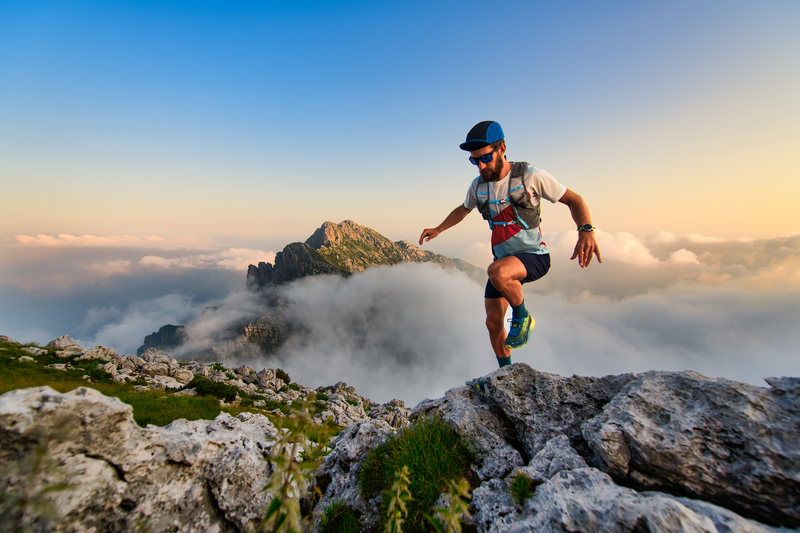
94% of researchers rate our articles as excellent or good
Learn more about the work of our research integrity team to safeguard the quality of each article we publish.
Find out more
REVIEW article
Front. Med. , 27 March 2025
Sec. Pulmonary Medicine
Volume 12 - 2025 | https://doi.org/10.3389/fmed.2025.1577559
This article is part of the Research Topic Application of Multimodal Data and Artificial Intelligence in Pulmonary Diseases View all articles
The pathophysiology and clinical manifestations of pulmonary embolism are complex, heterogeneous, and the disease burden is severe, and its prediction and diagnosis are of major challenges. Artificial intelligence (AI) is a field of computer science that involves the development of programs and complex data analysis designed to replicate human cognitive processes. In recent years, with the continuous development of medical information technology, the application of AI in the diagnosis and treatment of diseases has made rapid progress, especially in the field of pulmonary embolism, which is mainly based on imaging. In this review, we summarize the current application prospects and directions of AI in early prediction, screening, diagnosis, and prognosis of PE, and discuss the main challenges and future of AI in pulmonary embolism (PE), in order to provide a theoretical basis for the application of AI in the risk assessment and standardized management of PE.
Acute PE is the third leading cause of cardiovascular death worldwide (1), presenting with mild clinical symptoms or atypical features, and current diagnosis relies primarily on CT imaging, with computed tomography pulmonary angiography (CTPA) considered the diagnostic benchmark (2). However, interpreting CTPA results requires experienced medical expertise and avoiding missed and misdiagnosed due to subjective bias. Studies have shown that CTPA has a missed diagnosis rate of 14% and an overdiagnosis rate of 10% (3). Due to the insidious onset and diverse etiologies of PE, the detection rate is less than 5% (4), and the short-term mortality rate of untreated PE is as high as 30% (5, 6). Improving the survival rate of PE patients depends on early and accurate prediction, as well as active PE management. Therefore, early prediction, identification, and diagnosis of PE play a key role in patient outcomes (7, 8).
AI excels in medical image analysis as a subtype of information technology that uses algorithms to analyze (receive, process, and interpret) medical information and perform complex mathematical calculations (9). Machine learning (ML) is an emerging branch of artificial intelligence, whose core competitiveness lies in the ability to efficiently extract and analyze rich clinical features from massive case record databases, and accurately identify statistically significant patterns. Deep learning (DL), a subtype of ML that analyzes large amounts of data to improve the accuracy of creating concepts and accurately predicting pathologies (10), s currently one of the most widely used algorithms for medical purposes. ML has great potential in the medical field, including early prediction, rapid diagnosis, and risk assessment (11, 12).
AI plays an important role in thromboembolic diseases, especially in the early prediction and diagnosis of the disease (13). Early intervention with anticoagulants or other prophylaxis can reduce the incidence of PE and reduce patient mortality. A number of ML-based models have recently been developed to assess the risk of death from clinical disease, thereby enhancing clinical diagnosis and patient prognosis (14). Developing and validating ML models that accurately predict the risk of PE patients can help clinicians make informed decisions and improve patient survival. In this article, we summarize the current application of AI and ML in pulmonary embolism to elucidate the impact of AI in the medical field.
AI, as a branch of computer science, explores and studies the nature of intelligent behavior and creates intelligent machines that respond in a similar way to human intelligence. These intelligent behaviors, including but not limited to learning, reasoning, problem solving, knowledge representation, planning, natural language processing, perception, pattern recognition, and creation, have become an umbrella term for technologies that mimic human “natural intelligence” or cognitive functions. ML is a subfield of AI that enables computers to derive knowledge and experience from data and use this knowledge and experience to make pattern recognition, prediction, and decision-making through the learning of computer systems and automated reasoning. In contrast to traditional statistical methods, which provide insights from observed inter-population differences based on collected clinical features, ML excels at revealing complex relationships between various features, thereby facilitating precise classification (15–17). Deep learning, on the other hand, is a subset of ML that represents an advanced approach to processing data using artificial neural networks with brain-like structures, with little or no artificial feature engineering, and is better at processing complex, high-dimensional data.
ML, the core discipline of AI, connects statistics and computer science to create predictive models capable of analyzing new data using large data sets (18), which stands out among several medical specialties and is the pathway to smart medicine (19, 20). Considering that clinicians need to evaluate large amounts of patient information to further guide clinical decision-making (21), it can be a daunting task for anyone. ML can create algorithms with comparable performance to human physicians (22), and when applied to medical images, algorithms are used to analyze and extract information from images generated by diagnostic imaging equipment, train algorithms to recognize patterns, identify relevant features, and perform specific tasks, such as diagnosing diseases, detecting abnormalities, or classifying medical conditions, and integrating and understanding relevant clinical data at scale, providing fast and accurate analytical tools to support medical professionals to assess risk, aid in diagnosis, It is excellent in judging prognosis and guiding personalized treatment, and is able to detect patterns or features that are not immediately visible to the naked eye, and is excellent in the processing of complex and large amounts of medical data (23, 24). ML has shown considerable potential in medical research and disease prediction (10, 25). For pulmonary embolism, ML models can help clinicians identify high-risk patients in the early stages of PE, enabling early intervention.
