- 1Maccabi Health Services, Tel Aviv, Israel
- 2Division of Data-Driven and Digital Medicine (D3M), Icahn School of Medicine at Mount Sinai, New York, NY, United States
- 3Edith Wolfson Medical Center, Holon, Israel
- 4Department of Gastroenterology, Sheba Medical Center, Tel HaShomer, Israel
Background and aim: In the last years, natural language processing (NLP) has transformed significantly with the introduction of large language models (LLM). This review updates on NLP and LLM applications and challenges in gastroenterology and hepatology.
Methods: Registered with PROSPERO (CRD42024542275) and adhering to PRISMA guidelines, we searched six databases for relevant studies published from 2003 to 2024, ultimately including 57 studies.
Results: Our review of 57 studies notes an increase in relevant publications in 2023–2024 compared to previous years, reflecting growing interest in newer models such as GPT-3 and GPT-4. The results demonstrate that NLP models have enhanced data extraction from electronic health records and other unstructured medical data sources. Key findings include high precision in identifying disease characteristics from unstructured reports and ongoing improvement in clinical decision-making. Risk of bias assessments using ROBINS-I, QUADAS-2, and PROBAST tools confirmed the methodological robustness of the included studies.
Conclusion: NLP and LLMs can enhance diagnosis and treatment in gastroenterology and hepatology. They enable extraction of data from unstructured medical records, such as endoscopy reports and patient notes, and for enhancing clinical decision-making. Despite these advancements, integrating these tools into routine practice is still challenging. Future work should prospectively demonstrate real-world value.
Introduction
Recent advances in Natural Language Processing (NLP) show potential for being integrated in the field of gastroenterology and hepatology (1, 2). Since the last review in 2014 by Hou et al.—which highlighted NLP’s growing utility in gastroenterology, particularly for extracting structured data from colonoscopy and pathology reports to track quality metrics and improve disease detection (2)—the field has evolved considerably. The earlier work by Hou et al. demonstrated promising performance in relatively focused domains, such as colonoscopy quality measure extraction and improving case-finding for inflammatory bowel disease, yet it largely described proof-of-concept implementations and noted challenges with integration into routine clinical workflows and data heterogeneity across settings.
In contrast, significant strides in technology, including the advent of Large Language Models (LLMs) such as Generative Pre-trained Transformer (GPT) and Bidirectional Encoder Representations from Transformers (BERT) (3), have expanded the scope of NLP applications. While Hou et al.’s era of NLP research centered on rule-based or traditional machine learning methods optimized for specific tasks, newer LLMs can handle a broader range of complex and context-rich functions, from automating routine documentation tasks to supporting sophisticated diagnostic reasoning and therapeutic decision-making (4). These contemporary models may better address scalability and integration challenges, moving beyond static data extraction toward dynamic interactions with unstructured clinical narratives.
NLP and LLMs extract and interpret data from patient records, notes, and reports (5–7). In gastroenterology and hepatology, they streamline the review of endoscopy, radiology, and pathology reports. This technology can help create research cohorts for clinical trials, flag complications, and support decision-making systems. Examples include managing complex conditions like IBD and hepatocellular carcinoma (5, 7, 8).
This review discusses the current applications and challenges of NLP and LLMs in gastroenterology and hepatology.
Methods
Registration and protocol
This systematic literature review was registered with the International Prospective Register of Systematic Reviews, PROSPERO, under the registration code CRD42024542275 (9). Our methodology adhered to the Preferred Reporting Items for Systematic Reviews and Meta-Analyses (PRISMA) guidelines (10).
Search strategy
We conducted a systematic search of six key databases (PubMed, Embase, Web of Science, and Scopus, Cochrane library and IEEE Xplore) for studies published until April 2024. Our focus was on the outcomes of integrating NLP and LLM models in gastroenterology and hepatology. We designed Boolean search strings tailored to each database. To maximize coverage, we supplemented our search with a manual reference screening of included studies and targeted searches on Google Scholar. Details of the specific Boolean strings used are provided in the Supplementary materials.
Study screening and selection
Our review encompasses original research articles, and full conference papers (11). The exclusion criteria were confined to preprints, review papers, case reports, commentaries, protocol studies, editorials, and non-English publications. For the initial screening, we used the Rayyan web application (12). The initial screening and study selection, which were conducted according to predefined criteria, were independently performed by two reviewers (MO and EK). Discrepancies were resolved through discussion. Fleiss’ kappa was calculated for the agreement between the two independent reviewers.
Data extraction
Data extraction was conducted by researchers MO and EK using a standardized form to ensure consistent and accurate data capture. This included details such as author, publication year, sample size, data type, task type, specific field, model used, results, numeric metrics, conclusions, and limitations. Any discrepancies in data extraction were resolved through discussion and a third reviewer was consulted when necessary.
Risk of bias assessment
To ensure a thorough evaluation of the included studies, we used three tools, each tailored to a specific study design within our review. The Risk Of Bias In Non-randomized Studies of Interventions (ROBINS-I) tool has been employed in interventional studies assessing NLP in applications such as management, prescription guidance, and clinical inquiry responses (13). For diagnostic studies where NLP models were compared with physicians or a reference standard for diagnosing and detection, the Quality Assessment of Diagnostic Accuracy Studies-2 (QUADAS-2) tool was used (14). Finally, the Prediction model Risk Of Bias ASsessment Tool (PROBAST) tool was utilized for the remaining studies, which involved NLP models prediction, without direct comparison to reference standards (15). This multitool approach allowed us to appropriately address the diverse methodologies and applications presented in the reviewed studies.
Results
Search results and study selection
A total of 720 articles were identified through initial screening. After the removal of 114 duplicates, 606 articles remained for further evaluation. Title and abstract screening led to the exclusion of 524 articles, leaving 82 articles for full-text review. Of these, the reasons for exclusion and the number of articles excluded for each reason remain the same as described earlier. Ultimately, 55 studies met all inclusion criteria. By employing reference checking and snowballing techniques, two additional studies were identified, resulting in a final tally of 57 studies (16–72). A PRISMA flowchart visually represents the screening process in Figure 1. Fleiss’ kappa for the agreement between screeners was calculated as 0.957, which is considered very high (73).
An overview of the included studies
Our systematic review incorporates a total of 57 studies (16–72). Among these, a substantial majority, 49 studies, are centered on gastroenterology, while hepatology is the focus of 8 studies. These studies span from 2018 to 2024, with a notable increase in publications in the last 2 years, particularly between 2023 and 2024, which collectively account for 28 of the total included studies. This uptick highlights a growing interest in advanced NLP models like GPT-3 and GPT-4.
