and stability of microbial networks, indicating fragmentation may not necessarily be due to fertilizer types. Thus, other factors such as soil types, irrigation and land preparation that impact soil microbial diversity may be influential.
In microbial networks, modules comprise species, which are interconnected with more frequent and intensive interactions than other parts of the community (Ling et al., 2022). Compared to conventional farms, the organic farms had high numbers of complex topological structures and modules, which suggests high niche differentiation in organic farms (Figure 7; Table 3). Unique microbes play key roles in the functioning of bacterial communities by contributing to the information current across the entire network (Banerjee et al., 2018). Similar to our observation, Gu et al. (2019) reported no network hubs in their study, suggesting unique phylotypes for specific functions are absent. However, some generalists, including Bacillus, Nocardioides, Agromyces, and Blastocatella were detected, signifying diverse keystone species that could drive the soil microbial communities in each of the farms (Gu et al., 2019). Module hub, network hub and connectors harbor keystone communities whose identification further improves the understanding of microbial community structure and interactions (Pan et al., 2021). In this study, Bacillus found in organic farms is a unique generalist, participating in nutrient solubilization and mineralization; therefore, can generate a niche for other plant growth-promoting rhizobacteria. On the other hand, the conventional farm network has Agromyces as a major connector, which is involved in xylan degradation; thus, may create a niche for microbial populations that cannot degrade polysaccharides (Rivas et al., 2004).
To further improve our agroecosystem management knowledge, we recommend that the impact of soil nutrient management on soil microbial diversity and functional structure be investigated across seasons. Data on crops cultivated in the previous seasons, irrigation systems and sources of fertilizers were not available, contributing to some of the limitations in this study.
This study revealed the soil bacterial community diversity and functions are influenced by farm sites and fertilizer types. Unique bacterial communities, which contribute to ecosystem functions were also differentiated across these fixed factors. To a large extent, the discovery of unique bacterial communities such as Bacillus and Rubrobacter with plant growth-promoting and niche-creation potentials across the farms could improve the understanding of soil microbial dynamics for enhancing soil and plant productivity. In addition, conventional farms had reduced keystone taxa compared to organic farms, signifying fertilizer types impact the abundance of keystone taxa, which is a key factor in ecosystem functioning. This study provided comprehensive details on rhizosphere soil bacterial diversity and functions, which could be useful in the identification of biomarker species for monitoring soil productivity in a particular nutrient management system. Keystone taxa, such as Bacillus and Agromyces could be a good source of microbial resources for bioformulation production. Overall, the findings provide a baseline for understanding how fertilizer types, organic and conventional fertilizers, impact soil bacteria community structure and related ecological functions. Such knowledge may be useful for monitoring invasive species or formulating a strategy for replenishing extinct beneficial species following land-use intensification or a particular agronomic practice.
The datasets presented in this study can be found in online repositories. The names of the repository/repositories and accession number(s) can be found below: https://www.ncbi.nlm.nih.gov/, PRJNA904574.
RA: conceptualization, methodology, validation, formal analysis, investigation, resources, writing: review and editing, project administration. AR: validation, resources, writing—original draft, review and editing, methodology, validation, formal data analysis, and investigation. OE: validation, data analysis, writing—review and editing. All authors contributed to the article and approved the submitted version.
This research was funded by the National Research Foundation (NRF) South Africa, under grant number 116251 and the Technology Innovation Agency with grant number NWU SF2210012/TIA1396/01.
The authors appreciate Dr. Deidre Van Wyk, Ms. Mariska Kleyn, Ms. Sharon Mokubedi, Mr. Abram Mahlatsi, and Mrs. Lee Chanhaka for their various supports. The authors are also thanking the farm managers and supervisors, including Mr. Gilbert, Ms. Lerato, and Mr. Malungani, for their support and cooperation during sampling. The authors acknowledge the Centre for High-Performance Computing (CHPC), South Africa, for providing computational resources to this research study (grant number 116251). The views expressed in this study are those of the authors and not of the funding partners.
The authors declare that the research was conducted in the absence of any commercial or financial relationships that could be construed as a potential conflict of interest.
All claims expressed in this article are solely those of the authors and do not necessarily represent those of their affiliated organizations, or those of the publisher, the editors and the reviewers. Any product that may be evaluated in this article, or claim that may be made by its manufacturer, is not guaranteed or endorsed by the publisher.