With the advent of AI as a predictive tool, some studies have applied AI to the prediction and screening of PE. The ML model performs better than the traditional risk scoring in PE risk stratification, and the new diagnostic model based on ML analysis can help overcome the limitations of the traditional score, improve the risk prediction performance, and accelerate the diagnosis of PE (26). A recent retrospective study analyzed the clinical data characteristics of 1,480 patients with suspected PE who were hospitalized in West China Hospital of Sichuan University from May 2015 to April 2020, used different MLs to make a PE prediction model, and evaluated their performance using the area under the receiver operating characteristic curve (AUC), and verified their predictive ability using SHapley Additive Interpretation (SHAP) values. The results showed an AUC of 0.776 (95% CI 0.774–0.778), suggesting that AI-based ML-based PE prediction models can help optimize early diagnosis and timely treatment strategies, thereby improving the prognosis of PE patients. At the same time, it provides literature support for the use of SHAP values to explain the contribution of ML in PE prediction. The convergence of engineering and medicine has shown excellent performance in predicting PE risk stratification (27). CTPA is the mainstay of clinical diagnosis of PE, but it is time-consuming, expensive, and in some cases unavailable, especially in primary care settings, and its simultaneous application may have adverse effects, particularly in patients with a history of kidney disease or allergies (28, 29). AI uses routinely collected patient healthcare data to derive the disease prognosis of patients with suspected PE, which can provide a reliable basis for patients’ care decisions. One study designed and evaluated a ML modeling approach called the PE Outcome Prediction Model (PERFORM) to predict PE imaging outcomes based on patient electronic medical record (EMR) data, including variables such as demographics, vital signs (absolute and change from baseline), diagnosis, medications, and laboratory test results, to provide patients with further CT imaging with a patient-specific risk score, improve detection rates, and reduce unnecessary CT examinations (30). To validate the performance of ML in predicting the risk of PE in high-risk inpatients with PE, Shen et al. retrospectively analyzed approximately 2 million adult hospitalized cases in the United States from 2011 to 2017, using demographics, vital signs, and laboratory tests of adult inpatients from 12 institutions to train the XGBoost model (31), and patient populations from 32 healthcare facilities performed external validation of the model, as measured by the area under the receiver operating characteristic curve (AUROC) Evaluating model performance, using backward elimination regression to determine the correlation between external validation set features and AUROC, the results showed that the external test AUROC ranged from 0.79 to 0.93 with a mean of 0.88, and the backward elimination regression determined a negative correlation between the percentage of PE positive visits and AUROC (β = −0.015, p < 0.001), indicating that this PE prediction model performed well in different external patient populations, demonstrating its potential as a clinical decision support tool (32).
PE itself may lead to pulmonary hypertension, cardiac insufficiency, respiratory failure and other serious consequences, and these pathological processes may be further exacerbated when complicated by other diseases, and the disease is often more complex, making diagnosis and treatment more difficult, and the prognosis is often poor, and delayed or missed diagnosis can be life-threatening. Therefore, the development of AI models to predict the risk of PE in other diseases and predict the risk of PE in other diseases plays an important role in improving the survival rate of patients and improving the quality of clinical decision-making.
Heart failure is a risk factor for predicting and stratifying mortality and risk stratification for PE, and short-term mortality is high in patients with PE (PE) and heart failure (33, 34). There is a need to predict the short-term mortality risk of patients with PE in heart failure in clinical practice, reduce death and disability through early detection and prevention, and improve patient outcomes. Liu et al. enrolled 472 patients with PE and heart failure, developed 6 ML models after feature selection, and externally validated patients in the eICU Cooperative Research Database (eICU-CRD) (35) by area under the curve (AUC), calibration curve, decision curve analysis (DCA), net weight classification improvement (NRI), and comprehensive discrimination improvement (IDI) to evaluate predictive performance, The Support Vector Machine model was found to perform the best prediction, with an AUC of 0.835, a higher degree of calibration, a wider risk threshold for clinical benefit (from 0 to 90%), and better than traditional mortality risk assessment systems such as the PE Severity Index and the Simplified PE Severity Index (34, 36, 37). Atrial fibrillation is one of the important factors contributing to the increase in morbidity and mortality worldwide, and its abnormal flow pattern can lead to the formation of blood clots in the atrium, which can break off and spread through the bloodstream, leading to arterial occlusion in various organs, such as PE (38). In order to predict the occurrence of composite thromboembolic events in elderly patients with atrial fibrillation, REN et al. collected data from 6,079 elderly inpatients admitted to the General Hospital of the Chinese People’s Liberation Army from January 2010 to June 2022, and randomly divided them into a training dataset (n = 4,225) and a validation dataset (n = 1,824) in a ratio of 7:3, and trained on the training dataset using four ML models (logistic regression, decision tree, random forest, XGBoost). The results showed that the random forest model showed good clinical validity, outperforming other models (ACC: 0.9144, SEN: 0.7725, SPE: 0.9489, AUC: 0.927, 95% CI: 0.9105–0.9443), showing the best predictive performance (39). Sun et al. conducted a retrospective observational study on data from 930 patients, developed five ML prediction models using data from the development cohort, selected the best model through five-fold cross-validation, and validated using an external validation cohort to assess the risk of PE in tuberculosis patients, and the results showed that the random forest model outperformed the other models with an AUC of 0.839 (95% CI 0.780–0.899) and maintained the highest average performance (AUC: 0.906 ± 0.041), indicating that random forests have significant advantages in predicting the risk of PE in TB patients compared to various ML models used to predict PE in TB patients, and demonstrating the application of AI in healthcare (40). Patients with autoimmune inflammatory rheumatic diseases have a high mortality rate after PE (41), and accurate stage prediction and risk assessment can improve survival. Hu et al. conducted a retrospective, multicenter study designed clinical data from 7,254 patients in Tongji Hospital from 2014 to 2022 to train an ML model using univariate logistic regression and minimal absolute contraction and selection operators to select clinical features, and the results showed that the ML-based model can accurately and conveniently predict the occurrence of PE in patients with autoimmune inflammatory rheumatism who are clinically suspected of PE (42). This study significantly improves the efficiency of predicting specific pathophysiological conditions, provides substantial help for doctors to finalize the diagnosis, and realizes the early and accurate diagnosis and risk prediction of PE in other diseases in clinical practice. It is of great significance in the field of prevention and treatment of PE to develop AI models that predict the risk of PE in other diseases and improve the early warning ability of patients with PE risk of other diseases.
