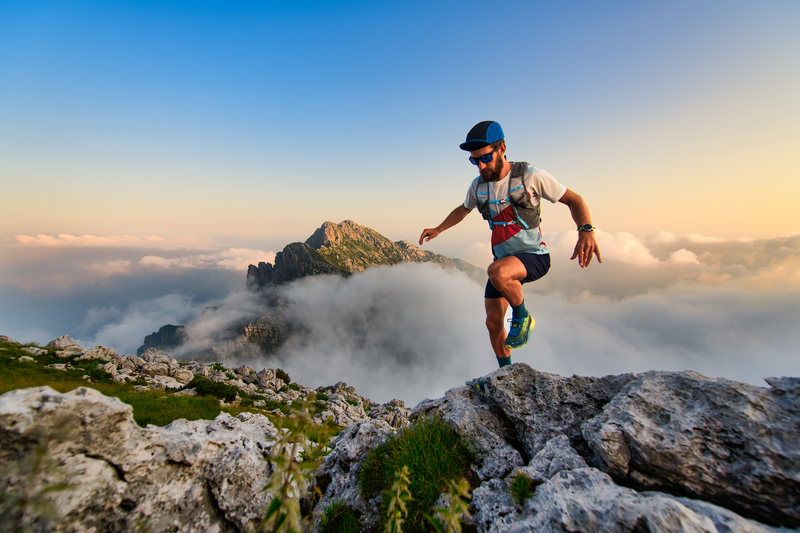
94% of researchers rate our articles as excellent or good
Learn more about the work of our research integrity team to safeguard the quality of each article we publish.
Find out more
ORIGINAL RESEARCH article
Front. Med.
Sec. Gastroenterology
Volume 12 - 2025 | doi: 10.3389/fmed.2025.1502315
The final, formatted version of the article will be published soon.
You have multiple emails registered with Frontiers:
Please enter your email address:
If you already have an account, please login
You don't have a Frontiers account ? You can register here
This study sought to clarify the utility of T2-weighted imaging (T2WI)-based radiomics to predict the recurrence of acute pancreatitis (AP) in subjects with metabolic syndrome (MetS). Methods: Data from 196 patients with both AP and MetS from our hospital were retrospectively analyzed. These patients were separated into two groups according to their clinical follow-up outcomes, including those with first-onset AP (n=114) and those with recurrent AP (RAP) (n=82).The 196 cases were randomly divided into a training (n=137) and test sets (n=59) at a 7:3 ratio. The clinical characteristics of these patients were systematically compiled for further analysis. For each case, the pancreatic parenchyma was manually delineated slice by slice using 3D Slicer software, and the appropriate radiomics characteristics were retrieved. The K-best approach, the least absolute shrinkage and selection operator (LASSO) algorithm, and variance thresholding were all used in the feature selection process. The establishment of clinical, radiomics, and combined models for forecasting AP recurrence in patients with MetS was then done using a random forest classifier. Model performance was measured using the area under the receiver operating characteristic curve (AUC), and model comparison was done using the DeLong test. The clinical utility of these models was evaluated using decision curve analysis (DCA), and the optimal model was determined via a calibration curve.: In the training set, the clinical, radiomics, and combined models yielded respective AUCs of 0.651, 0.825, and 0.883, with corresponding test sets of AUCs of 0.606, 0.776, and 0.878. Both the radiomics and combined models exhibited superior predictive effectiveness compared to the clinical model in both the training (P=0.001, P<0.001) and test sets (P=0.04, P<0.001). The combined model outperformed the radiomics model (training set: P=0.025, test set: P=0.019). The DCA demonstrated that the radiomics and combined models had greater clinical efficacy than the clinical model. The calibration curve for the combined model demonstrated good agreement between the predicted probability of AP recurrence and the observed outcomes. Conclusion: These findings highlight the superior predictive power of a T2WI-based radiomics model for predicting AP recurrence in patients with MetS, potentially supporting early interventions that can mitigate or alleviate RAP.
Keywords: acute pancreatitis, metabolic syndrome, Recurrence, Magnetic Resonance Imaging, Radiomics
Received: 01 Oct 2024; Accepted: 24 Feb 2025.
Copyright: © 2025 Wang, Wan, Liu, Liu and Huang. This is an open-access article distributed under the terms of the Creative Commons Attribution License (CC BY). The use, distribution or reproduction in other forums is permitted, provided the original author(s) or licensor are credited and that the original publication in this journal is cited, in accordance with accepted academic practice. No use, distribution or reproduction is permitted which does not comply with these terms.
* Correspondence:
Xiao-hua Huang, Department of Radiology, Affiliated Hospital of North Sichuan Medical College, Nanchong, Sichuan Province, China
Disclaimer: All claims expressed in this article are solely those of the authors and do not necessarily represent those of their affiliated organizations, or those of the publisher, the editors and the reviewers. Any product that may be evaluated in this article or claim that may be made by its manufacturer is not guaranteed or endorsed by the publisher.
Research integrity at Frontiers
Learn more about the work of our research integrity team to safeguard the quality of each article we publish.