- 1The Endemic Disease (Thalassemia) Clinical Research Center of Jiangxi Province, Department of Hematology, First Affiliated Hospital of Gannan Medical University, Ganzhou, China
- 2The Third Clinical Medical College of Gannan Medical University, Ganzhou, China
- 3Department of Hematology, School of Medicine, Renji Hospital, Shanghai Jiao Tong University, Shanghai, China
Background: Optimizing the diagnosis and treatment of hematological diseases is a challenging yet crucial research area. Effective treatment plans typically require the comprehensive integration of cell morphology, immunology, cytogenetics, and molecular biology. These plans also consider patient-specific factors such as disease stage, age, and genetic mutation status. With the advancement of artificial intelligence (AI), more “AI + medical” application models are emerging. In clinical practice, many AI-assisted systems have been successfully applied to the diagnosis and treatment of hematological diseases, enhancing precision and efficiency and offering valuable solutions for clinical practice.
Objective: This study summarizes the research progress of various AI-assisted systems applied in the clinical diagnosis and treatment of hematological diseases, with a focus on their application in morphology, immunology, cytogenetics, and molecular biology diagnosis, as well as prognosis prediction and treatment.
Methods: Using PubMed, Web of Science, and other network search engines, we conducted a literature search on studies from the past 5 years using the main keywords “artificial intelligence” and “hematological diseases.” We classified the clinical applications of AI systems according to the diagnosis and treatment. We outline and summarize the current advancements in AI for optimizing the diagnosis and treatment of hematological diseases, as well as the difficulties and challenges in promoting the standardization of clinical diagnosis and treatment in this field.
Results: AI can significantly shorten turnaround times, reduce diagnostic costs, and accurately predict disease outcomes through applications in image-recognition technology, genomic data analysis, data mining, pattern recognition, and personalized medicine. However, several challenges remain, including the lack of AI product standards, standardized data, medical–industrial collaboration, and the complexity and non-interpretability of AI systems. In addition, regulatory gaps can lead to data privacy issues. Therefore, more research and improvements are needed to fully leverage the potential of AI to promote standardization of the clinical diagnosis and treatment of hematological diseases.
Conclusion: Our results serve as a reference point for the clinical diagnosis and treatment of hematological diseases and the development of AI-assisted clinical diagnosis and treatment systems. We offer suggestions for further development of AI in hematology and standardization of clinical diagnosis and treatment.
1 Introduction
During the past decade, comprehensive clinical diagnosis and treatment of hematological diseases have become increasingly challenging. Hematological diseases are numerous and complex, and include leukemia, lymphoma, myelodysplastic syndrome (MDS), multiple myeloma (MM), and myeloproliferative neoplasms. In the realm of hematological disorders, a thorough diagnostic approach frequently integrates morphological assessment, immunological evaluation, cytogenetic analysis, and molecular biological techniques. While these diagnostic modalities are indeed effective, they impose significant demands on the clinicians’ expertise, detection methodologies, and experiential knowledge. Furthermore, the accuracy of test results improves with the number of samples, requiring substantial infrastructure investment. This makes it difficult to achieve full coverage in rural or underdeveloped areas, creating an uneven healthcare system. Most hematological diseases are refractory, and traditional clinical treatments such as general supportive therapy, immunotherapy, and radiotherapy are tailored to different diseases and systemic symptoms. In addition, it is often challenging to achieve high prognostic accuracy. Although new treatment options have been introduced in recent years, including targeted therapies, immunotherapy, and hematopoietic stem cell transplantation, some patients still experience poor outcomes and face potential drug resistance, post-transplantation relapse, and related complications.
Artificial intelligence (AI) represents a sophisticated technical solution that emulates human cognitive functions, whereas machine learning (ML) and deep learning (DL) are specialized subsets of AI dedicated to developing software systems capable of learning from data and enhancing their performance accordingly. Machine learning, which enables computers to derive insights from data without the need for explicit programming, serves as the foundational methodology for endowing machines with human-like intelligence. With the accumulation of massive datasets and the rapid development of AI, the medical research field is increasingly embracing the “AI + medical” model. AI algorithms, including DL, support vector machine (SVM), random forest (RF), genetic algorithms, and natural language processing (NLP), enable computers to ‘learn” (1). This allows them to quickly and accurately process vast amounts of medical data from different diagnostic modalities. AI has been widely applied to hematology-related morphological testing, immunological testing, chromosome karyotype analysis, gene sequencing, biomarker identification, drug development, risk stratification, and prognosis monitoring.
In recent years, advances AI technology have led to AI models that can achieve high efficiency and accuracy, comparable to or even surpassing human experts. In addition, the lower marginal cost of AI (the cost per additional sample after system training) and its ability to process massive amounts of data indicate its potential to build data analytics models that can be remotely accessed and dynamically tracked (2). This capability can optimize medical treatment protocols, personalize clinical treatment guidance, and help medical facilities to accurately allocate healthcare resources, thereby promoting the precision treatment of hematological disorders.
Several key scientific advances in AI have been made in regard to the diagnosis and treatment of hematological diseases. For example, in the comprehensive typing diagnosis, AI-assisted detection systems such as ML-based CellaVision (FDA approved) (3), Scopio Labs X100 (FDA approved) (4), Techcyte (5), and Morphogo (6) have been developed to automate morphological identification, labeling, counting, and analysis of bone marrow smears and peripheral blood smears. The integration of multi-algorithmic DeepFlow (7–9) has streamlined multiparameter flow cytometry (FC), optimizing the process of immunological diagnosis. AI systems for automatic karyotype analysis in cytogenetics based on convolutional neural networks (CNNs), such as Varifocal-Net (10) and KaryoNet (11), have improved on issues such as the low resolution of karyotypic maps and the difficulty detecting cryptic chromosomal abnormalities (12–14).
Using DL algorithms and high-throughput sequencing technology, the potential heterogeneity of blood diseases can be mined in genomics, transcriptomics, and proteomics, particularly in the diagnosis of leukemia. In addition, the newly developed chromatin interaction neural network (ChINN) overcomes limitations in regional genomic testing (15). Numerous clinical applications have shown that AI systems are very effective for assisting medical diagnosis, with high productivity and accuracy comparable to experienced physicians. However, manual assessment by an expert is still essential for disease reclassification, validation, and interpretation of results.
ML has demonstrated promising results in the personalized and precise treatment of hematological diseases. It can use clinical data to build data analytics models for disease prediction, risk stratification, and the tailoring of optimal treatment plans based on each patient’s individual characteristics and condition (16–19). Furthermore, it can assist in mining new drug therapy targets or pathways (20), promoting the innovative development of the clinical treatment of hematological diseases.
However, there are challenges in applying AI technology, such as the lack of access standards for AI products, standardized data, medical–industrial collaboration, privacy and ethical issues, and the “black-box” nature of algorithms. Therefore, the regulation of AI products should be prioritized, along with addressing technical shortcomings and continuously strengthening education and training in the interdisciplinary field of AI and medicine. This is particularly important for clinical hematologists and undergraduate medical students.
During the past 5 years, the outbreak of COVID-19, the surge in the prevalence of hematological malignancies, and the globalization of the epidemic have caused significant harm to humanity. These challenges have also prompted consideration of AI-assisted diagnosis and treatment. There is a pressing need for reliable methods with high efficiency, high precision, and low cost to enhance the diagnostic and therapeutic environment for hematological diseases.
Here, we review the application of AI in hematology diagnosis and treatment over the past 5 years, with a particular focus on comprehensive typing diagnosis (including morphology, immunology, cytogenetics, and molecular biology) (Figure 1) to clarify the role and limitations of AI in areas such as disease prediction, drug development, risk stratification, and prognosis tracking. We speculate on how the integration of AI algorithms in the future will optimize the intelligence, standardization, and consistency of routine hematology diagnosis and treatment. The aim is to provide uniform and standardized treatments for patients with specific hematological disorders, reduce variation in patient outcomes due to differences in medical practice, and significantly improve the efficiency of diagnosis and treatment and the integration of medical resources.