PE is a serious medical emergency that requires prompt recognition and intervention, and early initiation of anticoagulation may improve outcomes for suspected PE (43). However, due to nonspecific symptoms associated with PE, less than 10% of patients evaluated are ultimately diagnosed with PE (44, 45). Missed diagnoses and misdiagnoses lead to delays in treatment and exacerbate disease progression (46–48). Therefore, the development and validation of natural language processing models to identify low-risk PE in real time and facilitate safe outpatient management is critical to reduce mortality from PE (49–51). In asymptomatic patients, radiologists may overlook most incidental PEs. The AI model can quickly analyze a large amount of imaging data, maintain sensitivity and specificity when interpreting suboptimal CTPA examinations, significantly enhance the detection of occasional PE, and significantly shorten the diagnosis time, which is crucial for patients with acute PE who need timely diagnosis and intervention, helping to shorten the time from symptom onset to diagnosis, and buying valuable treatment time for patients. AI algorithms have shown significantly higher sensitivity in detecting PE in routine scans compared to initial reports from radiologists, and the application of AI has significantly reduced the rate of missed diagnosis of incidental PE by radiologists (52). For example, one study showed that the implementation of AI algorithms significantly reduced the missed diagnosis rate from 50 to 7.1% compared to the absence of AI assistance, resulting in improved diagnostic accuracy (53). Langius-Wiffen et al. retrospectively analyzed the data of serial CTPA scans of 3,316 patients referred for suspected PE between February 24, 2018 and December 31, 2020, using a CE-marked and FDA-approved AI algorithm (54), and compared the output of the AI with the report of the attending radiologist, and the results showed that the sensitivity of the AI algorithm in detecting PE was significantly higher compared to the radiology report (96.8% vs. 91.6, p < 0.001). The specificity of AI was also significantly higher (99.9% vs. 99.7%, p = 0.035), and the implementation of AI-assisted reporting could reduce the number of missed (55). The development of AI methods with good diagnostic capabilities for PE can improve the performance and reproducibility of radiological diagnostics (56–58).
Ventilated/perfused single-photon emission computed tomography (V/Q-SPECT) is one of the most widely used imaging techniques for the diagnosis of PE, according to the 2019 guidelines for the diagnosis and management of PE developed in collaboration with the European Respiratory Society, however, V/Q-SPECT is invasive, expensive, and not as widely available as other imaging modalities such as CT, especially in resource-limited hospital settings (59). Li et al. selected potential perfusion-related features for integration into the model and found that the spatial distribution of features and the visualization of model output showed a high degree of agreement with lung function imaging, validating the feasibility of using quantitative texture analysis and data-driven ML pipelines to generate voxel-level lung perfusion surrogates between different institutions, providing a radiation-free, widely available alternative for functional lung imaging for the management of pulmonary vascular disease, and facilitating noninvasive screening of PE (60). AI-assisted workflows prioritize episodic PE with routine CT scans, and cancer patients have demonstrated high diagnostic accuracy (61). Wiklund et al. retrospectively analyzed the impact of AI algorithms on the detection rate of incidental PE in cancer-related patients, and showed that the reported prevalence of incidental PE was significantly higher and significantly shortened the reporting turnaround time and treatment time of patients with cancer-related incidental PE for a period of time after the implementation of AI (62).
Compared with larger embolism, small and easily overlooked PE has the characteristics of insidious symptoms, difficult diagnosis, and the need for active prevention and screening, which makes the detection of small PE often lead to misdiagnosis and missed diagnosis, therefore, in clinical work, doctors should maintain a high degree of vigilance, conduct detailed medical history and physical examination of patients suspected of PE, and timely diagnosis and treatment, and solve the detection problem of small PE is very important to improve the overall diagnostic results. In order to improve the identification and diagnosis of small and easily overlooked PE, Wu et al. collected the data of 142 CTPA examinations conducted at Tianjin Medical University General Hospital from January 2017 to October 2018 for a retrospective study, and developed a deep learning model SPE-YOLO, which showed 90.70% sensitivity and 86.45% accuracy in the data of the external validation set, showing strong diagnostic ability in identifying small PE, It provides clinicians with more accurate and efficient diagnostic tools (63). Yehuda et al. collected demographic, comorbidity, and drug data from 2,568 patients with PE and 52,598 control patients to build an accurate and informative ML model that would facilitate the early diagnosis of PE during patient hospitalization (64). Deep learning can improve detection performance and help clinicians complete diagnostic tasks (65–69).
The development and application of AI models is an important part of promoting the intelligent transformation of the medical industry, which will help improve the prognosis and quality of life of patients with PE. By analyzing the patient’s clinical data, imaging features, laboratory test results and other information, AI can predict the future development trend of the patient’s disease and the risk of possible complications, which can help clinicians formulate coping strategies in advance, guide subsequent treatment, and improve patient prognosis. ML models have been shown to accurately predict the 30-day mortality rate in critically ill PE patients, which can be further used to reduce the burden of ICU admissions, reduce mortality, and improve the quality of life of critically ill PE patients (70). Lian et al. conducted a retrospective analysis of the clinical data of 312 patients diagnosed with PE using CTPA in the Taicang Affiliated Hospital of Soochow University from 2016 to 2024 to evaluate the sensitivity, accuracy, specificity and AUC of four ML models (XGBoost, Random Forest, Logistic Regression and SVM) in predicting the prognosis of PE, and the results showed that the XGBoost model showed good performance, The accuracy was 0.882, the F1 score was 0.889, the precision was 0.917, the sensitivity was 0.863, the specificity was 0.905, and the AUC was 0.873. Future studies could combine these data to enhance the understanding of PE risk factors and improve predictive models for better clinical outcomes (71). Sadegh-Zadeh et al. utilized different oversampling techniques to improve the performance of various ML models, for early mortality prediction, and the results showed that the randomly oversampled RF model demonstrated superior performance across the 5 models evaluated, achieving higher accuracy and precision in predicting death grades, The study also highlighted the potential of ML to improve the accuracy of mortality prediction in patients with acute PE and provide a theoretical basis for clinical decision-making (72).
Anticoagulation is the mainstay of treatment for patients with PE (73). However, in clinical practice, some patients with PE may need to discontinue the drug early for a variety of reasons, including active bleeding, urgent need for surgery, contraindications to anticoagulation, etc. Identifying the likelihood of an increased risk of adverse outcomes before discontinuing anticoagulation can help improve patient outcomes. Mora et al. used data from the RIETE (74) registry to compare the prognostic capabilities of five ML models, including decision trees, K-nearest neighbors, support vector machines, ensembles, and neural networks (NN), to evaluate model performance by evaluating the model performance by measuring the test data for each model and the confusion matrix measure of the calibration plot to assess patients at increased risk of a compound of fatal PE or recurrent venous thromboembolism 30 days after discontinuation of the drug. The results showed that the ML-NN method was able to reliably predict fatal PE, sudden death, or recurrent VTE after premature cessation of anticoagulation, with an area under the ROC curve of 96 percent, an improvement of approximately 0.20 over traditional logistic regression, far exceeding the performance of traditional logistic regression models (75). Muñoz et al. extracted unstructured data from electronic health records of 9 hospitals in Spain between 2014 and 2018 using natural language processing (NLP) and ML-based EHRead@ techniques, performed clinical feature selection, and trained different predictive models to assess the risk of VTE recurrence within 6 months in cancer patients who received anticoagulation therapy at the time of initial VTE diagnosis. The findings can help clinicians identify high-risk patients and improve their clinical management (76).
