- 1Federal Research and Clinical Centre of Intensive Care Medicine and Rehabilitology, Moscow, Russia
- 2Department of Anaesthesia and Intensive Care, IRCCS San Raffaele Scientific Institute, Milan, Italy
- 3Department of Anesthesiology, Vita-Salute San Raffaele University, Milan, Italy
- 4Department of Anesthesiology, I.M. Sechenov First Moscow State Medical University, Moscow, Russia
Background: With machine learning (ML) carving a niche in diverse medical disciplines, its role in sepsis prediction, a condition where the ‘golden hour’ is critical, is of paramount interest. This study assesses the factors influencing the efficacy of ML models in sepsis prediction, aiming to optimize their use in clinical practice.
Methods: We searched Medline, PubMed, Google Scholar, and CENTRAL for studies published from inception to October 2023. We focused on studies predicting sepsis in real-time settings in adult patients in any hospital settings without language limits. The primary outcome was area under the curve (AUC) of the receiver operating characteristic. This meta-analysis was conducted according to PRISMA-NMA guidelines and Cochrane Handbook recommendations. A Network Meta-Analysis using the CINeMA approach compared ML models against traditional scoring systems, with meta-regression identifying factors affecting model quality.
Results: From 3,953 studies, 73 articles encompassing 457,932 septic patients and 256 models were analyzed. The pooled AUC for ML models was 0.825 and it significantly outperformed traditional scoring systems. Neural Network and Decision Tree models demonstrated the highest AUC metrics. Significant factors influencing AUC included ML model type, dataset type, and prediction window.
Conclusion: This study establishes the superiority of ML models, especially Neural Network and Decision Tree types, in sepsis prediction. It highlights the importance of model type and dataset characteristics for prediction accuracy, emphasizing the necessity for standardized reporting and validation in ML healthcare applications. These findings call for broader clinical implementation to evaluate the effectiveness of these models in diverse patient groups.
Systematic review registration: https://inplasy.com/inplasy-2023-12-0062/, identifier, INPLASY2023120062.
1 Introduction
Sepsis is a critical medical condition characterized by a substantial risk of mortality (1). Prompt identification of sepsis is crucial for the successful treatment of this life-threatening condition. Adhering to the ‘golden hour’ principle, which suggests that patient outcomes are significantly improved when treatment is initiated within the first hour following diagnosis, is pivotal for enhancing patient survival rates. Concurrently, there is a robust endorsement for employing systematic screening procedures for early sepsis identification (2).
The accuracy of current clinical scales and diagnostic methodologies in detecting and predicting sepsis seems to be significantly suboptimal, leading to delays in therapeutic interventions (3–5). Despite the widespread use of traditional sepsis scoring systems, such as SOFA, NEWS, MEWS, SIRS, and SAPS II, these tools exhibit several limitations, including their reliance on static thresholds and suboptimal predictive performance. As a result, traditional sepsis scoring systems often lack the sensitivity and specificity required for timely, accurate sepsis detection.
This gap underscores the urgent need for more precise and reliable diagnostic and prognostic tools. In this regard, there is a shift in focus towards innovative approaches such as machine learning (6–13). Particularly, right-aligned models are drawing significant attention for their capacity to predict the development of sepsis hours before its clinical confirmation (14). Evidence increasingly suggests that machine learning methodologies offer a distinct advantage over traditional sepsis scoring systems (6).
To date, three meta-analyses have been conducted in this area of study (6, 15, 16), with one demonstrating the superiority of machine learning over traditional clinical scales in sepsis prognosis (6). In second research, the evidence presented lacks robustness (16), whereas in a third investigation, the focus was solely on the comparative assessment of different machine learning methodologies (15). However, the significant clinical heterogeneity, not entirely unambiguous diagnostic criteria, diverse prognostic time frames, and differing approaches to data preprocessing and model development across patient populations preclude definitive conclusions about the prognostic efficacy of these machine learning models.
In response to these challenges, our objective was to conduct a pioneering network meta-analysis to address this heterogeneity and to surpass the confines of previous research. Through meta-regression, we aimed to identify key factors that influence the effectiveness of predictive models, thereby guiding the development of an optimal model for sepsis prognosis, tailored to the complexities of clinical scenarios.
2 Materials and methods
This study was conducted in accordance with the Preferred Reporting Items for Systematic Reviews and Meta-Analyses (PRISMA) Extension Statement for Reporting of Systematic Reviews Incorporating Network Meta-analyses of Health Care Interventions (PRISMA-NMA) guidelines (17) and the Cochrane Handbook recommendations (18). The study protocol was registered with the International Platform of Registered Systematic Review and Meta-analysis Protocols (INPLASY) under the registration number INPLASY 2023120062 (doi: 10.37766/inplasy2023.12.0062). The completed PRISMA-NMA checklist is presented in Supplementary Table S1.
2.1 Search strategy
We performed a systematic search of the literature across Medline, PubMed, Google Scholar, and the Cochrane Central Register of Controlled Trials (CENTRAL) from inception to October 2023. The search was conducted by two independent investigators. Backward and forward citation tracking was also employed to identify additional studies, leveraging the Litmaps service (19). No language restrictions were applied. Details of the search strategy, including full queries, are provided in Supplementary Appendix A.
2.2 Eligibility criteria and study selection
Following the automatic removal of duplicate records, two independent researchers screened the remaining studies for eligibility. We applied the PICOS (Population, Intervention, Comparator, Outcome, and Study design) framework to guide study selection (Supplementary Appendix B).
Studies were considered eligible if they focused on real-time prediction of sepsis onset (right alignment (14)) in adult patients across any hospital setting. Both prospective and retrospective diagnostic test accuracy studies were included. The target condition was the onset of sepsis, defined by Sepsis-3 criteria (20) or other operational definitions provided by the authors (Supplementary Table S5).
Studies were excluded if they met one of the following criteria: (1) were review articles, case reports or case series without control groups; (2) had no sepsis definition criteria; (3) reported no AUC for sepsis development; (4) reported AUC for other outcome (e.g., reported data on mortality only); (5) focused on pediatric patients; (6) reported no data on patient cohort (sample size, age, sex, etc.); (7) were published as conference papers or preprints only.
Any disagreement was solved by consultation until consensus was reached. Divergences were resolved by consensus with the involvement of the supervisor.
2.3 Outcome measures and data extraction
A standardized data collection form was developed specifically for this review. Three independent authors used this form to systematically evaluate the full text, supplemental materials, and additional files of all included studies. Data extraction was performed independently by three authors, with any discrepancies resolved through discussion to achieve consensus.
Extracted information encompassed: (1) Basic study details such as the first author, publication year, country, journal, study design, data collection period, mean age, sex, hospital mortality, prediction method, and sample size; (2) ML model characteristics: data source, prediction model, sepsis definition criteria, department, prediction window, external validation, imputation, features; (3) Outcome data: area under the curve of the receiver operating characteristic (AUC) as performance metric.
ML prediction models were grouped as detailed in Supplementary Appendix C.
In an attempt to reduce the number of comparisons, when multiple models from the same group were used in a single article (employing different factor selection and optimization methods), the analysis focused on the highest AUC value. The standard deviation (SD) for AUC was either extracted directly from the article, requested from the authors, converted from the 95% confidence interval (CI) according to the Cochrane Handbook recommendations, or imputed using the Iterative Imputer algorithm based on a Bayesian regression model (Python’s sklearn library).
