- 1Unité Molécules de Communication et Adaptation des Microorganismes (MCAM), Muséum National d’Histoire Naturelle (MNHN), Centre National de la Recherche Scientifique (CNRS), CP 54, Paris, France
- 2CNRS, Laboratoire de Biodiversité et Biotechnologies Microbiennes (LBBM), USR3579, Observatoire Océanologique de Banyuls, Sorbonne Université, Banyuls-sur-Mer, France
- 3Muséum National d’Histoire Naturelle, UMR BOREA 7208 MNHN-Sorbonne Université-CNRS-UCN-UA-IRD, Station Marine de Concarneau, Paris, France
- 4CNRS, Observatoire Océanologique de Banyuls, Sorbonne Université, FR3724, Banyuls-sur-Mer, France
Brown macroalgae are an essential component of temperate coastal ecosystems and a growing economic sector. They harbor diverse microbial communities that regulate algal development and health. This algal holobiont is dynamic and achieves equilibrium via a complex network of microbial and host interactions. We now report that bacterial and fungal endophytes associated with four brown algae (Ascophyllum nodosum, Pelvetia canaliculata, Laminaria digitata, and Saccharina latissima) produce metabolites that interfere with bacterial autoinducer-2 quorum sensing, a signaling system implicated in virulence and host colonization. Additionally, we performed co-culture experiments combined to a metabolomic approach and demonstrated that microbial interactions influence production of metabolites, including metabolites involved in quorum sensing. Collectively, the data highlight autoinducer-2 quorum sensing as a key metabolite in the complex network of interactions within the algal holobiont.
Introduction
Large marine brown algae, i.e., kelp, are an essential component of temperate coastal ecosystems. Indeed, these organisms are important primary producers that generate a specific habitat and thereby shape coastal marine life (Egan et al., 2013). In addition, kelp farming has been a growing economic sector over the last decades (FAO, 2018).
Like most eukaryotes, macroalgae are colonized by various microorganisms (the microbiota) that interact with them throughout the life cycle, and that modify their physiology (Wahl et al., 2012; Egan et al., 2013; Singh and Reddy, 2014). For example, commensal bacteria have profound effects on seaweed development, nutrition, and defense (Wahl et al., 2012; Singh and Reddy, 2014; Tapia et al., 2016). Algal tissues are also asymptomatically colonized by filamentous fungi (Debbab et al., 2012), although these fungi and their role are yet to be fully characterized (Fries, 1979; Zuccaro et al., 2003, 2008; Loque et al., 2010; Jones et al., 2012). Previously, we isolated and characterized the molecular diversity of cultivable fungi in different parts of the brown algae Ascophyllum nodosum, Pelvetia canaliculata, Laminaria digitata, and Saccharina latissima. We also found that metabolites produced by endophytic fungi are key mediators of interactions among macroalgae, their fungal microbiota, and protistan pathogens (Vallet et al., 2018).
Endophytic bacteria are likely to interact with fungi in the algal host to maintain the host-microbiota equilibrium and thus contribute to host health (Deveau et al., 2018; Hassani et al., 2018). Indeed, structural changes in the microbiota, i.e., dysbiosis, have been linked to disease in marine organisms and seaweeds (Fernandes et al., 2012; Zozaya-Valdes et al., 2015; Egan and Gardiner, 2016). However, the mechanisms underlying bacterial–fungal homeostasis remain unclear, although they appear crucial to macroalgal physiology not only in nature but also in farms, highlighting their importance in light of intensifying algal culture (Gachon et al., 2010).
Bacterial–fungal interactions consist of multiple and concomitant mechanisms ranging from nutrient competition to antibiosis, many of which depend on chemical signaling. Quorum sensing, which allows bacteria to coordinate gene expression based on the density of specific signaling molecules, is of particular interest, since it is essential for virulence, colonization, biofilm formation, and toxin production (Atkinson and Williams, 2009). Indeed, various types of quorum signals, also known as auto-inducers (AI), are already known. Some have been found in only one genus whereas others, such as type 1 (AI-1) or type 2 (AI-2), are present in various bacterial genera. Indeed, AI-2 molecules appear widespread among prokaryotes, and is produced by over 50% of sequenced bacterial species, including both Gram-positive and Gram-negative species (Hammer and Bassler, 2003; Federle, 2009). AI-2 molecules are derived from a common precursor, (S)-4,5-dihydroxypentane-2,3-dione (DPD), which is synthesized by the enzyme LuxS. By spontaneous cyclization, this precursor is transformed to 4-hydroxy-5-methyl-3(2H)furanone, (2R,4S)-2-methyl-2,3,3,4-tetrahydroxytetrahydrofuran, and, especially in marine environments, to the furanosyl borate diester (Hardie and Heurlier, 2008).
Various marine bacteria were found to produce AI-2 (Bodor et al., 2008; Doberva et al., 2015; Pérez-Rodríguez et al., 2015), although it is considered in some species to be a metabolic by product and not a signaling molecule (Rezzonico and Duffy, 2008). Nevertheless, many studies showed that AI-2 regulates niche-specific behaviors in commensal and pathogenic bacteria, including biofilm formation or dispersion, cell division, virulence, bioluminescence, and motility (Hammer and Bassler, 2003; Hardie and Heurlier, 2008; Federle, 2009). Accordingly, secreted AI-2 is now recognized as a key signaling molecule that affects bacterial behavior at species and community level (Whiteley et al., 2017).
Strikingly, quorum sensing molecules are not exclusively produced by bacteria. Indeed, these compounds were also shown to regulate fungal morphogenesis, germination, apoptosis, biofilm development, or pathogenicity (Wongsuk et al., 2016). Importantly, some recent studies showed that both bacterial and fungal quorum sensing compounds mediate cross-kingdom signaling. For instance, eukaryotes may interfere with bacterial quorum sensing (Martín-Rodríguez et al., 2014; Ismail et al., 2016), while bacteria may react to fungal quorum signals (Cugini et al., 2007; Fourie et al., 2016). Cross-kingdom signaling is also modulated by quorum sensing inhibitors, i.e., quorum quenchers (Grandclément et al., 2016; Rolland et al., 2016). To date, halogenated furanones synthesized by the marine red algae Delisea pulchra are the best-studied naturally occurring quorum sensing inhibitors in eukaryotes. These compounds regulate bacterial colonization of algal surfaces by interfering with AI-1 and AI-2 (Defoirdt et al., 2007; Harder et al., 2012).
Despite these breakthroughs, the role of AI-2 quorum sensing in marine environments in general, and in holobionts in particular, remains poorly characterized (Doberva et al., 2015; Hmelo, 2017). One important shortcoming is the lack of quantitative measurements, since AI-2 itself is difficult to quantify (Wang et al., 2018). In this study, we hypothesized that AI-2 quorum sensing is involved in interspecies chemical signaling among endophytic fungi and bacteria in seaweeds. Accordingly, we first isolated and molecularly characterized the cultivable bacteria associated with the brown algae A. nodosum, P. canaliculata, L. digitata, and S. latissima. These bacteria were then investigated for their ability to produce or inhibit production of AI-2 along with fungi previously isolated from the same samples (Vallet et al., 2018). Co-cultures experiments, between several fungal and bacterial endophytic strains isolated from S. latissima microbiota combined with metabolomics approach pointed out that inter-species interactions involve metabolites production that modulates AI-2 production. Altogether these results suggest that dynamic interactions driven by microbial metabolites may occur within the microbiota and impact AI-2 QS signaling.
Materials and Methods
Sampling and Endophyte Isolation
Fungi and bacteria were previously isolated from the brown algae L. digitata, S. latissima, A. nodosum, and P. canaliculata. L. digitata, and A. nodosum were collected in triplicate in Roscoff, France, in January 2013. Samples of all four species were also collected in triplicate in Oban, Scotland, in July 2013. Algae were surface-sterilized with 70% ethanol and 0.1% sodium hypochlorite, and cut into small pieces. Around 4,600 of these pieces were then aseptically transferred to different solid media, using at least 10 replicates from each algal part on each type of medium (Supplementary Table S1). Resulting cultures were then grown and preserved following previously described protocols (Vallet et al., 2018).
Taxonomic Identification of Endophytic Bacteria
Genomic DNA was extracted with Wizard® Genomic DNA Purification Kit (Promega, Charbonnières-les-Bains, France) from single colonies of 209 bacterial isolates that were grown in marine broth. 16S rRNA genes were then amplified using 2× KAPA2G Ready Mix (Clinisciences, Nanterre, France), 1 μL bacterial DNA, and the universal primers 27F mod (5′-AGRGTTTGATCMTGGCTCAG-3′) and 1492R mod (5′-TACGGYTACCTTGTTAYGACTT-3′). Targets were amplified over one cycle of denaturation at 94°C for 5 min, 35 cycles at 94°C for 15 s, 50°C for 15 s, and 72°C for 20 s, and final extension at 72°C for 10 min. PCR products were sequenced by Sanger sequencing on the Bio2Mar platform (Observatoire Océanologique, Banyuls-sur-Mer, France), using primer 907R (Eurofins MWG Operon, Ebersberg, Germany).
The quality of each sequence was checked manually and the closest match in NCBI databases was determined by BLAST (Altschul et al., 1990). Further, sequences were aligned in Muscle, as implemented in MEGA 7.0 (Edgar, 2004; Kumar et al., 2016). Alignments were reviewed manually to verify mismatches, and a phylogenetic tree was constructed by maximum likelihood using the K2, G+I model. The reliability of each node in the tree was assessed by bootstrapping over 500 replicates.
Screening for the Production of Quorum Sensing Mediators
The QS bioluminescent reporter strain Vibrio campbellii MM32 (luxN::Cm, luxS::Tn5Kan) was used to detect AI-2 in bacterial and fungal extracts, as previously described (Miller et al., 2004). The receptor luxN is mutated in this strain to abolish sensing of acyl homoserine lactones, while the synthase gene luxS is mutated to abolish AI-2 production but not sensing. It was previously constructed by introducing luxS::Tn5Kan onto the chromosome of strain JAF305 (luxN::Cm) (Bassler et al., 1993; Freeman and Bassler, 1999).
To obtain bacterial supernatants, 1 mL was collected from each of 209 bacterial cultures grown for 24 h at 22°C in marine broth. Samples were then centrifuged at 17,000 × g for 10 min, and resulting supernatants were filtered at 0.22 μm. To obtain fungal extracts, 43 fungal isolates were grown for 3 weeks at 19°C in MEA/ASW medium, and extracted three times with ethyl acetate. A detailed recipe of the medium is provided in Supplementary Table S1. Extracts were tested at a final concentration of 250 μg/mL, with final concentration of DMSO 2.5%.
Bacterial supernatants and fungal extracts were tested for the production of molecules interfering with AI-2 quorum sensing. Briefly, 20 μL of test samples and corresponding controls were mixed with 180 μL of V. campbellii MM32 diluted 1:5,000 and incubated at 30°C and 100 rpm. Luminescence and cell density (OD620) were measured after 24 h. Data were collected in triplicate, and luminescence change was calculated as (lumiSN/E – lumiControl)/lumiControl, where lumiSN/E is bioluminescence (normalized to cell density) from the reporter strain in the presence of supernatant or extract, and lumiControl is bioluminescence (normalized to cell density) in the presence of either marine broth (when supernatants are tested) or DMSO (when extracts are tested).