The development and implementation of AI in various clinical efforts across the medical field requires the inclusion of more clinical data in multiple centers and the minimization of possible bias due to unbalanced training, poor architecture design or selection, and uneven application of models, which can not only improve the reliability and accuracy of predictive models, but also avoid the influence of regional or technical factors and demographic characteristics on the model (77, 78). According to the FDA, despite tens of thousands of articles related to AI and computer-aided diagnosis (CAD) published over the past 20 years, as of July 30, 2023, only 692 market-liquidated AI medical algorithms are available in the United States (79). When clinical data is only partially available and laboratory test results are still unavailable, the diagnosis needs to be made immediately after the patient’s emergency department presentation. In a study conducted by Cheik et al., software utilizing AI for image interpretation demonstrated the ability to identify 219 suspected PEs, of which 176 were confirmed to be true PE. The AI system had the highest sensitivity and negative predictive value (NPV) of 92.6 and 98.6%, respectively, outpacing radiologists who achieved 90% sensitivity and 98.1% NPV. Meanwhile, radiologists performed well in specificity and positive predictive value, with a specificity of 99.1% and a positive predictive value of 95%, compared to a specificity of 95.8% and a positive predictive value of 80.4% for AI. These results highlight the potential of AI to enhance PE detection, while also revealing the excellent performance of radiologists in reducing false positives (80). In order to address the challenge of bias in algorithm development brought about by population differences within the healthcare system, a bias-free symptom prediction framework was proposed using the state-of-the-art PE detection backbone and large-scale clinical language model, which achieved a higher survival correlation than the clinical assessment index, reduced the inequity of medical implementation leading to PE prognosis and other diseases, and improved the fairness and accuracy of medical AI systems (81). At the same time, the integration of ML into clinical practice introduces key ethical considerations, including issues of liability for medical errors, healthcare professionals’ understanding of how these algorithms generate predictions, and concerns about ethics, safety, and patient data control (82, 83).
AI has played an important role in the prediction, diagnosis, and prognosis assessment of PE, and with the popularization of PE prediction models, attempts have been made to construct optimal PE prediction models based on cause classification to maximize the clinical outcomes of PE patients (84, 85). Future research should continue to evaluate the feasibility and cost-effectiveness of clinically relevant AI tools, requiring further research and interdisciplinary collaboration to engage a variety of stakeholders in AI development and deepen insights into potential biases and ethical considerations. Select, support, and fund more highly focused AI projects that address challenges encountered during development and implementation, and further explore the impact of AI on patient outcomes.
In the medical field, AI has many applications, followed by significant advances in a relatively short period of time (86). ML is one of the fastest and most convenient methods to detect diseases through AI techniques (87, 88). The synergy between ML and medicine has transformed disease prevention and treatment (89). Given the sheer volume and complexity of clinical data generated today, diagnostic algorithms based solely on physician clinical experience or traditional statistical methods often fail to deliver superior performance. ML, as part of artificial intelligence, has strong learning capabilities and predictive performance, the ability to process large cubes, identify patterns and correlations that may not be apparent using traditional statistical methods, and objectively analyze large amounts of data to predict clinical outcomes, which can improve diagnostic accuracy and predict complex medical conditions (90, 91). PE (PE) is a major health problem, ranking third among cardiovascular diseases (92). By analyzing medical image data, AI-based applications can effectively extract important features from images, enhance their utility in medical image analysis, identify potential missed PE, improve the accuracy and efficiency of PE diagnosis, and improve patient outcomes through timely intervention (93–96). Therefore, this article summarizes the application performance of AI in the optimization of clinical decision-making of PE, which has significant advantages in the early prediction of PE, timely screening and diagnosis, and improved prognosis. The development of predictive models with strong generalization capabilities to assess the risk of PE in patients using ML algorithms has great potential for development (97). However, rigorous implementation and validation are essential to ensure the safety and efficacy of these techniques in clinical practice.
YuL: Writing – original draft, Writing – review & editing. LZ: Data curation, Software, Writing – review & editing. HL: Investigation, Writing – review & editing. YaL: Data curation, Investigation, Methodology, Writing – review & editing. ZL: Conceptualization, Data curation, Investigation, Writing – review & editing.
The author(s) declare that financial support was received for the research and/or publication of this article. This work was supported by the Liaoning Provincial Department of Education Basic Research Project for Higher Education Institutions [Liao Jiao Tong (2024) No. 341] and the Dalian Science and Technology Innovation Fund [Da ke fa (2023) No. 209].
The authors declare that the research was conducted in the absence of any commercial or financial relationships that could be construed as a potential conflict of interest.
The authors declare that no Gen AI was used in the creation of this manuscript.
All claims expressed in this article are solely those of the authors and do not necessarily represent those of their affiliated organizations, or those of the publisher, the editors and the reviewers. Any product that may be evaluated in this article, or claim that may be made by its manufacturer, is not guaranteed or endorsed by the publisher.