Data on the AUC metrics for traditional scoring systems were also extracted if available. These systems included SOFA (Sequential Organ Failure Assessment), qSOFA (quick SOFA), NEWS/NEWS2 (National Early Warning Score), MEWS (Modified Early Warning Score), SAPS II (Simplified Acute Physiology Score), and SIRS (Systemic Inflammatory Response Syndrome). In cases where multiple traditional scoring systems were used, the best metric was considered.
2.4 Data analysis and synthesis
Traditional meta-analysis was conducted to calculate pooled AUCs. Inter-study heterogeneity was evaluated using the I-squared (I2) statistic and the Cochrane Q test; random-effects model (restricted maximum–likelihood, REML) was used. Statistical significance was set at 0.05 for hypothesis testing. We conducted a meta-regression analysis, leveraging the REML random-effects model, to ascertain if the AUC metrics might be affected by covariates such as study design and ML model characteristics (21). All covariates were first tested in a univariate model, significant covariates were then considered for a multivariable model. The results of the meta-regression were graphically represented using bubble-plots.
We also conducted a frequentist, random-effects Network Meta-Analysis (NMA) using CINeMA (Confidence in Network Meta-Analysis) approach (22), CINeMA software (23), ROB-MEN web application (24) and STATA 17.0 (StataCorp, College Station, TX) software. Articles were included in the NMA if they compared any two ML models with different ML models or any ML model with a traditional scoring system. The Mean Difference (MD) with corresponding 95% CI was calculated for AUCs. Results of NMA were presented using network plots, league tables, contribution tables and NMA forest plots. To assess between-study heterogeneity, we utilized Bayesian NMA with τ2 calculation. A τ2 value exceeding the clinically important effect size (MD ≥ 0.15) indicated significant heterogeneity.
2.5 Internal validity and risk of bias assessment
The internal validity and risk of bias were assessed by three independent reviewers (MY, AS, IK) using the ‘Quality Assessment of Diagnostic Accuracy Studies’ (QUADAS-2) tool (25) combined with an adapted version of the ‘Joanna Briggs Institute Critical Appraisal checklist for analytical cross-sectional studies’ (26) (Supplementary Table S2). Publication bias and small-study effects were assessed using Bayesian NMA meta-regression and funnel plot analysis (for comparisons with 10 or more studies). The certainty of evidence was assessed with GRADE methodology integrated in CINeMA approach. We conducted a sensitivity analysis using studies with low to moderate risk of bias.
3 Results
3.1 Study characteristics
The initial literature search identified 3,953 studies from various databases, with an additional 24 studies from other sources (Figure 1). After removing duplicates and abstract screening, 97 papers underwent eligibility screening. A total of 256 models from 73 studies (457,932 septic patients) were included (14, 27–98) with major exclusions list presented in Supplementary Table S1. The specialty journal with the largest number of articles was Critical Care Medicine (39, 41, 53, 62, 87).
Most of the studies included in the analysis were conducted in the ICU (n = 49; 67.1%), followed by hospital wards (n = 12; 16.4%) and emergency departments (ED, n = 9; 12.3%) (Supplementary Tables S4–S8). The median sepsis prevalence across the studies was 14.3% (IQR 7.3–32.4%), with the mean patient age ranging from 35 to 70 years. The median (IQR) mortality rate was 2.3% (6.9–14.8%). Sepsis was most frequently defined by the Sepsis-3 criteria (57.5%), with other definitions including Sepsis-2, ICD-9, ICD-10, and SIRS. Prediction windows varied widely, ranging from immediate (0 h) to 7 days. External validation was performed in 16 studies (21.9%), and imputation techniques were employed in 44 studies (60.3%). Notably, 53% of studies utilized public datasets such as MIMIC, eICU, and the Computing in Cardiology Challenge 2019, while the remaining studies relied on proprietary hospital datasets.
3.2 Pooled AUCs
The pooled AUC for machine learning models was 0.825 (95% CI 0.809–0.840, p < 0.001) across 73 studies (Supplementary Table S9). In comparison, the AUC for the SOFA score was 0.667 (95% CI 0.586–0.748) across 17 studies, for qSOFA 0.612 (95% CI 0.574–0.650) across 16 studies, for NEWS/NEWS2 0.719 (95% CI 0.674–0.764) across 9 studies, for MEWS 0.651 (95% CI 0.612–0.690) across 12 studies, for SIRS 0.666 (95% CI 0.643–0.688) across 19 studies, and for SAPS II 0.662 (95% CI 0.589–0.736) across 2 studies (all p < 0.001, Supplementary Table S9). Heterogeneity across studies was high (I2 > 95%, p < 0.001).
3.3 Network meta-analysis
3.3.1 ML vs. scoring systems
All ML models exhibited a significant performance advantage over traditional scoring systems when performing a NMA (Figures 2, 3, Supplementary Tables S10–S12).
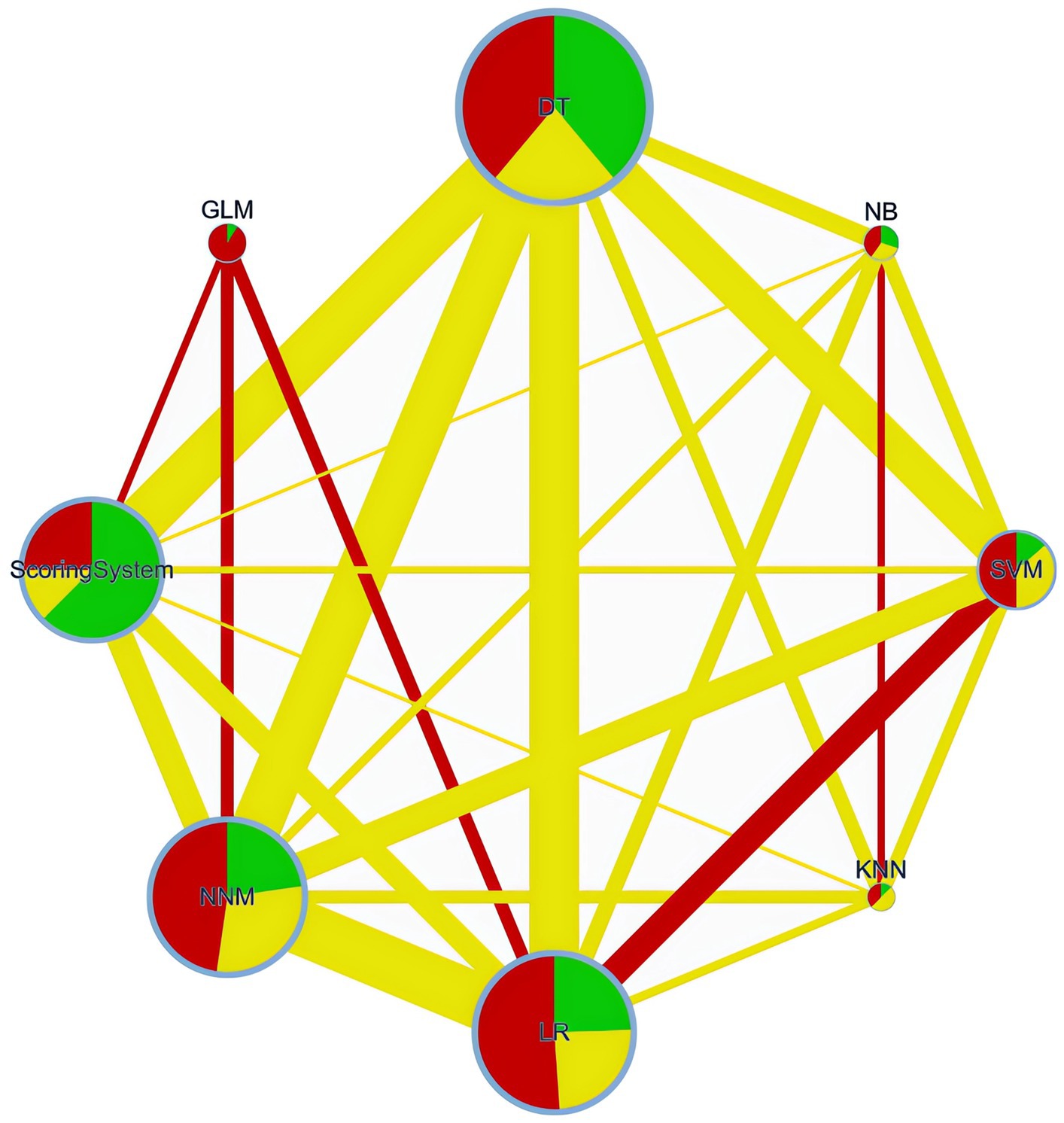
Figure 2. Network plot. DT, Decision Tree; NNM, Neural Network Model; SVM, Support Vector Machine; LR, Logistic Regression; NB, Naïve Bayes; GLM, Generalized Linear Model; KNN, K-Nearest Neighbors.