Quantification of AI-2 Precursor by LC–MS/MS
Due to low ionization potential and instability, AI-2 and DPD are not directly detectable by mass spectrometry (MS). However, quinoxaline derivatives of DPD, obtained by reaction with 4,5-dimethyl-1,2-phenylenediamine, are detectable by LC–MS/MS. DPD was thus quantified in bacterial supernatants after performing a derivatization reaction as described (Xu et al., 2017). Briefly, triplicate DPD standard solutions with concentration 2.6 nM–26 μM were obtained by diluting a stock solution of DPD (16.64 nM) in marine broth. To obtain quinoxaline derivatives, 250 μL of standard solution or supernatant was reacted with 250 μL of 0.1 mg/mL 4,5-dimethyl-1,2-phenylenediamine (Sigma, St. Louis, MO, United States) in 0.1M HCl. Samples were thoroughly mixed for 1 min, and incubated for 5 h at 25°C with agitation. Samples were then desalted with two volumes of water using Sep-Pak C18 SPE cartridges (Waters, Beverly, MA, United States), and eluted with two volumes of acetonitrile. Subsequently, samples were analyzed by LC–MS/MS on a Dionex Ultimate 3000 HPLC system coupled to a Q ExactiveTM Focus mass spectrometer (Thermo Fisher Scientific, Waltham, MA, United States) and fitted with an electrospray ionization source and a Hypersil GOLD C18 column (2.1 mm × 150 mm, 1.9 μm particle size; Thermo Scientific, Waltham, MA, United States) operating at 20°C. In this system, eluates are introduced directly into the mass spectrometer. LC–MS parameters are detailed in Supplementary Table S1. Data were collected using Xcalibur, in parallel reaction monitoring mode targeting the precursor ion at m/z 233.1285. The product ion at m/z 186.1140 was used for quantification.
AI-2 Antagonist Activity in Fungal Extracts
To test AI-2 antagonist activity in fungal extracts, 20 μL samples were reacted as described with 180 μL of V. campbellii MM32 diluted 1:5,000 in marine broth and supplemented with 2 μM DPD (purchased from Rita Ventura’s research group at ITQB, Oeiras, Portugal). To confirm that loss of luminescence, if any, was not due to cytotoxicity, 100 μL of culture was reacted with 30 μL of 0.01% resazurin (Graça et al., 2013) immediately after measurement of luminescence, and fluorescence (λex: 530 nm, λem: 590 nm) was measured after incubating for 4 h at 30°C with agitation. As control, a 96-well plate containing 20 μL of fungal extract in marine broth was assayed in the same manner to assess background luminescence, fluorescence, and absorbance.
Co-culture Experiment: Culture Conditions and Impact on Quorum Sensing
Fungal and bacterial isolates were co-cultured in triplicate in marine broth supplemented with 10% malt extract, 4% glucose, and 1.5% agar, adjusted to pH 7, and plated. Plates were inoculated with 2 mL of a mixture of 2 × 104 fungal spores and bacteria diluted to OD 0.1. Corresponding monocultures were prepared in triplicate in the same manner. Cultures were then incubated for 21 days at 19°C and on a 12-h light/dark cycle. Petri dishes containing only culture medium (n = 3) were used as blank. Cultures and corresponding controls were extracted with ethyl acetate for 30 min, in a sonicator at room temperature. Samples were then filtered through a filter paper, and dried under vacuum using a centrifugal evaporator. Extracts were tested for their impact on quorum sensing at a final concentration of 250 μg/mL, with final concentration of DMSO 2.5%. Briefly, 20 μL of extract were mixed with 180 μL of V. campbellii MM32 diluted 1:5,000 and incubated at 30°C and 100 rpm. Luminescence and cell density (OD620) were measured after 24 h. Data were collected in triplicate, and luminescence change was calculated as (lumiE – lumiControl)/lumiControl, where lumiE is bioluminescence (normalized to cell density) from the reporter strain in the presence of extract, and lumiControl is bioluminescence (normalized to cell density) in the presence of DMSO. To assess the viability of the biosensor, 100 μL of culture was reacted with 30 μL of 0.01% resazurin (Graça et al., 2013) immediately after measurement of luminescence, and fluorescence (λex: 530 nm, λem: 590 nm) was measured after incubating for 4 h at 30°C with agitation.
Co-culture Experiment: LC–MS-Based Metabolomic Analysis
Dried extracts were solubilized in methanol at 0.5 mg/mL, and analyzed by HPLC–MS in one batch and in a random sequence. Samples were loaded onto a Dionex Ultimate 3000 HPLC system fitted with a C18 AcclaimTM RSLC PolarAdvantage II column (2.1 mm × 100 mm, 2.2 μm pore size; Thermo Scientific, Waltham, MA, United States) operating at 40°C, and coupled to a Maxis IITM QTOF mass spectrometer (Bruker, Bellerica, MA, United States) with an electrospray ionization source. Data were acquired with Data Analysis software. LC–MS parameters are listed in Supplementary Table S1. Raw LC–MS data were calibrated and converted to netCDF format using Data Analysis software (Bruker), and processed using the R package XCMS (Smith et al., 2006). Based on analytical conditions and raw data characteristics, final peak picking parameters were method = ‘centWave,’ ppm = 10, and peak width = c(5,20), while final grouping parameters were bw = 5, mzwid = 0.015, and retention time correction method = ‘obiwarp.’ Other parameters were set to default values. To limit noise from compounds already present in culture media, the dataset was filtered with an in-house script to retain only those features with intensity in at least one sample more than fivefold its average intensity in blank samples.
Statistical Procedures
All analyses and graphs were performed using the R statistical framework (R Core Team, 2019). Van der Waerden tests followed by a post hoc test using the Fischer’s Least Significant Difference (LSD) criterion was performed to test the contrasting effect of mono and co-culture on QS activity. Multivariate analyses were done using the R library mixOmics (Rohart et al., 2017).
Results
Diversity of Cultivable Endophytic Bacteria From Brown Algae
A total of 209 bacterial isolates was obtained, and classified based according to 16S rRNA genes into 4 phyla, 12 orders, 19 families, 27 genera, and 88 taxonomically unique units (Figures 1, 2 and Supplementary Table S2). The most abundant phyla in L. digitata and S. latissima were Firmicutes (comprising 47 and 35% of all isolates, respectively) and Proteobacteria (39 and 53%). In P. canaliculata, Proteobacteria, and Actinobacteria were predominant (44 and 38%), whereas Firmicutes accounted for 77% of bacteria isolated from A. nodosum. Gammaproteobacteria was between 50 and 100% of all Proteobacteria depending on algal species, although Alphaproteobacteria was occasionally present. On the other hand, Bacillus and Pseudoalteromonas were the most abundant genera in S. latissima (33 and 30%) and L. digitata (47 and 22%). Isolates from P. canaliculata were mostly Pseudoalteromonas, Rhodococcus, and Bacillus (19, 16, and 13%), whereas Bacillus was dominant in A. nodosum (63%). Rhodococcus, Bacillus, Cobetia, and Pseudoalteromonas were isolated from all four algal species.
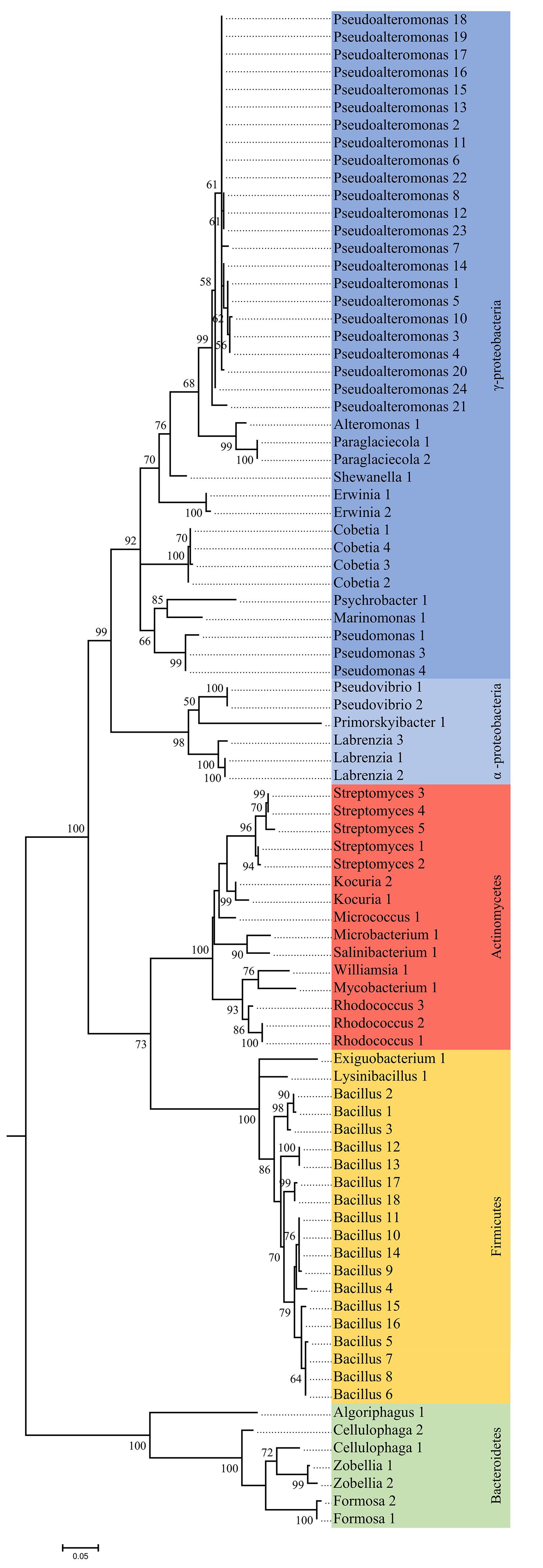
Figure 1. Phylogenetic tree of unique 16S rRNA sequences from bacteria isolated from four algal species. The tree was constructed by maximum likelihood using the K2, G+I model. The reliability of each node was assessed by bootstrapping over 500 replicates.