The models employed in these studies vary widely, with traditional NLP methods and more recent LLMs like GPT-3 and GPT-4. For instance, Kong et al. (2024) utilized GPT-4 among other versions for medical counseling (38), while Schneider et al. (2023) employed rule-based NLP algorithms for detecting undiagnosed hepatic steatosis (54).
Sample sizes in these studies range from very small datasets to large-scale analyses involving millions of data points, such as in the study by Schneider et al., which analyzed data from over 2.7 million imaging reports (54). The type of data analyzed also varies significantly, encompassing electronic health records (EHRs), pathology reports, and data generated from AI models responding to preset medical queries.
Tasks performed by these models are equally diverse, from diagnostic assistance and disease monitoring to providing patient education and supporting clinical decision-making. Specific examples include the work by Truhn et al. (2024), which focused on extracting structured data from colorectal cancer reports (49), and Lahat et al. (2023), who evaluated the utility of GPT models in answering patient questions related to gastroenterology (47).
Risk of bias
We used ROBINS-I, QUADAS-2, and PROBAST to map potential biases. Notably, most of the included studies were published in Q1 journals, affirming their scholarly impact and supported by strong SCImago Journal Rank (SJR) scores (Figure 2).
PROBAST results (Supplementary Table S1)
This assessment mostly highlighted low-risk ratings in outcome and analysis domains. However, several studies encountered issues with high participant-related applicability biases, influencing the generalizability of their findings.
QUADAS-2 results (Supplementary Table S2)
A synthesis of QUADAS-2 results revealed that most studies (20 out of 32) exhibited low risk of bias across all four assessed domains. This underscores their methodological robustness and reliability. However, three studies were identified as having a high risk of bias in one of the four categories. Patient selection applicability concerns were notable, primarily due to the reliance on single-center data with specific documentation styles, which may limit the broader applicability of these findings.
ROBINS-I results (Supplementary Table S3)
Analysis of ROBINS-I revealed that 14 studies displayed a moderate risk of bias overall, while one study exhibited a high risk. This was largely due to biases in the selection of participants into the study and confounding factors, particularly because many studies utilized specific questions, queries, or fictional vignettes and case scenarios. Despite these concerns, the other assessment categories predominantly showed low risk. Nonetheless, six studies demonstrated low risk across all evaluated domains.
NLP applications
We categorized the applications of the NLP and LLM models under three main categories for a synthesized analysis of the results: Disease Detection and Diagnosis (n = 30), Patient Care (n = 22), and Education and Research applications (n = 5). Disease Detection and Diagnosis was further divided into Colonoscopy Reports and Other Diagnostic Applications, recognizing that digitized pathology reports—though ultimately part of the broader EHR—were considered separately to better capture unique NLP tasks. Patient Care was subdivided into Management and Communication and Clinical Decision Support, focusing on patient-centered and healthcare professional–oriented applications, respectively (Tables 1, 2 and Figure 3).
Disease detection and diagnosis
Most of the studies evaluated NLP models in extracting data from colonoscopy reports (n = 17) (Figure 4). Nonetheless, there were many unique applications.
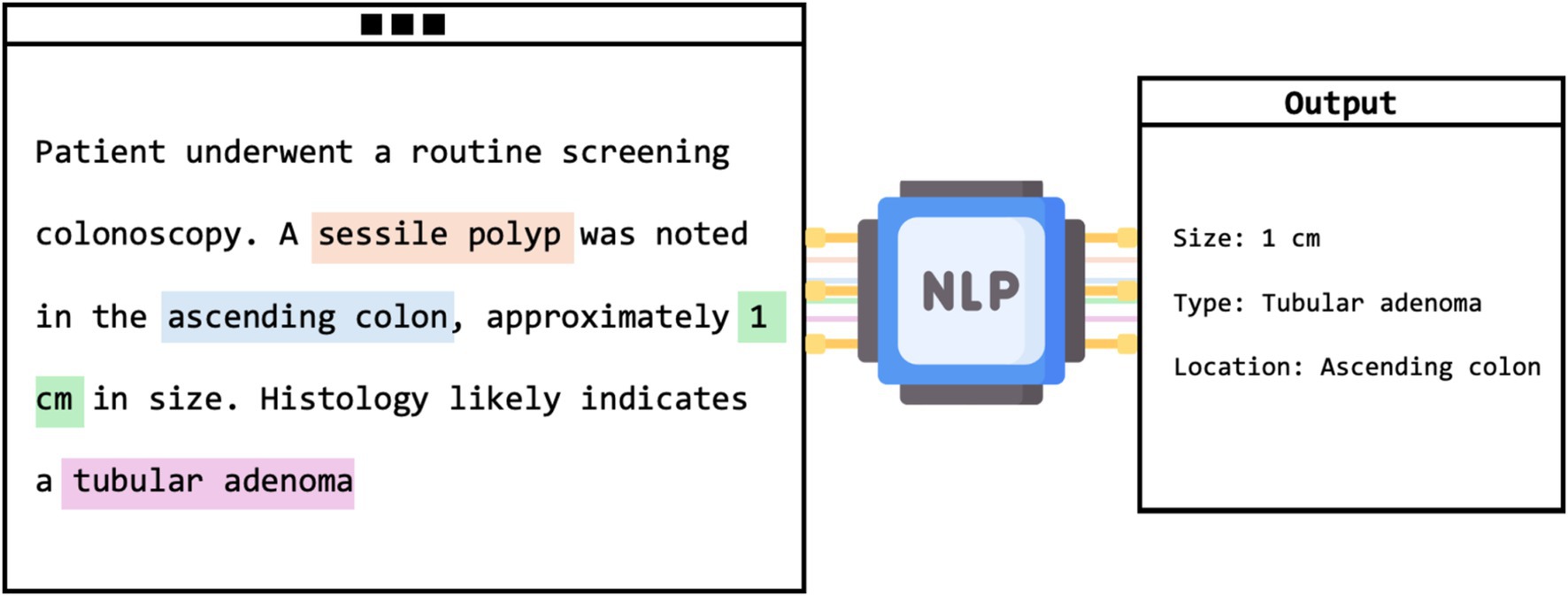
Figure 4. Visual framework of NLP extracting adenoma characteristics from unstructured colonoscopy report.
