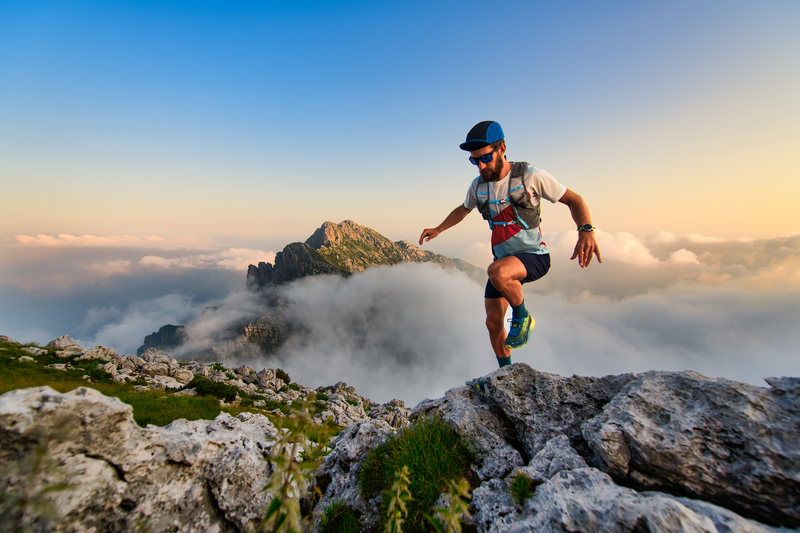
94% of researchers rate our articles as excellent or good
Learn more about the work of our research integrity team to safeguard the quality of each article we publish.
Find out more
ORIGINAL RESEARCH article
Front. Med.
Sec. Gastroenterology
Volume 12 - 2025 | doi: 10.3389/fmed.2025.1512173
The final, formatted version of the article will be published soon.
You have multiple emails registered with Frontiers:
Please enter your email address:
If you already have an account, please login
You don't have a Frontiers account ? You can register here
Background: Fatty liver is characterized by hepatic steatosis and is associated with dyslipidemia and insulin resistance. Carotid atherosclerosis, characterized by plaque formation, may be related to increased lipid deposition. High-density lipoprotein cholesterol (HDL-C) plays a role in reverse cholesterol transport. Colorectal cancer (CRC) is significantly associated with lipid metabolismrelated diseases. However, there is a paucity of research on the relationship between lipid metabolism disorders and CRC.Objective: To determine whether fatty liver (F), carotid atherosclerosis (A), and HDL-C (H) models (FAH) have predictive value for the occurrence of CRC and can be used for CRC screening.A case-control study was conducted on 166 patients with CRC and 448 patients who underwent physical examinations at Ziyang People's Hospital between September 2018 and August 2023. A 1:3 individual matching strategy was used to establish the independent risk factors for CRC using univariate and multivariate analyses. A model was constructed based on independent risk factors, and its accuracy and sensitivity were verified. The discriminative ability, calibration, and clinical utility of the predictive model were evaluated using the Receiver Operating Characteristic curve, bootstrap resampling method, the Hosmer-Lemeshow goodness-of-fit test, and Decision Curve Analysis (DCA).Results: Fatty liver (F), carotid atherosclerosis (A), HDL-C (H), and intestinal dysbiosis (D) were identified as independent risk factors for CRC. The odds ratios were 2. 885, 11.452, 24.659, and 22.445, respectively, P<0.001. Based on these results, an FAH prediction model was established. The Horser-Lemeshow test for the FAH prediction model yielded P=0.710. The cut-off value was 0.275, with the area under the curve of 0.902 (95% Confidence Interval: 0.875-0.929), P<0.001. The sensitivity was 86.7%, and the specificity was 78.1%. A nomogram was created, and the internal calibration chart showed that the calibration curve closely aligned with the standard curve, indicating good discrimination and predictive ability of the model. DCA demonstrated that the model had a favorable clinical net benefit.The FAH model has predictive value for CRC occurrence owing to its noninvasive nature and easy availability of data, making it worthy of further clinical research.
Keywords: colorectal cancer, Fatty Liver, carotid atherosclerosis, High-density lipoprotein cholesterol, Intestinal dysbiosis
Received: 16 Oct 2024; Accepted: 24 Feb 2025.
Copyright: © 2025 Ma, Wu and Wu. This is an open-access article distributed under the terms of the Creative Commons Attribution License (CC BY). The use, distribution or reproduction in other forums is permitted, provided the original author(s) or licensor are credited and that the original publication in this journal is cited, in accordance with accepted academic practice. No use, distribution or reproduction is permitted which does not comply with these terms.
* Correspondence:
Qingming Wu, School of Public Health, Wuhan University of Science and Technology, Wuhan, 430065, Hebei Province, China
Disclaimer: All claims expressed in this article are solely those of the authors and do not necessarily represent those of their affiliated organizations, or those of the publisher, the editors and the reviewers. Any product that may be evaluated in this article or claim that may be made by its manufacturer is not guaranteed or endorsed by the publisher.
Research integrity at Frontiers
Learn more about the work of our research integrity team to safeguard the quality of each article we publish.