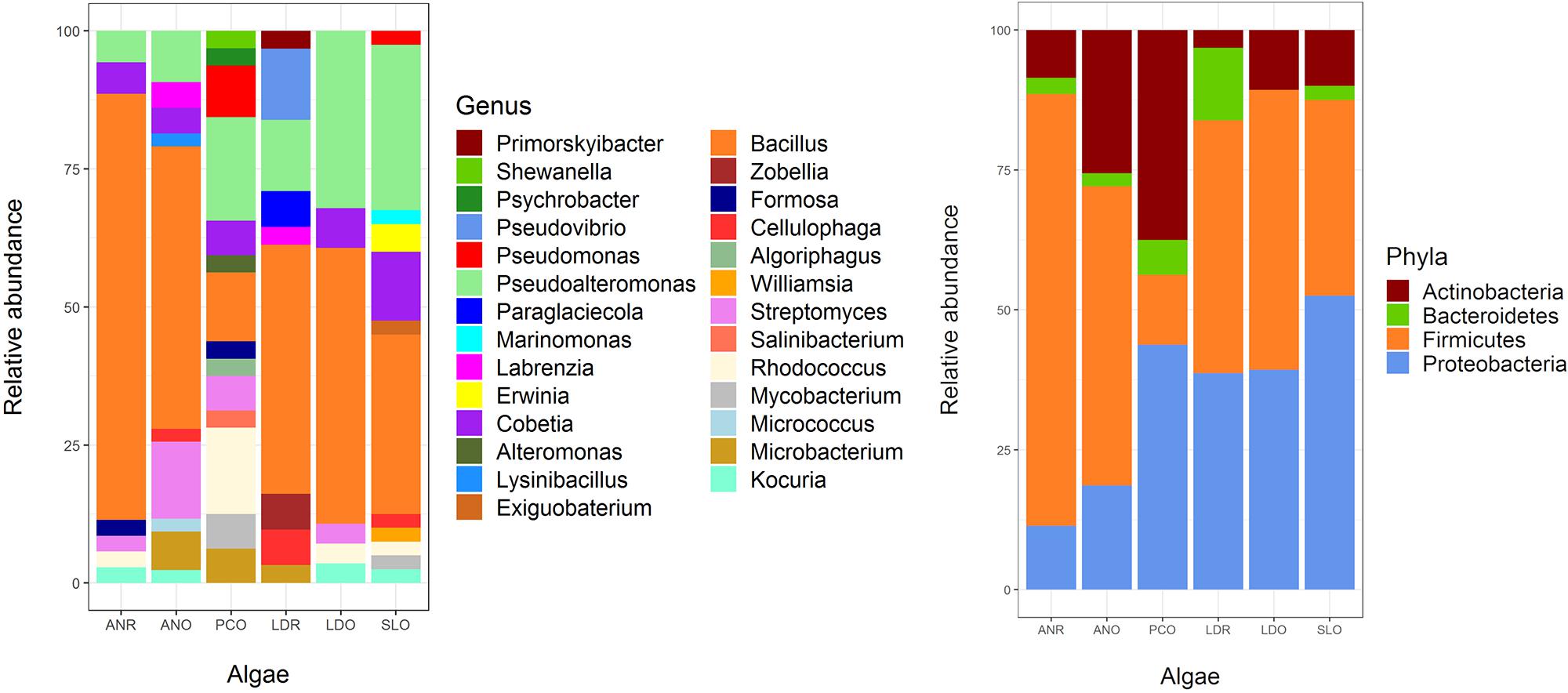
Figure 2. Abundance of cultivable bacterial genus (left) and phyla (right) by algal host and sampling site. ANR, Ascophylum nodosum from Roscoff; ANO, A. nodosum from Oban; PCO, Pelvetia canaliculata from Oban; LDR, Laminaria digitata from Roscoff; LDO, L. digitata from Oban; SLO, Saccharina latissima from Oban.
Production of AI-2 Compounds by Bacterial Endophytes
Most (86%) bacterial endophytes isolated from brown algae elicited an increase in luminescence from V. campbellii MM32, a quorum sensing reporter strain (Table 1 and Supplementary Data S1). Strikingly, 10% of isolates boosted luminescence by over 50%, including a Kocuria isolate, six Bacillus isolates, and 13 Proteobacteria. Indeed, a Marinomonas isolate and five Cobetia isolates increased luminescence by at least 100%. In contrast, all but one Pseudoalteromonas isolate increased luminescence by less than 50%.
To confirm these results, DPD production by Marinomonas 424 and Cobetia 352b, two of the strongest inducers of luminescence, was quantified by LC–MS/MS. For comparison, DPD production was also quantified in Pseudoalteromonas 352a, which was co-isolated with Cobetia 352b and is a weak inducer of luminescence. Interestingly, culture supernatants from Marinomonas 424 and Cobetia 352b contained similar levels of DPD (692 and 585 nM, respectively), whereas a lower amount was found in the supernatant from Pseudoalteromonas 352a (66 nM). Thus, our results based on LC–MS/MS confirmed the quorum sensing patterns observed using the biosensor-based approach (Figure 3).
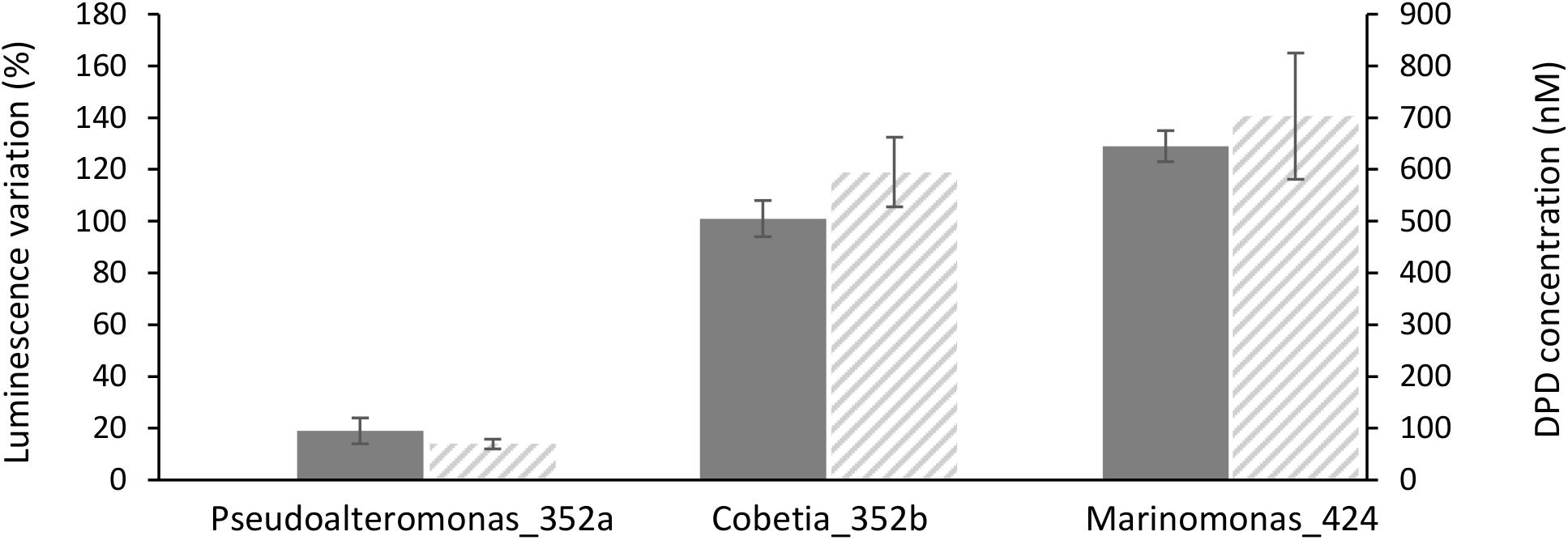
Figure 3. Induction of luminescence in the biosensor Vibrio campbellii MM32 by (gray bars), and DPD concentration in three bacterial supernatants (hatched bars). Luminescence induction was quantified as described in Section “Materials and Methods.” Error bars indicate standard deviation.
Impact of Metabolites of Endophytic Fungi on Quorum Sensing
As presented in Figure 4, extracts from 13 fungi boosted the luminescence from the biosensor. Of these, eight (Verticillium biguttatum AN130T, Chaetomium globosum LD13H, Microsphaeropsis olivacea LD50H, Botryotinia fuckeliana LD535H, Leptosphaeria marina SL457T, and Diaporthe eres SL473T) increased the luminescence by over 50%. Conversely, extracts from 30 fungi strongly diminished the luminescence, as shown in Figure 5. For 14 of these extracts, the loss of luminescence was due to toxicity against the biosensor V. campbellii MM32. In contrast, the other 16 extracts evidently inhibited AI-2, blocking the effects of 2 μM DPD but with very limited impact on the biosensor V. campbellii MM32 metabolism (Figure 5).
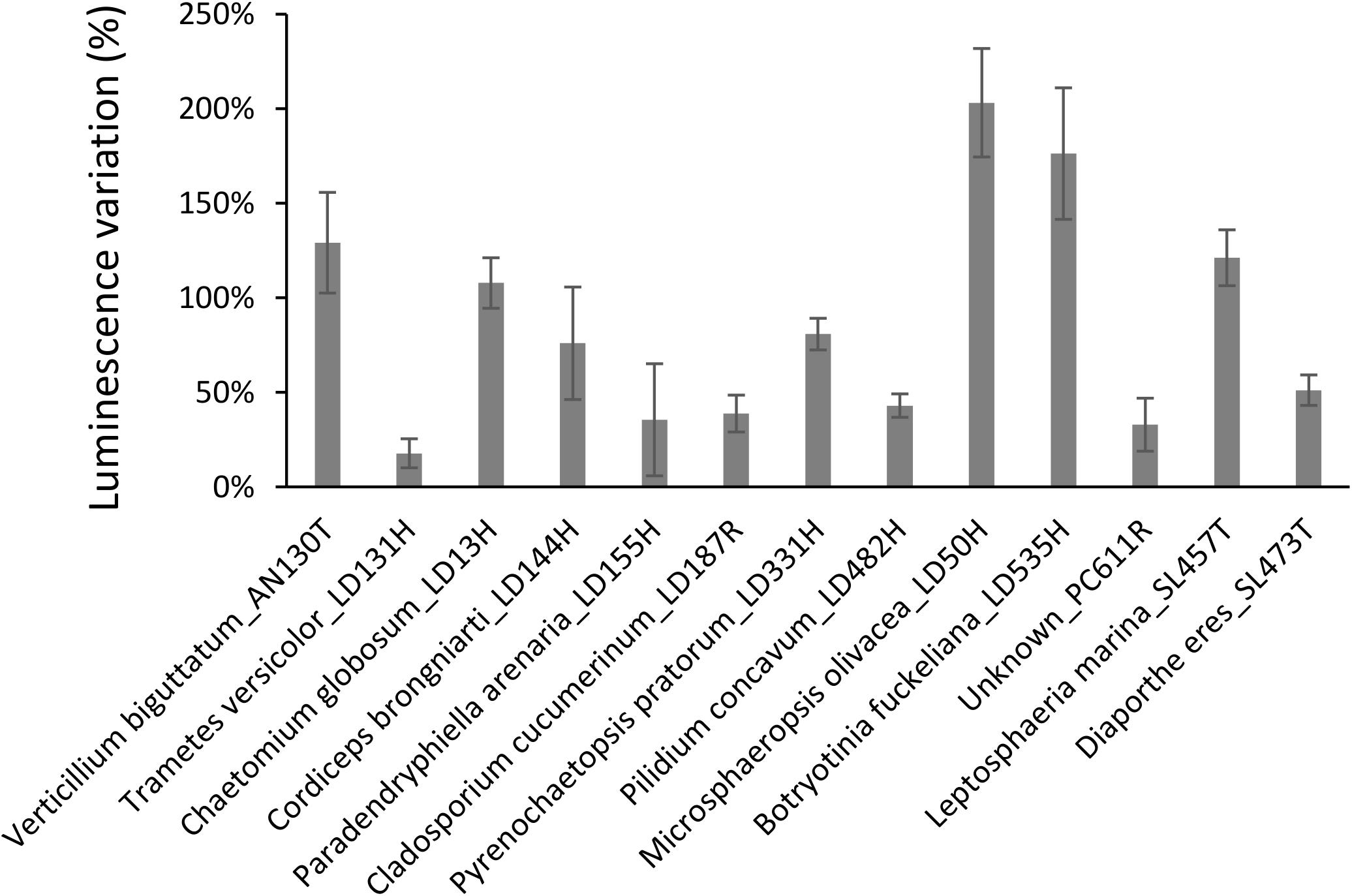
Figure 4. Induction of luminescence in the biosensor V. campbellii MM32 by fungal extracts. Error bars indicate standard deviation.