The Supplementary material for this article can be found online at: https://www.frontiersin.org/articles/10.3389/fmicb.2023.1229873/full#supplementary-material
Acharya, M., Ashworth, A. J., Yang, Y., Burke, J. M., Lee, A., and Acharya, R. S. (2021). Soil microbial diversity in organic and non-organic pasture systems. PeerJ 9:e11184. doi: 10.7717/peerj.11184
Adeleke, R. A., Nunthkumar, B., Roopnarain, A., and Obi, L. (2019). “Applications of plant-microbe interactions in agro-ecosystem” in Microbiome in plant health and disease. eds. V. Kumar, R. Prasad, M. Kumar, and D. K. Choudhary (Singapore: Springer Nature), 1–34. doi: 10.1007/978-981-13-8495-0_1
Ahkami, A. H., Allen, R., Iii, W., Handakumbura, P. P., and Jansson, C. (2017). Rhizosphere rhizosphere engineering: enhancing sustainable plant ecosystem productivity. Rhizosphere 3, 233–243. doi: 10.1016/j.rhisph.2017.04.012
Aziz, M. A., Hazra, F., Salma, S., and Nursyamsi, D. N. (2017). Soil chemical characteristics of organic and conventional agriculture. J. Trop. Soils 21:19. doi: 10.5400/jts.2016.v21i1.19-25
Bach, E. M., Baer, S. G., Meyer, C. K., and Six, J. (2010). Soil Biology & Biochemistry Soil texture affects soil microbial and structural recovery during grassland restoration. Soil Biol. Biochem. 42, 2182–2191. doi: 10.1016/j.soilbio.2010.08.014
Banerjee, S., Schlaeppi, K., and van der Heijden, M. G. A. (2018). Keystone taxa as drivers of microbiome structure and functioning. Nat. Rev. Microbiol. 16, 567–576. doi: 10.1038/s41579-018-0024-1
Bardgett, R. D., and Caruso, T. (2020). Soil microbial community responses to climate extremes: resistance, resilience and transitions to alternative states. Philos. Trans. R. Soc. B Biol. Sci. 375:20190112. doi: 10.1098/rstb.2019.0112
Barillot, C. D. C., Sarde, C. O., Bert, V., Tarnaud, E., and Cochet, N. (2013). A standardized method for the sampling of rhizosphere and rhizoplane soil bacteria associated to a herbaceous root system. Ann. Microbiol. 63, 471–476. doi: 10.1007/s13213-012-0491-y
Baubin, C., Farrell, A. M., Št’Ovíček, A., Ghazaryan, L., Giladi, I., and Gillor, O. (2021). The role of ecosystem engineers in shaping the diversity and function of arid soil bacterial communities. Soil 7, 611–637. doi: 10.5194/soil-7-611-2021
Blondel, V. D., Guillaume, J. L., Lambiotte, R., and Lefebvre, E. (2008). Fast unfolding of communities in large networks. J. Stat. Mech. Theory Exp. 2008:P10008. doi: 10.1088/1742-5468/2008/10/P10008
Bolger, A. M., Lohse, M., and Usadel, B. (2014). Trimmomatic: a flexible trimmer for Illumina sequence data. Bioinformatics 30, 2114–2120. doi: 10.1093/bioinformatics/btu170
Bolyen, E., Rideout, J. R., Dillon, M. R., Bokulich, N. A., Abnet, C. C., Al-Ghalith, G. A., et al. (2019). Reproducible, interactive, scalable and extensible microbiome data science using QIIME 2. Nat. Biotechnol. 37, 852–857. doi: 10.1038/s41587-019-0209-9
Bulska, E., and Wagner, B. (2016). Quantitative aspects of inductively coupled plasma mass spectrometry. Philos. Trans. R. Soc. A Math. Phys. Eng. Sci. 374:20150369. doi: 10.1098/rsta.2015.0369
Callahan, B. J., McMurdie, P. J., Rosen, M. J., Han, A. W., Johnson, A. J. A., and Holmes, S. P. (2016). DADA2: high-resolution sample inference from Illumina amplicon data. Nat. Methods 13, 581–583. doi: 10.1038/nmeth.3869
Cordero, I., Snell, H., and Bardgett, R. D. (2019). High throughput method for measuring urease activity in soil. Soil Biol. Biochem. 134, 72–77. doi: 10.1016/j.soilbio.2019.03.014
Cregut, M., Piutti, S., Slezack-deschaumes, S., and Benizri, E. (2013). Compartmentalization and regulation of arylsulfatase activities in Streptomyces sp., Microbacterium sp. and Rhodococcus sp. soil isolates in response to inorganic sulfate limitation. Microbiol. Res. 168, 12–21. doi: 10.1016/j.micres.2012.08.001