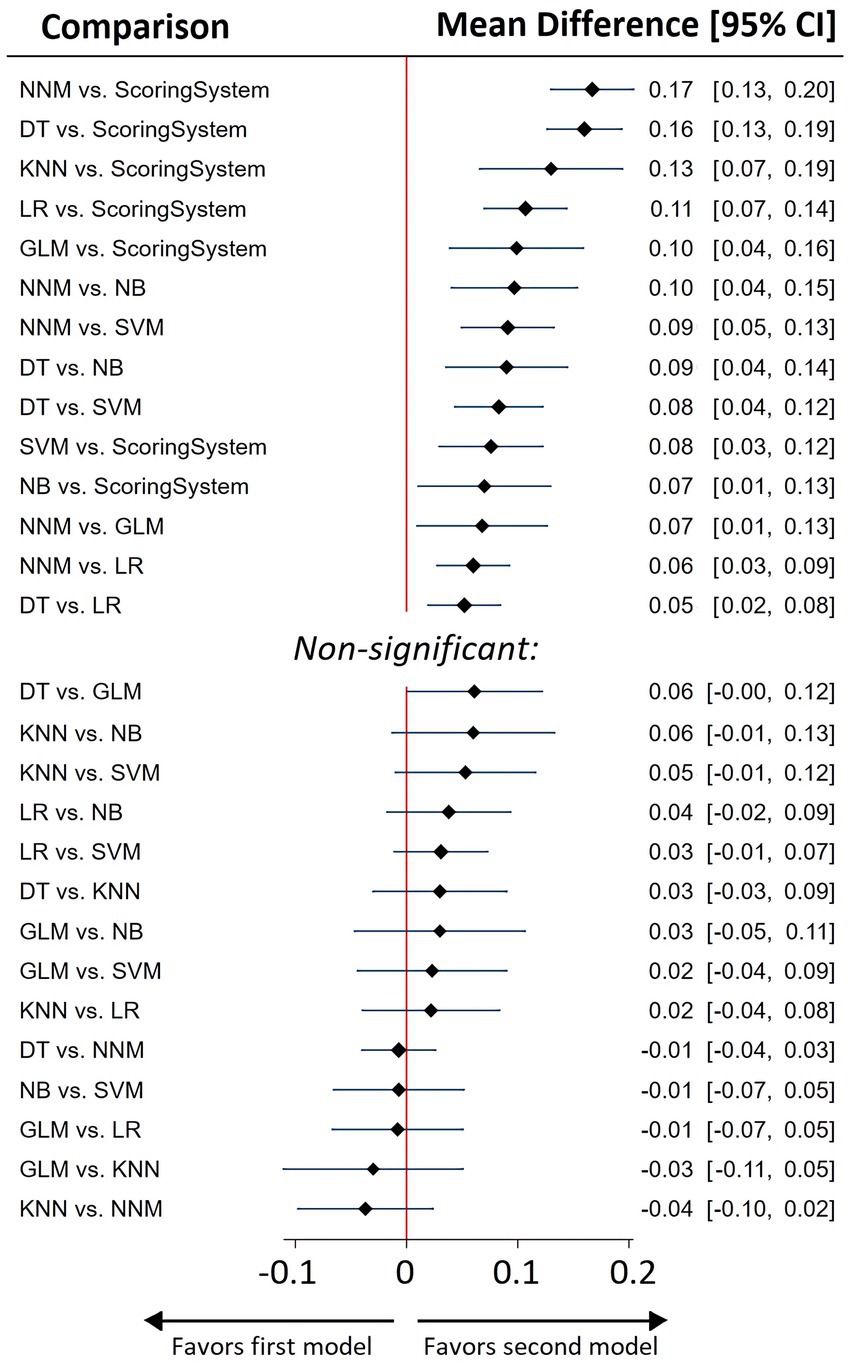
Figure 3. Network meta-analysis summary forest plot for predictive efficacy of various ML models and best of scoring system used for sepsis prediction.
A network plot is a visual tool in network meta-analyses, showing interventions (groups) as nodes and their direct comparisons as connecting lines. This visual tool helps in understanding the complex relationships and the extent of evidence available for each comparison in the network meta-analysis.
Network of retrospective diagnostic test accuracy studies comparing the AUCs of various machine learning models and best of scoring system used. The size of nodes and width of the edges are proportional to the number of studies. The colors of edges and nodes refer to the average risk of bias: low (green), moderate (yellow), and high (red).
3.3.2 ML models
As indicated by the NMA results, Neural Network Models (NNM) and Decision Tree (DT) models exhibited the highest AUC metrics (Figure 3, Supplementary Tables S11, S12).
3.4 Meta-regression
In the multivariable model, only ML model type, dataset type (with non-freely available hospital datasets showing higher AUCs), and prediction window (showing a negative association) had significant contributions to the AUC. (Supplementary Table S13, Supplementary Figures S1, S2).
3.5 Risk of bias and GRADE assessment
The overall risk of bias of the 73 enrolled studies was judged as ‘low’ in 29 studies, with ‘some concerns’ in 14 studies and ‘high’ in 30 studies (Supplementary Figure S3). The main sources of bias identified were insufficient description of the study population and data sources, along with the use of different sepsis definitions.
Risk of bias bar chart is presented in Supplementary Figure S4. Publication bias and small-study effects assessment results are summarized in Supplementary Table S14 and Supplementary Figure S5. Between-study variance was not significant (τ2 = 0.095, with the clinically important effect size stated as 0.1). Contribution matrices are presented in Supplementary Tables S15, S16.
The CINeMA ratings can be found in Supplementary Figure S6. The level of evidence supporting the superiority of ML models over traditional scoring systems was categorized as ‘low’.