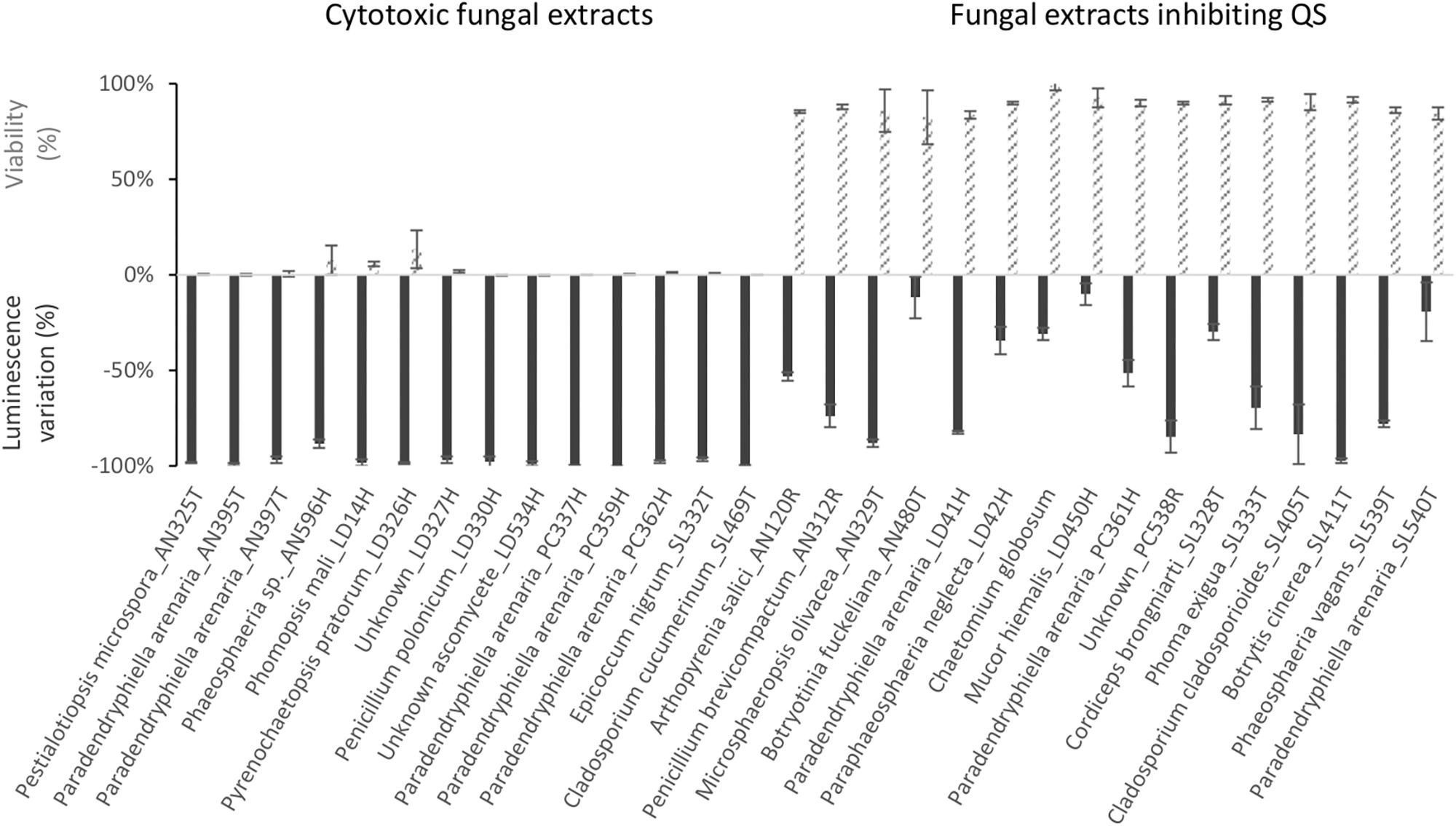
Figure 5. Impact of fungal extracts on quorum sensing in and viability of the biosensor V. campbellii MM32, as measured, respectively, by inhibition of luminescence in the presence of 2 μM DPD and by resazurin test. Assays are described in Section “Materials and Methods.” Error bars indicate standard deviation.
Impact of Bacterial–Fungal Interactions on Quorum Sensing
Metabolites produced in co-cultures of Cladosporium SL405T with Pseudoalteromonas 352a or Cobetia 352b reduced luminescence from V. campbellii MM32 by 44 and 20%, respectively. In contrast, metabolites from monocultures of Cladosporium SL405T decreased luminescence only slightly (12%), whereas metabolites from monocultures of either bacterium elicited a stronger decrease in luminescence (49%) (Supplementary Data S3). These results highlight the contrasting effects of co-cultures and monocultures on quorum sensing supported by a non-parametric test for independent samples (Van Der Waerden test), followed by a post hoc test using the Fischer’s Least Significant Difference (LSD) criterion (Figure 6). Furthermore, the partial least squares discriminant analysis of samples covering the different co-culture conditions characterized through 4,221 metabolomic features collected by LC–MS showed that each monoculture or co-culture is characterized by a specific set of features corresponding to a unique set of metabolites (Supplementary Data S2). Sparse partial least squares discriminant analysis also identified four latent variables of 180 features (720 features in total) that discriminate a culture from all others (Supplementary Data S2). In a second round of partial least squares discriminant analysis based only on these 720 features (Figure 7), the co-cultures were differentiated in the third dimension both from each other and from every monoculture. Analysis of variance of scores get on the 3rd dimension confirmed that selected features significantly separate co-cultures from each other and from monocultures (F2,12 = 167.2, p < 1.7 × 10–9). This result implies that bacterial–fungal interactions impact very significantly metabolite production in specific ways.
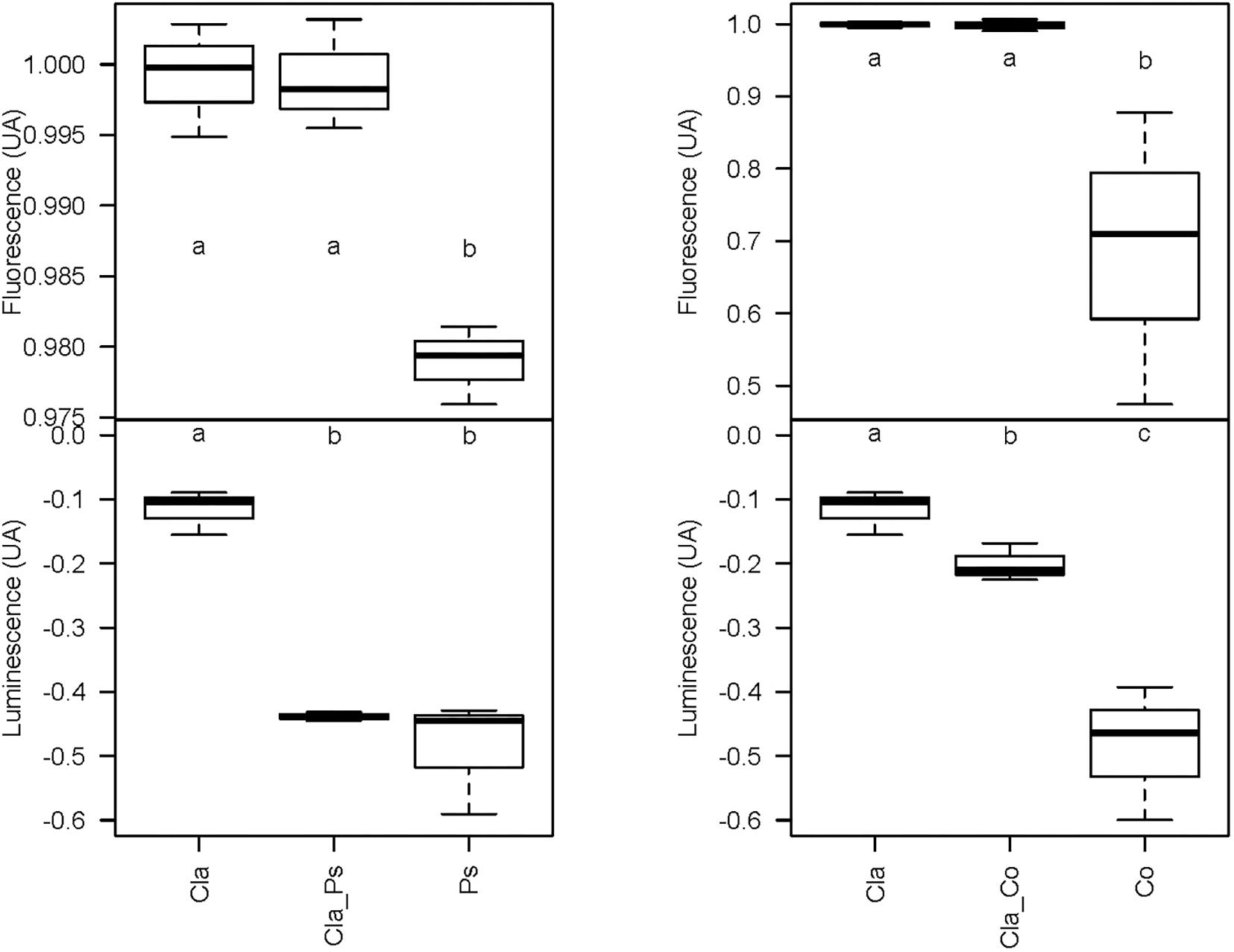
Figure 6. Box plot representing the variation of the luminescence and viability (fluorescence) of the biosensor V. campbellii MM32 by the bacterial or the fungal monoculture and the co-culture. Cla, Cladosporium monocultures; Cla_Co, Cladosporium-Cobetia co-cultures; Co, Cobetia monocultures; Cla_Ps, Cladosporium-Pseudoalteromonas co-cultures; Ps, Pseudoalteromonas monocultures. Error bars represent standard deviation for three replicates. Different letters indicate statistically significant differences between groups [mean ± SEM, N = 3, Van Der Waerden test followed by a post hoc test using the Fischer’s least significant difference (LSD), p < 0.05].