Colonoscopy reports
This category, which includes 17 studies, primarily explored NLP’s role in enhancing the interpretation of unstructured colonoscopy reports. Various quality and diagnostic measures were evaluated, such as the adenoma detection rate (ADR), a frequent subject of investigation. For instance, Nayor et al. reported that their NLP pipeline achieved high precision and recall in the automated calculation of ADR (57). Other assessments included polyp detection and sizing, with Imler et al. demonstrating accuracies of 98% for pathology level identification and 96% for size estimation (58). Additionally, Raju et al. noted that NLP matched or exceeded manual methods in identifying and categorizing adenomas with a detection rate of 43% (59). Overall, NLP models showed a broad range of accuracies from 84 to 100%, consistently outperforming manual review methods. Despite needing GPUs, these models reduce the time and effort of manual evaluations.
Other diagnostic applications
Beyond colonoscopy, NLP was applied to a diverse array of diagnostic contexts in gastroenterology and hepatology.
In gastroenterology, several innovative NLP applications have emerged. For example, Wenker et al. utilized NLP to identify dysplasia in Barrett’s Esophagus from esophagogastroduodenoscopy (EGD) reports with a high accuracy of 98.7% (69). Song et al. developed a model to extract detailed clinical information such as disease presence, location, size, and stage from unstructured EGD reports, achieving high sensitivity, precision, and accuracy scores (61). Denny et al. applied NLP to enhance colorectal cancer screening by identifying references to four CRC tests within electronic clinical documentation, demonstrating superior recall compared to traditional manual and billing record reviews (63). Additionally, Blumenthal et al. and Parthasarathy et al. used NLP for patient monitoring, with the former detecting non-adherence to follow-up colonoscopies with an AUC of 70.2%, and the latter identifying patients meeting WHO criteria for serrated polyposis syndrome with 93% accuracy (18, 65).
For IBDs, Stidham et al. utilized NLP to detect and infer the activity status of extraintestinal manifestations from clinical notes, enhancing detection accuracy to 94.1% and specificity to 95% (52). Ananthakrishnan et al. explored improving case definitions for Crohn’s disease and ulcerative colitis by combining codified data with narrative clinical texts, which identified 6–12% more patients than models using codified data alone, with AUCs of 0.95 for Crohn’s disease and 0.94 for ulcerative colitis (53).
In hepatology, NLP has facilitated significant advancements in disease identification and progression monitoring. Sada et al. combined NLP with ICD-9 codes to improve the identification of hepatocellular carcinoma cases from EHR data, significantly enhancing sensitivity and specificity, with an F2 score of 0.92 (71). Van Vleck et al. employed NLP to track disease progression in patients with non-alcoholic fatty liver disease (NAFLD), demonstrating superior sensitivity and F2 scores compared to traditional methods, effectively identifying disease progression from NAFLD to NASH or cirrhosis with sensitivity of 0.93 and an F2 score of 0.92 (30). Furthermore, Sherman et al. developed an NLP model capable of automatically scoring and classifying histological features found in pathology reports related to metabolic associated steatohepatitis (17). The goal was to estimate the risk of progression towards cirrhosis. The model demonstrated high positive and negative predictive values, ranging from 93.5 to 100%, across various histological features (17). Importantly, this NLP model facilitated the creation of a large and quality-controlled cohort of MASLD patients (17).
Patient care
The patient care section is subdivided into two categories: patient management and communication, which comprises 13 studies, and clinical decision support, encompassing 9 studies.
Management and communication
This category explores the use of NLP and LLMs in facilitating communication and management.
In gastroenterology, studies like Lahat et al. evaluated ChatGPT’s ability to answer real-life gastroenterology-related patient queries, achieving moderate effectiveness with accuracy scores ranging from 3.4 to 3.9 (47). Choo et al. reported an 86.7% concordance rate between ChatGPT’s recommendations for managing complex colorectal cancer cases and decisions made by multidisciplinary teams (39). Furthermore, Lim et al. demonstrated that a contextualized GPT-4 model provided accurate colonoscopy interval advice, significantly outperforming standard models by adhering closely to established guidelines (33). Imler et al. used the cTAKES system to achieve an 81.7% agreement with guideline-adherent colonoscopy surveillance intervals, substantially surpassing manual review accuracies (36). However, studies like Huo et al. and Atarere et al. indicated variability in ChatGPT’s performance, suggesting the need for enhancements in AI consistency and reliability (25, 44). In the area of IBD, Zand et al. developed an NLP model that categorized electronic dialog data, showing a 95% agreement with physician evaluations and underscoring the potential of automated chatbots in patient interaction (23). Sciberras et al. found ChatGPT to provide highly accurate (84.2%) and moderately complete responses to patient inquiries about IBD, with particular strengths in topics like smoking and medication (20).
In hepatology, Yeo et al. tested GPT’s proficiency in delivering emotional support and accurate information on cirrhosis and hepatocellular carcinoma, achieving correct response rates of 79.1% for cirrhosis and 74% for carcinoma (29). Samaan et al. explored GPT’s effectiveness in Arabic, noting a 72.5% accuracy rate, though it was less accurate than its English counterpart, indicating disparities in language performance (34).
Clinical decision support
NLP models were tested for their accuracy and effectiveness in decision-making scenarios. For example, Kong et al. evaluated LLMs’ capability to provide counseling on Helicobacter pylori, noting that while accuracy was generally high (90% acceptable responses), completeness needed improvement (38). Li et al.’s integration of NLP with machine learning for predicting liver metastases showed impressive results with accuracy and F1 scores around 80.4% (24). The study by Becker et al. utilized an NLP pipeline tailored for German, achieving high precision and recall in guideline-based treatment extraction from clinical notes (64). Further, Wang et al.’s “DeepCausality” framework accurately assessed causal factors for drug-induced liver injuries, aligning well with clinical guidelines (41). Another significant study, Wagholikar et al., demonstrated that an NLP-powered clinical decision support system could assist in making guideline-adherent recommendations for colonoscopy surveillance, as it made optimal recommendations in 48 out of 53 cases (35).
Education and research
Five studies focused on this aspect. Generally, NLP and LLMs have demonstrated a promising capacity to enhance learning and knowledge dissemination. Benedicenti et al. explored the accuracy of ChatGPT in solving clinical vignettes against gastroenterologists, noting an initial 40% accuracy that improved to 65% over time, suggesting a potential for future clinical integration with continued advancements (56). Zhou et al. assessed GPT-3.5 and GPT-4 for their ability to provide consultation recommendations and analyze gastroscopy reports related to gastric cancer, with GPT-4 achieving 91.3% appropriateness and 95.7% consistency (48). Lahat et al. utilized GPT to generate research questions in gastroenterology, finding the questions relevant and clear but lacking in originality (46). Meanwhile, Gravina et al. highlighted the efficacy of ChatGPT 3.5 in medical education, as it outperformed Perplexity AI in residency exam questions with a 94.11% accuracy rate (32). Additionally, Pradhan et al. compared AI-generated patient educational materials on cirrhosis with human-derived content, finding no significant differences in readability or accuracy, though human materials were deemed more actionable (28).