1. Wang, Y, Qiu, L, Yu, D, Yu, Y, Hu, L, and Gu, Y. Effects and related mechanism of alpha-adrenergic receptor inhibitor phentolamine in a rabbit model of acute pulmonary embolism combined with shock. Eur J Med Res. (2022) 27:238. doi: 10.1186/s40001-022-00842-5
2. Wittram, C, Maher, MM, Yoo, AJ, Kalra, MK, Shepard, JA, and McLoud, TC. CT angiography of pulmonary embolism: diagnostic criteria and causes of misdiagnosis. Radiographics. (2004) 24:1219–38. doi: 10.1148/rg.245045008
3. Lucassen, WA, Beenen, LF, Buller, HR, Erkens, PM, Schaefer-Prokop, CM, van den Berk, IA, et al. Concerns in using multi-detector computed tomography for diagnosing pulmonary embolism in daily practice. A cross-sectional analysis using expert opinion as reference standard. Thromb Res. (2013) 131:145–9. doi: 10.1016/j.thromres.2012.11.027
4. Righini, M, Robert-Ebadi, H, and Le Gal, G. Diagnosis of acute pulmonary embolism. J Thromb Haemost. (2017) 15:1251–61. doi: 10.1111/jth.13694
5. Freund, Y, Cohen-Aubart, F, and Bloom, B. Acute pulmonary embolism: a review. JAMA. (2022) 328:1336–45. doi: 10.1001/jama.2022.16815
6. Konstantinides, SV, Meyer, G, Becattini, C, Bueno, H, Geersing, GJ, Harjola, VP, et al. 2019 ESC guidelines for the diagnosis and management of acute pulmonary embolism developed in collaboration with the European Respiratory Society (ERS). Eur Heart J. (2020) 41:543–603. doi: 10.1093/eurheartj/ehz405
7. Barco, S, Valerio, L, Ageno, W, Cohen, AT, Goldhaber, SZ, Hunt, BJ, et al. Age-sex specific pulmonary embolism-related mortality in the USA and Canada, 2000-18: an analysis of the WHO mortality database and of the CDC multiple cause of death database. Lancet Respir Med. (2021) 9:33–42. doi: 10.1016/S2213-2600(20)30417-3
8. Peris, M, Lopez-Nunez, JJ, Maestre, A, Jimenez, D, Muriel, A, Bikdeli, B, et al. Clinical characteristics and 3-month outcomes in cancer patients with incidental versus clinically suspected and confirmed pulmonary embolism. Eur Respir J. (2021) 58:2002723. doi: 10.1183/13993003.02723-2020
9. Visco, V, Ferruzzi, GJ, Nicastro, F, Virtuoso, N, Carrizzo, A, Galasso, G, et al. Artificial intelligence as a business partner in cardiovascular precision medicine: An emerging approach for disease detection and treatment optimization. Curr Med Chem. (2021) 28:6569–90. doi: 10.2174/0929867328666201218122633
10. Sun, X, Yin, Y, Yang, Q, and Huo, T. Artificial intelligence in cardiovascular diseases: diagnostic and therapeutic perspectives. Eur J Med Res. (2023) 28:242. doi: 10.1186/s40001-023-01065-y
11. Xue, B, Li, D, Lu, C, King, CR, Wildes, T, Avidan, MS, et al. Use of machine learning to develop and evaluate models using preoperative and intraoperative data to identify risks of postoperative complications. JAMA Netw Open. (2021) 4:e212240. doi: 10.1001/jamanetworkopen.2021.2240
12. Vollmer, S. Machine learning and artificial intelligence research for patient benefit: 20 critical questions on transparency, replicability, ethics, and effectiveness. BMJ. (2024) 387:q2390. doi: 10.1136/bmj.q2390
13. Stamate, E, Piraianu, AI, Ciobotaru, OR, Crassas, R, Duca, O, Fulga, A, et al. Revolutionizing cardiology through artificial intelligence-big data from proactive prevention to precise diagnostics and cutting-edge treatment-a comprehensive review of the past 5 years. Diagnostics. (2024) 14:1103. doi: 10.3390/diagnostics14111103
14. Badrick, T, Banfi, G, Bietenbeck, A, Cervinski, MA, Loh, TP, and Sikaris, K. Machine learning for clinical chemists. Clin Chem. (2019) 65:1350–6. doi: 10.1373/clinchem.2019.307512
15. Van Calster, B, and Wynants, L. Machine learning in medicine. N Engl J Med. (2019) 380:2588–90. doi: 10.1056/NEJMc1906060
16. Peiffer-Smadja, N, Rawson, TM, Ahmad, R, Buchard, A, Georgiou, P, Lescure, FX, et al. Machine learning for clinical decision support in infectious diseases: a narrative review of current applications. Clin Microbiol Infect. (2020) 26:584–95. doi: 10.1016/j.cmi.2019.09.009
17. Greener, JG, Kandathil, SM, Moffat, L, and Jones, DT. A guide to machine learning for biologists. Nat Rev Mol Cell Biol. (2022) 23:40–55. doi: 10.1038/s41580-021-00407-0
18. Sultan, AS, Elgharib, MA, Tavares, T, Jessri, M, and Basile, JR. The use of artificial intelligence, machine learning and deep learning in oncologic histopathology. J Oral Pathol Med. (2020) 49:849–56. doi: 10.1111/jop.13042
19. Lemke, HU. AI based IT system design and applications for a wisdom-oriented health care system. Int J Comput Assist Radiol Surg. (2023) 18:1543–6. doi: 10.1007/s11548-023-03009-7
20. Lemke, HU. Model guided medicine and the search for truth. Int J Comput Assist Radiol Surg. (2024) 19:601–7. doi: 10.1007/s11548-024-03097-z
21. Weber, GM, Mandl, KD, and Kohane, IS. Finding the missing link for big biomedical data. JAMA. (2014) 311:2479–80. doi: 10.1001/jama.2014.4228
22. Gulshan, V, Peng, L, Coram, M, Stumpe, MC, Wu, D, Narayanaswamy, A, et al. Development and validation of a deep learning algorithm for detection of diabetic retinopathy in retinal fundus photographs. JAMA. (2016) 316:2402–10. doi: 10.1001/jama.2016.17216
23. Cowan, IA, MacDonald, SL, and Floyd, RA. Measuring and managing radiologist workload: measuring radiologist reporting times using data from a radiology information system. J Med Imaging Radiat Oncol. (2013) 57:558–66. doi: 10.1111/1754-9485.12092
24. Beam, AL, and Kohane, IS. Big data and machine learning in health care. JAMA. (2018) 319:1317–8. doi: 10.1001/jama.2017.18391
25. Feng, S, Wang, J, Wang, L, Qiu, Q, Chen, D, Su, H, et al. Current status and analysis of machine learning in hepatocellular carcinoma. J Clin Transl Hepatol. (2023):000–91. doi: 10.14218/JCTH.2022.00077S
26. Villacorta, H, Pickering, JW, Horiuchi, Y, Olim, M, Coyne, C, Maisel, AS, et al. Machine learning with D-dimer in the risk stratification for pulmonary embolism: a derivation and internal validation study. Eur Heart J Acute Cardiovasc Care. (2022) 11:13–9. doi: 10.1093/ehjacc/zuab089
27. Zhou, Q, Huang, R, Xiong, X, Liang, Z, and Zhang, W. Prediction of pulmonary embolism by an explainable machine learning approach in the real world. Sci Rep. (2025) 15:835. doi: 10.1038/s41598-024-75435-9