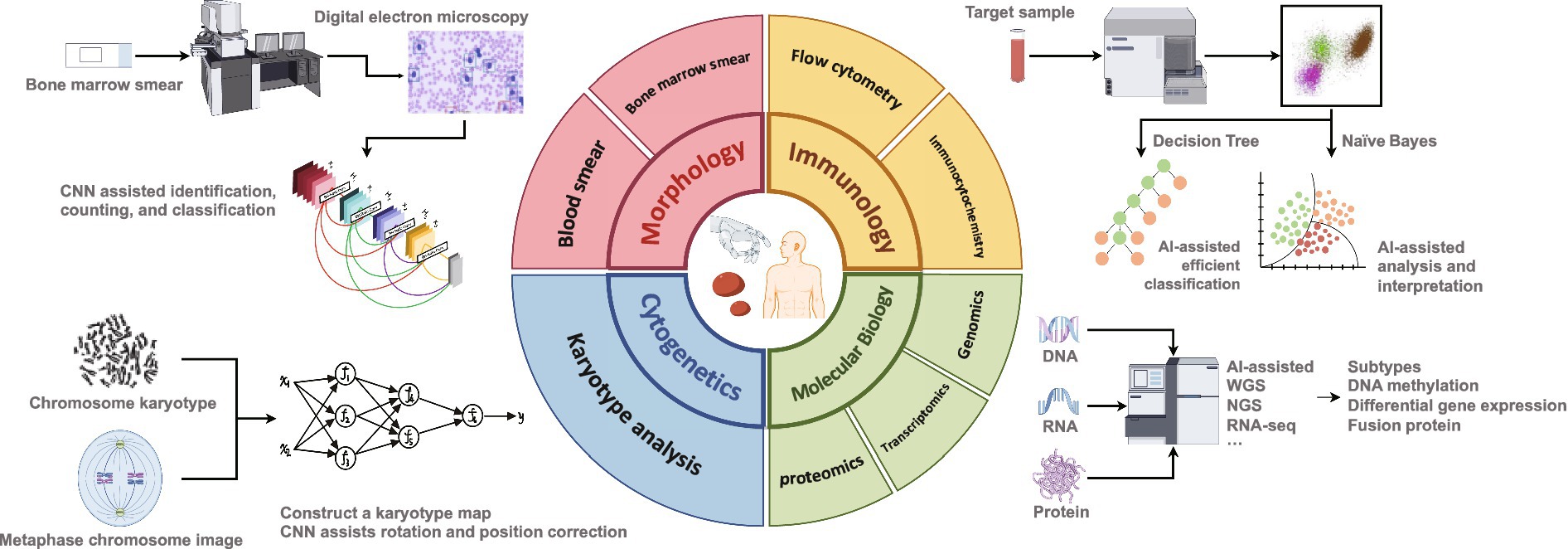
Figure 1. Assistance of artificial intelligence in comprehensive diagnosis of hematological diseases.
2 Application of AI in the diagnosis of hematological disease
2.1 AI-assisted morphological diagnosis of hematological disease
Hematological disorders are a complex group of diseases for which early diagnosis is crucial for effective clinical management. Cytomorphometric testing of bone marrow smears is one of the most common and effective diagnostic methods for blood diseases in clinic. Traditional manual analysis of smears begins with a hematologist selecting an area of interest with an appropriate distribution of cell trajectories and then performing a differential cell count on hundreds of cells to identify various cellular subsets and subtypes. This process is labor-intensive, cumbersome, and time-consuming (21). Because of the inherent complexity of bone marrow aspirate (BMA) samples, diagnosis largely depends on the hematopathologist’s experience, which makes the results highly subjective (22). In recent years, a promising technology has emerged to improve the accuracy and efficiency of morphological diagnosis: the combination of AI ML and digital morphological pathology analysis (Table 1). This approach aids diagnosis, with CNNs being particularly effective for the automated morphological detection and typing of BMA samples. CNNs are widely used for this purpose (23), and those enhanced with the CatBoost and XGBoost algorithms are particularly optimal (24).
The powerful role of CNN in automatic analysis of bone marrow smear data has been applied to a variety of blood diseases, such as aplastic anemia (AA), MDS, AML, etc. (25–28). The CNN-based you only look once (YOLO) model is a target-detection algorithm developed to rapidly and accurately detect and classify individual target cells in bone marrow smears (Figure 2). As early as 2020, this algorithm was used to evaluate individual cells in bone marrow smears of patients with MDS morphology (29). In order to improve accuracy, Wang et al. (30) developed a new YOLOX-s model. By combining MLFL-Net, a new architecture with multi-level features, the total accuracy was as high as 89.53%, and the diagnosis and prediction of acute leukemia also reached 92.5% of the cohort (compared with manual experts), which was better than all other relevant models. In 2024, a significant development in microscopy digital systems emerged with a single-trial detection architecture and the MobileNet V2 backbone. The most important innovation of this system is its ability to overcome the limitations of traditional AI-based BMA analysis systems, such as the inability to analyze and share data remotely and issues with low reproducibility. This new system facilitates human–computer interaction in any hospital through an intelligent BMA digital platform, eliminating the need for specific and complex medical electronic devices (31). This advancement strongly supports the feasibility of AI in enhancing the diagnosis of clinical hematological disease.
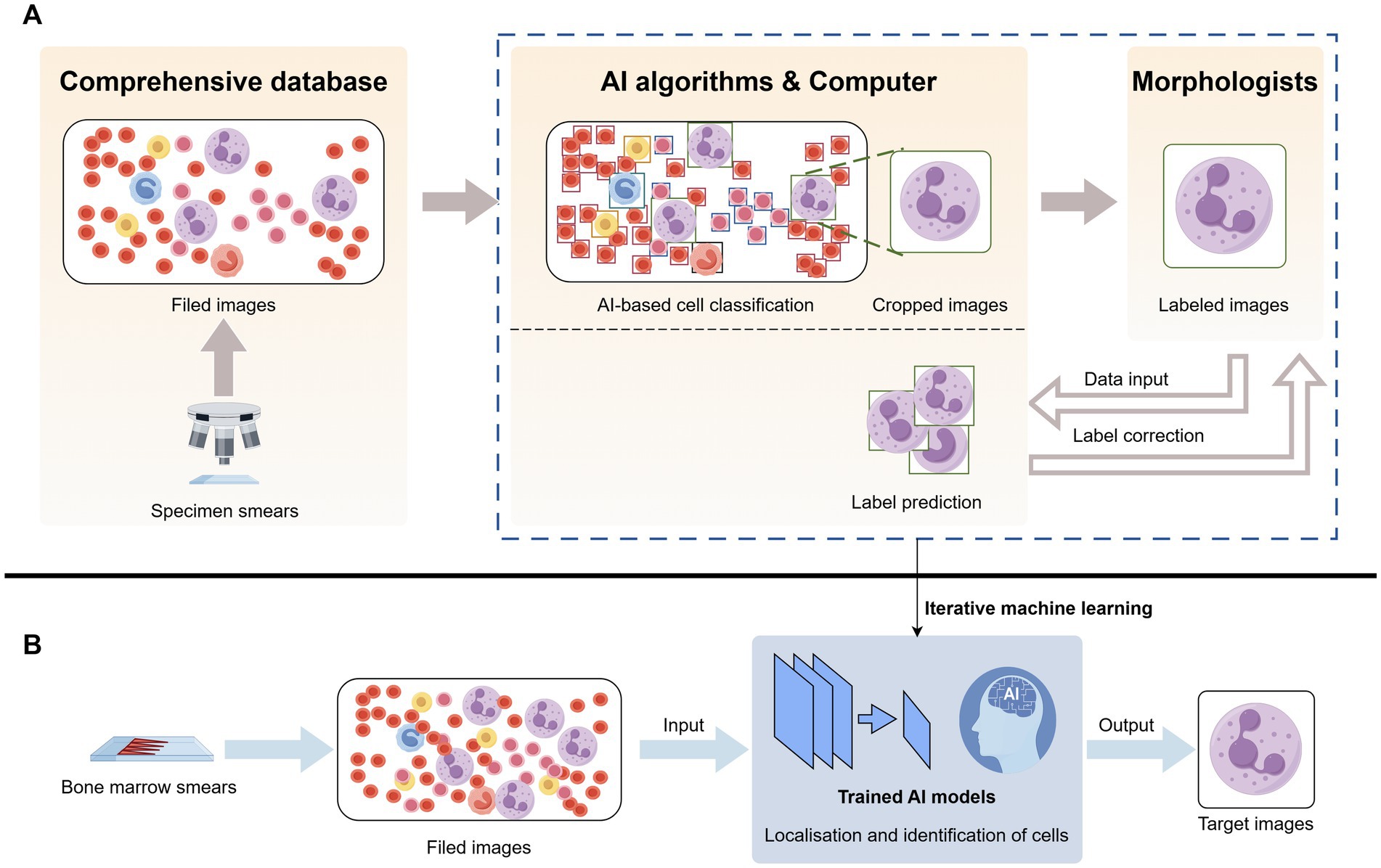
Figure 2. Artificial intelligence identifies neutrophils in bone marrow smears. (A) Bone marrow smears were obtained from a database and raw images were acquired, the raw images were located and identified by a computer AI algorithm to crop out the neutrophils and reviewed by a morphologist and corrected in several iterations. (B) After obtaining raw bone marrow smears, selecting images, and feeding them into an artificial intelligence model trained by iterative machine learning, the target cell neutrophils are output.