Validation and comparisons
Of the 57 studies, only 5 performed external validation using independent datasets. A total of 30 studies used internal validation, with 27 applying classical subsets of the same data for re-testing and validating their main results. Three studies employed a method of running LLM prompts multiple times (2–3 times) to assess the consistency of responses. Meanwhile, 22 studies did not perform any validation. Regarding direct comparisons of NLPs and LLMs with human counterparts, 44 studies compared the model’s performance with manual review by physicians or manual data extraction methods. The number of human reviewers varied between studies, ranging from 1 to 5. Thirteen studies did not perform direct comparisons (Table 2).
Discussion
Our systematic review assessed the integration of NLP and LLMS in gastroenterology and hepatology, registering significant advancements. We reviewed 57 studies, highlighting a sharp increase in research over the last 2 years, particularly focusing on newer models like GPT-3 and GPT-4. These studies reflect a shift from traditional tasks, such as report analysis, to more dynamic roles in patient management and research facilitation.
To present the findings in a clear and easily interpretable manner, we opted to categorize the reviewed studies into a minimal set of broad application areas. We acknowledge that these categories are not absolute and that certain studies may naturally span multiple domains (for example, colonoscopy surveillance intervals, while placed under one heading, could also be considered a form of clinical decision support). Nonetheless, by grouping the research into broader, more encompassing categories, we aimed to give readers a high-level understanding of where progress is most pronounced, and which areas appear closer to real-world clinical integration.
The results show that certain NLP applications seem ready for immediate clinical use. For example, Schneider et al. (2023) identified 42,000 hepatic steatosis cases using an NLP model on 2.15 million pathology reports and 2.7 million imaging reports. This level of precision (PPV 99.7%) exemplifies NLP’s readiness to support diagnostic processes in large-scale healthcare settings. Similarly, Truhn et al. (2024) successfully employed GPT-4 to extract structured data from colorectal cancer reports with a precision of 99% for T-stage identification, suggesting a high reliability of NLP in processing and structuring complex pathological data.
Conversely, the technology’s expansion into more dynamic roles such as comprehensive disease management and holistic patient care is still evolving. For instance, Kong et al. (2024) found that while the accuracy and comprehensibility of GPT-4’s responses to medical inquiries about Helicobacter pylori were high, the completeness of the information was less satisfactory. This indicates ongoing challenges in ensuring that NLP outputs are not only accurate but also fully informative.
Our results suggest that both classic NLP methods and newer models can be effectively integrated to streamline manual tasks such as extracting data and making diagnoses from complex and unstructured reports, with an accuracy that typically surpasses manual screening (16–18, 21, 22, 27, 33). This builds upon and adds on a previous systematic review of NLP in gastroenterology and hepatology conducted by Hou et al. (2). While he found promising results, he emphasized the need for careful consideration of the quality of clinical data within EHRs, and also highlighted the importance of understanding variations and deviations from established clinical practice standards (2). Our updated results indicate that these models consistently demonstrate high accuracies (16–18, 21, 22, 27, 33). This trend is observable in other fields utilizing NLP, such as radiology and infectious diseases (74, 75). However, our research suggests that applying these methods to more complex tasks like patient management, education, and clinical decision-making is still challenging (20, 29, 34, 37). While newer models show promising results, there are significant limitations and variability that require further development (67). This trend is consistent with data and the current findings from other fields (76, 77).
Several limitations of our review must be acknowledged. Many studies utilize single-institution datasets, which could affect the generalizability of the findings. This is important especially because only 5 studies (8.7%) reported performing an external validation. The accuracy of NLP outputs is heavily dependent on the quality of the input data, with errors or inconsistencies in medical records potentially leading to inaccurate results (78). The opaque nature of AI decision-making processes (‘black box’) raises concerns about the transparency and trustworthiness of these models in clinical settings (79). Ethical considerations around potential biases in training data and algorithmic outputs underscore the necessity for careful implementation to ensure fairness and equity in healthcare delivery (80). Moreover, the accuracy and reliability of NLP and LLM outputs are directly tied to the quality of the input data. EHRs, clinical notes, and imaging reports often contain incomplete, ambiguous, or inaccurately recorded information. These data imperfections can lead to propagation of errors and compound biases within the model’s output, potentially influencing clinical decision-making and patient care. Additionally, while many NLP and LLM models show promise in structured tasks like disease detection or data extraction, they remain susceptible to “hallucinations”—generating plausible-sounding but factually incorrect statements (81). Such errors, if undetected, may result in misguided clinical judgments, suboptimal patient management, and delayed interventions. An additional critical dimension of these limitations involves the potential for algorithmic biases, including those related to sociodemographic factors such as race, ethnicity, gender, language proficiency, and socioeconomic status (82, 83). Models trained on unrepresentative or historically biased data risk perpetuating systemic inequalities in healthcare. Despite the promising accuracy of some NLP applications, they are not yet widely integrated into day-to-day clinical workflows, particularly for patient care and decision-making; current limitations and the need for thorough testing and validation—especially for newer, less researched techniques—have thus far hindered their routine implementation in practice.
In conclusion, our systematic review highlights the impact of NLP and LLMs in gastroenterology and hepatology. On one hand, NLP has already proven its utility in screening and analyzing medical reports, facilitating streamlined screening policies with impressive outcomes. On the other hand, the capabilities of newer LLMs are still unfolding, with their full potential in complex management and research roles yet to be fully realized. The results demonstrate that while some applications of NLP are well-established and highly effective, newer LLMs offer exciting, emerging applications that promise to further enhance clinical practice. Moving forward, research focus should be on refining these models, and externally validating the results to ensure prospectively they meet real-world clinical needs.
Data availability statement
The original contributions presented in the study are included in the article/Supplementary material, further inquiries can be directed to the corresponding author.
Author contributions
MO: Conceptualization, Data curation, Formal analysis, Funding acquisition, Investigation, Methodology, Project administration, Resources, Software, Supervision, Validation, Visualization, Writing – original draft, Writing – review & editing. SN: Supervision, Validation, Writing – review & editing. KS: Supervision, Validation, Writing – review & editing. BG: Conceptualization, Formal analysis, Writing – review & editing. GN: Supervision, Writing – review & editing. EK: Conceptualization, Supervision, Validation, Writing – original draft, Writing – review & editing.