28. Yazici, S, Kiris, T, Emre, A, Ceylan, US, Akyuz, S, Uzun, AO, et al. Relation of contrast nephropathy to adverse events in pulmonary emboli patients diagnosed with contrast CT. Am J Emerg Med. (2016) 34:1247–50. doi: 10.1016/j.ajem.2016.03.053
29. Williams, LS, Walker, GR, Loewenherz, JW, and Gidel, LT. Association of Contrast and Acute Kidney Injury in the critically ill: a propensity-matched study. Chest. (2020) 157:866–76. doi: 10.1016/j.chest.2019.10.005
30. Banerjee, I, Sofela, M, Yang, J, Chen, JH, Shah, NH, Ball, R, et al. Development and performance of the pulmonary embolism result forecast model (PERFORM) for computed tomography clinical decision support. JAMA Netw Open. (2019) 2:e198719. doi: 10.1001/jamanetworkopen.2019.8719
31. Chen, T, and Guestrinn, C. Boost: a scalable tree boosting system. Proceedings of the 22nd ACM SIGKDD International Conference on Knowledge Discovery and Data Mining. New York, NY, USA: Association for Computing Machinery, pp. 785–794. doi: 10.1145/2939672.2939785
32. Shen, J, Casie Chetty, S, Shokouhi, S, Maharjan, J, Chuba, Y, Calvert, J, et al. Massive external validation of a machine learning algorithm to predict pulmonary embolism in hospitalized patients. Thromb Res. (2022) 216:14–21. doi: 10.1016/j.thromres.2022.05.016
33. Chowdhury, JM, Brown, P, and Kasarabada, A. Risk stratification of pulmonary embolism. Curr Opin Pulm Med. (2023) 29:363–9. doi: 10.1097/MCP.0000000000000998
34. Aujesky, D, Obrosky, DS, Stone, RA, Auble, TE, Perrier, A, Cornuz, J, et al. Derivation and validation of a prognostic model for pulmonary embolism. Am J Respir Crit Care Med. (2005) 172:1041–6. doi: 10.1164/rccm.200506-862OC
35. Johnson, A, Bulgarelli, L, Pollard, T, Horng, S, Celi, LA, and Mark, R. MIMIC-IV (version 2.2). PhysioNet. (2023). doi: 10.13026/6mm1-ek67
36. Hariharan, P, Takayesu, JK, and Kabrhel, C. Association between the pulmonary embolism severity index (PESI) and short-term clinical deterioration. Thromb Haemost. (2011) 105:706–11. doi: 10.1160/TH10-09-0577
37. Liu, J, Li, R, Yao, T, Liu, G, Guo, L, He, J, et al. Interpretable machine learning approach for predicting 30-day mortality of critical ill patients with pulmonary embolism and heart failure: a retrospective study. Clin Appl Thromb Hemost. (2024) 30:10760296241304764. doi: 10.1177/10760296241304764
38. Liu, D, Shi, S, Liu, X, Ye, T, Wang, L, Qu, C, et al. Retrospective cohort study of new-onset atrial fibrillation in acute pulmonary embolism on prognosis. BMJ Open. (2021) 11:e047658. doi: 10.1136/bmjopen-2020-047658
39. Ren, J, Wang, H, Lai, S, Shao, Y, Che, H, Xue, Z, et al. Machine learning-based model to predict composite thromboembolic events among Chinese elderly patients with atrial fibrillation. BMC Cardiovasc Disord. (2024) 24:420. doi: 10.1186/s12872-024-04082-9
40. Kong, H, Li, Y, Shen, Y, Pan, J, Liang, M, Geng, Z, et al. Predicting the risk of pulmonary embolism in patients with tuberculosis using machine learning algorithms. Eur J Med Res. (2024) 29:618. doi: 10.1186/s40001-024-02218-3
41. Zoller, B, Li, X, Sundquist, J, and Sundquist, K. Risk of pulmonary embolism in patients with autoimmune disorders: a nationwide follow-up study from Sweden. Lancet. (2012) 379:244–9. doi: 10.1016/S0140-6736(11)61306-8
42. Hu, Z, Hu, Y, Zhang, S, Dong, L, Chen, X, Yang, H, et al. Machine-learning-based models assist the prediction of pulmonary embolism in autoimmune diseases: a retrospective, multicenter study. Chin Med J. (2024) 137:1811–22. doi: 10.1097/CM9.0000000000003025
43. Smith, SB, Geske, JB, Maguire, JM, Zane, NA, Carter, RE, and Morgenthaler, TI. Early anticoagulation is associated with reduced mortality for acute pulmonary embolism. Chest. (2010) 137:1382–90. doi: 10.1378/chest.09-0959
44. Lefevre-Scelles, A, Jeanmaire, P, Freund, Y, Joly, LM, Phillipon, AL, and Roussel, M. Investigation of pulmonary embolism in patients with chest pain in the emergency department: a retrospective multicenter study. Eur J Emerg Med. (2020) 27:357–61. doi: 10.1097/MEJ.0000000000000680
45. Freund, Y, Chauvin, A, Jimenez, S, Philippon, AL, Curac, S, Femy, F, et al. Effect of a diagnostic strategy using an elevated and age-adjusted D-dimer threshold on thromboembolic events in emergency department patients with suspected pulmonary embolism: a randomized clinical trial. JAMA. (2021) 326:2141–9. doi: 10.1001/jama.2021.20750
46. Di Nisio, M, van Es, N, and Buller, HR. Deep vein thrombosis and pulmonary embolism. Lancet. (2016) 388:3060–73. doi: 10.1016/S0140-6736(16)30514-1
47. Rali, PM, and Criner, GJ. Submassive pulmonary embolism. Am J Respir Crit Care Med. (2018) 198:588–98. doi: 10.1164/rccm.201711-2302CI
48. Millington, SJ, Aissaoui, N, Bowcock, E, Brodie, D, Burns, KEA, Doufle, G, et al. High and intermediate risk pulmonary embolism in the ICU. Intensive Care Med. (2024) 50:195–208. doi: 10.1007/s00134-023-07275-6
49. Amin, KD, Weissler, EH, Ratliff, W, Sullivan, AE, Holder, TA, Bury, C, et al. Development and validation of a natural language processing model to identify low-risk pulmonary embolism in real time to facilitate safe outpatient management. Ann Emerg Med. (2024) 84:118–27. doi: 10.1016/j.annemergmed.2024.01.036
50. Root, CW, Dudzinski, DM, Zakhary, B, Friedman, OA, Sista, AK, and Horowitz, JM. Multidisciplinary approach to the management of pulmonary embolism patients: the pulmonary embolism response team (PERT). J Multidiscip Healthc. (2018) 11:187–95. doi: 10.2147/JMDH.S151196
51. Roussel, M, Bloom, B, Taalba, M, Choquet, C, Douillet, D, Femy, F, et al. Temporal trends in the use of computed tomographic pulmonary angiography for suspected pulmonary embolism in the emergency department: a retrospective analysis. Ann Intern Med. (2023) 176:761–8. doi: 10.7326/M22-3116
52. Batra, K, Xi, Y, Al-Hreish, KM, Kay, FU, Browning, T, Baker, C, et al. Detection of incidental pulmonary embolism on conventional contrast-enhanced chest CT: comparison of an artificial intelligence algorithm and clinical reports. AJR Am J Roentgenol. (2022) 219:895–902. doi: 10.2214/AJR.22.27895
