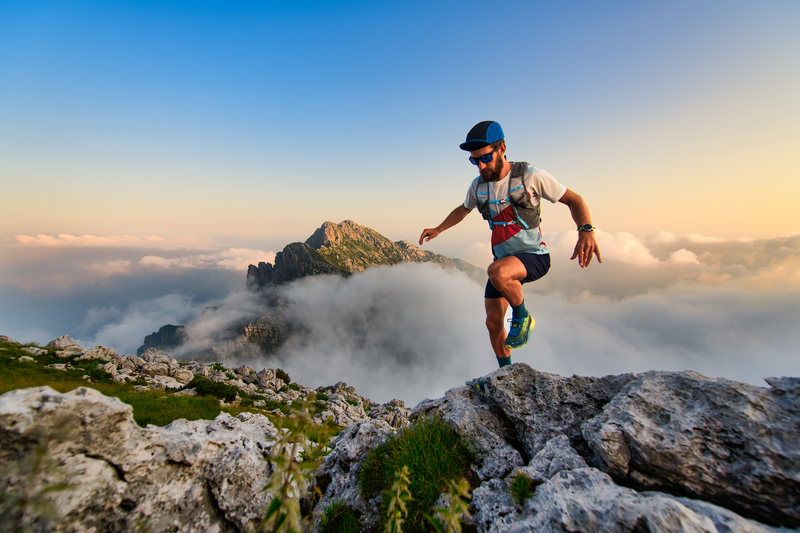
94% of researchers rate our articles as excellent or good
Learn more about the work of our research integrity team to safeguard the quality of each article we publish.
Find out more
ORIGINAL RESEARCH article
Front. Med.
Sec. Family Medicine and Primary Care
Volume 12 - 2025 | doi: 10.3389/fmed.2025.1499670
This article is part of the Research Topic Advances in the Pathogenesis and Treatment of Osteoporosis: From Bench to Bedside View all 8 articles
The final, formatted version of the article will be published soon.
You have multiple emails registered with Frontiers:
Please enter your email address:
If you already have an account, please login
You don't have a Frontiers account ? You can register here
Osteoporosis increases the risk of fragility fractures, especially of the lumbar spine and femur. As fractures affect life expectancy, it is crucial to detect the early stages of osteoporosis. Dual X-ray absorptiometry (DXA) is the gold standard for bone mineral density (BMD) measurement and the diagnosis of osteoporosis; however, its low screening usage is problematic. The accurate estimation of BMD using chest radiographs (CXR) could expand screening opportunities. This study aimed to indicate the clinical utility of osteoporosis screening using deep-learning-based estimation of BMD using bidirectional CXRs. This study included 1,624 patients aged ≥ 20 years who underwent DXA and bidirectional (frontal and lateral) chest radiography at a medical facility. A dataset was created using BMD and bidirectional CXR images. Inception-ResNet-V2-based models were trained using three CXR input types (frontal, lateral, and bidirectional). We compared and evaluated the BMD estimation performances of the models with different input information. In the comparison of models, the model with bidirectional CXR showed the highest accuracy. The correlation coefficients between the model estimates and DXA measurements were 0.766 and 0.683 for the lumbar spine and femoral BMD, respectively. Osteoporosis detection based on bidirectional CXR showed higher sensitivity and specificity than the models with single-view CXR input, especially for osteoporosis based on T-score ≤ -2.5, with 92.8% sensitivity at 50.0% specificity. These results suggest that bidirectional CXR contributes to improved accuracy of BMD estimation and osteoporosis screening compared with single-view CXR. This study proposes a new approach for early detection of osteoporosis using a deep learning model with frontal and lateral CXR inputs. BMD estimation using bidirectional CXR showed improved detection performance for low bone mass and osteoporosis, and has the potential to be used as a clinical decision criterion. The proposed method shows potential for more appropriate screening decisions, suggesting its usefulness in clinical practice.
Keywords: bone mineral density, Osteoporosis, screening, Chest radiograph, artificial intelligence
Received: 21 Sep 2024; Accepted: 07 Mar 2025.
Copyright: © 2025 Yoshida, Sato, Kai, Hirono, Sato and Kasai. This is an open-access article distributed under the terms of the Creative Commons Attribution License (CC BY). The use, distribution or reproduction in other forums is permitted, provided the original author(s) or licensor are credited and that the original publication in this journal is cited, in accordance with accepted academic practice. No use, distribution or reproduction is permitted which does not comply with these terms.
* Correspondence:
Satoshi Kasai, Department of Radiological Technology, Faculty of Medical Technology, Niigata University of Health and Welfare, Niigata, Niigata, Japan
Disclaimer: All claims expressed in this article are solely those of the authors and do not necessarily represent those of their affiliated organizations, or those of the publisher, the editors and the reviewers. Any product that may be evaluated in this article or claim that may be made by its manufacturer is not guaranteed or endorsed by the publisher.
Research integrity at Frontiers
Learn more about the work of our research integrity team to safeguard the quality of each article we publish.