Funding
The author(s) declare that no financial support was received for the research, authorship, and/or publication of this article.
Conflict of interest
The authors declare that the research was conducted in the absence of any commercial or financial relationships that could be construed as a potential conflict of interest.
Publisher’s note
All claims expressed in this article are solely those of the authors and do not necessarily represent those of their affiliated organizations, or those of the publisher, the editors and the reviewers. Any product that may be evaluated in this article, or claim that may be made by its manufacturer, is not guaranteed or endorsed by the publisher.
Supplementary material
The Supplementary material for this article can be found online at: https://www.frontiersin.org/articles/10.3389/fmed.2024.1512824/full#supplementary-material
References
1. Klang, E, Sourosh, A, Nadkarni, GN, Sharif, K, and Lahat, A. Evaluating the role of chat GPT in gastroenterology: a comprehensive systematic review of applications, benefits, and limitations. Ther Adv Gastroenterol. (2023) 16:17562848231218618. doi: 10.1177/17562848231218618
2. Hou, JK, Imler, TD, and Imperiale, TF. Current and future applications of natural language processing in the field of digestive diseases. Clin Gastroenterol Hepatol Off Clin Pract J Am Gastroenterol Assoc. (2014) 12:1257–61. doi: 10.1016/j.cgh.2014.05.013
3. Dave, T, Athaluri, SA, and Singh, S. Chat GPT in medicine: an overview of its applications, advantages, limitations, future prospects, and ethical considerations. Front Artif Intell. (2023) 6:1169595. doi: 10.3389/frai.2023.1169595
4. Nehme, F, and Feldman, K. Evolving role and future directions of natural language processing in gastroenterology. Dig Dis Sci. (2021) 66:29–40. doi: 10.1007/s10620-020-06156-y
5. Tavanapong, W, Oh, J, Riegler, MA, Khaleel, M, Mittal, B, and de Groen, PC. Artificial intelligence for colonoscopy: past, present, and future. IEEE J Biomed Health Inform. (2022) 26:3950–65. doi: 10.1109/JBHI.2022.3160098
6. Stidham, RW. Artificial intelligence for understanding imaging, text, and data in gastroenterology. Gastroenterol Hepatol. (2020) 16:341–9.
7. Zaver, HB, and Patel, T. Opportunities for the use of large language models in hepatology. Clin Liver Dis. (2023) 22:171–6. doi: 10.1097/CLD.0000000000000075
8. Shahab, O, El Kurdi, B, Shaukat, A, Nadkarni, G, and Soroush, A. Large language models: a primer and gastroenterology applications. Ther Adv Gastroenterol. (2024) 17:17562848241227031. doi: 10.1177/17562848241227031
9. Schiavo, JH. PROSPERO: An international register of systematic review protocols. Med Ref Serv Q. (2019) 38:171–80. doi: 10.1080/02763869.2019.1588072
10. Page, MJ, McKenzie, JE, Bossuyt, PM, Boutron, I, Hoffmann, TC, Mulrow, CD, et al. The PRISMA 2020 statement: an updated guideline for reporting systematic reviews. BMJ. (2021) 372:n71. doi: 10.1136/bmj.n71
11. Brietzke, E, Gomes, FA, Gerchman, F, and Freire, RCR. Should systematic reviews and meta-analyses include data from preprints? Trends Psychiatry Psychother. (2023) 45:e20210324.
12. Ouzzani, M, Hammady, H, Fedorowicz, Z, and Elmagarmid, A. Rayyan-a web and mobile app for systematic reviews. Syst Rev. (2016) 5:210. doi: 10.1186/s13643-016-0384-4
13. Sterne, JA, Hernán, MA, Reeves, BC, Savović, J, Berkman, ND, Viswanathan, M, et al. ROBINS-I: a tool for assessing risk of bias in non-randomised studies of interventions. BMJ. (2016) 355:i4919. doi: 10.1136/bmj.i4919
14. Whiting, PF, Rutjes, AWS, Westwood, ME, Mallett, S, Deeks, JJ, Reitsma, JB, et al. QUADAS-2: a revised tool for the quality assessment of diagnostic accuracy studies. Ann Intern Med. (2011) 155:529–36. doi: 10.7326/0003-4819-155-8-201110180-00009
15. Wolff, RF, Moons, KGM, Riley, RD, Whiting, PF, Westwood, M, Collins, GS, et al. PROBAST: a tool to assess the risk of Bias and applicability of prediction model studies. Ann Intern Med. (2019) 170:51–8. doi: 10.7326/M18-1376
16. Li, J, Hu, S, Shi, C, Dong, Z, Pan, J, Ai, Y, et al. A deep learning and natural language processing-based system for automatic identification and surveillance of high-risk patients undergoing upper endoscopy: a multicenter study. EClinicalMedicine. (2022) 53:101704. doi: 10.1016/j.eclinm.2022.101704
17. Sherman, MS, Challa, PK, Przybyszewski, EM, Wilechansky, RM, Uche-Anya, EN, Ott, AT, et al. A natural language processing algorithm accurately classifies steatotic liver disease pathology to estimate the risk of cirrhosis. Hepatol Commun. (2024) 8:e 0403. doi: 10.1097/HC9.0000000000000403
18. Parthasarathy, G, Lopez, R, McMichael, J, and Burke, CA. A natural language-based tool for diagnosis of serrated polyposis syndrome. Gastrointest Endosc. (2020) 92:886–90. doi: 10.1016/j.gie.2020.04.077
19. A Transparent and Adaptable Method to Extract Colonoscopy and Pathology Data Using Natural Language Processing-Pub Med. (2023) Available at: https://pubmed.ncbi.nlm.nih.gov/32737597/
20. Sciberras, M, Farrugia, Y, Gordon, H, Furfaro, F, Allocca, M, Torres, J, et al. Accuracy of information given by chat GPT for patients with inflammatory bowel disease in relation to ECCO guidelines. J Crohns Colitis. (2024) 18:1215–21. doi: 10.1093/ecco-jcc/jjae040
21. Lee, JK, Jensen, CD, Levin, TR, Zauber, AG, Doubeni, CA, Zhao, WK, et al. Accurate identification of colonoscopy quality and polyp findings using natural language processing. J Clin Gastroenterol. (2019) 53:e25–30. doi: 10.1097/MCG.0000000000000929
22. Ganguly, EK, Purvis, L, Reynolds, N, Akram, S, Lidofsky, SD, and Zubarik, R. An accurate and automated method for adenoma detection rate and report card generation utilizing common electronic health records. J Clin Gastroenterol. (2023) 58:656–60. doi: 10.1097/MCG.0000000000001915