de Bruijn, F. J. (2015). “Biological Nitrogen Fixation” in Principles of Plant-Microbe Interactions: Microbes for Sustainable Agriculture. ed. B. Lugtenberg (Cham: Springer), 215–224. doi: 10.1007/978-3-319-08575-3
Delgado-Baquerizo, M. (2019). Obscure soil microbes and where to find them. ISME J. 13, 2120–2124. doi: 10.1038/s41396-019-0405-0
Diacono, M., and Montemurro, F. (2010). Long-term effects of organic amendments on soil fertility. A review. Agron. Sustain. Dev. 30, 401–422. doi: 10.1051/agro/2009040.hal-00886539
Edgmont, C. A., Herfort, L., Tebo, B. M., Delorenzo, S., Bra, S. L., and Zuber, P. (2012). Ubiquitous dissolved inorganic carbon assimilation by marine Bacteria in the Pacific northwest Coastal Ocean as determined by stable isotope probing. PLoS One 7:e46695. doi: 10.1371/journal.pone.0046695
Ezeokoli, O. T., Bezuidenhout, C. C., Maboeta, M. S., Khasa, D. P., and Adeleke, R. A. (2020). Structural and functional differentiation of bacterial communities in post-coal mining reclamation soils of South Africa: bioindicators of soil ecosystem restoration. Sci. Rep. 10, 1759–1714. doi: 10.1038/s41598-020-58576-5
Faust, K., and Raes, J. (2016). CoNet app: inference of biological association networks using Cytoscape [version 1; referees: 2 approved with reservations]. F1000Research 5, 1–17. doi: 10.12688/F1000RESEARCH.9050.1
Felipe-Lucia, M. R., Soliveres, S., Penone, C., Fischer, M., Ammer, C., Boch, S., et al. (2020). Land-use intensity alters networks between biodiversity, ecosystem functions, and services. Proc. Natl. Acad. Sci. U. S. A. 117, 28140–28149. doi: 10.1073/pnas.2016210117
Furtak, K., Grządziel, J., Gałązka, A., and Niedźwiecki, J. (2020). Prevalence of unclassified bacteria in the soil bacterial community from floodplain meadows (fluvisols) under simulated flood conditions revealed by a metataxonomic approachss. Catena 188:104448. doi: 10.1016/j.catena.2019.104448
Galili, T. (2015). Dendextend: An R package for visualizing, adjusting and comparing trees of hierarchical clustering data and text mining dendextend: An R package for visualizing, adjusting, and comparing trees of hierarchical clustering. Bioinformatics 31, 3718–3720. doi: 10.1093/bioinformatics/btv428
Garnica, S., Rosenstein, R., and Schön, M. E. (2020). Belowground fungal community diversity, composition and ecological functionality associated with winter wheat in conventional and organic agricultural systems. PeerJ 8:e9732. doi: 10.7717/peerj.9732
Ge, Y., He, J. Z., Zhu, Y. G., Zhang, J. B., Xu, Z., Zhang, L. M., et al. (2008). Differences in soil bacterial diversity: driven by contemporary disturbances or historical contingencies? ISME J. 2, 254–264. doi: 10.1038/ismej.2008.2
Glick, B. R. (2012). Plant growth-promoting Bacteria: Mechanisms and applications. Scientifica (Cairo) 2012, 1–15. doi: 10.6064/2012/963401
Gomez-Garzon, C., Hernandez-Santana, A., and Dussan, J. (2017). A genome-scale metabolic reconstruction of Lysinibacillus sphaericus unveils unexploited biotechnological potentials. PLoS One 12, e0179666–e0179621. doi: 10.1371/journal.pone.0179666
Gu, S., Hu, Q., Cheng, Y., Bai, L., Liu, Z., Xiao, W., et al. (2019). Application of organic fertilizer improves microbial community diversity and alters microbial network structure in tea (Camellia sinensis) plantation soils. Soil Tillage Res. 195:104356. doi: 10.1016/j.still.2019.104356
Guimera, R., and Amaral, L. A. N. (2005). Functional cartography of complex metabolic networks. Lett. Nat. 433, 895–900. doi: 10.1038/nature03286.1