4 Discussion
4.1 Key findings
This network meta-analysis is the first to comprehensively evaluate the performance of (ML) models in sepsis prediction, demonstrating that ML algorithms, particularly neural network models and decision trees, significantly outperform traditional scoring systems. These findings underscore the enhanced ability of ML models to analyse and interpret complex clinical data, pointing to a potential paradigm shift in sepsis prediction strategies.
A critical aspect of our findings relates to the impact of the type of ML model and the nature of the dataset on model performance. The choice of the ML model itself emerged as a significant determinant of model performance in our study. This finding indicates that the inherent characteristics and algorithms of different ML models substantially influence their ability to predict sepsis effectively. Models utilizing freely available datasets exhibited lower AUCs, which could be a result of overfitting in models based on non-freely available hospital datasets.
Another key finding from our study is the temporal dynamics in prediction accuracy. We observed a negative association between prediction window and AUC, indicating that ML models are more accurate in short-term than in long-term sepsis prediction.
Interestingly, factors such as the size of the training dataset, sepsis prevalence, the department in which the study was conducted, the presence of imputation and external validation, the use of laboratory indicators, and the number of predictors did not significantly influence the quality of the predictive models.
4.2 Relationship with previous studies
The results of our systematic review and meta-analysis can be compared with those of 3 previous meta-analyses. Islam et al. in 2019 were the first demonstrated that ML approach outperforms existing scoring systems in predicting sepsis (6). Fleuren et al. (15) suggested that ML models can accurately predict the onset of sepsis with good discrimination in retrospective cohorts, and this study was the first to indicate that the choice of ML model could impact AUC. The authors also suggested that NNM had advantages over DT, and that the inclusion of body temperature and laboratory indicators enhanced prediction quality. The only other meta-analysis performed so far demonstrated the superiority of XGBoost and random forest models but with high heterogeneity (I2) (16). In other systematic reviews, quantitative meta-analysis was not conducted due to significant heterogeneity among studies (10–13). We were the first to apply a NMA technique, which allowed us to overcome high heterogeneity of previous meta-analyses. This approach enables comparisons between two ML models or an ML model and a traditional scoring system within the same study on a single patient cohort, employing a unified approach and standardized definition of sepsis. In our research, NNM did not demonstrate superiority over DT, and the use of body temperature and laboratory indicators as predictors did not enhance the predictive quality.
4.3 Significance of the study findings
Our network meta-analysis, which evaluated 73 articles encompassing 457,932 septic patients, revealed that ML algorithms significantly outperform traditional sepsis scoring systems. The integration of ML into sepsis prediction marks a significant step forward in improving the early diagnosis and management of this life-threatening condition in emergency and intensive care settings. Unlike traditional scoring systems, ML models can process vast amounts of real-time clinical data, offering early warning systems that may identify sepsis before the appearance of clinical symptoms, thereby facilitating timely and targeted interventions. This has profound implications for clinical practice, as prompt treatments such as early antibiotic administration are known to significantly improve patient outcomes, particularly when initiated within the ‘golden hour’ (3–5).
Our study makes a key contribution by identifying the factors that impact the effectiveness of sepsis prediction models. Specifically, we found that the number of predictors and sepsis prevalence do not substantially influence model performance, challenging the traditional assumption that larger datasets and a perfectly balanced cohort (with a 50/50% split) are essential for robust predictions. Instead, our findings underscore the importance of data quality and the careful selection of relevant predictors, which has direct implications for how ML models should be developed and deployed in real-world clinical environments.
The heterogeneity in external validation and imputation methods across studies underscores a significant gap in standardizing ML model development and validation for sepsis prediction. While we did not find a notable impact of external validation on AUC, its role in enhancing the robustness and generalizability of prediction models should not be underestimated. Furthermore, it’s noteworthy that even in the context of established and stringent diagnostic guidelines for sepsis, there exists a number of studies where researchers have opted to utilize alternative definitions of sepsis in their studies.
Another notable aspect of our research pertains to the ‘black box’ nature of some ML models, which limits the clinician’s ability to understand the logic behind decision-making. Our study demonstrates that the use of DT is not inferior to the more complex NNM. This is a pivotal finding as DT models offer greater transparency in decision-making processes, which is crucial for clinical applications where understanding the rationale behind predictions is as important as the predictions themselves.
While ML models show substantial promise in predicting sepsis onset, their clinical utility remains limited by the challenge of initiating treatment before the appearance of clinical symptoms.
4.4 Strengths and limitations
This research is the first to quantitatively demonstrate the superiority of ML models over traditional scoring systems using NMA. Furthermore, this study is the first to employ NMA to reveal the advantages of NNM and DT over other ML models in the prediction of sepsis. Through meta-regression, we identified several critical factors that influence model performance, providing valuable insights for future model development. The application of the CINeMA approach provided a structured methodology to rate the certainty of our evidence, enhancing the reliability of our findings.
However, limitations of our study must be acknowledged: we found high clinical heterogeneity among the included studies and therefore used random-effects modelling and sensitivity analyses; while the AUC was pragmatically chosen as the summary measure, it may not be as effective in imbalanced datasets, yet it remains the most frequently reported measure in this field.
4.5 Future studies and prospects
The growing body of evidence supporting the advantages of ML models over traditional scoring systems in sepsis prediction underscores the need to integrate these technologies into routine clinical practice. Future research should focus on conducting well-structured prospective trials to evaluate how ML-predicted sepsis outcomes influence the timing and initiation of antibiotic therapy. A critical component of these trials will be assessing the time interval between ML model predictions and clinical recognition by healthcare providers, as delays in treatment initiation can significantly affect patient outcomes.
We propose a randomized, double-blind controlled trial comparing the efficacy of early antibiotic therapy initiated based on ML predictions versus placebo during this pre-recognition window. Such a study could provide definitive evidence regarding the clinical utility of ML-based early warning systems and their potential to reduce mortality and morbidity in sepsis by enabling earlier interventions.
5 Conclusion
Our systematic review and network meta-analysis revealed that machine learning models, specifically neural network models and decision trees, exhibit superior performance in predicting sepsis compared to traditional scoring systems. This study highlights the significant impact of machine learning model type and dataset characteristics on prediction accuracy. Despite the promise of machine learning models in clinical settings, their potential is yet to be fully realized due to study heterogeneity and the variability in sepsis definitions. To bridge this gap, there is an urgent need for standardized reporting and validation frameworks, ensuring that machine learning tools are both reliable and generalizable in diverse clinical settings.
Data availability statement
The original contributions presented in the study are included in the article/Supplementary material, further inquiries can be directed to the corresponding author.
Author contributions
MY: Data curation, Formal analysis, Investigation, Writing – original draft, Writing – review & editing. GL: Investigation, Writing – original draft, Writing – review & editing. LB: Data curation, Formal analysis, Investigation, Writing – original draft, Writing – review & editing. PP: Formal analysis, Writing – original draft, Writing – review & editing. KK: Conceptualization, Methodology, Writing – original draft, Writing – review & editing. AS: Data curation, Formal analysis, Investigation, Writing – original draft, Writing – review & editing. IK: Data curation, Formal analysis, Investigation, Writing – original draft, Writing – review & editing. MS: Visualization, Writing – original draft, Writing – review & editing. AY: Supervision, Writing – original draft, Writing – review & editing. VL: Conceptualization, Methodology, Writing – original draft, Writing – review & editing.