23. Zand, A, Sharma, A, Stokes, Z, Reynolds, C, Montilla, A, Sauk, J, et al. An exploration into the use of a Chatbot for patients with inflammatory bowel diseases: retrospective cohort study. J Med Internet Res. (2020) 22:e15589. doi: 10.2196/15589
24. Li, J, Wang, X, Cai, L, Sun, J, Yang, Z, Liu, W, et al. An interpretable deep learning framework for predicting liver metastases in postoperative colorectal cancer patients using natural language processing and clinical data integration. Cancer Med. (2023) 12:19337–51. doi: 10.1002/cam4.6523
25. Atarere, J, Naqvi, H, Haas, C, Adewunmi, C, Bandaru, S, Allamneni, R, et al. Applicability of online chat-based artificial intelligence models to colorectal Cancer screening. Dig Dis Sci. (2024) 69:791–7. doi: 10.1007/s10620-024-08274-3
26. Laique, SN, Hayat, U, Sarvepalli, S, Vaughn, B, Ibrahim, M, McMichael, J, et al. Application of optical character recognition with natural language processing for large-scale quality metric data extraction in colonoscopy reports. Gastrointest Endosc. (2021) 93:750–7. doi: 10.1016/j.gie.2020.08.038
27. Mehrotra, A, Dellon, ES, Schoen, RE, Saul, M, Bishehsari, F, Farmer, C, et al. Applying a natural language processing tool to electronic health records to assess performance on colonoscopy quality measures. Gastrointest Endosc. (2012) 75:1233–1239.e14. doi: 10.1016/j.gie.2012.01.045
28. Pradhan, F, Fiedler, A, Samson, K, Olivera-Martinez, M, Manatsathit, W, and Peeraphatdit, T. Artificial intelligence compared with human-derived patient educational materials on cirrhosis. Hepatol Commun. (2024) 8:e 0367. doi: 10.1097/HC9.0000000000000367
29. Yeo, YH, Samaan, JS, Ng, WH, Ting, PS, Trivedi, H, Vipani, A, et al. Assessing the performance of chat GPT in answering questions regarding cirrhosis and hepatocellular carcinoma. Clin Mol Hepatol. (2023) 29:721–32. doi: 10.3350/cmh.2023.0089
30. Van Vleck, TT, Chan, L, Coca, SG, Craven, CK, Do, R, Ellis, SB, et al. Augmented intelligence with natural language processing applied to electronic health records for identifying patients with non-alcoholic fatty liver disease at risk for disease progression. Int J Med Inform. (2019) 129:334–41. doi: 10.1016/j.ijmedinf.2019.06.028
31. Hou, JK, Chang, M, Nguyen, T, Kramer, JR, Richardson, P, Sansgiry, S, et al. Automated identification of surveillance colonoscopy in inflammatory bowel disease using natural language processing. Dig Dis Sci. (2013) 58:936–41. doi: 10.1007/s10620-012-2433-8
32. Gravina, AG, Pellegrino, R, Palladino, G, Imperio, G, Ventura, A, and Federico, A. Charting new AI education in gastroenterology: cross-sectional evaluation of chat GPT and perplexity AI in medical residency exam. Dig Liver Dis Off J Ital Soc Gastroenterol Ital Assoc Study Liver. (2024) S1590-8658:00302–5.
33. Lim, DYZ, Tan, YB, Koh, JTE, Tung, JYM, Sng, GGR, Tan, DMY, et al. Chat GPT on guidelines: providing contextual knowledge to GPT allows it to provide advice on appropriate colonoscopy intervals. J Gastroenterol Hepatol. (2024) 39:81–106. doi: 10.1111/jgh.16375
34. Samaan, JS, Yeo, YH, Ng, WH, Ting, PS, Trivedi, H, Vipani, A, et al. Chat GPT’s ability to comprehend and answer cirrhosis related questions in Arabic. Arab J Gastroenterol Off Publ Pan-Arab Assoc Gastroenterol. (2023) 24:145–8.
35. Wagholikar, K, Sohn, S, Wu, S, Kaggal, V, Buehler, S, Greenes, R, et al. Clinical decision support for colonoscopy surveillance using natural language processing In: 2012 IEEE second international conference on healthcare informatics, imaging and systems biology (2012). 12–21.
36. Imler, TD, Morea, J, and Imperiale, TF. Clinical decision support with natural language processing facilitates determination of colonoscopy surveillance intervals. Clin Gastroenterol Hepatol Off Clin Pract J Am Gastroenterol Assoc. (2014) 12:1130–6. doi: 10.1016/j.cgh.2013.11.025
37. Pereyra, L, Schlottmann, F, Steinberg, L, and Lasa, J. Colorectal Cancer prevention: is chat generative Pretrained transformer (chat GPT) ready to assist physicians in determining appropriate screening and surveillance recommendations? J Clin Gastroenterol. (2024) 58:1022–7. doi: 10.1097/MCG.0000000000001979
38. Kong, Q, Ju, K, Wan, M, Liu, J, Wu, X, Li, Y, et al. Comparative analysis of large language models in medical counseling: a focus on Helicobacter pylori infection. Helicobacter. (2024) 29:e13055. doi: 10.1111/hel.13055
39. Choo, JM, Ryu, HS, Kim, JS, Cheong, JY, Baek, SJ, Kwak, JM, et al. Conversational artificial intelligence (chat GPT™) in the management of complex colorectal cancer patients: early experience. ANZ J Surg. (2024) 94:356–61. doi: 10.1111/ans.18749
40. Seong, D, Choi, YH, Shin, SY, and Yi, BK. Deep learning approach to detection of colonoscopic information from unstructured reports. BMC Med Inform Decis Mak. (2023) 23:28. doi: 10.1186/s12911-023-02121-7
41. Wang, X, Xu, X, Tong, W, Liu, Q, and Liu, Z. Deep causality: a general AI-powered causal inference framework for free text: a case study of liver Tox. Front Artif Intell. (2022) 5:5. doi: 10.3389/frai.2022.999289
42. Harkema, H, Chapman, WW, Saul, M, Dellon, ES, Schoen, RE, and Mehrotra, A. Developing a natural language processing application for measuring the quality of colonoscopy procedures. J Am Med Inform Assoc JAMIA. (2011) 18:i150–6. doi: 10.1136/amiajnl-2011-000431
43. Ma, H, Ma, X, Yang, C, Niu, Q, Gao, T, Liu, C, et al. Development and evaluation of a program based on a generative pre-trained transformer model from a public natural language processing platform for efficiency enhancement in post-procedural quality control of esophageal endoscopic submucosal dissection. Surg Endosc. (2024) 38:1264–72. doi: 10.1007/s00464-023-10620-x
44. Huo, B, McKechnie, T, Ortenzi, M, Lee, Y, Antoniou, S, Mayol, J, et al. GPT will see you now: the ability of large language model-linked chatbots to provide colorectal cancer screening recommendations. Health Technol. (2024) 14:463–9. doi: 10.1007/s12553-024-00836-9