Han, S. H., An, J. Y., Hwang, J., Kim, S., Bin,, and Park, B. B. (2016). The effects of organic manure and chemical fertilizer on the growth and nutrient concentrations of yellow poplar (Liriodendron tulipifera Lin.) in a nursery system. For. Sci. Technol. 12, 137–143. doi: 10.1080/21580103.2015.1135827
Hermans, S. M., Buckley, H. L., Case, B. S., Curran-cournane, F., and Taylor, M. (2017). Bacteria as emerging indicators of soil condition. Appl. Environ. Microbiol. 83, 1–13. doi: 10.1128/AEM.02826-16
Hernandez, D. J., David, A. S., Menges, E. S., Searcy, C. A., and Afkhami, M. E. (2021). Environmental stress destabilizes microbial networks. ISME J. 15, 1722–1734. doi: 10.1038/s41396-020-00882-x
Hernandez, T., Berlanga, J. G., Tormos, I., and Garcia, C. (2021). Organic versus inorganic fertilizers: Response of soil properties and crop yield. AIMS Geosci. 7, 415–439. doi: 10.3934/geosci.2021024
Hoch, J. M. K., Rhodes, M. E., Shek, K. L., Dinwiddie, D., Hiebert, T. C., Gill, A. S., et al. (2019). Soil microbial assemblages are linked to plant community composition and contribute to ecosystem services on urban green roofs. Front. Ecol. Evol. 7:198. doi: 10.3389/fevo.2019.00198
Hu, J., Lin, X., Wang, J., Dai, J., Chen, R., Zhang, J., et al. (2011). Microbial functional diversity, metabolic quotient, and invertase activity of a sandy loam soil as affected by long-term application of organic amendment and mineral fertilizer. J. Soils Sediments 11, 271–280. doi: 10.1007/s11368-010-0308-1
Huang, R., McGrath, S. P., Hirsch, P. R., Clark, I. M., Storkey, J., Wu, L., et al. (2019). Plant–microbe networks in soil are weakened by century-long use of inorganic fertilizers. Microb. Biotechnol. 12, 1464–1475. doi: 10.1111/1751-7915.13487
Ito, K., Takagi, K., Kataoka, R., Kiyota, H., and Iwasaki, A. (2019). Dissipation, dehalogenation, and denitration of chloroaromatic compounds by Nocardioides sp. strain PD653: Characterization of the substrate specificity. J. Pestic Sci. 44, 171–176. doi: 10.1584/jpestics.D19-024
Jaramillo, J. E. P., Mendes, R., and Raaijmakers, J. M. (2016). Impact of plant domestication on rhizosphere microbiome assembly and functions. Plant Mol. Biol. 90, 635–644. doi: 10.1007/s11103-015-0337-7
Kamaa, M., Mburu, H., Blanchart, E., Chibole, L., Chotte, J. L., Kibunja, C., et al. (2011). Effects of organic and inorganic fertilization on soil bacterial and fungal microbial diversity in the Kabete long-term trial. Kenya Biol. Fertil. Soils 47, 315–321. doi: 10.1007/s00374-011-0539-3
Kanehisa, M., Goto, S., Sato, Y., Furumichi, M., and Tanabe, M. (2012). KEGG for integration and interpretation of large-scale molecular data sets. Nucleic Acids Res. 40, D109–D114. doi: 10.1093/nar/gkr988
Klindworth, A., Pruesse, E., Schweer, T., Peplies, J., Quast, C., Horn, M., et al. (2013). Evaluation of general 16S ribosomal RNA gene PCR primers for classical and next-generation sequencing-based diversity studies. Nucleic Acids Res. 41, e1–e11. doi: 10.1093/nar/gks808
Koishi, A., Bragazza, L., Maltas, A., Guillaume, T., and Sinaj, S. (2020). Long-term effects of organic amendments on soil organic matter quantity and quality in conventional cropping systems in Switzerland. Agronomy 10:1977. doi: 10.3390/agronomy10121977
Kome, G. K., Enang, R. K., and Tabi, F. O. (2019). Influence of clay minerals on some soil fertility attributes: A review. Open J. Soil Sci. 9, 155–188. doi: 10.4236/ojss.2019.99010
Kulski, J. K. (2016). “Next-generation sequencing — An overview of the history, tools, and ‘Omic’ applications” in Next generation sequencing-advances, applications and challenges. ed. J. Buxeraud (INTECH), 1–60.