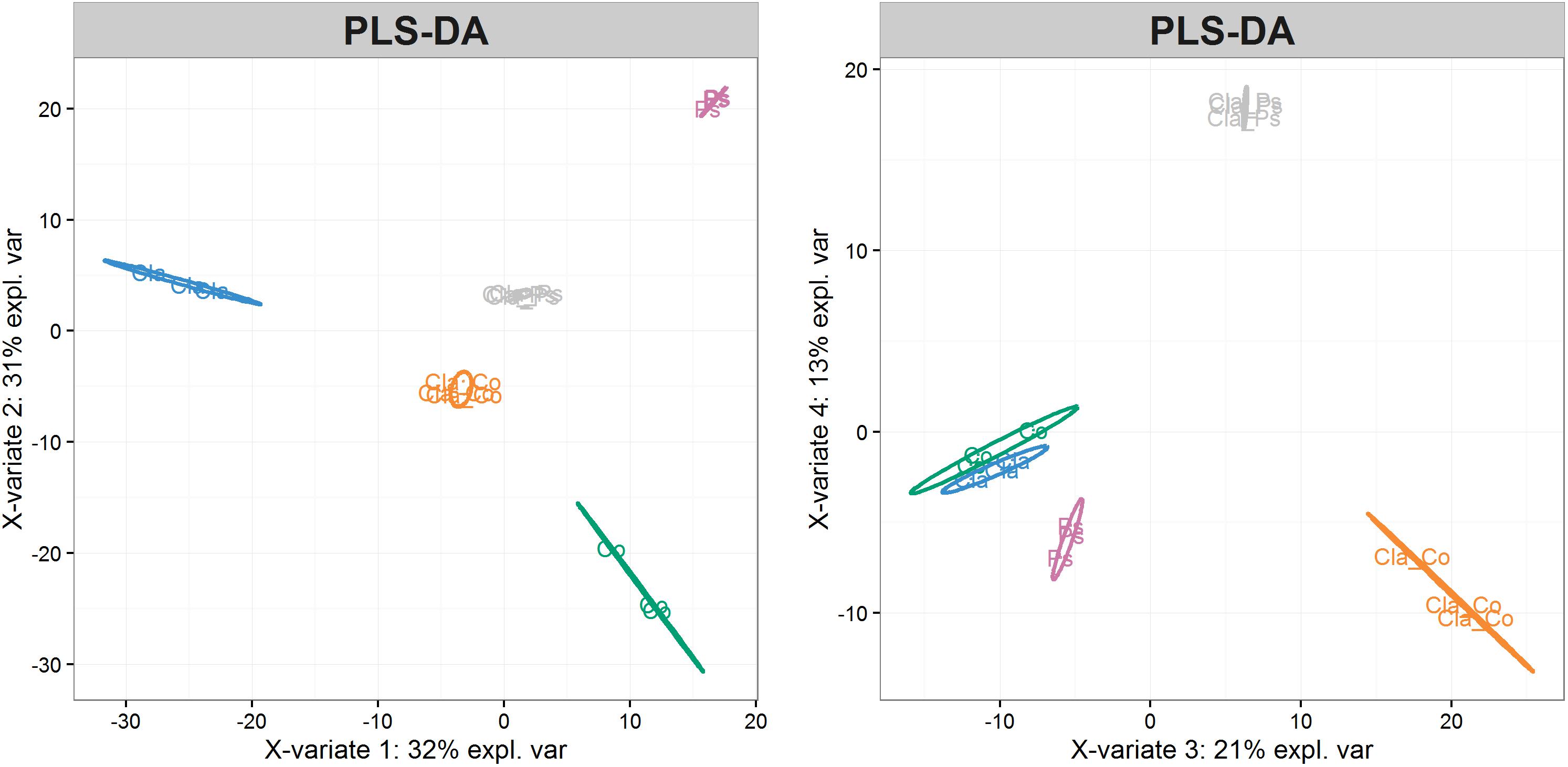
Figure 7. Visualization of samples using the first four latent variables from partial least squares discriminant analysis of 720 selected features. Ps, Pseudoalteromonas monocultures (violet); Co, Cobetia monocultures (green); Cla, Cladosporium monocultures (blue); Cla-Ps, Cladosporium-Pseudoalteromonas co-cultures (gray); Cla-Co, Cladosporium-Cobetia co-cultures (orange). Ellipses represent 95% confidence intervals.
Features that distinguish a culture and that are altered more than 10-fold over other cultures are listed in Supplementary Table S3. When compared to the corresponding monocultures, 28 and 5 of such features were identified in co-cultures of Cladosporium SL405T with Cobetia 352b and Pseudoalteromonas 352a, respectively. Conversely, 120 and 87 of such features were identified in Cobetia 352b and Pseudoalteromonas 352a monocultures when compared to the corresponding co-cultures with Cladosporium SL405T. Moreover, 93 features diminished by at least a factor of 10 in co-cultures when compared to Cladosporium SL405T monocultures. Unfortunately, top-ranked metabolites were not identified by annotation against ISDB (Allard et al., 2016), GNPS, and MassBank. Similarly, identification against SIRIUS 4.0. (Böcker and Rasche, 2008) and Pubchem was inconclusive.
Search of a Multivariate Link Between Luminescence Measurements and MS Metabolomic Variables
A link between the global response given by the luminescence representing an integrated measurement of the QS and the metabolome in the different mono or co-culture conditions was obtained thanks a PLS-based regression (Figure 8). Complementary filters such as (i) VIP above 1.20 with a VIP standard error coming from repeated cross-validation calculus lower than the VIP value for the variable, and (ii) absolute value of the correlation between primary metabolomic variables and the predicted luminescence response above 0.75, were used to select from the initial set of 4221 variables a subset of 521 variables. The comprehensive heatmap obtained (Figure 9) showcased nine and one variables displaying a significantly higher mean value for co-culture between Cla and Co and Cla and Ps, respectively (Figure 10). All these 10 significant variables are supposed to be induced in these co-culture conditions when compared to mono-culture conditions as revealed by the multiple comparison of means based on the Student-Newman-Keuls test. Indeed, these variables are of prime importance candidates that would be putatively involved in some metabolic pathways explaining the QS event.
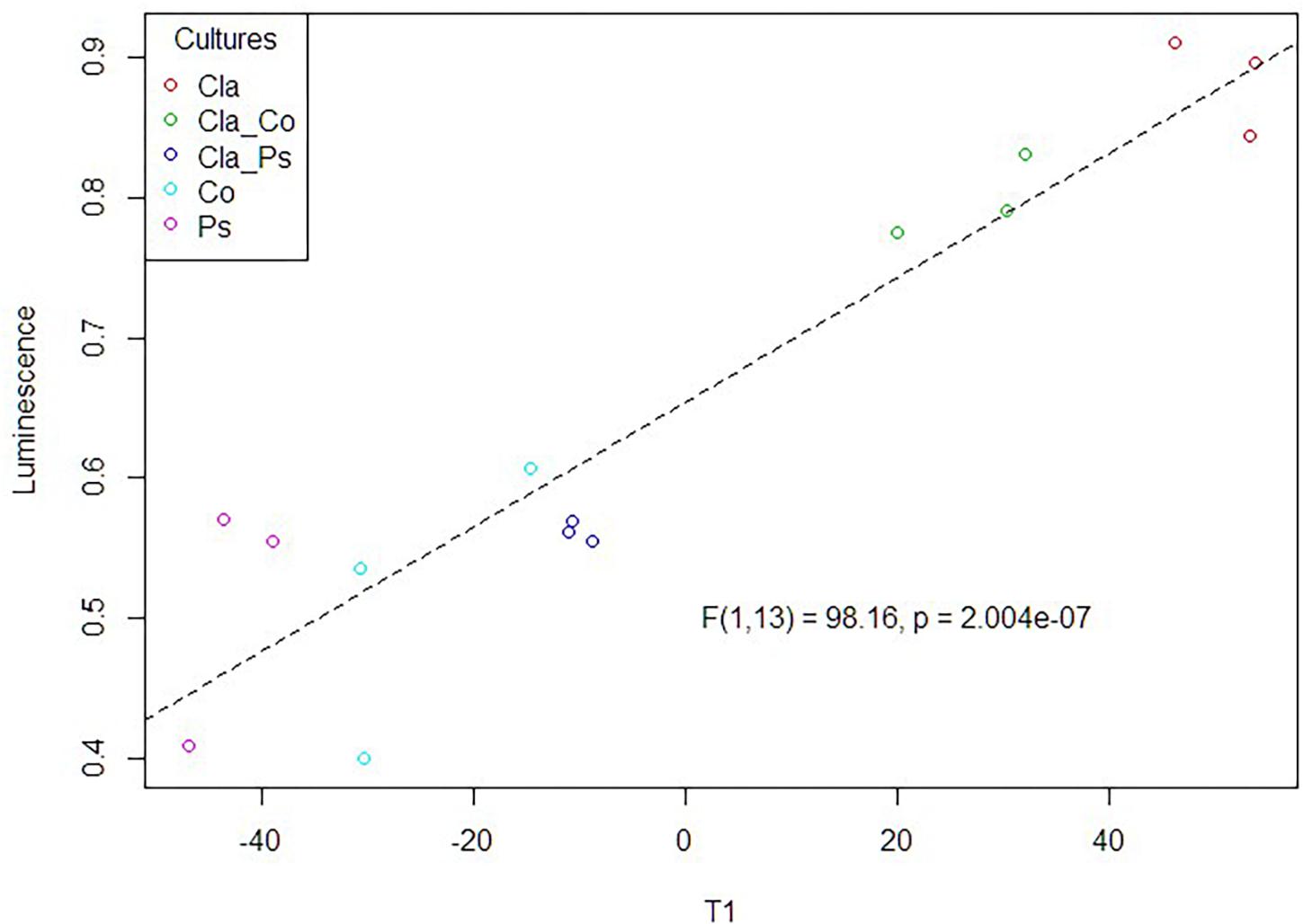
Figure 8. PLS-based regression between the luminescence measurement (QS activity) and the metabolomic profiles obtained in the different culture or co-culture conditions.