45. Peng, W, Feng, Y, Yao, C, Zhang, S, Zhuo, H, Qiu, T, et al. Evaluating AI in medicine: a comparative analysis of expert and chat GPT responses to colorectal cancer questions. Sci Rep. (2024) 14:2840. doi: 10.1038/s41598-024-52853-3
46. Lahat, A, Shachar, E, Avidan, B, Shatz, Z, Glicksberg, BS, and Klang, E. Evaluating the use of large language model in identifying top research questions in gastroenterology. Sci Rep. (2023) 13:4164. doi: 10.1038/s41598-023-31412-2
47. Lahat, A, Shachar, E, Avidan, B, Glicksberg, B, and Klang, E. Evaluating the utility of a large language model in answering common patients’ gastrointestinal health-related questions: are we there yet? Diagnostics. (2023) 13:1950. doi: 10.3390/diagnostics13111950
48. Zhou, J, Li, T, Fong, SJ, Dey, N, and González-Crespo, R. Exploring chat GPT’s potential for consultation, recommendations and report diagnosis: gastric Cancer and gastroscopy reports’ case. Int J Interact Multimed Artif Intell. (2023) 8:7–13. doi: 10.9781/ijimai.2023.04.007
49. Truhn, D, Loeffler, CM, Müller-Franzes, G, Nebelung, S, Hewitt, KJ, Brandner, S, et al. Extracting structured information from unstructured histopathology reports using generative pre-trained transformer 4 (GPT-4). J Pathol. (2024) 262:310–9. doi: 10.1002/path.6232
50. Denny, JC, Peterson, JF, Choma, NN, Xu, H, Miller, RA, Bastarache, L, et al. Extracting timing and status descriptors for colonoscopy testing from electronic medical records. J Am Med Inform Assoc JAMIA. (2010) 17:383–8. doi: 10.1136/jamia.2010.004804
51. Gorelik, Y, Ghersin, I, Maza, I, and Klein, A. Harnessing language models for streamlined postcolonoscopy patient management: a novel approach. Gastrointest Endosc. (2023) 98:639–641.e4. doi: 10.1016/j.gie.2023.06.025
52. Stidham, RW, Yu, D, Zhao, X, Bishu, S, Rice, M, Bourque, C, et al. Identifying the presence, activity, and status of Extraintestinal manifestations of inflammatory bowel disease using natural language processing of clinical notes. Inflamm Bowel Dis. (2023) 29:503–10. doi: 10.1093/ibd/izac109
53. Ananthakrishnan, AN, Cai, T, Savova, G, Cheng, SC, Chen, P, Perez, RG, et al. Improving case definition of Crohn’s disease and ulcerative colitis in electronic medical records using natural language processing: a novel informatics approach. Inflamm Bowel Dis. (2013) 19:1411–20. doi: 10.1097/MIB.0b013e31828133fd
54. Schneider, CV, Li, T, Zhang, D, Mezina, AI, Rattan, P, Huang, H, et al. Large-scale identification of undiagnosed hepatic steatosis using natural language processing. eClinicalMedicine. (2023) 62:102149. doi: 10.1016/j.eclinm.2023.102149
55. Benson, R, Winterton, C, Winn, M, Krick, B, Liu, M, Abu-el-rub, N, et al. Leveraging natural language processing to extract features of colorectal polyps from pathology reports for epidemiologic study. JCO Clin Cancer Inform. (2023):7. doi: 10.1200/CCI.22.00131
56. Benedicenti, F, Pessarelli, T, Corradi, M, Michelon, M, Nandi, N, Lampertico, P, et al. Mirror, mirror on the wall, who is the best of them all? Artificial intelligence versus gastroenterologists in solving clinical problems. Gastroenterol Rep. (2023) 11:goad052.
57. Nayor, J, Borges, LF, Goryachev, S, Gainer, VS, and Saltzman, JR. Natural language processing accurately calculates adenoma and sessile serrated polyp detection rates. Dig Dis Sci. (2018) 63:1794–800. doi: 10.1007/s10620-018-5078-4
58. Imler, TD, Morea, J, Kahi, C, and Imperiale, TF. Natural language processing accurately categorizes findings from colonoscopy and pathology reports. Clin Gastroenterol Hepatol Off Clin Pract J Am Gastroenterol Assoc. (2013) 11:689–94. doi: 10.1016/j.cgh.2012.11.035
59. Raju, GS, Lum, PJ, Slack, RS, Thirumurthi, S, Lynch, PM, Miller, E, et al. Natural language processing as an alternative to manual reporting of colonoscopy quality metrics. Gastrointest Endosc. (2015) 82:512–9. doi: 10.1016/j.gie.2015.01.049
60. Bae, JH, Han, HW, Yang, SY, Song, G, Sa, S, Chung, GE, et al. Natural language processing for assessing quality indicators in free-text colonoscopy and pathology reports: development and usability study. JMIR Med Inform. (2022) 10:e35257. doi: 10.2196/35257
61. Song, G, Chung, SJ, Seo, JY, Yang, SY, Jin, EH, Chung, GE, et al. Natural language processing for information extraction of gastric diseases and its application in large-scale clinical research. J Clin Med. (2022) 11:2967. doi: 10.3390/jcm11112967
62. Li, D, Udaltsova, N, Layefsky, E, Doan, C, and Corley, DA. Natural language processing for the accurate identification of colorectal Cancer mismatch repair status in Lynch syndrome screening. Clin Gastroenterol Hepatol Off Clin Pract J Am Gastroenterol Assoc. (2021) 19:610–612.e1. doi: 10.1016/j.cgh.2020.01.040
63. Denny, JC, Choma, NN, Peterson, JF, Miller, RA, Bastarache, L, Li, M, et al. Natural language processing improves identification of colorectal cancer testing in the electronic medical record. Med Decis Mak Int J Soc Med Decis Mak. (2012) 32:188–97. doi: 10.1177/0272989X11400418
64. Becker, M, Kasper, S, Böckmann, B, Jöckel, KH, and Virchow, I. Natural language processing of German clinical colorectal cancer notes for guideline-based treatment evaluation. Int J Med Inform. (2019) 127:141–6. doi: 10.1016/j.ijmedinf.2019.04.022
65. Blumenthal, DM, Singal, G, Mangla, SS, Macklin, EA, and Chung, DC. Predicting non-adherence with outpatient colonoscopy using a novel electronic tool that measures prior non-adherence. J Gen Intern Med. (2015) 30:724–31. doi: 10.1007/s11606-014-3165-6
66. Imler, TD, Sherman, S, Imperiale, TF, Xu, H, Ouyang, F, Beesley, C, et al. Provider-specific quality measurement for ERCP using natural language processing. Gastrointest Endosc. (2018) 87:164–173.e2. doi: 10.1016/j.gie.2017.04.030
67. Cankurtaran, RE, Polat, YH, Aydemir, NG, Umay, E, and Yurekli, OT. Reliability and usefulness of chat GPT for inflammatory bowel diseases: An analysis for patients and healthcare professionals. Cureus. (2023) 15:e46736.