Li, H. B., Singh, R. K., Singh, P., Song, Q. Q., Xing, Y. X., Yang, L. T., et al. (2017). Genetic diversity of nitrogen-fixing and plant growth promoting Pseudomonas species isolated from sugarcane rhizosphere. Front. Microbiol. 8, 1–20. doi: 10.3389/fmicb.2017.01268
Li, M., Zhang, J., Wu, B., Zhou, Z., and Xu, Y. (2018). Identifying keystone species in the microbial community based on cross-sectional data. Curr. Gene Ther. 18, 296–306. doi: 10.2174/1566523218666181008155734
Lin, L., Xu, K., Shen, D., Chou, S. H., Gomelsky, M., and Qian, G. (2021). Antifungal weapons of Lysobacter, a mighty biocontrol agent. Environ. Microbiol. 23, 5704–5715. doi: 10.1111/1462-2920.15674
Ling, N., Wang, T., and Kuzyakov, Y. (2022). Rhizosphere bacteriome structure and functions. Nat. Commun. 13, 836–813. doi: 10.1038/s41467-022-28448-9
Liu, C., Cui, Y., Li, X., and Yao, M. (2021). Microeco: an R package for data mining in microbial community ecology. FEMS Microbiol. Ecol. 97:fiaa255. doi: 10.1093/femsec/fiaa255
Liu, G., and Hanlon, E. (2018). Soil pH range for optimum commercial vegetable production. Florida: University of Florida, Institute of Food and Agricultural Sciences.
Liu, X., Pang, L., Yue, Y., Li, H., Chatzisymeon, E., Lu, Y., et al. (2023). Insights into the shift of microbial community related to nitrogen cycle, especially N2O in vanadium-polluted soil. Environ. Pollut. 322:121253. doi: 10.1016/j.envpol.2023.121253
Liu, X., Wang, Q., Li, L., Sun, X., Lv, A., and Chen, C. (2020). Characterization of aerobic denitrification genome sequencing of Vibrio parahaemolyticus strain HA2 from recirculating mariculture system in China. Aquaculture 526:735295. doi: 10.1016/j.aquaculture.2020.735295
Lugtenberg, B. (Ed.) (2015). Principles of plant-microbe interactions: Microbes for sustainable agriculture. Cham: Springer.
Martínez-García, L. B., Korthals, G., Brussaard, L., Jørgensen, H. B., and De Deyn, G. B. (2018). Organic management and cover crop species steer soil microbial community structure and functionality along with soil organic matter properties. Agric. Ecosyst. Environ. 263, 7–17. doi: 10.1016/j.agee.2018.04.018
Macaskill, C. (2016). Agriculture in the provinces. Agri Handb. South Africa, Johannesbg. SimplyPhi Inf. Available at: https://agribook.co.za/introduction/agriculture-in-the-provinces/. (Accessed December 30, 2022).