Funding
The author(s) declare that no financial support was received for the research, authorship, and/or publication of this article.
Conflict of interest
The authors declare that the research was conducted in the absence of any commercial or financial relationships that could be construed as a potential conflict of interest.
The author(s) declared that they were an editorial board member of Frontiers, at the time of submission. This had no impact on the peer review process and the final decision.
Publisher’s note
All claims expressed in this article are solely those of the authors and do not necessarily represent those of their affiliated organizations, or those of the publisher, the editors and the reviewers. Any product that may be evaluated in this article, or claim that may be made by its manufacturer, is not guaranteed or endorsed by the publisher.
Supplementary material
The Supplementary material for this article can be found online at: https://www.frontiersin.org/articles/10.3389/fmed.2024.1491358/full#supplementary-material
References
1. Fleischmann, C, Scherag, A, Adhikari, NKJ, Hartog, CS, Tsaganos, T, Schlattmann, P, et al. Assessment of global incidence and mortality of hospital-treated sepsis current estimates and limitations. Am J Respir Crit Care Med. (2016) 193:259–72. doi: 10.1164/rccm.201504-0781OC
2. Evans, L, Rhodes, A, Alhazzani, W, Antonelli, M, Coopersmith, CM, French, C, et al. Surviving sepsis campaign: international guidelines for management of sepsis and septic shock 2021. Intensive Care Med. (2021) 47:1181–247. doi: 10.1007/s00134-021-06506-y
3. Rababa, M, Hamad, DB, and Hayajneh, AA. Sepsis assessment and management in critically ill adults: a systematic review. PLoS One. (2022) 17:e0270711. doi: 10.1371/journal.pone.0270711
4. Il, KH, and Park, S. Sepsis: early recognition and optimized treatment. Tuberc Respir Dis (Seoul). (2019) 82:6–14. doi: 10.4046/trd.2018.0041
5. Im, Y, Kang, D, Ko, RE, Lee, YJ, Lim, SY, Park, S, et al. Time-to-antibiotics and clinical outcomes in patients with sepsis and septic shock: a prospective nationwide multicenter cohort study. Crit Care. (2022) 26:19. doi: 10.1186/s13054-021-03883-0
6. Islam, MM, Nasrin, T, Walther, BA, Wu, CC, Yang, HC, and Li, YC. Prediction of sepsis patients using machine learning approach: a meta-analysis. Comput Methods Prog Biomed. (2019) 170:1–9. doi: 10.1016/j.cmpb.2018.12.027
7. Schinkel, M, van der Poll, T, and Wiersinga, WJ. Artificial intelligence for early Sepsis detection a word of caution. Am J Respir Crit Care Med. (2023) 207:853–4. doi: 10.1164/rccm.202212-2284VP
8. van der Vegt, AH, Scott, IA, Dermawan, K, Schnetler, RJ, Kalke, VR, and Lane, PJ. Deployment of machine learning algorithms to predict sepsis: systematic review and application of the SALIENT clinical AI implementation framework. J Am Med Informatics Assoc. (2023) 30:1349–61. doi: 10.1093/jamia/ocad075
9. Komorowski, M, Green, A, Tatham, KC, Seymour, C, and Antcliffe, D. Sepsis biomarkers and diagnostic tools with a focus on machine learning. EBioMedicine. (2022) 86:104394. doi: 10.1016/j.ebiom.2022.104394
10. Moor, M, Rieck, B, Horn, M, Jutzeler, CR, and Borgwardt, K. Early prediction of Sepsis in the ICU using machine learning: a systematic review. Front Med. (2021) 8:607952. doi: 10.3389/fmed.2021.607952
11. Deng, HF, Sun, MW, Wang, Y, Zeng, J, Yuan, T, Li, T, et al. Evaluating machine learning models for sepsis prediction: a systematic review of methodologies. iScience. (2022) 25:103651. doi: 10.1016/j.isci.2021.103651
12. Yan, MY, Gustad, LT, and Nytrø, Ø. Sepsis prediction, early detection, and identification using clinical text for machine learning: a systematic review. J Am Med Informatics Assoc. (2022) 29:559–75. doi: 10.1093/jamia/ocab236
13. Kausch, SL, Moorman, JR, Lake, DE, and Keim-Malpass, J. Physiological machine learning models for prediction of sepsis in hospitalized adults: an integrative review. Intensive Crit Care Nurs. (2021) 65:103035. doi: 10.1016/j.iccn.2021.103035
14. Lauritsen, SM, Thiesson, B, Jørgensen, MJ, Riis, AH, Espelund, US, Weile, JB, et al. The framing of machine learning risk prediction models illustrated by evaluation of sepsis in general wards. Npj Digit Med. (2021) 4:1–12. doi: 10.1038/s41746-021-00529-x
15. Fleuren, LM, Klausch, TLT, Zwager, CL, Schoonmade, LJ, Guo, T, Roggeveen, LF, et al. Machine learning for the prediction of sepsis: a systematic review and meta-analysis of diagnostic test accuracy. Intensive Care Med. (2020) 46:383–400. doi: 10.1007/s00134-019-05872-y
16. Yang, Z, Cui, X, and Song, Z. Predicting sepsis onset in ICU using machine learning models: a systematic review and meta-analysis. BMC Infect Dis. (2023) 23:635–21. doi: 10.1186/s12879-023-08614-0
17. Hutton, B, Salanti, G, Caldwell, DM, Chaimani, A, Schmid, CH, Cameron, C, et al. The PRISMA extension statement for reporting of systematic reviews incorporating network meta-analyses of health care interventions: checklist and explanations. Ann Intern Med. (2015) 162:777–84. doi: 10.7326/M14-2385
18. Higgins, JPT, Thomas, J, Chandler, J, Cumpston, M, Li, T, Page, MJ, et al. Cochrane handbook for systematic reviews of interventions. Cochrane Handb Syst Rev Interv. (2019):1–694. doi: 10.1002/9781119536604
19. Litmaps Literature Map Software for Lit Reviews and Research. (2023) Available at: https://www.litmaps.com/ (Accessed November 24, 2023).
20. Singer, M, Deutschman, CS, Seymour, C, Shankar-Hari, M, Annane, D, Bauer, M, et al. The third international consensus definitions for sepsis and septic shock (sepsis-3). JAMA. (2016) 315:801–10. doi: 10.1001/jama.2016.0287
21. Harbord, RM, and Higgins, JPT. Meta-regression in Stata. Stata J. (2008) 8:493–519. doi: 10.1177/1536867x0800800403
22. Nikolakopoulou, A, Higgins, JPT, Papakonstantinou, T, Chaimani, A, Del, GC, Egger, M, et al. Cinema: an approach for assessing confidence in the results of a network meta-analysis. PLoS Med. (2020) 17:e1003082. doi: 10.1371/JOURNAL.PMED.1003082
23. Papakonstantinou, T, Nikolakopoulou, A, Higgins, JPT, Egger, M, and Salanti, G. CINeMA: software for semiautomated assessment of the confidence in the results of network meta-analysis. Campbell Syst Rev. (2020) 16:e1080. doi: 10.1002/cl2.1080
24. Chiocchia, V, Nikolakopoulou, A, Higgins, JPT, Page, MJ, Papakonstantinou, T, Cipriani, A, et al. ROB-MEN: a tool to assess risk of bias due to missing evidence in network meta-analysis. BMC Med. (2021) 19:304. doi: 10.1186/s12916-021-02166-3
25. Whiting, PF, Rutjes, AWS, Westwood, ME, Mallett, S, Deeks, JJ, Reitsma, JB, et al. Quadas-2: a revised tool for the quality assessment of diagnostic accuracy studies. Ann Intern Med. (2011) 155:529–36. doi: 10.7326/0003-4819-155-8-201110180-00009
26. Aromataris, E, Munn, Z, Moola, S, Tufanaru, C, Sears, K, Sfetcu, R, et al. Checklist for analytical cross sectional studies. (2020). Available at: https://synthesismanual.jbi.global/ (Accessed November 24, 2023).