In the context of identifying leukemia subtypes, CNNs outperform other ML algorithms, including plain Bayes, SVMs, k-nearest neighbor, and decision tree algorithms (32). Newer algorithms, such as Cat-Swarm Optimization, further optimize CNN performance by combining them with CNN architectures. Current research favors either highly accurate single-algorithmic systems or systems that combine multiple algorithms for early detection of leukemia. For example, artificial neural networks, feed-forward neural networks, AlexNet + SVM, and ResNet-18 + SVM can all achieve 100% accuracy in leukemia diagnosis (33). These systems not only reveal morphological features but also predict the mutation status of genes in leukemia, such as the NPM1 mutation, common in AML (34). The high numerical accuracy of CNN model applications highlight the promise of more efficient and accurate hematological diagnoses in the clinical setting. This indicates a new direction for subsequent research to continue refining and optimizing CNN models for optimal clinical integration.
Morphological diagnosis in hematopathology also relies heavily on the analysis of peripheral blood smears. However, current digital cell imaging systems can only analyze limited areas of these smears and require manual intervention. With the continuous advancement of ML, more applications in hematological digital pathology can now extract and aggregate information from multiple data sources, including peripheral blood smears. These applications support diagnosis by simulating manual recognition workflows and thought modeling, thus enhancing the diagnostic process through improved accuracy and efficiency (35). Previous studies have developed advanced algorithms such as CellaVision and computer-assisted peripheral blood smear analysis for diagnosis. These technologies expand the hematopathologist’s capabilities, dramatically streamline workflow, and reduce turnaround time. By automating the analysis process and providing rapid, accurate results, these systems help enhance the efficiency and accuracy of hematological diagnoses (3). For example, the Scopio Labs X100 (Scopio Labs; Tel Aviv-Yafo, Israel) is an AI-based digital microscope imaging system that uses a full-field view approach to localize and classify blood cells in peripheral blood smears, marking a significant achievement by integrating a digital system with a microscope (4). Its digital nature allows for remote viewing by clinicians (36). Techcyte (Techcyte Inc., Orem, UT, United States) also supports remote viewing (5). These systems drastically reduce turnaround time for clinical work and significantly improve the efficacy of identifying malignant cells in leukemia over time (37). Compared to traditional morphological analysis, a fully automated ML pipeline can more rapidly provide an objective and accurate initial differential assessment of peripheral blood smears. This capability is particularly valuable for regions or institutions with limited healthcare resources and specialized hematopathology talent (38).
2.2 AI-assisted immunophenotypic diagnosis of hematological disease
In the clinical setting, FC and multiparameter FC (MFC) are essential auxiliary methods for examining the bone marrow of patients with hematological disease (39). These technologies are a class of high-throughput and high-sensitivity detection technologies that use a combination of different fluorescently labeled antibodies to detect the expression of antigens on the surface or intracellular of hematopoietic cells, and then analyze and determine the serial origin, differentiation degree, and abnormal phenotypic carnivore of cells. They have become an essential tool in the diagnosis and typing of blood diseases. However, traditional FC analysis relies on manual interpretation by the analyzer, which is time-consuming and prone to errors. The sensitivity and accuracy of these tests are subjective and depend significantly on the analyzer’s experience. Continuous exploration and development in recent years have led to computational analysis methods combined with AI, which has achieved promising results. These AI-enhanced methods have improved the accuracy and efficiency of the diagnostic process. In addition to identifying and classifying cells, DL-based AI models can reveal new biological associations between FC marker expression and cytogenetic and molecular variants in hematological disorders (40), providing clinicians with various differential diagnoses (39). Furthermore, they enhance the accuracy and standardization of clinical diagnoses through advanced visualization and automated gated analysis techniques (41).
However, the MFC data for most AI models are limited by specific panels and are often idiosyncratic. Therefore, DeepFlow, a clinically generalized FC panel based on the multidimensional density-phenotype coupling algorithm, has been developed in recent years (7–9). It dynamically adapts to changes in reagents and instrument settings, reducing the time required to accurately cluster and analyze cell lineages to less than 5 min (42). In addition, the accuracy of the results of leukemia classification and diagnosis using DeepFlow in combination with unsupervised learning algorithms, multidimensional clustering algorithms, and RF is almost identical to that of manual analysis (9). This leads to faster determinations and more accurate classifications of lymphocyte subpopulations, compensating for the deficiencies of traditional techniques and improving quality and standardization. The integration of AI and ML is expected to address healthcare disparities by enhancing the precision and consistency of immunological diagnostic processes, thus providing more equitable healthcare solutions (42). However, this does not mean that the role of humans will be completely replaced by AI. Instead, AI plays a crucial role in providing objective and standardized clinical advice to assist healthcare professionals.
Immunohistochemistry (IHC) represents a sophisticated immunological detection technique that aids in the diagnosis of hematological disorders. However, the rapid growth of IHC data makes manual interpretation of IHC data inefficient. In order to solve this problem, an artificial intelligence system based on Bayesian theorem probabilistic decision tree algorithm, ImmunoGenius, has been developed. It can be applied to mobile platforms such as iOS and Android platforms to diagnose and predict B-cell lymphoma and T-cell lymphoma, and provide clinical hematopathologists and hematologists with more convenient interpretation of IHC. However, due to the lack of specific IHC markers and overlapping IHC profiles in the clinical detection of AI systems, it is still necessary to combine clinical artificial and other diagnostic information such as histology (43–45) (Table 2).
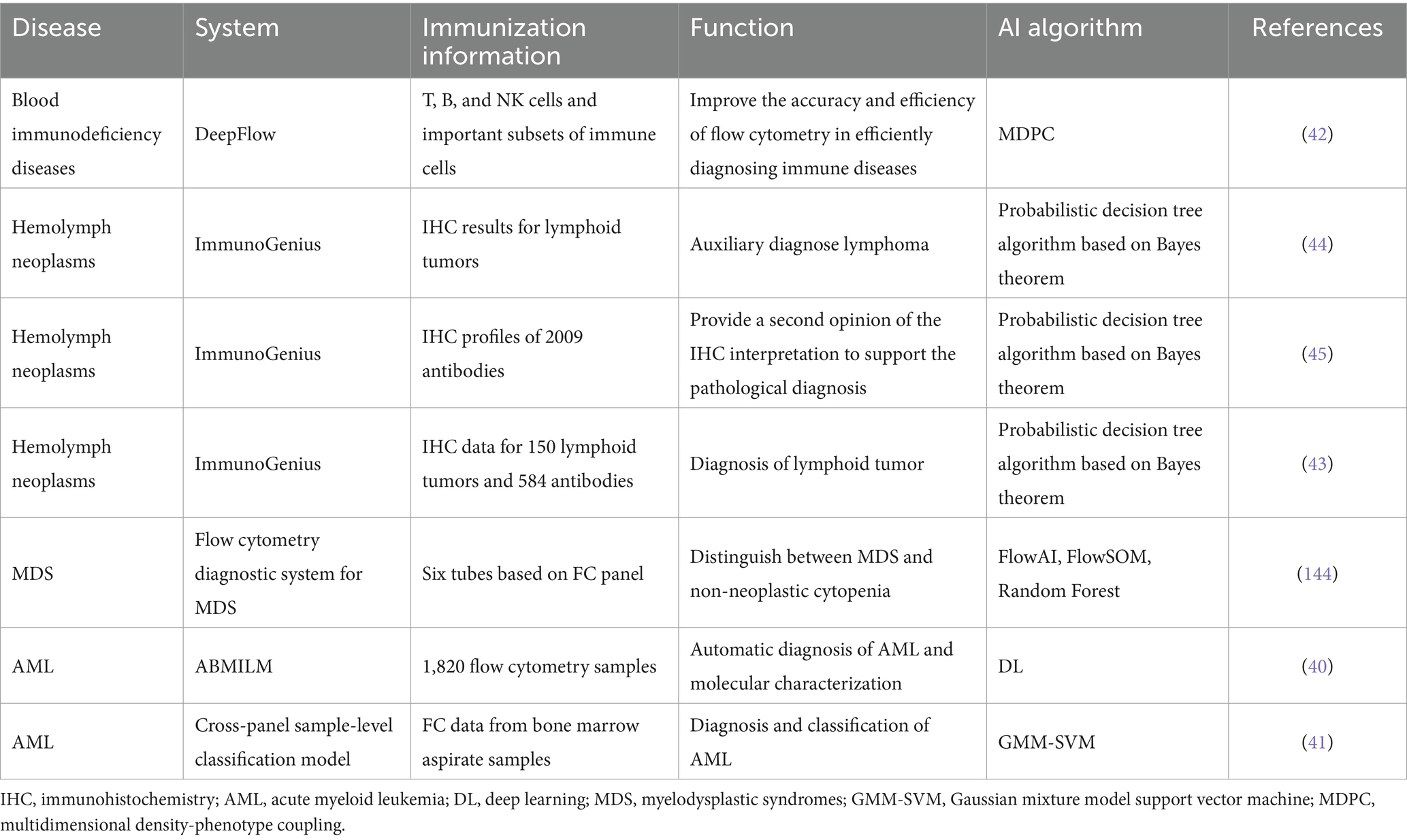
Table 2. Application of artificial intelligence in immunology in the diagnosis of hematological diseases.