Meena, A., and Rao, K. S. (2021). Assessment of soil microbial and enzyme activity in the rhizosphere zone under different land use/cover of a semiarid region. India. Ecol. Process. 10, 1–12. doi: 10.1186/s13717-021-00288-3
Mendes, R., Garbeva, P., and Raaijmakers, J. M. (2013). The rhizosphere microbiome: significance of plant beneficial, plant pathogenic, and human pathogenic microorganisms. FEMS Microbiol. Rev. 37, 634–663. doi: 10.1111/1574-6976.12028
Mendes, L. W., Palma, L., Braga, P., Acacio, A., De Souza, D. G., Silva, G. G. Z., et al. (2017). Using metagenomics to connect microbial community biodiversity and functions. Curr. Issues Mol. Biol. 24, 103–118. doi: 10.21775/cimb.024.103
Moore, J. A., Kimsey, M. J., Garrison-johnston, M., Shaw, T. M., Mika, P., and Poolakkal, J. (2022). Geologic soil parent material influence on Forest surface soil chemical characteristics in the inland northwest, USA. Forests 13, 1–13. doi: 10.3390/f13091363
Musyoka, M. W., Adamtey, N., Muriuki, A. W., and Cadisch, G. (2017). Effect of organic and conventional farming systems on nitrogen use efficiency of potato, maize and vegetables in the central highlands of Kenya. Eur. J. Agron. 86, 24–36. doi: 10.1016/j.eja.2017.02.005
Nan, J., Chao, L., Ma, X., Xu, D., Mo, L., Zhang, X., et al. (2020). Microbial diversity in the rhizosphere soils of three Stipa species from the eastern inner Mongolian grasslands. Glob. Ecol. Conserv. 22:e00992. doi: 10.1016/j.gecco.2020.e00992
Neina, D. (2019). The role of soil pH in plant nutrition and soil remediation. Appl. Environ. Soil Sci. 2019, 1–9. doi: 10.1155/2019/5794869
Nielsen, U. N., Wall, D. H., and Six, J. (2015). Soil biodiversity and the environment. Soil Biodiver. Environ. 40, 1–28. doi: 10.1146/annurev-environ-102014-021257
Pan, Z., Chen, Y., Zhou, M., McAllister, T. A., and Guan, L. L. (2021). Microbial interaction-driven community differences as revealed by network analysis. Comput. Struct. Biotechnol. J. 19, 6000–6008. doi: 10.1016/j.csbj.2021.10.035
Pan, C., Liu, C., Zhao, H., and Wang, Y. (2013). European journal of soil biology changes of soil physico-chemical properties and enzyme activities in relation to grassland salinization. Eur. J. Soil Biol. 55, 13–19. doi: 10.1016/j.ejsobi.2012.09.009
Peltoniemi, K., Velmala, S., Fritze, H., Lemola, R., and Pennanen, T. (2021). Long-term impacts of organic and conventional farming on the soil microbiome in boreal arable soil. Eur. J. Soil Biol. 104:103314. doi: 10.1016/j.ejsobi.2021.103314
Pittarello, M., Ferro, N. D., Chiarini, F., and Morari, F. (2021). Influence of tillage and crop rotations in organic and conventional farming systems on soil organic matter, bulk density and enzymatic activities in a short-term field experiment. Agronomy 11:724. doi: 10.3390/agronomy11040724
Quast, C., Pruesse, E., Yilmaz, P., Gerken, J., Schweer, T., Yarza, P., et al. (2013). The SILVA ribosomal RNA gene database project: Improved data processing and web-based tools. Nucleic Acids Res. 41, 590–596. doi: 10.1093/nar/gks1219
R Development Core Team (2018). A language and environment for statistical computing R Foundation for Statistical Computing, 2.
Rachwał, K., Gustaw, K., Kazimierczak, W., and Waśko, A. (2021). Is soil management system really important? Comparison of microbial community diversity and structure in soils managed under organic and conventional regimes with some view on soil properties. PLoS One 16, 1–22. doi: 10.1371/journal.pone.0256969
Raimi, A., Adeleke, R., and Roopnarain, A. (2017). Soil fertility challenges and Biofertiliser as a viable alternative for increasing smallholder farmer crop productivity in sub-Saharan Africa. Cogent Food Agric. 3, 1–26. doi: 10.1080/23311932.2017.1400933
Ramos, F. T., Dores, E. F. C., Weber, O. L. S., Beber, D. C., Campelo, J. H., and Maia, J. C. D. S. (2018). Soil organic matter doubles the cation exchange capacity of tropical soil under no-till farming in Brazil. J. Sci. Food Agric. 98, 3595–3602. doi: 10.1002/jsfa.8881
Rigane, H., Medhioub, K., Rebai, A., and Ammar, E. (2020). Effects of compost and manure application rate on the soil Physico-chemical layers properties and plant productivity. Waste Biomass Valorizat. 11, 1883–1894. doi: 10.1007/s12649-018-0543-z
Rivas, R., Trujillo, M. E., Mateos, P. F., Martínez-Molina, E., and Velázquez, E. (2004). Agromyces ulmi sp. nov., xylanolytic bacterium isolated from Ulmus nigra in Spain. Int. J. Syst. Evol. Microbiol. 54, 1987–1990. doi: 10.1099/ijs.0.63058-0
Rajkai, K., Tóth, B., Barna, G., Hernádi, H., Kocsis, M., Makó, A., et al. (2015). Particle-size and organic matter effects on structure and water retention of soils. Biol. 70, 1456–1461. doi: 10.1515/biolog-2015-0176
Rosen, C. J., and Eliason, R. (2005). Nutrient Management for Commercial Fruit & vegetable crops in Minnesota. Minnesota: University of Minnesota Extension Service.