53. Graeve, VIJ, Laures, S, Spirig, A, Zaytoun, H, Gregoriano, C, Schuetz, P, et al. Implementation of an AI algorithm in clinical practice to reduce missed incidental pulmonary embolisms on chest CT and its impact on short-term survival. Investig Radiol. (2024) 60:260–6. doi: 10.1097/RLI.0000000000001122
54. Petry, M, Lansky, C, Chodakiewitz, Y, Maya, M, and Pressman, B. Decreased hospital length of stay for ICH and PE after adoption of an artificial intelligence-augmented radiological worklist triage system. Radiol Res Pract. (2022) 2022:2141839–7. doi: 10.1155/2022/2141839
55. Langius-Wiffen, E, de Jong, PA, Hoesein, FAM, Dekker, L, van den Hoven, AF, Nijholt, IM, et al. Retrospective batch analysis to evaluate the diagnostic accuracy of a clinically deployed AI algorithm for the detection of acute pulmonary embolism on CTPA. Insights Imaging. (2023) 14:102. doi: 10.1186/s13244-023-01454-1
56. Belkouchi, Y, Lederlin, M, Ben Afia, A, Fabre, C, Ferretti, G, De Margerie, C, et al. Detection and quantification of pulmonary embolism with artificial intelligence: the SFR 2022 artificial intelligence data challenge. Diagn Interv Imaging. (2023) 104:485–9. doi: 10.1016/j.diii.2023.05.007
57. Jin, ZG, Zhang, H, Tai, MH, Yang, Y, Yao, Y, and Guo, YT. Natural language processing in a clinical decision support system for the identification of venous thromboembolism: algorithm development and validation. J Med Internet Res. (2023) 25:e43153. doi: 10.2196/43153
58. Huang, SC, Kothari, T, Banerjee, I, Chute, C, Ball, RL, Borus, N, et al. PENet-a scalable deep-learning model for automated diagnosis of pulmonary embolism using volumetric CT imaging. NPJ Digit Med. (2020) 3:61. doi: 10.1038/s41746-020-0266-y
59. Hansen, SL, de Nijs, R, Mortensen, J, and Berg, RMG. Ventilation-perfusion SPECT versus CTPA in young adult females with suspected pulmonary embolism. Eur Respir J. (2020) 55:2000448. doi: 10.1183/13993003.00448-2020
60. Li, Z, Zhao, M, Li, Z, Huang, YH, Chen, Z, Pu, Y, et al. Quantitative texture analysis using machine learning for predicting interpretable pulmonary perfusion from non-contrast computed tomography in pulmonary embolism patients. Respir Res. (2024) 25:389. doi: 10.1186/s12931-024-03004-9
61. Topff, L, Ranschaert, ER, Bartels-Rutten, A, Negoita, A, Menezes, R, Beets-Tan, RGH, et al. Artificial intelligence tool for detection and worklist prioritization reduces time to diagnosis of incidental pulmonary embolism at CT. Radiol Cardiothorac Imaging. (2023) 5:e220163. doi: 10.1148/ryct.220163
62. Wiklund, P, and Medson, K. Use of a deep learning algorithm for detection and triage of Cancer-associated incidental pulmonary embolism. Radiol Artif Intell. (2023) 5:e220286. doi: 10.1148/ryai.220286
63. Wu, H, Xu, Q, He, X, Xu, H, Wang, Y, and Guo, L. SPE-YOLO: a deep learning model focusing on small pulmonary embolism detection. Comput Biol Med. (2025) 184:109402. doi: 10.1016/j.compbiomed.2024.109402
64. Ben Yehuda, O, Itelman, E, Vaisman, A, Segal, G, and Lerner, B. Early detection of pulmonary embolism in a general patient population immediately upon hospital admission using machine learning to identify new, unidentified risk factors: model development study. J Med Internet Res. (2024) 26:e48595. doi: 10.2196/48595
65. Rothenberg, SA, Savage, CH, Abou Elkassem, A, Singh, S, Abozeed, M, Hamki, O, et al. Prospective evaluation of AI triage of pulmonary emboli on CT pulmonary angiograms. Radiology. (2023) 309:e230702. doi: 10.1148/radiol.230702
66. Langius-Wiffen, E, Nijholt, IM, de Boer, E, Nijboer-Oosterveld, J, Huurman, L, Rozema, I, et al. Computer-aided pulmonary embolism detection on virtual monochromatic images compared to conventional CT angiography. Radiology. (2021) 301:420–2. doi: 10.1148/radiol.2021204620
67. Somani, SS, Honarvar, H, Narula, S, Landi, I, Lee, S, Khachatoorian, Y, et al. Development of a machine learning model using electrocardiogram signals to improve acute pulmonary embolism screening. Eur Heart J Digit Health. (2022) 3:56–66. doi: 10.1093/ehjdh/ztab101
68. Wiklund, P, Medson, K, and Elf, J. Incidental pulmonary embolism in patients with cancer: prevalence, underdiagnosis and evaluation of an AI algorithm for automatic detection of pulmonary embolism. Eur Radiol. (2023) 33:1185–93. doi: 10.1007/s00330-022-09071-0
69. Liu, L, Li, Y, Liu, N, Luo, J, Deng, J, Peng, W, et al. Establishment of machine learning-based tool for early detection of pulmonary embolism. Comput Methods Prog Biomed. (2024) 244:107977. doi: 10.1016/j.cmpb.2023.107977
70. Wang, G, Xu, J, Lin, X, Lai, W, Lv, L, Peng, S, et al. Machine learning-based models for predicting mortality and acute kidney injury in critical pulmonary embolism. BMC Cardiovasc Disord. (2023) 23:385. doi: 10.1186/s12872-023-03363-z
71. Lian, Z, Wei, XN, and Chai, D. Machine learning-based prediction of pulmonary embolism prognosis using nutritional and inflammatory indices. Clin Appl Thromb Hemost. (2024) 30:10760296241300484. doi: 10.1177/10760296241300484
72. Sadegh-Zadeh, SA, Sakha, H, Movahedi, S, Fasihi Harandi, A, Ghaffari, S, Javanshir, E, et al. Advancing prognostic precision in pulmonary embolism: a clinical and laboratory-based artificial intelligence approach for enhanced early mortality risk stratification. Comput Biol Med. (2023) 167:107696. doi: 10.1016/j.compbiomed.2023.107696
73. Kearon, C, Akl, EA, Ornelas, J, Blaivas, A, Jimenez, D, Bounameaux, H, et al. Antithrombotic therapy for VTE disease: CHEST guideline and expert panel report. Chest. (2016) 149:315–52. doi: 10.1016/j.chest.2015.11.026
74. Bikdeli, B, Jimenez, D, Hawkins, M, Ortiz, S, Prandoni, P, Brenner, B, et al. Rationale, design and methodology of the computerized registry of patients with venous thromboembolism (RIETE). Thromb Haemost. (2018) 118:214–24. doi: 10.1160/TH17-07-0511
75. Mora, D, Nieto, JA, Mateo, J, Bikdeli, B, Barco, S, Trujillo-Santos, J, et al. Machine learning to predict outcomes in patients with acute pulmonary embolism who prematurely discontinued anticoagulant therapy. Thromb Haemost. (2022) 122:570–7. doi: 10.1055/a-1525-7220