2.3 AI-assisted cytogenetic karyotyping for diagnosis of blood disorders
Hematological diseases exhibit a wide range of cytogenetic abnormalities, with significant variability even among patients with the same condition. Hematological cancer cells often display multiple aberrations such as rearrangements, deletions, and duplications. Correct interpretation of these abnormalities is crucial for disease classification, prognostic determination, and therapeutic decision-making in patients with hematological malignancies.
Despite improved understanding of hematological malignancies due to increased genetic data availability, applying these large, complex, and time-consuming datasets in clinical practice remains challenging. Chromosomal karyotyping is one of the most important diagnostic tools in hematological cytogenetic laboratory tests. Typically, pathologists manually classify and construct karyotype maps of G-banded metaphase chromosomes based on each chromosome’s specific length and banding pattern. However, this is both costly and inefficient.
Over the years, ML methods have shown great potential for automating the analysis of large amounts of cytogenetic data (Table 3). To increase the rate of chromosome classification and karyotyping, various AI systems for automated mid-cycle capture, semi-automated, or interactive karyotyping have been introduced, leading to progressively wider clinical applications. These AI-driven systems enhance efficiency and accuracy, reducing the time and costs associated with traditional manual chromosomal karyotyping (46, 47). Using the CNN model to correct rotation and position in conventional karyotype analysis, the accuracy of identifying and classifying intermediate chromosomes of fluorescent R-bands could be improved to 98.8% (48). The accuracy of R-band and G-band chromosome classification can be improved by training with the characteristics of intra-karyotype interaction and category distribution through ML, which provides a new idea for accurate karyotype analysis of patients with different types of numerical abnormalities (11).
Notably, most chromosome classification and karyotype analysis systems have certain limitations, such as low-quality and poor-resolution karyotype maps. These issues can easily lead to missed or difficult-to-prepare reports on breakpoints within chromosomes. To improve the clinical analysis of low-quality karyotype maps, several AI models have been explored in recent years. They can convert low-quality karyotype maps into high-resolution images by specific algorithms, so as to accurately detect hidden chromosomal abnormalities (49) or obtain clearer chromosomal features, with an accuracy of up to 97.55% (12). In addition, type and polarity classification can speed up the process of generating karyotype maps, but chromosomes are prone to bending in microscopic images. This hinders cytogeneticists from analyzing chromosome types. Chromosome classification and straightening based on interleaving and multitasking networks can make chromosome band information easier to read (13). To address this, Li et al. (14) developed a shielded conditional variational autoencoder for chromosome straightening. Combining this with other karyotyping systems can greatly improve the chromosome classification performance of various DL models, reduce the burden on clinicians, and save time and materials.
2.4 AI-assisted diagnosis of hematological disease in molecular biology
Molecular biology tests are another critical aspect of the comprehensive clinical diagnosis of hematological disease. Molecular testing of blood, including polymerase chain reaction, DNA sequencing, transgenics, and gene chip (DNA chip) technology, can predict and diagnose disease progression by testing various genes and their mutations in leukemia cells, particularly those that may lead to disease onset or regression.
With the introduction of high-throughput sequencing technologies such as multi-gene sequencing, whole-genome sequencing, whole-exome sequencing, and transcriptome sequencing, molecular biology has entered the realm of big data. These advanced sequencing technologies are now approved for clinical purposes and greatly facilitate the diagnosis of hematological disorders, ushering in the era of “precision diagnosis” for these diseases (Table 4).
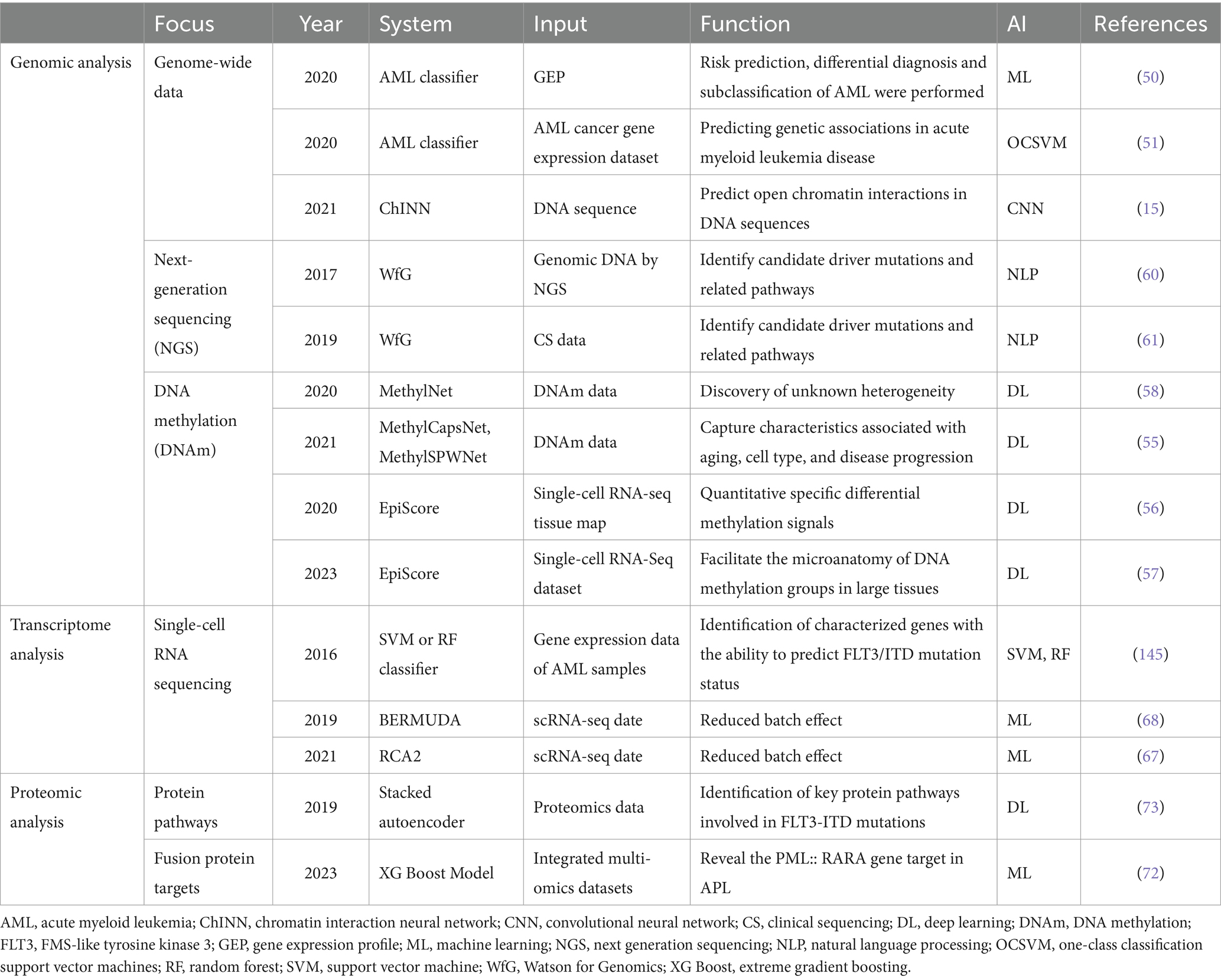
Table 4. Application of artificial intelligence in molecular biology diagnosis of hematological diseases.
2.4.1 Genomic analysis
Due to the sheer volume of data analyzed, annotation of clinically actionable genomic regions and their biological functions with prioritization of genetic variants is necessary for clinical decision-making and is the basis for automated disease classification, a process known as functional genomics. More research is being conducted to sequence a set of gene regions associated with a suspected disease, which not only reduces costs but also increases efficiency.
AML is a serious hematopoietic malignancy, and prediction of its status by whole-genome sequencing is essential for clinical diagnosis and treatment. Using deep neural networks of high-dimensional machine learning, combined with genomic analysis, it is now possible to subtype, differential diagnose, and predict risk for AML, while also obtaining meaningful predictive genetic signatures. The accuracy of this AML classifier is directly proportional to the number of samples, so the establishment of reference blood gene expression profiling data sets of a large number of samples is helpful to the obtained high-precision classifier, which can effectively solve the problem of lack of professionals in some regions or medical environments and greatly reduce the cost (50). Like most models, systems for predicting AML-associated genes are typically binary classification models that distinguish between known causative genes and unknown genes. A more accurate AML classifier focusing on known genes has been developed based on a one-class SVM (51). The reduced potential error rate compared to binary classification models demonstrates that gene expression profiling can provide more efficient, objective, robust, and prospective clinical guidance with the assistance of AI.