Sánchez-Romero, J. J., and Olguin, L. F. (2015). Choline sulfatase from Ensifer (Sinorhizobium) meliloti: characterization of the unmodified enzyme. Biochem. Biophys. Rep. 3, 161–168. doi: 10.1016/j.bbrep.2015.08.002
Segata, N., Izard, J., Waldron, L., Gevers, D., Miropolsky, L., Garrett, W. S., et al. (2011). Metagenomic biomarker discovery and explanation. Genome Biol. 12:R60. doi: 10.1186/gb-2011-12-6-r60
Singh, T., and Singh, D. K. (2019). Rhizospheric Microbacterium sp. P27 showing potential of Lindane degradation and plant growth promoting traits. Curr. Microbiol. 76, 888–895. doi: 10.1007/s00284-019-01703-x
Siwik-Ziomek, A., Lemanowicz, J., and Koper, J. (2016). Arylsulphatase activity and sulphate content in relation to crop rotation and fertilization of soil. Int. Agrophysics 30, 359–367. doi: 10.1515/intag-2015-0098
Shahwar, D., Mushtaq, Z., Mushtaq, H., Alqarawi, A. A., Park, Y., Alshahrani, T. S., et al. (2023). Role of microbial inoculants as bio fertilizers for improving crop productivity: A review. Heliyon 9, e16134. doi: 10.1016/j.heliyon.2023.e16134
SSSSA (1990). Handbook of standard soil testing methods for advisory purposes. Pretoria: Soil Science Society of South Africa.
Thiel, V., Tank, M., and Bryant, D. A. (2018). Diversity of Chlorophototrophic Bacteria revealed in the omics era. Annu. Rev. Plant Biol. 69, 21–49. doi: 10.1146/annurev-arplant-042817-040500
Tomašić, M., Žgorelec, Ţ., Jurišić, A., Kisić, I., Hernandez, T., Berlanga, J. G., et al. (2021). Cation exchange capacity of dominant soil types in the Republic of Croatia. PLoS One 16, 1–22. doi: 10.5513/JCEA01/14.3.1286
Ullah, S., Ai, C., Huang, S., Zhang, J., Jia, L., and Ma, J. (2019). The responses of extracellular enzyme activities and microbial community composition under nitrogen addition in an upland soil. PLoS One 14:e0223026. doi: 10.1371/journal.pone.0223026
van Wyk, D. A. B., Adeleke, R., Rhode, O. H. J., Bezuidenhout, C. C., and Mienie, C. (2017). Ecological guild and enzyme activities of rhizosphere soil microbial communities associated with Bt-maize cultivation under field conditions in north West Province of South Africa. J. Basic Microbiol. 57, 781–792. doi: 10.1002/jobm.201700043
Vignesh, M., Shankar, S. R. M., Mubarak Ali, D., and Hari, B. N. V. (2021). A novel Rhizospheric bacterium: Bacillus velezensis NKMV-3 as a biocontrol agent against Alternaria leaf blight in tomato. Appl. Biochem. Biotechnol. 194, 1–17. doi: 10.1007/s12010-021-03684-9
Warncke, D., Dahl, J., Jacobs, L., and Laboski, C. (2009). Nutrient Recommendations for 971 Field Crops in Michigan. Michigan State University Extension Bulletin. E2904, 1–32.