27. Abromavičius, V, Plonis, D, Tarasevičius, D, and Serackis, A. Two-stage monitoring of patients in intensive care unit for sepsis prediction using non-overfitted machine learning models. Electron. (2020) 9:1–14. doi: 10.3390/electronics9071133
28. Camacho-Cogollo, JE, Bonet, I, Gil, B, and Iadanza, E. Machine learning models for early prediction of Sepsis on large healthcare datasets. Electron. (2022) 11:1507. doi: 10.3390/electronics11091507
29. Chen, M, and Hernández, A. Towards an explainable model for Sepsis detection based on sensitivity analysis. Irbm. (2022) 43:75–86. doi: 10.1016/j.irbm.2021.05.006
30. Chen, Q, Li, R, Lin, CC, Lai, C, Chen, D, Qu, H, et al. Transferability and interpretability of the sepsis prediction models in the intensive care unit. BMC Med Inform Decis Mak. (2022) 22:343–10. doi: 10.1186/s12911-022-02090-3
31. Chen, C, Chen, B, Yang, J, Li, X, Peng, X, Feng, Y, et al. Development and validation of a practical machine learning model to predict sepsis after liver transplantation. Ann Med. (2023) 55:624–33. doi: 10.1080/07853890.2023.2179104
32. Choi, JS, Trinh, TX, Ha, J, Yang, MS, Lee, Y, Kim, YE, et al. Implementation of complementary model using optimal combination of hematological parameters for Sepsis screening in patients with fever. Sci Rep. (2020) 10:273–10. doi: 10.1038/s41598-019-57107-1
33. Delahanty, RJ, Alvarez, JA, Flynn, LM, Sherwin, RL, and Jones, SS. Development and evaluation of a machine learning model for the early identification of patients at risk for Sepsis. Ann Emerg Med. (2019) 73:334–44. doi: 10.1016/j.annemergmed.2018.11.036
34. Desautels, T, Calvert, J, Hoffman, J, Jay, M, Kerem, Y, Shieh, L, et al. Prediction of sepsis in the intensive care unit with minimal electronic health record data: a machine learning approach. JMIR Med Informatics. (2016) 4:e28. doi: 10.2196/medinform.5909
35. Duan, Y, Huo, J, Chen, M, Hou, F, Yan, G, Li, S, et al. Early prediction of sepsis using double fusion of deep features and handcrafted features. Appl Intell. (2023) 53:17903–19. doi: 10.1007/s10489-022-04425-z
36. El-Rashidy, N, Abuhmed, T, Alarabi, L, El-Bakry, HM, Abdelrazek, S, Ali, F, et al. Sepsis prediction in intensive care unit based on genetic feature optimization and stacked deep ensemble learning. Neural Comput & Applic. (2022) 34:3603–32. doi: 10.1007/s00521-021-06631-1
37. Fagerström, J, Bång, M, Wilhelms, D, and Chew, MS. LiSep LSTM: a machine learning algorithm for early detection of septic shock. Sci Rep. (2019) 9:15132–8. doi: 10.1038/s41598-019-51219-4
38. Amrollahi, F, Shashikumar, SP, Razmi, F, and Nemati, S. Contextual Embeddings from clinical notes improves prediction of Sepsis. AMIA Annu Symp Proc. (2020) 2020:197–202.
39. Faisal, M, Scally, A, Richardson, D, Beatson, K, Howes, R, Speed, K, et al. Development and external validation of an automated computer-aided risk score for predicting sepsis in emergency medical admissions using the patient’s first electronically recorded vital signs and blood test results. Crit Care Med. (2018) 46:612–8. doi: 10.1097/CCM.0000000000002967
40. Gholamzadeh, M, Abtahi, H, and Safdari, R. Comparison of different machine learning algorithms to classify patients suspected of having sepsis infection in the intensive care unit. Inform Med Unlock. (2023) 38:101236. doi: 10.1016/j.imu.2023.101236
41. Giannini, HM, Ginestra, JC, Chivers, C, Draugelis, M, Hanish, A, Schweickert, WD, et al. A machine learning algorithm to predict severe Sepsis and septic shock: development, implementation, and impact on clinical practice. Crit Care Med. (2019) 47:1485–92. doi: 10.1097/CCM.0000000000003891
42. Goh, KH, Wang, L, Yeow, AYK, Poh, H, Li, K, Yeow, JJL, et al. Artificial intelligence in sepsis early prediction and diagnosis using unstructured data in healthcare. Nat Commun. (2021) 12:711–10. doi: 10.1038/s41467-021-20910-4
43. Horng, S, Sontag, DA, Halpern, Y, Jernite, Y, Shapiro, NI, and Nathanson, LA. Creating an automated trigger for sepsis clinical decision support at emergency department triage using machine learning. PLoS One. (2017) 12:e0174708. doi: 10.1371/journal.pone.0174708
44. Ibrahim, ZM, Wu, H, Hamoud, A, Stappen, L, Dobson, RJB, and Agarossi, A. On classifying sepsis heterogeneity in the ICU: insight using machine learning. J Am Med Informatics Assoc. (2020) 27:437–43. doi: 10.1093/jamia/ocz211
45. Kaji, DA, Zech, JR, Kim, JS, Cho, SK, Dangayach, NS, Costa, AB, et al. An attention based deep learning model of clinical events in the intensive care unit. PLoS One. (2019) 14:e0211057. doi: 10.1371/journal.pone.0211057
46. Kam, HJ, and Kim, HY. Learning representations for the early detection of sepsis with deep neural networks. Comput Biol Med. (2017) 89:248–55. doi: 10.1016/j.compbiomed.2017.08.015
47. Khojandi, A, Tansakul, V, Li, X, Koszalinski, RS, and Paiva, W. Prediction of Sepsis and in-hospital mortality using electronic health records. Methods Inf Med. (2018) 57:185–93. doi: 10.3414/ME18-01-0014
48. Kijpaisalratana, N, Sanglertsinlapachai, D, Techaratsami, S, Musikatavorn, K, and Saoraya, J. Machine learning algorithms for early sepsis detection in the emergency department: a retrospective study. Int J Med Inform. (2022) 160:104689. doi: 10.1016/j.ijmedinf.2022.104689
49. Aşuroğlu, T, and Oğul, H. A deep learning approach for sepsis monitoring via severity score estimation. Comput Methods Prog Biomed. (2021) 198:105816. doi: 10.1016/j.cmpb.2020.105816
50. Kuo, YY, Huang, ST, and Chiu, HW. Applying artificial neural network for early detection of sepsis with intentionally preserved highly missing real-world data for simulating clinical situation. BMC Med Inform Decis Mak. (2021) 21:290–11. doi: 10.1186/s12911-021-01653-0
51. Kwon, J, Myoung, LYR, Jung, MS, Lee, YJ, Jo, YY, Kang, DY, et al. Deep-learning model for screening sepsis using electrocardiography. Scand J Trauma Resusc Emerg Med. (2021) 29:1–12. doi: 10.1186/s13049-021-00953-8