The importance of DNA methylation (DNAm) in cancer development and progression has been revealed in recent years (52). DNAm involves the addition of methyl groups to nucleotides without a change in the DNA sequence, and it most often occurs on cytosine–guanine dinucleotides. Hypermethylation of suppressor genes and hypomethylation of oncogenes in hematological malignancies such as MDS and acute lymphoblastic leukemia (53, 54) are part of the pathogenesis of these diseases and can lead to a poor prognosis. Traditional analysis of heterogeneity changes associated with phenotypes involves multiple-hypothesis testing and managing multiple covariates, which presents a significant challenge for genomic data analysis. However, with the development and integration of various AI algorithms and applications, DL has shown great potential and promising results for discovering and analyzing DNAm on actionable genomic targets. This advancement correlates with improved diagnosis of blood diseases (55). Teschendorff et al. (56) used DL algorithms to develop EPISCORE, a single-cell histology reference model that reduces the cost of inferring DNAm differences in patients with acute lymphoblastic leukemia by analyzing DNAm cell heterogeneity using high-resolution datasets (57). Another DL model, MethylNet (58), can uncover unknown DNAm through a combination of unsupervised generation, clustering, cell type deconvolution, subtype classification, age regression, and smoking status classification. It is also user-friendly.
With the increasing availability of genetic data, next-generation sequencing (NGS) has become a prerequisite for accurate diagnosis and proper treatment in clinical hematology/oncology (59). Watson for Genomics (WfG), a semi-automated pipeline AI system based on in-house NLP algorithms, has been developed for applications in clinical sequencing and precision medicine for patients with hematological malignancies. Tojo (60) used malignant and normal tissues from more than 150 patients as inputs and combined them with WfG to analyze the sequence data, identify mutations and associated pathways, and infer applicable drug information. Yokoyama (61) demonstrated the role of AI in the manual interpretation process of clinical sequencing using clinical sequencing data from more than 300 patients with hematological cancers as inputs.
The gold standard for hematology diagnosis has been challenged by the introduction of whole-genome sequencing and NGS combined with AI (62). AI shows the potential to outperform experienced hematologists in comprehensive analysis of high-dimensional whole-genome sequencing and NGS data, effectively narrowing interobserver variability and improving diagnostic timeliness and accuracy. In addition, precise and comprehensive AI-assisted genomic analysis is expected to be used more often to aid clinical decision-making. Because of the limited availability of genome-wide data, predicting chromatin interactions between open chromatin regions using only limited DNA sequence data is a major challenge. However, the ChINN provides a potential solution by identifying open chromatin interactions in a genome-wide manner. This was verified in samples from patients with CLL, exhibiting extensive heterogeneity in chromatin interactions of prognostic genes and thus suggesting differential expression of oncogenes (15).
2.4.2 Transcriptomic analysis
RNA sequencing (RNA-seq) is an important method of transcriptomic analysis, which can reveal specific biological processes and the molecular mechanisms involved in the development of hematological disorders at a holistic level. RNA-seq is most commonly used to analyze differentially expressed genes, which is a challenge in the diagnosis of hematological neoplasms due to their heterogeneity. With the construction of dynamic RNA databases (63), the development of single-cell RNA-seq technology has significantly advanced. It is now possible to detect the underlying mechanisms of blood tumor heterogeneity and the biological behaviors of tumors to identify new potential targets in the clinical setting (64–66), providing deeper clinical insights. However, with the increasing amount of single-cell transcriptome data, there is a growing tendency to analyze data jointly, which can easily generate batch effects. RNA-seq, as a high-throughput sequencing method, is particularly sensitive to batch effects because of its high detection accuracy. Therefore, Schmidt et al. (67) established a model called RCA2, which helps reduce the batch effect while unifying single-cell data. The DL-based Batch Effect Removal using Deep Autoencoder (BERMUDA) can distinguish between different batches of small conditional RNA-seq data and different cell populations while removing the batch effect (68). This suggests that ML and DL of AI algorithms can optimize the transcriptomic analysis process and provide more objective and precise data for clinical transcriptional data analysis.
2.4.3 Proteomics analysis
The mining of protein variants from multivariate data is also an important part of the diagnosis of blood disorders. Increasing numbers of studies are exploring the clinical applications of proteomics technologies for monitoring treatment responses, disease progression, and microscopic residual foci, with initial results showing promise (69). Typically, proteomics studies involve thousands of observations and thus require preprocessing of data (70). ML techniques have been applied in proteomics analysis because of their powerful ability to categorize unknown samples (71). Among these techniques, SVMs have proven particularly effective for proteomics classification. In 2023, using a multi-omics dataset and an ML algorithm, a new fusion protein target called PML::RARA was reported. This target directs the mechanism of different features of the transcriptional response, confirming that disruption of the coagulation cascade response due to high expression of this gene is associated with acute promyelocytic leukemia coagulation dysfunction and hemorrhage, presenting significant clinical challenges (72). In addition, ML can aid the extraction of hierarchical features to identify key protein pathways in FLT3 internal tandem duplication mutations in patients with AML (73). Overall, with the increasing popularity of proteomics and the generation of large amounts of proteomics data, the future challenge lies in utilizing AI to process these big data effectively. The goal is to discover and analyze new actionable targets or pathways to facilitate the diagnosis and treatment of blood disorders.
3 Application of AI in precision therapy for hematological disease
3.1 Biomarker recognition
During the last decade, a large amount of molecular biology information has been mined, and ML algorithms have enabled the identification of biomarkers in the body fluids of patients with hematological disorders. These biomarkers can be used for early diagnosis, disease typing, and prognostic assessment, greatly broadening the horizons of hematological diagnosis. This advancement has deepened the understanding of the complex interactions among different molecular subgroups in hematological disorders, helping clinicians make precise estimations of interindividual variability and providing a reliable basis for subsequent targeted therapeutic regimens. This approach helps clinicians accurately estimate variability among individual patients and provides a solid foundation for developing targeted therapeutic programs (74). In addition, methods that incorporate ML create correlations between different layers of information, are user-friendly across various platforms, and enable the sharing of resources.
A few studies have relied on ML to discover or utilize new biomarkers for blood disorders. For example, Venezian Povoa et al. (75) used clinical and molecular data to predict treatment sensitivity in patients with MM and found that using gene expression values as markers improved the accuracy of predicting treatment sensitivity. In addition, combining high-throughput histology data with clinical data demonstrated higher clinical utility. Ghobadi et al. (76) characterized a variety of mRNAs and miRNAs in asymptomatic carriers of adult T-cell leukemia/lymphoma, identifying reliable miRNA–mRNA interactions for each subtype. These interactions serve as potential targets for subsequent therapy and as biomarkers for prognosis. Guerrero et al. (77) integrated cytogenetics [t(4; 14) and/or del (17p13)], tumor load (clonal size of bone marrow plasma cells and circulating tumor cells), and immune-related biomarkers to develop efficient, integrated weighted models for predicting undetectable measurable residual disease in MM episodes. Immune biomarkers showed the highest weight in these models, suggesting their importance in predicting the measurable residual disease status.
The potential of ML to aid the discovery of new biomarkers provides an opportunity to develop new AI systems that alleviate the pressure on clinicians to deal with massive amounts of data. At the same time, prognostic indicators based on ML-derived patient characteristics provide a more objective assessment of potential risk, facilitating the creation of clinically targeted therapies for new biomarkers and enabling personalized treatment for patients (74).
3.2 Drug development
Drug discovery and development is a long and costly process. During the past decade, ML, DL, and NLP have emerged as breakthrough technologies, accelerating the process because of their automated nature, predictive capabilities, and expected efficiency gains (78).
The persistence of leukemia stem cells has been shown to lead to acute leukemia treatment resistance. Li et al. (79) combined multiple ML algorithms to identify signatures specific to the gene expression of leukemia stem cells. Zhang et al. (80) found that genes associated with leukemia stem cells, particularly RFC4 and RFC5, exhibit strong cluster interactions and may serve as inhibitory therapeutic targets for AML. ML can analyze large amounts of high-quality molecular biology data to identify targets of drug resistance during treatment. By discovering drug–target interactions, it can predict therapeutic efficacy, aid the design of new drug molecular structures, optimize drug efficacy, and help minimize side effects. CNNs in particular excel in image analysis, aiding biomarker identification and optimizing drug formulations. An example of this is AlphaFold, a CNN-based protein structure prediction and drug formulation system developed by DeepMind (Google). This neural network-based system excels in protein structure prediction and drug repurposing, significantly advancing the field of drug discovery and development (81).
Analyzing high-dimensional data to predict interactions between ligands and proteins is a difficult task in the drug-discovery process. Janssen et al. (20) developed a fully open-source drug-discovery mapping model that predicts the activity of novel kinase inhibitors in the kinome, such as the new inhibitor of FLT3. Continued progression and relapse of CLL after treatment remains problematic in patients with CLL that have been treated with new targeted agents (ibrutinib and venetoclax). Given the important role of microenvironment interactions in the progression and relapse of CLL, Gimenez et al. (82) built a drug-discovery platform to elucidate the critical role of simvastatin in targeting the microenvironment and its synergistic enhancement with ibrutinib or venetoclax for the treatment of CLL. These research endeavors suggest that ML algorithms can be used to build AI platforms that aid the development of new medicines or new combination therapies for blood disorders.