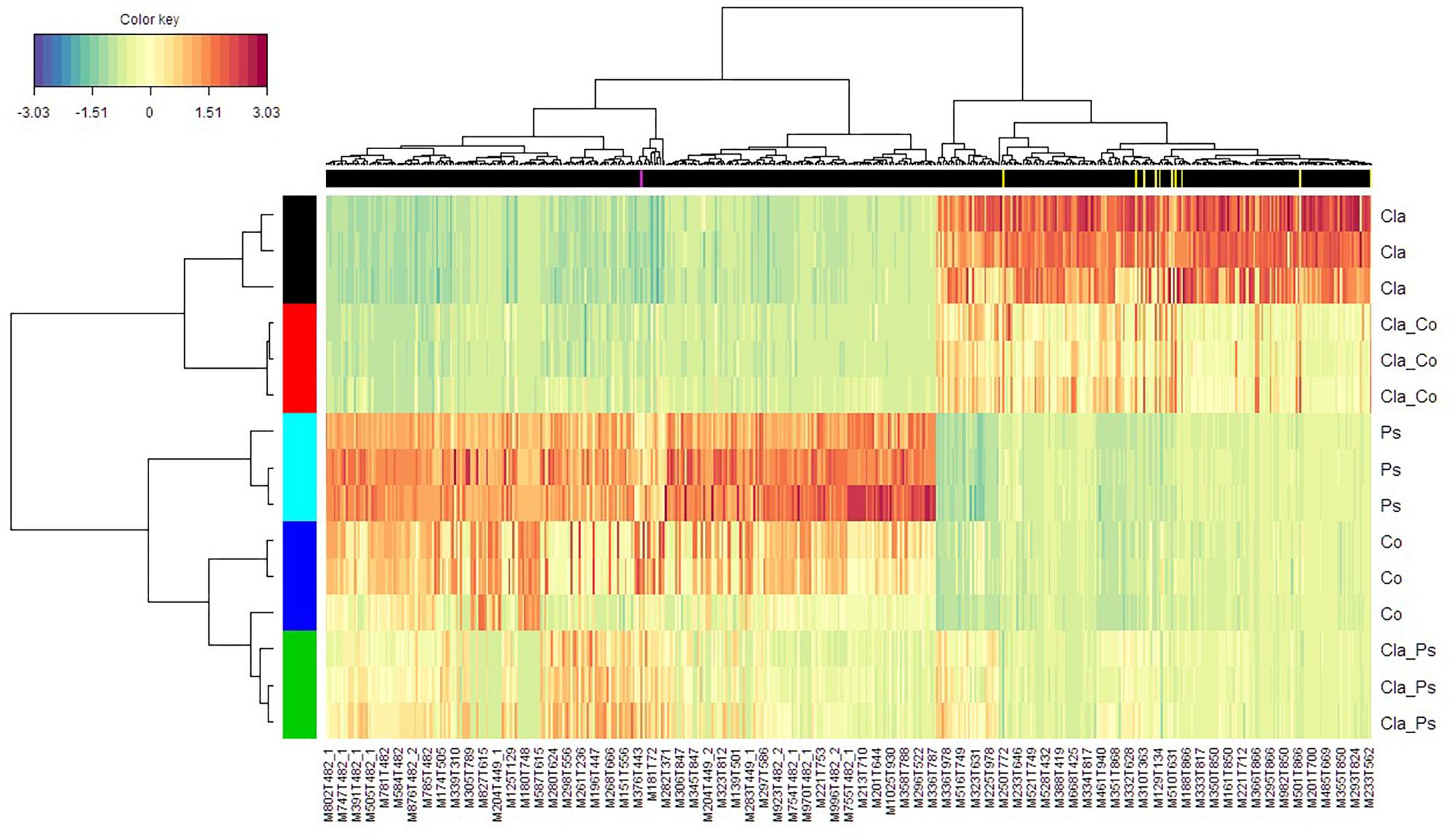
Figure 9. Heatmap displaying both the culture clusters in rows and the clusters of variables (m/z) in columns. Ps, Pseudoalteromonas; Co, Cobetia monocultures; Cla, Cladosporium monocultures; Cla-Ps, Cladosporium-Pseudoalteromonas co-cultures; Cla-Co, Cladosporium-Cobetia co-cultures. For convenience, not all variables are labeled. In the black banner are given variables marked in yellow and pink vertical lines for Cla-Co and Cla-Ps co-cultures, respectively, which were significantly filtered according to (i) a VIP value above 1.20 (and with respective SE values lower than VIP), and (ii) an absolute value of the correlation between the variables selected at the previous step and the PLS component which is above 0.75.
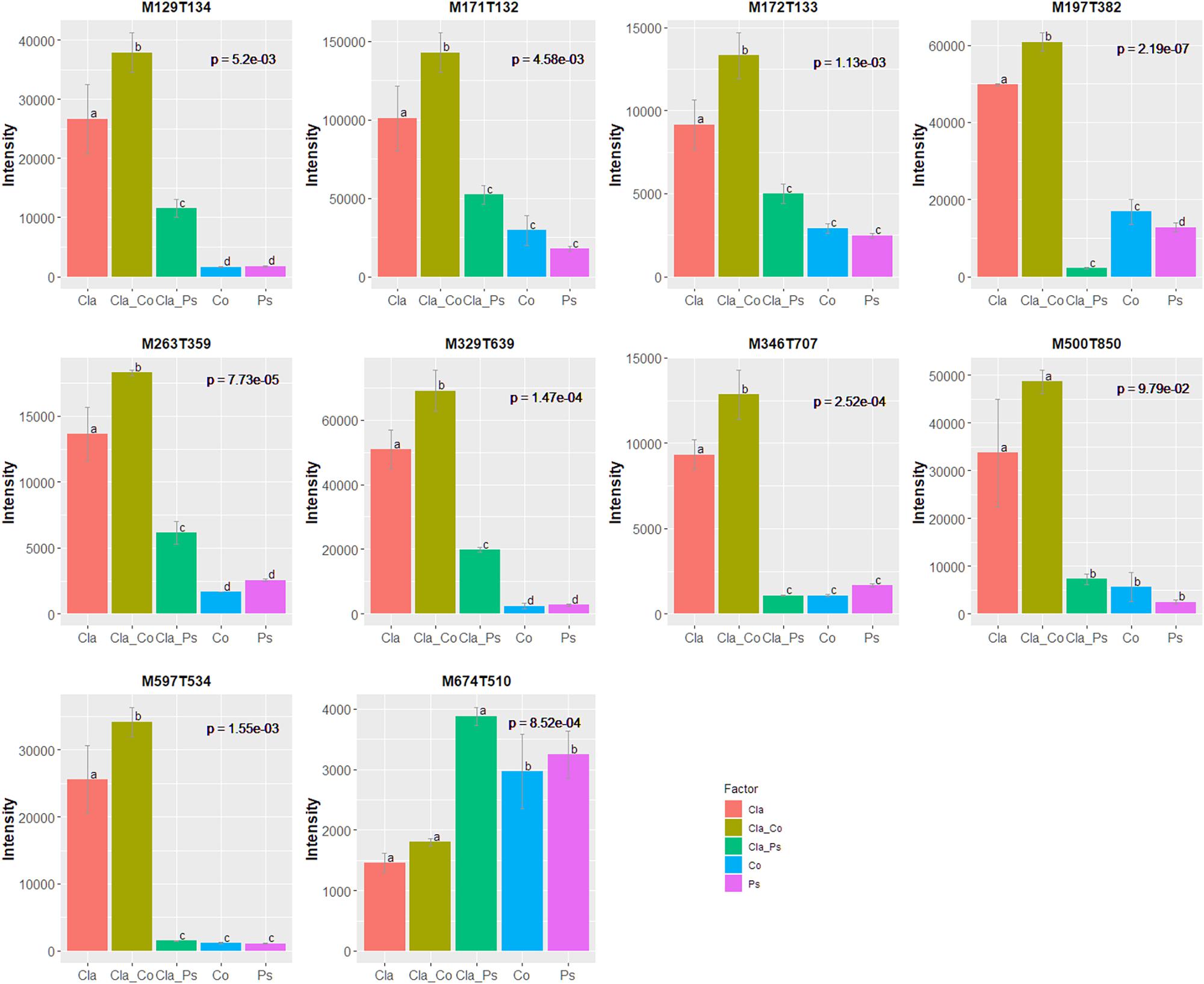
Figure 10. 10 variables displaying a correlation with the predicted luminescence response above 0.75 and a higher mean value in co-culture conditions compared to other mono-culture conditions or the alternate co-culture condition. p-Values coming from ANOVA are stringently corrected for the false discovery rate when considering the initial set of 4221 variables. Multiple comparisons of means used the Student-Newman-Keuls test.
Discussion
The data highlight the large diversity of cultivable bacterial endophytes associated with healthy L. digitata, S. latissima, A. nodosum, and P. canaliculata (Figures 1, 2). The four phyla (Proteobacteria, Firmicutes, Actinobacteria, and Bacteroidetes) and 27 genera that were identified are consistent with previous surveys of cultivable bacteria associated with macroalgae (Wiese et al., 2009; Hollants et al., 2013; KleinJan et al., 2017). Notably, the cultivable fraction of bacterial communities appears to vary depending on algal species, sampling site, and algal tissue. Nevertheless, bacteria classified as Cobetia and Pseudoalteromonas were isolated from every algal species, tissue, and sampling site. Moreover, these genera are the most frequently isolated from S. latissima, apart from Bacillus. Of note, Vibrio and Flavobacterium were not isolated from our samples, even though these are frequently isolated from brown algae (Hollants et al., 2013; Albakosh et al., 2016).
Among the isolated bacterial endophytes, 86% were found to produce AI-2, which triggers quorum sensing in the reporter strain V. campbellii MM32 (Table 1). Cobetia, Marinomonas, and Erwinia were the strongest inducers (>100% induction) of quorum sensing, whereas 93 and 73% of isolated Bacillus and Pseudoalteromonas induced quorum sensing only weakly (<50% induction). These results were confirmed by quantifying DPD, an AI-2 precursor, in bacterial supernatants using tandem mass spectrometry. As shown in Figure 3, Cobetia 352b and Marinomonas 424 produced around 700 nM of DPD, suggesting that these strains engage neighboring bacteria with AI-2 receptors. Pseudoalteromonas 352a also produced DPD but to a lesser extent (66 nM). Interestingly, fungal endophytes isolated along with these bacteria positively or negatively modulated AI-2 quorum sensing (Figures 4, 5). These results provide more evidence that AI-2 quorum sensing is involved in interkingdom signaling.
While inhibitory activity against quorum sensing was previously detected in marine fungi (Martín-Rodríguez et al., 2014), this is the first demonstration, to the best of our knowledge, that fungal metabolites may also enhance quorum sensing. However, such result is not surprising, as this effect was previously observed in metabolites from some other types of eukaryotes such as Chlamydomonas reinhardtii and Chlorella spp. microalgae (Rolland et al., 2016). Collectively, these findings suggest a key role for AI-2 signaling among endophytes of brown algae. On the other hand, we note that 14 fungal endophytes are strongly antimicrobial against the Vibrio biosensor (Figure 5), and thus may be similarly active against V. harveyi, a prominent pathogen in aquaculture (Zhang and Li, 2016).
As the fungus Cladosporium SL405T and the bacteria Pseudoalteromonas 352a and Cobetia 352b were isolated from the same holobiont (S. latissima), they were characterized in monoculture and in co-culture, with a view to assess the impact of fungal–bacterial interactions on metabolite production and quorum sensing. The data indicate that these isolates produce quorum sensing or quorum sensing-modulating compounds. Of note, these genera are frequently isolated from macroalgae (Hollants et al., 2013; Hulikere et al., 2016; Li et al., 2017). Pseudoalteromonas is of particular interest, as it was shown to produce antimicrobials or bioactive molecules against algal spores, invertebrate larvae, fungi, and other bacteria. Such molecules may help the host against surface colonization by these organisms (Holmström et al., 2002; Richards et al., 2017). Also, Pseudoalteromonas was implicated in Hole-Rotten disease (Wang et al., 2007).