Wemheuer, F., Taylor, J. A., Daniel, R., Johnston, E., Meinicke, P., and Thomas, T. (2020). Tax4Fun2: Prediction of habitat-specific functional profiles and functional redundancy based on 16S rRNA gene sequences. Environ. Microb. 15, 1–12. doi: 10.1186/s40793-020-00358-7
Wemheuer, F., Taylor, J. A., Daniel, R., Johnston, E., Meinicke, P., Thomas, T., et al. (2018). Tax4Fun2: a R-based tool for the rapid prediction of habitat-specific functional profiles and functional redundancy based on 16S rRNA gene marker gene sequences. bio Rxiv. doi: 10.1101/490037
Willms, I. M., Rudolph, A. Y., Göschel, I., Bolz, S. H., Schneider, D., Penone, C., et al. (2020). Globally abundant “Candidatus Udaeobacter” benefits from release of antibiotics in soil and potentially performs trace gas scavenging. mSphere 5, 1–17. doi: 10.1128/msphere.00186-20
Xu, A., Li, L., Xie, J., Zhang, R., Luo, Z., Cai, L., et al. (2022). Bacterial diversity and potential functions in response to Long-term nitrogen fertilizer on the semiarid loess plateau. Microorganisms 10, 1–18. doi: 10.3390/microorganisms10081579
Xue, C., Ryan Penton, C., Zhu, C., Chen, H., Duan, Y., Peng, C., et al. (2018). Alterations in soil fungal community composition and network assemblage structure by different long-term fertilization regimes are correlated to the soil ionome. Biol. Fertil. Soils 54, 95–106. doi: 10.1007/s00374-017-1241-x
Yan, Y., Li, B., Huang, Z., Zhang, H., Wu, X., Farooq, T. H., et al. (2021). Characteristics and driving factors of rhizosphere bacterial communities of Chinese fir provenances. Forests 12:1362. doi: 10.3390/f12101362
Yang, F., Chen, Q., Zhang, Q., Long, C., Jia, W., and Cheng, X. (2021). Keystone species affect the relationship between soil microbial diversity and ecosystem function under land use change in subtropical China. Funct. Ecol. 35, 1159–1170. doi: 10.1111/1365-2435.13769
Zang, X., Liu, M., Fan, Y., Xu, J., Xu, X., and Li, H. (2018). The structural and functional contributions of β-glucosidase-producing microbial communities to cellulose degradation in composting. Biotechnol. Biofuels 11, 51–13. doi: 10.1186/s13068-018-1045-8
Keywords: fertilizers, microbial networks, agroecosystem, soil-plant-microbe interactions, 16S rRNA gene sequencing, plant-soil ecology
Citation: Raimi AR, Ezeokoli OT and Adeleke RA (2023) Soil nutrient management influences diversity, community association and functional structure of rhizosphere bacteriome under vegetable crop production. Front. Microbiol. 14:1229873. doi: 10.3389/fmicb.2023.1229873
Received: 27 May 2023; Accepted: 01 September 2023;
Published: 28 September 2023.
Edited by:
Maqshoof Ahmad, The Islamia University of Bahawalpur, PakistanReviewed by:
Decai Jin, Chinese Academy of Sciences (CAS), ChinaCopyright © 2023 Raimi, Ezeokoli and Adeleke. This is an open-access article distributed under the terms of the Creative Commons Attribution License (CC BY). The use, distribution or reproduction in other forums is permitted, provided the original author(s) and the copyright owner(s) are credited and that the original publication in this journal is cited, in accordance with accepted academic practice. No use, distribution or reproduction is permitted which does not comply with these terms.
*Correspondence: Rasheed A. Adeleke, cmFzaGVlZC5hZGVsZWtlQG53dS5hYy56YQ==
†ORCID: Adekunle R. Raimi, https://orcid.org/0000-0001-6437-6419
Obinna T. Ezeokoli, https://orcid.org/0000-0003-1819-8804
Rasheed A. Adeleke, https://orcid.org/0000-0002-8974-422X
Disclaimer: All claims expressed in this article are solely those of the authors and do not necessarily represent those of their affiliated organizations, or those of the publisher, the editors and the reviewers. Any product that may be evaluated in this article or claim that may be made by its manufacturer is not guaranteed or endorsed by the publisher.
Research integrity at Frontiers
Learn more about the work of our research integrity team to safeguard the quality of each article we publish.