Furthermore, in addition to R&D to predict drug–target interactions and design new drugs, understanding the side effects of drugs and optimizing drug efficacy are aspects that cannot be ignored. Eltrombopag, a thrombopoietin receptor agonist for the treatment of primary immune thrombocytopenia, has side effects that affect systemic immunomodulatory responses. Therefore, Lozano et al. (83) developed the Therapeutic Performance Mapping System using AI pattern recognition to reveal the association between the target markers of eltrombopag (BCL1L2, BCL2, and BAX) and the key proteins involved in immune thrombocytopenia. This system has advanced our understanding of the drug’s mechanisms of action and side effects, providing valuable insights for drug optimization research and pointing to future directions for improving therapeutic efficacy and safety.
In summary, the incorporation of AI optimizes all aspects of drug development. It integrates with genomics, proteomics, and metabolomics to detect and dynamically monitor the effects of drugs, whether alone or in combination with other drugs. This greatly improves safety and efficacy outcomes and is expected to enhance patient prognosis and drive drug innovation. However, the use of AI technologies also faces a series of ethical and moral issues, such as data privacy and security, algorithmic bias and interpretability, and obtaining informed consent. Therefore, human oversight of intelligent algorithms is essential, and relevant laws and regulations need to be established or improved to address these challenges (84).
3.3 Disease prediction and risk stratification
Failure to treat hematological diseases in a timely manner is likely to be life-threatening. Malignant hematological diseases such as AML, MDS, and myeloproliferative neoplasms progress relatively rapidly and generally have a poor prognosis. However, previous studies have demonstrated that specific changes in these disorders can be detected prior to diagnosis (85). By analyzing a patient’s genomic data and lifestyle factors, AI can quickly and accurately predict the risk of developing a particular blood disorder, helping physicians to intervene early. Mahmood et al. (86) reported that using multiple supervised ML algorithms, it is possible to predict a significant risk of pediatric acute lymphoblastic leukemia based on clinical variables, phenotypic data, and environmental factors to inform treatment and prognosis. Nazha et al. (87) also demonstrated that stochastic survival algorithms combined with multidimensional data can facilitate dynamic risk stratification and personalized prediction of overall survival in patients with MDS. This ability of ML algorithms to quantify the risk of various myeloid tumor subtypes and make dynamic predictions can certainly provide more convenient one-stop prediction tools for clinical use (85).
A variety of ML algorithms can be employed to develop predictive models to optimize the rational allocation of clinical treatments, such as SVMs, artificial neural networks, RFs, decision trees, logistic regression, and k-nearest neighbors (88). However, most of these algorithms have limitations that are difficult to explain to clinicians. Consequently, recent studies have increased the use of explainable AI (XAI) methods such as Local Interpretable Model-agnostic Explanations (LIME) and Shapley Additive Explanations (SHAP), which are designed to help clinicians to more intuitively understand the impact of risk factors (89, 90). For immunocompromised patients with hematological malignancies, the SARS-CoV-2 mRNA vaccine is not effective and may increase the risk of severe breakthrough infections. According to Rodríguez-Belenguer et al. (91), ML algorithms (particularly SVM, which shows the best performance) and XAI can efficiently select patient-specific features to enhance predictions and improve healthcare strategies, which makes the widespread use of AI systems in the clinical setting more promising.
3.4 Individualized treatment and prognosis
With the continuous accumulation and expansion of systems biology data, including molecular biology, genomics, proteomics, metabolomics, and bioinformatics, along with precise estimation of interindividual variability and ongoing efforts to establish correlations between different layers of information, improved targeted therapeutic regimens are increasingly emerging in clinical practice. It is evident that the demand for both precision medicine and personalized medicine is gradually increasing among both doctors and patients. AI holds significant promise in promoting personalized medicine by enhancing the accuracy and efficacy of these tailored therapeutic approaches (92). Previous studies have demonstrated that, in conjunction with systems biology data, AI-automated networks can generate dynamic data clouds that are unique to each patient. These data clouds help physicians understand disease mechanisms, identify relevant blood biomarkers, and define druggable molecular targets. Then this information can be used to develop individualized treatment regimens, including the selection of the most effective drug and determination of the optimal dosage (93).
AI-assisted individualized treatment and prognostic prediction models are widely used in patients with leukemia. Previous studies have shown that AI models based on ML algorithms can identify key prognostic molecular markers for AML using high-throughput sequencing expression profiling data (94). These models can also predict complete remission or event-free survival, calculate prognostic indices, and stratify survival for individualized prognostic prediction (95, 96). For pediatric patients with acute lymphoblastic leukemia receiving cranial radiotherapy, ML algorithms can also predict treatment efficiency by stacking integrated classifiers, helping physicians to track the patients’ status (97). Notably, most current predictive models are trained with multi-source data from a single patient, which facilitates more accurate and personalized treatment for that particular patient. However, developers should note that in-depth gene sequencing is rare in routine clinical diagnosis. If an ML model is trained on genomic data alone and lacks clinical variables, the stability and generalizability of the resulting AI model will be greatly reduced (95).
Despite the gradual application of targeted therapies with innovative treatments for leukemia, conventional treatment is still usually performed using allogeneic hematopoietic stem cell transplantation (allo-HSCT) (98). Such treatment carries risks of high therapeutic toxicity, infectious complications, graft-versus-host disease (GVHD), graft failure, and relapse. ML has been used to predict risk factors for death in patients treated with allo-HSCT (99), with alternate decision trees showing great potential (100, 101). This allows the analysis of multiple factors simultaneously and ensures the generalization and high accuracy of the results. In addition, the output of alternate decision trees has the advantage of visualization, which lays the foundation for extended clinical use (100). Among the many prognostic risks of allo-HSCT, GVHD is the top priority. Recent studies have utilized decision tree tools to stratify post-transplant survival and have successfully identified seven unique phenotypes of patients with chronic GVHD (101).
Moreover, several new prognostic scoring systems have been proposed for the treatment of myelofibrosis over the last 2 years, aiming to improve the identification of patients with the poorest prognosis following treatment with ruxolitinib and to increase the accuracy of patient stratification. Notably, two systems, the Artificial Intelligence Prognostic Scoring System for Myelofibrosis (AIPSS-MF) and Response to Ruxolitinib After 6 Months (RR6), have been developed (102, 103). Both systems outperform traditional models in stratifying patients, enabling early identification of patients with myelofibrosis who have impaired survival after ruxolitinib treatment. This advancement provides a foundation for personalized AI-based prognostic models.
New candidate biomarkers for disease progression, treatment response, and chemotherapy resistance in MM have been identified by mass spectrometry-based proteomics (69). The proteasome influences tumor development through tumor signaling pathways, immunomodulation, and drug resistance (104), with the ubiquitin proteasome pathway playing a crucial role in the development of MM. Ren et al. (105) constructed a Ubiquitin Proteasome Pathway Risk Score (UPPRS) system that combines nine genes related to the ubiquitin proteasome pathway. This system stratifies MM patients and utilizes the International Staging System to efficiently predict overall survival. In addition, a prognostic column chart was produced to uncover mechanisms of resistance to proteasome inhibitors. This can be used to identify new targets for inhibiting the ubiquitin proteasome pathway, aiming to reduce the recurrence rate and increase the cure rate of MM (105). Furthermore, an ML-based decision support system can identify proteomic profiles to provide sensitive/resistant chemotherapeutic agents for patients with MM, offering a more precise approach for personalized treatment (106).
XGBoost models, based on an ML gradient boosting framework, can more accurately represent nonlinear relationships between multiple features and outcomes than traditional linear models. This advantage was recently validated in the comprehensive prognosis of mantle cell lymphoma (107). Despite the success of these AI models in individualized treatment and prognosis of hematological disorders, they still have limitations. These include a lack of external validation cohorts and generalization issues, which need to be addressed in further research. To improve these models, future studies should focus on creating more standardized training samples and integrating multiple features from multi-omics and clinical measures. This integration will enhance the accuracy of prognostic predictions (Table 5).