As shown in Figures 6, 7, co-cultures of Cladosporium SL405T with two different bacteria produce different metabolites. These metabolites are also different from those produced by corresponding monocultures. For example, 28 and 5 metabolic features were at least 10-fold more abundant in co-cultures of Cladosporium SL405T with Cobetia 352b and Pseudoalteromonas 352a than in corresponding monocultures. This result implies that microbial interactions induce production of specific metabolites (Supplementary Table S3). Conversely, 120 and 87 features were at least 10-fold more abundant in Cobetia 352b monocultures and Pseudoalteromonas 352a monocultures than in co-cultures, suggesting either that production of these metabolites is inhibited by microbial interaction, or that these metabolites are degraded in co-cultures. Similarly, 105 and 108 features (of which 93 are common) were at least 10-fold more abundant in Cladosporium SL405T monocultures than in co-cultures with Cobetia 352b and Pseudoalteromonas 352a.
Taken together, these results demonstrate that metabolomes in co-cultures fundamentally differ from metabolomes in monocultures due to microbial interactions. Unfortunately, chemical characterization of culture-specific metabolites was not possible since none matched known natural products. Identification of the source of metabolites in co-cultures also remains a major challenge, since the structure of such metabolites and other related biochemical information would be required (Xu et al., 2018). Nevertheless, we found that different cultures have variable impact on quorum sensing (Figure 6), such that metabolites obtained from co-cultures of the same fungus with two different bacteria clearly display contrasting effects on quorum sensing (Figure 6). For instance, metabolites from a co-culture of Cladosporium SL405T and Pseudoalteromonas 352a diminish luminescence from the biosensor by 40%, while metabolites from a co-culture of Cladosporium SL405T and Cobetia 352b led to a loss of only 20%. The link between the impact on quorum sensing and the metabolites present in different culture conditions was strengthened by the PLS-regression based analysis highlighting 10 variables, highly correlated with the predicted luminescence response (absolute value of the correlation above 0.75), and specially induced in the co-culture conditions when compared to mono-culture conditions (Figure 10).
Collectively, our data provide the first evidence of quorum sensing and quorum quenching in bacterial and fungal endophytes of brown algae. These results highlight the importance of chemical communication among microbial components of a holobiont. Indeed, our laboratory model clearly demonstrates the impact of interspecies interactions on the production of metabolites, including metabolites involved in quorum quenching or in antagonizing other microorganisms. Our model also demonstrates the various phenotypes that may be observed in a given fungal or bacterial strain depending on environmental conditions. Hence, these results provide a glimpse of the complexity of molecular dialogues in the holobiont, and how this may impact host fitness.
Accordingly, the data also highlight the need to fully understand the functional role of all microbial members in the seaweed holobiont and their impact on algal fitness either in nature or in farms. Indeed, quorum sensing is already known to significantly affect the expression of virulence genes in aquaculture pathogens (Zhao et al., 2015). Quorum sensing was also demonstrated to control microbial colonization in the red algae Delisea pulchra, notably by release of halogenated furanone, which inhibits pathogenic epiphytic bacteria such as Nautella sp. (Harder et al., 2012). Hence, quorum sensing represents a very promising target in future studies of approaches to limit pathogenic effects in algae.
Data Availability
The datasets generated for this study can be found in GenBank, SUB4670131.
Author Contributions
AT carried out the experiments. AT, AP, RL, CH, KE, and SP analyzed the data. AT, RL, and SP wrote the manuscript. All authors conceived this study.
Funding
This work was supported by ATM “Microorganisms” grant from the Natural History Museum of Paris (SP), EMBRC project No. 262280 and PEPS ExoMod CNRS grant (SP), EC2CO Roseocom CNRS grant (RL), and the Convergence Sorbonne Université program (RL and SP). This work was funded through the IDEX SUPER (AT).
Conflict of Interest Statement
The authors declare that the research was conducted in the absence of any commercial or financial relationships that could be construed as a potential conflict of interest.
Acknowledgments
We acknowledge the Scottish Association for Marine Science (SAMS) and the Biological Station of Roscoff for hosting the field missions. We would like to greatly thank Philippe Potin (Marine Biology Station of Roscoff, France) for taxonomic identification of the brown macroalgae species during the field mission. We are grateful to the Bio2Mar platform (http://bio2mar.obs-banyuls.fr) for providing technical help and support.
Supplementary Material
The Supplementary Material for this article can be found online at: https://www.frontiersin.org/articles/10.3389/fmicb.2019.01693/full#supplementary-material
References
Albakosh, M. A., Naidoo, R. K., Kirby, M., and Bauer, R. (2016). Identification of epiphytic bacterial communities associated with the brown alga Splachnidium rugosum. J. Appl. Phycol. 28, 1891–1901. doi: 10.1007/s10811-015-0725-z
Allard, P. M., Péresse, T., Bisson, J., Gindro, K., Marcourt, L., Pham, V. C., et al. (2016). Integration of molecular networking and in-silico MS/MS fragmentation for natural products dereplication. Anal. Chem. 88, 3317–3323. doi: 10.1021/acs.analchem.5b04804
Altschul, S. F., Gish, W., Miller, W., Myers, E. W., and Lipman, D. J. (1990). Basic local alignment search tool. J. Mol. Biol. 215, 403–410. doi: 10.1016/S0022-2836(05)80360-2
Atkinson, S., and Williams, P. (2009). Quorum sensing and social networking in the microbial world. J. R. Soc. 6, 959–978. doi: 10.1098/rsif.2009.0203
Bassler, B. L., Wright, M., Showalter, R. E., and Silverman, M. R. (1993). Intercellular signalling in Vibrio harveyi: sequence and function of genes regulating expression of luminescence. Mol. Microbiol. 9, 773–786. doi: 10.1111/j.1365-2958.1993.tb01737.x
Böcker, S., and Rasche, F. (2008). Towards de novo identification of metabolites by analyzing tandem mass spectra. Bioinformatics 24, i49–i55. doi: 10.1093/bioinformatics/btn270
Bodor, A., Elxnat, B., Thiel, V., Schulz, S., and Wagner-Döbler, I. (2008). Potential for LuxS related signalling in marine bacteria and production of autoinducer-2 in the genus Shewanella. BMC Microbiol. 8:13. doi: 10.1186/1471-2180-8-13
R Core Team (2019). R: A Language and Environment for Statistical Computing. Vienna: R Foundation for Statistical Computing.
Cugini, C., Calfee, M. W., Farrow, J. M., Morales, D. K., Pesci, E. C., and Hogan, D. A. (2007). Farnesol, a common sesquiterpene, inhibits PQS production in Pseudomonas aeruginosa. Mol. Microbiol. 65, 896–906. doi: 10.1111/j.1365-2958.2007.05840.x
Debbab, A., Aly, A. H., and Proksch, P. (2012). Endophytes and associated marine derived fungi—ecological and chemical perspectives. Fungal Divers. 57, 45–83. doi: 10.1007/s13225-012-0191-8
Defoirdt, T., Miyamoto, C. M., Wood, T. K., Meighen, E. A., Sorgeloos, P., Verstraete, W., et al. (2007). The natural furanone (5Z)-4-bromo-5-(Bromomethylene)-3-butyl-2(5H)-furanone disrupts quorum sensing-regulated gene expression in Vibrio Harveyi by decreasing the DNA-binding activity of the transcriptional regulator protein LuxR. Environ. Microbiol. 9, 2486–2495. doi: 10.1111/j.1462-2920.2007.01367.x
Deveau, A., Bonito, G., Uehling, J., Paoletti, M., Becker, M., Bindschedler, S., et al. (2018). Bacterial–fungal interactions: ecology, mechanisms and challenges. FEMS Microbiol. Rev. 42, 335–352. doi: 10.1093/femsre/fuy008
Doberva, M., Ferandin, S. S., Toulza, E., Lebaron, P., and Lami, R. (2015). Diversity of quorum sensing autoinducer synthases in the global ocean sampling metagenomic database. Aquat. Microb. Ecol. 74, 107–119. doi: 10.3354/ame01734
Edgar, R. C. (2004). MUSCLE: multiple sequence alignment with high accuracy and high throughput. Nucleic Acids Res. 32, 1792–1797. doi: 10.1093/nar/gkh340
Egan, S., and Gardiner, M. (2016). Microbial dysbiosis: rethinking disease in marine ecosystems. Front. Microbiol. 7:991. doi: 10.3389/fmicb.2016.00991
Egan, S., Harder, T., Burke, C., Steinberg, P., Kjelleberg, S., and Thomas, T. (2013). The Seaweed holobiont: understanding seaweed–bacteria interactions. FEMS Microbiol. Rev. 37, 462–476. doi: 10.1111/1574-6976.12011
FAO (2018). The State of World Fisheries and Aquaculture. Rome: Food and Agriculture Organization of the United Nations.
Federle, M. J. (2009). “Autoinducer-2-based chemical communication in bacteria: complexities of interspecies signaling,” in Contributions to Microbiology, Vol. 16, eds M. Collin and R. Schuch (Basel: KARGER), 18–32. doi: 10.1159/000219371
Fernandes, N., Steinberg, P., Rusch, D., Kjelleberg, S., and Thomas, T. (2012). Community structure and functional gene profile of bacteria on healthy and diseased thalli of the red seaweed Delisea Pulchra. PloS One 7:e50854. doi: 10.1371/journal.pone.0050854
Fourie, R., Ells, R., Swart, C. W., Sebolai, O. M., Albertyn, J., and Pohl, C. H. (2016). Candida albicans and Pseudomonas aeruginosa interaction, with focus on the role of eicosanoids. Front. Physiol. 7:64. doi: 10.3389/fphys.2016.00064
Freeman, J. A., and Bassler, B. L. (1999). Sequence and function of LuxU: a two-component phosphorelay protein that regulates quorum sensing in Vibrio Harveyi. J. Bacteriol. 181, 899–906.