52. Lauritsen, SM, Kalør, ME, Kongsgaard, EL, Lauritsen, KM, Jørgensen, MJ, Lange, J, et al. Early detection of sepsis utilizing deep learning on electronic health record event sequences. Artif Intell Med. (2020) 104:101820. doi: 10.1016/j.artmed.2020.101820
53. Li, X, Xu, X, Xie, F, Xu, X, Sun, Y, Liu, X, et al. A time-phased machine learning model for real-time prediction of Sepsis in critical care. Crit Care Med. (2020) 48:E884–8. doi: 10.1097/CCM.0000000000004494
54. Lin, PC, Chen, KT, Chen, HC, Islam, MM, and Lin, MC. Machine learning model to identify sepsis patients in the emergency department: algorithm development and validation. J Pers Med. (2021) 11:1055. doi: 10.3390/jpm11111055
55. Liu, Z, Khojandi, A, Li, X, Mohammed, A, Davis, RL, and Kamaleswaran, R. A machine learning–enabled partially observable Markov decision process framework for early Sepsis prediction. INFORMS J Comput. (2022) 34:2039–57. doi: 10.1287/ijoc.2022.1176
56. Liu, F, Yao, J, Liu, C, and Shou, S. Construction and validation of machine learning models for sepsis prediction in patients with acute pancreatitis. BMC Surg. (2023) 23:267–13. doi: 10.1186/s12893-023-02151-y
57. Maharjan, J, Thapa, R, Calvert, J, Attwood, MM, Shokouhi, S, Casie Chetty, S, et al. A new standard for Sepsis prediction algorithms: using time-dependent analysis for earlier clinically relevant alerts. SSRN Electron J. (2022). doi: 10.2139/ssrn.4130480
58. Mao, Q, Jay, M, Hoffman, JL, Calvert, J, Barton, C, Shimabukuro, D, et al. Multicentre validation of a sepsis prediction algorithm using only vital sign data in the emergency department, general ward and ICU. BMJ Open. (2018) 8:e017833. doi: 10.1136/bmjopen-2017-017833
59. McCoy, A, and Das, R. Reducing patient mortality, length of stay and readmissions through machine learning-based sepsis prediction in the emergency department, intensive care unit and hospital floor units. BMJ Open Qual. (2017) 6:e000158. doi: 10.1136/bmjoq-2017-000158
60. Bao, C, Deng, F, and Zhao, S. Machine-learning models for prediction of sepsis patients mortality. Med Intens. (2023) 47:315–25. doi: 10.1016/j.medine.2022.06.024
61. Moor, M, Bennett, N, Plečko, D, Horn, M, Rieck, B, Meinshausen, N, et al. Predicting sepsis using deep learning across international sites: a retrospective development and validation study. eClinicalMedicine. (2023) 62:102124. doi: 10.1016/j.eclinm.2023.102124
62. Nemati, S, Holder, A, Razmi, F, Stanley, MD, Clifford, GD, and Buchman, TG. An interpretable machine learning model for accurate prediction of Sepsis in the ICU. Crit Care Med. (2018) 46:547–53. doi: 10.1097/CCM.0000000000002936
63. Nesaragi, N, and Patidar, S. An explainable machine learning model for early prediction of Sepsis using ICU data. Infect Sepsis Dev. (2021). doi: 10.5772/intechopen.98957
64. Oei, SP, van Sloun, RJ, van der Ven, M, Korsten, HH, and Mischi, M. Towards early sepsis detection from measurements at the general ward through deep learning. Intell Med. (2021) 5:100042. doi: 10.1016/j.ibmed.2021.100042
65. Persson, I, Östling, A, Arlbrandt, M, Söderberg, J, and Becedas, D. A machine learning Sepsis prediction algorithm for intended intensive care unit use (NAVOY Sepsis): proof-of-concept study. JMIR Form Res. (2021) 5:e28000. doi: 10.2196/28000
66. Rafiei, A, Rezaee, A, Hajati, F, Gheisari, S, and Golzan, M. SSP: early prediction of sepsis using fully connected LSTM-CNN model. Comput Biol Med. (2021) 128:104110. doi: 10.1016/j.compbiomed.2020.104110
67. Rangan, ES, Pathinarupothi, RK, Anand, KJS, and Snyder, MP. Performance effectiveness of vital parameter combinations for early warning of sepsis - an exhaustive study using machine learning. JAMIA Open. (2022) 5:1–11. doi: 10.1093/jamiaopen/ooac080
68. Rosnati, M, and Fortuin, V. MGP-AttTCN: an interpretable machine learning model for the prediction of sepsis. PLoS One. (2021) 16:e0251248. doi: 10.1371/journal.pone.0251248
69. Sadasivuni, S, Saha, M, Bhatia, N, Banerjee, I, and Sanyal, A. Fusion of fully integrated analog machine learning classifier with electronic medical records for real-time prediction of sepsis onset. Sci Rep. (2022) 12:1–11. doi: 10.1038/s41598-022-09712-w
70. Sharma, DK, Lakhotia, P, Sain, P, and Brahmachari, S. Early prediction and monitoring of sepsis using sequential long short term memory model. Expert Syst. (2022) 39:e12798. doi: 10.1111/exsy.12798
71. Barton, C, Chettipally, U, Zhou, Y, Jiang, Z, Lynn-Palevsky, A, Le, S, et al. Evaluation of a machine learning algorithm for up to 48-hour advance prediction of sepsis using six vital signs. Comput Biol Med. (2019) 109:79–84. doi: 10.1016/j.compbiomed.2019.04.027
72. Scherpf, M, Gräßer, F, Malberg, H, and Zaunseder, S. Predicting sepsis with a recurrent neural network using the MIMIC III database. Comput Biol Med. (2019) 113:103395. doi: 10.1016/j.compbiomed.2019.103395
73. Schamoni, S, Lindner, HA, Schneider-Lindner, V, Thiel, M, and Riezler, S. Leveraging implicit expert knowledge for non-circular machine learning in sepsis prediction. Artif Intell Med. (2019) 100:101725. doi: 10.1016/j.artmed.2019.101725
74. Shashikumar, SP, Stanley, MD, Sadiq, I, Li, Q, Holder, A, Clifford, GD, et al. Early sepsis detection in critical care patients using multiscale blood pressure and heart rate dynamics. J Electrocardiol. (2017) 50:739–43. doi: 10.1016/j.jelectrocard.2017.08.013
75. Shashikumar, SP, Li, Q, Clifford, GD, and Nemati, S. Multiscale network representation of physiological time series for early prediction of sepsis. Physiol Meas. (2017) 38:2235–48. doi: 10.1088/1361-6579/aa9772
76. Shashikumar, SP, Josef, CS, Sharma, A, and Nemati, S. DeepAISE – an interpretable and recurrent neural survival model for early prediction of sepsis. Artif Intell Med. (2021) 113:102036. doi: 10.1016/j.artmed.2021.102036
77. Singh, YV, Singh, P, Khan, S, and Singh, RS. A machine learning model for early prediction and detection of Sepsis in intensive care unit patients. J Healthc Eng. (2022) 2022:1–11. doi: 10.1155/2022/9263391