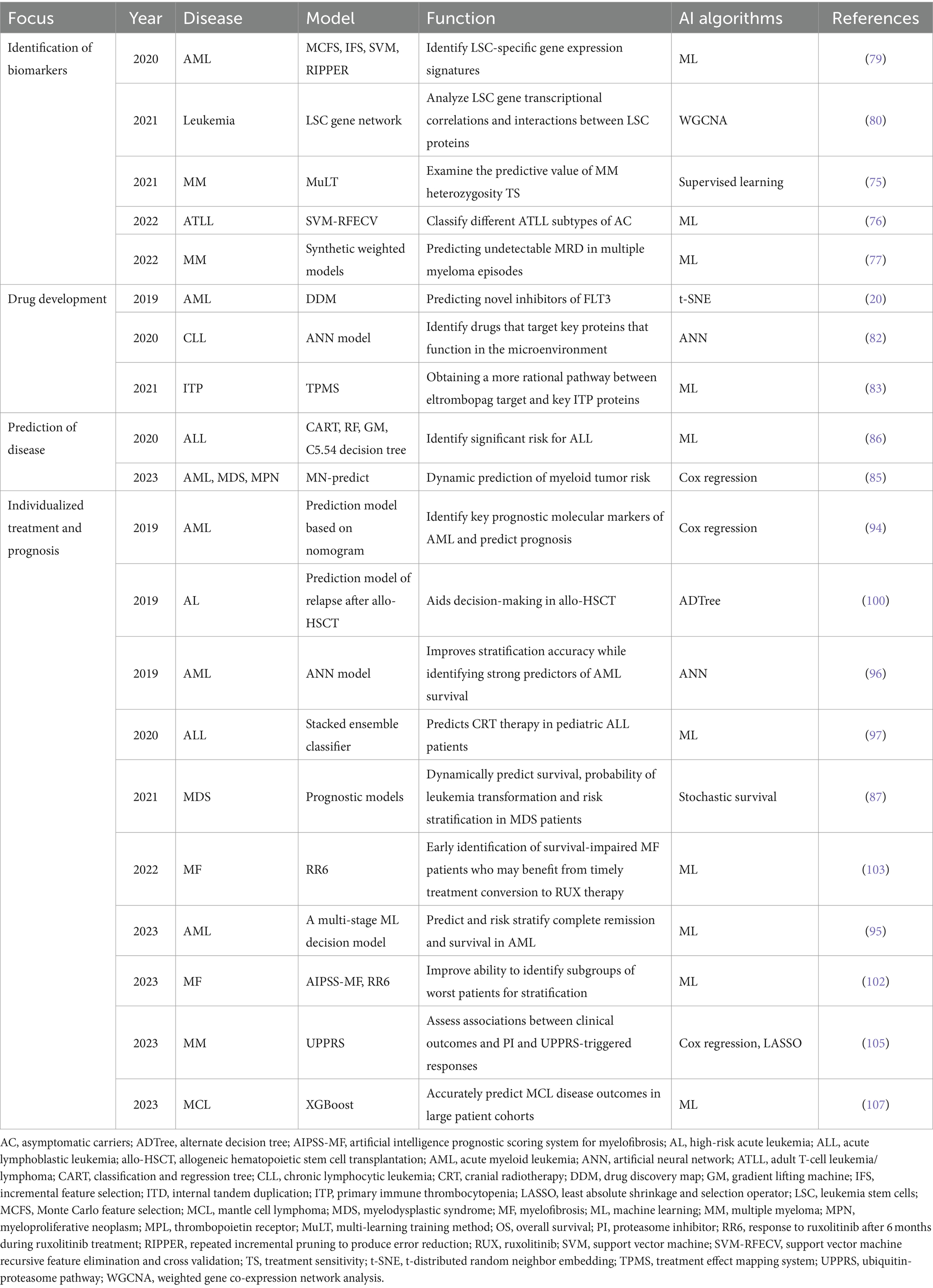
Table 5. Application of artificial intelligence in the precision treatment of hematological diseases.
4 Difficulties and limitations of AI-assisted applications
Optimizing standardized clinical care for hematological diseases is a crucial and challenging area of research. Standardized care in hematology involves providing uniform treatments for patients based on clinical practice guidelines and best practices to achieve optimal outcomes and consistent patient care regimens, reducing variability due to differences in medical practice. The development of standardized treatments is typically based on extensive clinical research and experience to determine the most effective and safest treatments for specific situations. These guidelines and specifications are often developed by professional medical organizations, academic institutions, or government agencies.
In the past 5 years, the rapid advancement of emerging technologies such as AI, ML, and DL has led to the development of a series of AI applications based on generalized comprehensive diagnosis and treatment. These applications have been widely used throughout the entire process of managing hematology patients, from prediction and diagnosis to treatment and prognosis. They range from analyzing peripheral blood smears and bone marrow smears to identifying genome heterogeneity and molecular biomarkers.
The integration of AI and human expertise is effectively advancing the intelligence, standardization, and normalization of hematology diagnosis and treatment. AI algorithms help humans analyze and interpret vast clinical data, improving the efficiency, objectivity, and accuracy of diagnosis and guiding clinical decision-making, particularly for inexperienced doctors. In addition, they can integrate data from multiple sources and uncover potential new clinical discoveries, thereby extending human capabilities and deepening knowledge and understanding of hematological disorders. This integration opens new directions and pathways for future research and improvement of treatment prognoses. In essence, AI enhances our understanding of blood diseases and offers new opportunities for improving therapeutic outcomes.
However, there are many difficulties and shortcomings in the process of using AI to these ends (Figure 3). First, any AI product needs to obtain a medical device registration certificate before it can be used clinically, which means there needs to be a standardized AI product that has been comprehensively validated. This is a prerequisite for integrating AI models into clinical practice. In terms of morphological recognition, many digital image analyzers using AI algorithms have been developed to automate blood smear examination and pre-classify cells. However, these come from different manufacturers and exhibit variation in staining methods, optical magnification, color, and display characteristics, hardware, software, and file formats. This lack of standardization presents significant challenges for consistent and reliable clinical application (108). The lack of standardization of parameters throughout the analysis process could also somewhat limit the extended use of this technology. In addition, because AI undergoes continuous ML to improve its performance, the integration and training of new data during the ML process is dynamic and flexible. This means that the system’s heterogeneity is susceptible to analytical confounders that do not align with highly stringent clinical trial standards and regulations. These confounders include batch effects, interinstitutional differences in sample collection, and pre-analytical processing protocols, which lead to difficulties in comparing and evaluating new algorithms (109). Therefore, the incorporation of AI technology does not mean the complete liberation or exclusion of humans. Instead, it emphasizes the need for human-supervised use to assist in clinical diagnosis and treatment. It also highlights the importance of establishing comprehensive guidelines and standard evaluation criteria for AI tools.
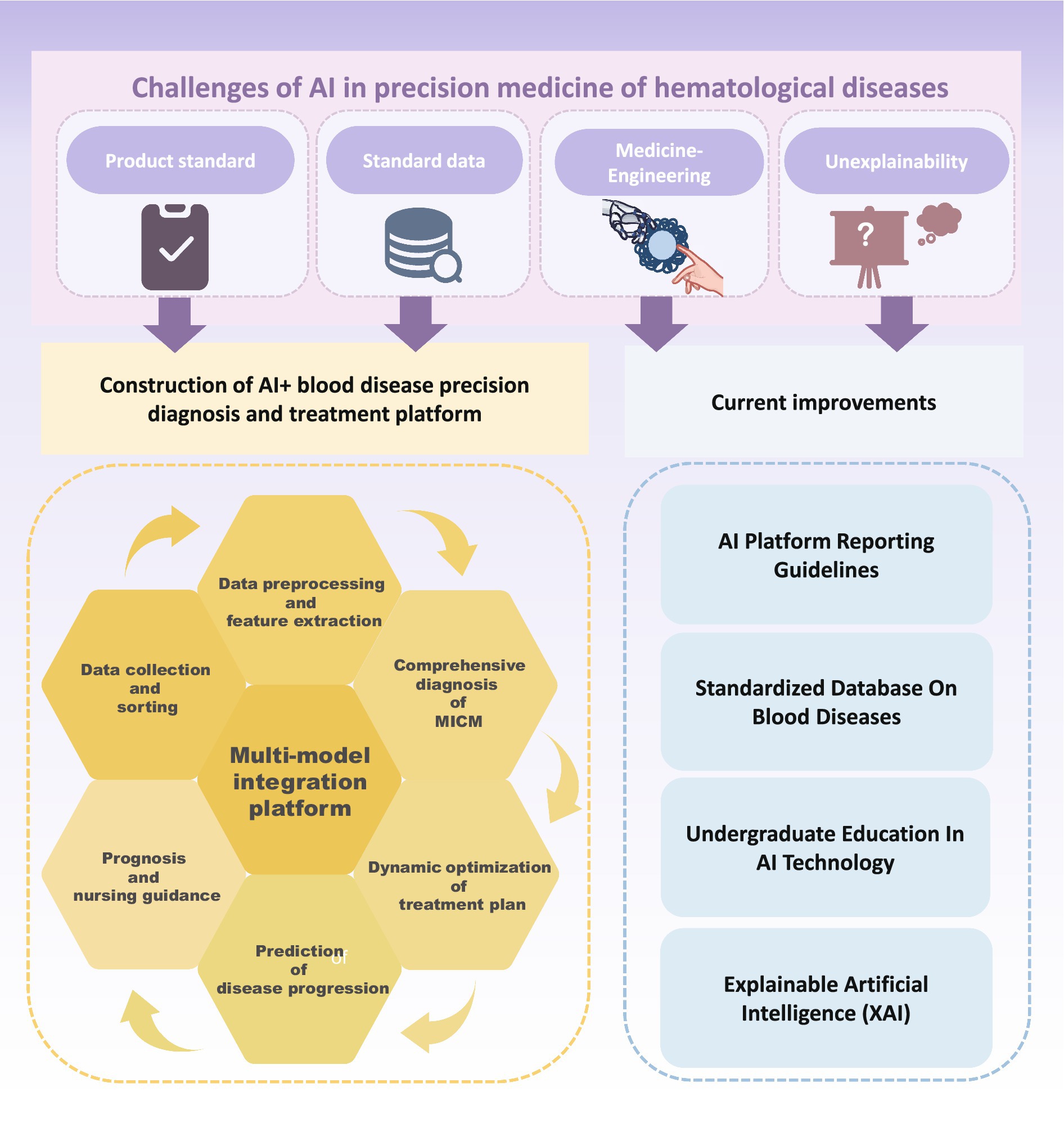
Figure 3. Challenges and future improvement of artificial intelligence in precision medicine of hematological diseases.