Fries, N. (1979). Physiological characteristics of Mycosphaerella ascophylli, a fungal endophyte of the marine brown alga Ascophyllum nodosum. Physiol. Plant. 45, 117–121. doi: 10.1111/j.1399-3054.1979.tb01674.x
Gachon, C. M., Sime-Ngando, T., Strittmatter, M., Chambouvet, A., and Kim, G. H. (2010). Algal diseases: spotlight on a black box. Trends Plant Sci. 15, 633–640. doi: 10.1016/j.tplants.2010.08.005
Graça, A. P., Bondoso, J., Gaspar, H., Xavier, J. R., Monteiro, M. C., de la Cruz, M., et al. (2013). Antimicrobial activity of heterotrophic bacterial communities from the marine sponge Erylus discophorus (Astrophorida, Geodiidae). PLoS One 8:e78992. doi: 10.1371/journal.pone.0078992
Grandclément, C., Tannières, M., Moréra, S., Dessaux, Y., and Faure, D. (2016). Quorum quenching: role in nature and applied developments. FEMS Microbiol. Rev. 40, 86–116. doi: 10.1093/femsre/fuv038
Hammer, B. K., and Bassler, B. L. (2003). Quorum sensing controls biofilm formation in Vibrio cholerae. Mol. Microbiol. 50, 101–104. doi: 10.1046/j.1365-2958.2003.03688.x
Harder, T., Campbell, A. H., Egan, S., and Steinberg, P. D. (2012). “Chemical mediation of ternary interactions between marine holobionts and their environment as exemplified by the red alga Delisea pulchra.”J. Chem. Ecol. C 38, 442–450. doi: 10.1007/s10886-012-0119-5
Hardie, K. R., and Heurlier, K. (2008). Establishing bacterial communities by’word of mouth’: luxS and autoinducer 2 in biofilm development. Nat. Rev. Microbiol. 6, 635–643. doi: 10.1038/nrmicro1916
Hassani, M. A., Durán, P., and Hacquard, S. (2018). Microbial interactions within the plant holobiont. Microbiome 6:58. doi: 10.1186/s40168-018-0445-0
Hmelo, L. R. (2017). Quorum sensing in marine microbial environments. Ann. Rev. Marine. Sci. 9, 257–281. doi: 10.1146/annurev-marine-010816-060656
Hollants, J., Leliaert, F., De Clerck, O., and Willems, A. (2013). What we can learn from sushi: a review on seaweed-bacterial associations. FEMS Microbiol. Ecol. 83, 1–16. doi: 10.1111/j.1574-6941.2012.01446.x
Holmström, C., Egan, S., Franks, A., McCloy, S., and Kjelleberg, S. (2002). Antifouling activities expressed by marine surface associated Pseudoalteromonas species. FEMS Microbiol. Ecol. 41, 47–58. doi: 10.1111/j.1574-6941.2002.tb00965.x
Hulikere, M. M., Joshi, C. G., Ananda, D., Poyya, J., and Nivya, T. (2016). Antiangiogenic, wound healing and antioxidant activity of Cladosporium cladosporioides (Endophytic fungus) isolated from seaweed (Sargassum wightii). Mycology 7, 203–211. doi: 10.1080/21501203.2016.1263688
Ismail, A. S., Valastyan, J. S., and Bassler, B. L. (2016). A host-produced autoinducer-2 mimic activates bacterial quorum sensing. Cell Host Microbe 19, 470–480. doi: 10.1016/j.chom.2016.02.020
Jones, E. B. G., Pang, K. L., and Stanley, S. J. (2012). “Fungi from marine algae,” in Marine Fungi: And Fungal-Like Organisms, eds E. B. G. Jones and K.-L. Pang (Berlin: Walter de Gruyter).
KleinJan, H., Jeanthon, C., Boyen, C., and Dittami, S. M. (2017). Exploring the cultivable Ectocarpus microbiome. Front. Microbe 8:2456. doi: 10.3389/fmicb.2017.02456
Kumar, S., Stecher, G., and Tamura, K. (2016). MEGA7: molecular evolutionary genetics analysis version 7.0 for bigger datasets. Mol. Biol. Evol. 33, 1870–1874. doi: 10.1093/molbev/msw054
Li, H. L., Li, X. M., Mándi, A., Antus, S., Li, X., Zhang, P., et al. (2017). Characterization of cladosporols from the marine algal-derived endophytic fungus Cladosporium Cladosporioides EN-399 and configurational revision of the previously reported cladosporol derivatives. J. Org. Chem. 82, 9946–9954. doi: 10.1021/acs.joc.7b01277
Loque, C. P., Medeiros, A. O., Pellizzari, F. M., Oliveira, E. C., Rosa, C. A., and Rosa, L. H. (2010). Fungal community associated with marine macroalgae from Antarctica. Polar Biol. 33, 641–648. doi: 10.1007/s00300-009-0740-0
Martín-Rodríguez, A. J., Reyes, F., Martín, J., Pérez-Yépez, J., León-Barrios, M., Couttolenc, A., et al. (2014). Inhibition of bacterial quorum sensing by extracts from aquatic fungi: first report from marine endophytes. Mar. Drugs 12, 5503–5526. doi: 10.3390/md12115503
Miller, S. T., Xavier, K. B., Campagna, S. R., Taga, M. E., Semmelhack, M. F., Bassler, B. L., et al. (2004). Salmonella typhimurium recognizes a chemically distinct form of the bacterial quorum-sensing signal AI-2. Mol. Cell 15, 677–687. doi: 10.1016/j.molcel.2004.07.020
Pérez-Rodríguez, I., Bolognini, M., Ricci, J., Bini, E., and Vetriani, C. (2015). From deep-sea volcanoes to human pathogens: a conserved quorum-sensing signal in epsilon proteobacteria. ISME J. 9, 1222–1234. doi: 10.1038/ismej.2014.214
Rezzonico, F., and Duffy, B. (2008). Lack of genomic evidence of AI-2 receptors suggests a non-quorum sensing role for LuxS in most bacteria. BMC Microbiol. 8:154. doi: 10.1186/1471-2180-8-154
Richards, G. P., Watson, M. A., Needleman, D. S., Uknalis, J., Boyd, E. F., and Fay, J. P. (2017). Mechanisms for Pseudoalteromonas piscicida-induced killing of Vibrios and other bacterial pathogens. Appl. Environ. Microbiol. 83, e175–e117. doi: 10.1128/AEM.00175-17
Rohart, F., Gautier, B., Singh, A., and Le Cao, K.-A. (2017). mixOmics: an R package for ’omics feature selection and multiple data integration. PLoS Comput. Biol. 13:e1005752. doi: 10.1371/journal.pcbi.1005752
Rolland, J. L., Stien, D., Sanchez-Ferandin, S., and Lami, R. (2016). Quorum sensing and quorum quenching in the phycosphere of phytoplankton: a case of chemical interactions in ecology. J. Chem. Ecol. 42, 1201–1211. doi: 10.1007/s10886-016-0791-y
Singh, R. P., and Reddy, C. R. K. (2014). Seaweed-microbial interactions: key functions of seaweed-associated bacteria. FEMS Microbiol. Ecol. 88, 213–230. doi: 10.1111/1574-6941.12297
Smith, C. A., Want, E. J., O’Maille, G., Abagyan, R., and Siuzdak, G. (2006). XCMS: processing mass spectrometry data for metabolite profiling using nonlinear peak alignment, matching, and identification. Anal. Chem. 78, 779–787. doi: 10.1021/ac051437y
Tapia, J. E., González, B., Goulitquer, S., Potin, P., and Correa, J. A. (2016). Microbiota influences morphology and reproduction of the brown alga Ectocarpus sp. Front. Microbiol 7:197. doi: 10.3389/fmicb.2016.00197
Vallet, M., Strittmatter, M., Murúa, P., Lacoste, S., Dupont, J., Hubas, C., et al. (2018). Chemically-mediated interactions between macroalgae, their fungal endophytes, and protistan pathogens. Front. Microbiol. 9:3161. doi: 10.3389/fmicb.2018.03161
Wahl, M., Goecke, F., Labes, A., Dobretsov, S., and Weinberger, F. (2012). The second skin: ecological role of epibiotic biofilms on marine organisms. Front. Microbiol. 3:292. doi: 10.3389/fmicb.2012.00292
Wang, G., Shuai, L., Li, Y., Lin, W., Zhao, X., and Duan, D. (2007). Phylogenetic analysis of apiphytic marine bacteria on hole-rotten siseased sporophytes of Laminaria japonica. J. Appl. Phycol. 20, 403–409. doi: 10.1007/s10811-007-9274-4
Wang, T. N., Kaksonen, A. H., and Hong, P. Y. (2018). Evaluation of two autoinducer-2 quantification methods for application in marine environments. J. Appl. Microbiol. 124, 1469–1479. doi: 10.1111/jam.13725
Whiteley, M., Diggle, S. P., and Greenberg, E. P. (2017). Progress in and promise of bacterial quorum sensing research. Nature 551, 313–320. doi: 10.1038/nature24624
Wiese, J., Thiel, V., Nagel, K., Staufenberger, T., and Imhoff, J. F. (2009). Diversity of antibiotic-active bacteria associated with the brown alga Laminaria saccharina from the Baltic Sea. Marine Biotechnol. 11, 287–300. doi: 10.1007/s10126-008-9143-4
Wongsuk, T., Pumeesat, P., and Luplertlop, N. (2016). Fungal quorum sensing molecules: role in fungal morphogenesis and pathogenicity. J. Basic Microbiol. 56, 440–447. doi: 10.1002/jobm.201500759
Xu, F., Song, X., Cai, P., Sheng, G., and Yu, H. (2017). Quantitative determination of AI-2 quorum-sensing signal of bacteria using high performance liquid chromatography–tandem mass spectrometry. J. Environ. Sci. 52, 204–209. doi: 10.1016/j.jes.2016.04.018
Xu, X. Y., Shen, X. T., Yuan, X. J., Zhou, Y. M., Fan, H., Zhu, L. P., et al. (2018). Metabolomics investigation of an association of induced features and corresponding fungus during the Co-culture of Trametes versicolor and Ganoderma applanatum. Front. Microbiol. 8:2647. doi: 10.3389/fmicb.2017.02647
Zhang, W., and Li, C. (2016). Exploiting quorum sensing interfering strategies in Gram-negative bacteria for the enhancement of environ- mental applications. Front. Microbiol. 6:1535. doi: 10.3389/fmicb.2015.01535
Zhao, J., Chen, M., Quan, C. S., and Fan, S. D. (2015). Mechanisms of quorum sensing and strategies for quorum sensing disruption in aquaculture pathogens. J. Fish Dis. 38, 771–786. doi: 10.1111/jfd.12299
Zozaya-Valdes, E., Egan, S., and Thomas, T. (2015). A comprehensive analysis of the microbial communities of healthy and diseased marine macroalgae and the detection of known and potential bacterial pathogens. Front. Microbiol 6:146. doi: 10.3389/fmicb.2015.00146
Zuccaro, A., Schoch, C. L., Spatafora, J. W., Kohlmeyer, J., Draeger, S., and Mitchell, J. I. (2008). Detection and identification of fungi intimately associated with the brown seaweed Fucus serratus. Appl. Env. Microbiol. 74, 931–941. doi: 10.1128/AEM.01158-07
Keywords: quorum sensing (QS), AI-2, bacterial–fungal interaction, kelp microbiota, algal holobiont
Citation: Tourneroche A, Lami R, Hubas C, Blanchet E, Vallet M, Escoubeyrou K, Paris A and Prado S (2019) Bacterial–Fungal Interactions in the Kelp Endomicrobiota Drive Autoinducer-2 Quorum Sensing. Front. Microbiol. 10:1693. doi: 10.3389/fmicb.2019.01693
Received: 16 April 2019; Accepted: 09 July 2019;
Published: 31 July 2019.
Edited by:
Tom Defoirdt, Ghent University, BelgiumReviewed by:
Qian Yang, Yellow Sea Fisheries Research Institute (CAFS), ChinaNatrah Fatin Mohd Ikhsan, Putra Malaysia University, Malaysia
Copyright © 2019 Tourneroche, Lami, Hubas, Blanchet, Vallet, Escoubeyrou, Paris and Prado. This is an open-access article distributed under the terms of the Creative Commons Attribution License (CC BY). The use, distribution or reproduction in other forums is permitted, provided the original author(s) and the copyright owner(s) are credited and that the original publication in this journal is cited, in accordance with accepted academic practice. No use, distribution or reproduction is permitted which does not comply with these terms.
*Correspondence: Raphaël Lami, bGFtaUBvYnMtYmFueXVscy5mcg==; Soizic Prado, c29pemljLnByYWRvQG1uaG4uZnI=