68. Rammohan, R, Joy, MV, Magam, SG, Natt, D, Magam, SR, Pannikodu, L, et al. Understanding the landscape: the emergence of artificial intelligence (AI), chat GPT, and Google bard in gastroenterology. Cureus. (2024) 16
69. Nguyen Wenker, T, Natarajan, Y, Caskey, K, Novoa, F, Mansour, N, Pham, HA, et al. Using natural language processing to automatically identify dysplasia in pathology reports for patients with Barrett’s esophagus. Clin Gastroenterol Hepatol Off Clin Pract J Am Gastroenterol Assoc. (2023) 21:1198–204. doi: 10.1016/j.cgh.2022.09.005
70. Tinmouth, J, Swain, D, Chorneyko, K, Lee, V, Bowes, B, Li, Y, et al. Validation of a natural language processing algorithm to identify adenomas and measure adenoma detection rates across a health system: a population-level study. Gastrointest Endosc. (2023) 97:121–129.e1. doi: 10.1016/j.gie.2022.07.009
71. Sada, Y, Hou, J, Richardson, P, El-Serag, H, and Davila, J. Validation of case finding algorithms for hepatocellular Cancer from administrative data and electronic health records using natural language processing. Med Care. (2016) 54:e9–e14. doi: 10.1097/MLR.0b013e3182a30373
72. Wang, Y, Huang, Y, Nimma, IR, Pang, S, Pang, M, Cui, T, et al. Validation of GPT-4 for clinical event classification: a comparative analysis with ICD codes and human reviewers. J Gastroenterol Hepatol. (2024) 39:1535–43. doi: 10.1111/jgh.16561
73. Mandrekar, JN. Measures of interrater agreement. J Thorac Oncol. (2011) 6:6–7. doi: 10.1097/JTO.0b013e318200f983
74. Omar, M, Brin, D, Glicksberg, B, and Klang, E. Utilizing natural language processing and large language models in the diagnosis and prediction of infectious diseases: a systematic review. Am J Infect Control. (2024)
75. Casey, A, Davidson, E, Poon, M, Dong, H, Duma, D, Grivas, A, et al. A systematic review of natural language processing applied to radiology reports. BMC Med Inform Decis Mak. (2021) 21:179. doi: 10.1186/s12911-021-01533-7
76. Cheng, S, Chang, C, Chang, W, Wang, H, Liang, C, Kishimoto, T, et al. The now and future of chat GPT and GPT in psychiatry. Psychiatry Clin Neurosci. (2023) 77:592–6. doi: 10.1111/pcn.13588
77. Oh, N, Choi, GS, and Lee, WY. Chat GPT goes to the operating room: evaluating GPT-4 performance and its potential in surgical education and training in the era of large language models. Ann Surg Treat Res. (2023) 104:269–73. doi: 10.4174/astr.2023.104.5.269
78. Wang, J, Deng, H, Liu, B, Hu, A, Liang, J, Fan, L, et al. Systematic evaluation of research Progress on natural language processing in medicine over the past 20 years: bibliometric study on pub med. J Med Internet Res. (2020) 22:e16816. doi: 10.2196/16816
79. Poon, AIF, and Sung, JJY. Opening the black box of AI-medicine. J Gastroenterol Hepatol. (2021) 36:581–4. doi: 10.1111/jgh.15384
80. Herington, J, McCradden, MD, Creel, K, Boellaard, R, Jones, EC, Jha, AK, et al. Ethical considerations for artificial intelligence in medical imaging: deployment and governance. J Nucl Med Off Publ Soc Nucl Med. (2023) 64:1509–15.
81. Azamfirei, R, Kudchadkar, SR, and Fackler, J. Large language models and the perils of their hallucinations. Crit Care. (2023) 27:120. doi: 10.1186/s13054-023-04393-x
82. Omar, M, Soffer, S, Agbareia, R, Bragazzi, NL, Apakama, DU, Horowitz, CR, et al. Socio-demographic biases in medical decision-making by large language models: a large-scale multi-model analysis. med Rxiv. (2024):2024. doi: 10.1101/2024.10.29.24316368v1
83. Omar, M, Sorin, V, Agbareia, R, Apakama, DU, Soroush, A, Sakhuja, A, et al. Evaluating and addressing demographic disparities in medical large language models: a systematic review. med Rxiv. (2024). doi: 10.1101/2024.09.09.24313295v2
Keywords: natural language processing, large language models, gastroenterology, hepatology, electronic health records
Citation: Omar M, Nassar S, SharIf K, Glicksberg BS, Nadkarni GN and Klang E (2025) Emerging applications of NLP and large language models in gastroenterology and hepatology: a systematic review. Front. Med. 11:1512824. doi: 10.3389/fmed.2024.1512824
Edited by:
Raffaele Pellegrino, University of Campania Luigi Vanvitelli, ItalyReviewed by:
Sheng-Chieh Lu, University of Texas MD Anderson Cancer Center, United StatesAngus Roberts, King’s College London, United Kingdom
Copyright © 2025 Omar, Nassar, SharIf, Glicksberg, Nadkarni and Klang. This is an open-access article distributed under the terms of the Creative Commons Attribution License (CC BY). The use, distribution or reproduction in other forums is permitted, provided the original author(s) and the copyright owner(s) are credited and that the original publication in this journal is cited, in accordance with accepted academic practice. No use, distribution or reproduction is permitted which does not comply with these terms.
*Correspondence: Mahmud Omar, TWFobXVkb21hcjcwQGdtYWlsLmNvbQ==