76. Munoz, AJ, Souto, JC, Lecumberri, R, Obispo, B, Sanchez, A, Aparicio, J, et al. Development of a predictive model of venous thromboembolism recurrence in anticoagulated cancer patients using machine learning. Thromb Res. (2023) 228:181–8. doi: 10.1016/j.thromres.2023.06.015
77. An, C, Park, YW, Ahn, SS, Han, K, Kim, H, and Lee, SK. Radiomics machine learning study with a small sample size: single random training-test set split may lead to unreliable results. PLoS One. (2021) 16:e0256152. doi: 10.1371/journal.pone.0256152
78. Vrudhula, A, Kwan, AC, Ouyang, D, and Cheng, S. Machine learning and Bias in medical imaging: opportunities and challenges. Circ Cardiovasc Imaging. (2024) 17:e015495. doi: 10.1161/CIRCIMAGING.123.015495
79. Zhang, L, Xu, J, Li, Y, Meng, F, and Wang, W. Smoking on the risk of acute respiratory distress syndrome: a systematic review and meta-analysis. Crit Care. (2024) 28:122. doi: 10.1186/s13054-024-04902-6
80. Cheikh, AB, Gorincour, G, Nivet, H, May, J, Seux, M, Calame, P, et al. How artificial intelligence improves radiological interpretation in suspected pulmonary embolism. Eur Radiol. (2022) 32:5831–42. doi: 10.1007/s00330-022-08645-2
81. Zhong, Z, Li, J, Kulkarni, S, Zhang, H, Fayad, FH, Li, Y, et al. De-biased disentanglement learning for pulmonary embolism survival prediction on multimodal data. IEEE J Biomed Health Inform. (2024) 28:3732–41. doi: 10.1109/JBHI.2024.3384848
82. Daidone, M, Ferrantelli, S, and Tuttolomondo, A. Machine learning applications in stroke medicine: advancements, challenges, and future prospectives. Neural Regen Res. (2024) 19:769–73. doi: 10.4103/1673-5374.382228
83. O’Reilly-Shah, VN, Gentry, KR, Walters, AM, Zivot, J, Anderson, CT, and Tighe, PJ. Bias and ethical considerations in machine learning and the automation of perioperative risk assessment. Br J Anaesth. (2020) 125:843–6. doi: 10.1016/j.bja.2020.07.040
84. McCabe, BE, Veselis, CA, Goykhman, I, Hochhold, J, Eisenberg, D, and Son, H. Beyond pulmonary embolism; nonthrombotic pulmonary embolism as diagnostic challenges. Curr Probl Diagn Radiol. (2019) 48:387–92. doi: 10.1067/j.cpradiol.2018.07.007
85. Asah, D, Raju, S, Ghosh, S, Mukhopadhyay, S, and Mehta, AC. Nonthrombotic pulmonary embolism from inorganic particulate matter and foreign bodies. Chest. (2018) 153:1249–65. doi: 10.1016/j.chest.2018.02.013
86. Miller, DD, and Brown, EW. Artificial intelligence in medical practice: the question to the answer? Am J Med. (2018) 131:129–33. doi: 10.1016/j.amjmed.2017.10.035
87. Goodswen, SJ, Barratt, JLN, Kennedy, PJ, Kaufer, A, Calarco, L, and Ellis, JT. Machine learning and applications in microbiology. FEMS Microbiol Rev. (2021) 45:fuab015. doi: 10.1093/femsre/fuab015
88. Obermeyer, Z, and Emanuel, EJ. Predicting the future - big data, machine learning, and clinical medicine. N Engl J Med. (2016) 375:1216–9. doi: 10.1056/NEJMp1606181
89. Haug, CJ, and Drazen, JM. Artificial intelligence and machine learning in clinical medicine, 2023. N Engl J Med. (2023) 388:1201–8. doi: 10.1056/NEJMra2302038
90. Darze, ES, Latado, AL, Guimaraes, AG, Guedes, RA, Santos, AB, de Moura, SS, et al. Incidence and clinical predictors of pulmonary embolism in severe heart failure patients admitted to a coronary care unit. Chest. (2005) 128:2576–80. doi: 10.1378/chest.128.4.2576
91. Li, W, Hong, T, Liu, W, Dong, S, Wang, H, Tang, ZR, et al. Development of a machine learning-based predictive model for lung metastasis in patients with Ewing sarcoma. Front Med. (2022) 9:807382. doi: 10.3389/fmed.2022.807382
92. Goldhaber, SZ, and Bounameaux, H. Pulmonary embolism and deep vein thrombosis. Lancet. (2012) 379:1835–46. doi: 10.1016/S0140-6736(11)61904-1
93. Wildman-Tobriner, B, Ngo, L, Mammarappallil, JG, Konkel, B, Johnson, JM, and Bashir, MR. Missed incidental pulmonary embolism: harnessing artificial intelligence to assess prevalence and improve quality improvement opportunities. J Am Coll Radiol. (2021) 18:992–9. doi: 10.1016/j.jacr.2021.01.014
94. Langius-Wiffen, E, de Jong, PA, Mohamed Hoesein, FA, Dekker, L, van den Hoven, AF, Nijholt, IM, et al. Added value of an artificial intelligence algorithm in reducing the number of missed incidental acute pulmonary embolism in routine portal venous phase chest CT. Eur Radiol. (2024) 34:367–73. doi: 10.1007/s00330-023-10029-z
95. Lee, T. Editorial comment: enhancing radiologist sensitivity for incidental pulmonary embolism detection with artificial intelligence-a prospective validation study. AJR Am J Roentgenol. (2024) 223:e2431668. doi: 10.2214/AJR.24.31668
96. Topol, EJ. Toward the eradication of medical diagnostic errors. Science. (2024) 383:eadn9602. doi: 10.1126/science.adn9602
Keywords: artificial intelligence, machine learning, pulmonary embolism, prediction, diagnosis, prognosis
Citation: Li Y, Zhang L, Liu H, Li Y and Liu Z (2025) Research progress of artificial intelligence and machine learning in pulmonary embolism. Front. Med. 12:1577559. doi: 10.3389/fmed.2025.1577559
Received: 16 February 2025; Accepted: 17 March 2025;
Published: 27 March 2025.
Edited by:
Liang Zhao, Dalian University of Technology, ChinaCopyright © 2025 Li, Zhang, Liu, Li and Liu. This is an open-access article distributed under the terms of the Creative Commons Attribution License (CC BY). The use, distribution or reproduction in other forums is permitted, provided the original author(s) and the copyright owner(s) are credited and that the original publication in this journal is cited, in accordance with accepted academic practice. No use, distribution or reproduction is permitted which does not comply with these terms.
*Correspondence: Zhuo Liu, bHpodW8wMzEwQDEyNi5jb20=
Disclaimer: All claims expressed in this article are solely those of the authors and do not necessarily represent those of their affiliated organizations, or those of the publisher, the editors and the reviewers. Any product that may be evaluated in this article or claim that may be made by its manufacturer is not guaranteed or endorsed by the publisher.
Research integrity at Frontiers
Learn more about the work of our research integrity team to safeguard the quality of each article we publish.