78. Shimabukuro, DW, Barton, CW, Feldman, MD, Mataraso, SJ, and Das, R. Effect of a machine learning-based severe sepsis prediction algorithm on patient survival and hospital length of stay: a randomised clinical trial. BMJ Open Respir Res. (2017) 4:e000234. doi: 10.1136/bmjresp-2017-000234
79. Taneja, I, Reddy, B, Damhorst, G, Dave Zhao, S, Hassan, U, Price, Z, et al. Combining biomarkers with EMR data to identify patients in different phases of Sepsis. Sci Rep. (2017) 7:10800–12. doi: 10.1038/s41598-017-09766-1
80. Tang, G, Luo, Y, Lu, F, Li, W, Liu, X, Nan, Y, et al. Prediction of Sepsis in COVID-19 using laboratory indicators. Front Cell Infect Microbiol. (2021) 10:586054. doi: 10.3389/fcimb.2020.586054
81. Valik, JK, Ward, L, Tanushi, H, Johansson, AF, Färnert, A, Mogensen, ML, et al. Predicting sepsis onset using a machine learned causal probabilistic network algorithm based on electronic health records data. Sci Rep. (2023) 13:11760–12. doi: 10.1038/s41598-023-38858-4
82. Bedoya, AD, Futoma, J, Clement, ME, Corey, K, Brajer, N, Lin, A, et al. Machine learning for early detection of sepsis: an internal and temporal validation study. JAMIA Open. (2020) 3:252–60. doi: 10.1093/jamiaopen/ooaa006
83. van Wyk, F, Khojandi, A, Mohammed, A, Begoli, E, Davis, RL, and Kamaleswaran, R. A minimal set of physiomarkers in continuous high frequency data streams predict adult sepsis onset earlier. Int J Med Inform. (2019) 122:55–62. doi: 10.1016/j.ijmedinf.2018.12.002
84. Wang, D, Li, J, Sun, Y, Ding, X, Zhang, X, Liu, S, et al. A machine learning model for accurate prediction of Sepsis in ICU patients. Front Public Heal. (2021) 9:754348. doi: 10.3389/fpubh.2021.754348
85. Wang, Z, and Yao, B. Multi-branching temporal convolutional network for Sepsis prediction. IEEE J Biomed Heal Inform. (2022) 26:876–87. doi: 10.1109/JBHI.2021.3092835
86. Wong, A, Otles, E, Donnelly, JP, Krumm, A, McCullough, J, DeTroyer-Cooley, O, et al. External validation of a widely implemented proprietary sepsis prediction model in hospitalized patients. JAMA Intern Med. (2021) 181:1065–70. doi: 10.1001/jamainternmed.2021.2626
87. Yang, M, Liu, C, Wang, X, Li, Y, Gao, H, Liu, X, et al. An explainable artificial intelligence predictor for early detection of Sepsis. Crit Care Med. (2020) 48:E1091–6. doi: 10.1097/CCM.0000000000004550
88. Yang, D, Kim, J, Yoo, J, Cha, WC, and Paik, H. Identifying the risk of Sepsis in patients with Cancer using digital health care records: machine learning-based approach. JMIR Med Inform. (2022) 10:e37689. doi: 10.2196/37689
89. Yu, SC, Gupta, A, Betthauser, KD, Lyons, PG, Lai, AM, Kollef, MH, et al. Sepsis prediction for the general Ward setting. Front Digit Heal. (2022) 4:848599. doi: 10.3389/fdgth.2022.848599
90. Yuan, KC, Tsai, LW, Lee, KH, Cheng, YW, Hsu, SC, Lo, YS, et al. The development an artificial intelligence algorithm for early sepsis diagnosis in the intensive care unit. Int J Med Inform. (2020) 141:104176. doi: 10.1016/j.ijmedinf.2020.104176
91. Zargoush, M, Sameh, A, Javadi, M, Shabani, S, Ghazalbash, S, and Perri, D. The impact of recency and adequacy of historical information on sepsis predictions using machine learning. Sci Rep. (2021) 11:20869. doi: 10.1038/s41598-021-00220-x
92. Zhang, D, Yin, C, Hunold, KM, Jiang, X, Caterino, JM, and Zhang, P. An interpretable deep-learning model for early prediction of sepsis in the emergency department. Patterns. (2021) 2:100196. doi: 10.1016/j.patter.2020.100196
93. Bloch, E, Rotem, T, Cohen, J, Singer, P, and Aperstein, Y. Machine learning models for analysis of vital signs dynamics: a case for Sepsis onset prediction. J Healthc Eng. (2019) 2019:1–11. doi: 10.1155/2019/5930379
94. Zhang, TY, Zhong, M, Cheng, YZ, and Zhang, MW. An interpretable machine learning model for real-time sepsis prediction based on basic physiological indicators. Eur Rev Med Pharmacol Sci. (2023) 27:4348–56. doi: 10.26355/eurrev_202305_32439
95. Zhang, S, Duan, Y, Hou, F, Yan, G, Li, S, Wang, H, et al. Early prediction of sepsis using a high-order Markov dynamic Bayesian network (HMDBN) classifier. Appl Intell. (2023) 53:26384–99. doi: 10.1007/s10489-023-04920-x
96. Zhao, X, Shen, W, and Wang, G. Early prediction of Sepsis based on machine learning algorithm. Comput Intell Neurosci. (2021) 2021:6522633. doi: 10.1155/2021/6522633
97. Burdick, H, Pino, E, Gabel-Comeau, D, Gu, C, Roberts, J, Le, S, et al. Validation of a machine learning algorithm for early severe sepsis prediction: a retrospective study predicting severe sepsis up to 48 h in advance using a diverse dataset from 461 US hospitals. BMC Med Inform Decis Mak. (2020) 20:1–10. doi: 10.1186/s12911-020-01284-x
Keywords: sepsis, machine learning, network meta-analysis, decision trees, neural networks
Citation: Yadgarov MY, Landoni G, Berikashvili LB, Polyakov PA, Kadantseva KK, Smirnova AV, Kuznetsov IV, Shemetova MM, Yakovlev AA and Likhvantsev VV (2024) Early detection of sepsis using machine learning algorithms: a systematic review and network meta-analysis. Front. Med. 11:1491358. doi: 10.3389/fmed.2024.1491358
Edited by:
Qinghe Meng, Upstate Medical University, United StatesReviewed by:
Mercedes Sanchez Barba, University of Salamanca, SpainKhan Md Hasib, Bangladesh University of Business and Technology, Bangladesh
Copyright © 2024 Yadgarov, Landoni, Berikashvili, Polyakov, Kadantseva, Smirnova, Kuznetsov, Shemetova, Yakovlev and Likhvantsev. This is an open-access article distributed under the terms of the Creative Commons Attribution License (CC BY). The use, distribution or reproduction in other forums is permitted, provided the original author(s) and the copyright owner(s) are credited and that the original publication in this journal is cited, in accordance with accepted academic practice. No use, distribution or reproduction is permitted which does not comply with these terms.
*Correspondence: Valery V. Likhvantsev, bGlrMDcwNEBnbWFpbC5jb20=