The second issue is the lack of standardized data and poor reproducibility. Data form the foundation of AI applications, and the large global population base provides an opportunity to establish extensive databases. It is widely acknowledged and confirmed that increasing the sample size greatly benefits algorithm performance enhancement by improving AI model accuracy. However, available training data for blood diseases is highly complex and limited, which makes it time-consuming to collect a large number of samples. During this process, changes in equipment, procedures, and personnel may occur, potentially leading to incomplete capture of morphological, genetic, or molecular biological variation, as well as susceptibility to issues such as overfitting and accidental fitting of confounding factors. In actual research, different laboratories and teams utilize various AI detection platforms with differences in model workflow, detection reagents, and selection parameters, which are constrained by infrastructure limitations. This results in uneven quality of similar data, which significantly reduces homogeneity (110). In addition, the relative independence of medical institutions makes it difficult to intercommunicate and share data, compromising the transferability of AI models across different clinical institutions. In the field of diagnosis and treatment of hematological diseases, systematic, and comprehensive high-dimensional data for ML are needed to provide accurate and robust prediction results to guide treatment strategies (95). Particularly when using ML for analyses involving sensitive and confidential patient data, a lack of standardization is likely to raise ethical issues regarding data privacy and security (111). Therefore, it is necessary to screen and construct effective datasets while complying with relevant regulations such as the EU General Data Protection Regulation. Strengthening the integration of laboratory information systems and electronic health record systems with ML, building a mature data-sharing platform, and establishing a comprehensive data standard system are crucial to ensure data security and maximum sharing. Using ML on this basis is more likely to promote data-driven implementation into all aspects of clinical care, such as accelerating the process of drug discovery and development and reducing failure rates for hematological diseases (81).
Another key difficulty is the lack of intersection between medicine and engineering. Given the complexity of ML, which requires continuous refinement of AI, clinicians and researchers often encounter several pitfalls when designing and developing new models (112). When constructing a model, the data are usually decomposed into a training set and a test set. In experiments, researchers often overestimate the actual effect of the model because of improper data splitting. Typically, only the target variables are changed during the experiment; however, the ML algorithm is highly sensitive to hidden variables, leading to confounding factors. It is necessary to use multiple ML models to detect these hidden variables. Data output from an AI model may be biased, and model developers may misunderstand the goal. Therefore, the rich experience of professionals and standardized diagnosis and treatment processes are crucial for improving the applicability of ML in the diagnosis and treatment of hematological diseases. However, the number of experienced and professional hematologists is currently limited, and few clinicians have knowledge of AI technology or are skilled in its development, research, and use. Cultivating such composite high-end talents requires a significant amount of time and economic cost. This limitation hampers the increasing effectiveness, precision, and clinical adoption of AI products.
The “black box” is an objective shortcoming of AI technology. AI relies on continuous ML to improve its performance. ML includes three components: algorithms, training data, and models. An algorithm is a set of procedures that, in ML, learns to recognize patterns after being trained on large amounts of data. Once an ML algorithm is trained, the result is an ML model, which can be used by people. DL models usually contain a large number of parameters and complex network structures, enabling them to handle high-dimensional data and learn complex feature representations. However, the complexity of the model also makes its inner workings unexplainable. Typically, any one of these three components can be hidden or figuratively described as being placed in a black box. To protect intellectual property and achieve higher accuracy and generalization, AI developers often obscure the model or the data used to train the model. This means that the training data are placed in a black box, which makes it impossible for non-AI professionals, such as clinicians, to directly understand how the model extrapolates decisions during the decision-making process. This difficulty ensuring the credibility and safety of the results has, to some extent, hindered the full integration of AI ML technology and clinical practice. In view of this, research in recent years has focused on the development of new XAI technologies (113–116), such as LIME and SHAP (89, 90). XAI can capture the results and outputs of ML/DL algorithms and provide model decision-making and interpretation to overcome the limitations of the black box nature of AI. XAI has shown great promise in diagnosis and prediction of drug discovery and development (116–118). However, XAI technology is still in the exploration and development stages and has not been effectively and comprehensively standardized.
Overall, AI and ML have demonstrated promising results in laboratory research and hold potential for widespread application in clinical auxiliary diagnosis and treatment. However, the lack of standardized product standards, uniform data, and high-level expertise hinders the transferability of AI and ML, leading to complexity and challenges in interpreting algorithms and models. The main obstacles that need to be addressed include establishing reasonable and legal clinical decision-making guidelines and enhancing the integration of AI into clinical practice.
5 Current research hotspots and suggestions
Due to the deepening reform and development of “AI + medical,” the field of blood disease diagnosis and treatment is undergoing major changes. Clinical laboratories are transforming the traditional purely manual comprehensive diagnosis and treatment model by integrating clinical decision support systems with AI, ML, and DL algorithms (119). This entails the integration of morphology, immunology, genetics, and molecular biology with advanced high-precision technology. Through data collection, sorting, preprocessing, feature extraction, model establishment, validation, evaluation, and treatment optimization, AI has significant applications across all stages of blood disease healthcare. These stages include early diagnosis, personalized treatment plans, disease progression prediction, real-time prognosis monitoring, and drug management. The implementation of AI greatly reduces the need for manual intervention while significantly improving efficiency and accuracy. This enables doctors to make more informed decisions in clinical practice and assists in promoting the standardization of clinical diagnosis and treatment.
Despite the great convenience brought by AI, there are still many challenges to integrating it into clinical practice, highlighting directions for subsequent research. First, it is necessary to fill the gap of specific criteria for AI-assisted diagnosis of hematological diseases and establish a standardized database. The classification of chromosomes in the karyotype analysis of patients with blood diseases is cumbersome and error-prone. Most of the existing AI models have been developed from different private datasets with poor transferability. To address this, a research team has established publicly available clinical chromosome classification datasets (120). Similarly, the LeukmiR genetic database was developed based on AI algorithms (63).
Second, the application of AI products needs to be evaluated in clinical trials, which requires the establishment of comprehensive reporting guidelines. These guidelines ensure standardized data output, address potential sources of bias specific to AI interventions, and enable integration into AI DL models. Ensuring the successful application and transferability of AI models across teams or laboratories is crucial. To address potential risks associated with AI use in the clinic, prospective evaluations involving AI interventions are necessary. Since the first reporting guidelines for clinical trials involving AI interventions (SPIRIT-AI) were developed in 2019 and the first international standards for clinical trials were jointly released in 2020 (121–126), the quality of clinical trials has improved. The latest guidelines indicate that in addition to the core items of CONSORT 2010, the CONSORT AI extension adds 14 new items (127), and the SPIRIT AI extension adds 15 new items (128). These additions address the processing of inputs and outputs, the setting of interventions, the interaction between humans and AI, and the analysis of error cases. This improves transparency and integrity in reporting clinical trials of AI interventions. In addition, the Minimum Information about Clinical Artificial Intelligence Modelling (MI-CLAIM) guidelines and the Minimum Information for Medical AI Reporting (MINIMAR) series have been released. These guidelines are designed to help researchers improve their designs and model quality (121). It is believed that more comprehensive guidelines will be developed to evaluate and regulate the clinical application of AI products in the future.
In addition, with the rapid development of AI technologies such as ChatGPT and their application in the field of education, humans are gradually moving toward a new path of collaborative development and symbiosis with AI in education (129). Similarly, the collaboration between medical professionals and AI is becoming essential for the advancement of AI in healthcare, ensuring that AI can effectively adapt to and enhance medical treatment. The current clinical and research environment suggests an urgent need for more clinicians with strong professional abilities to participate in the research and development of AI systems. This involvement will help replicate and promote the experience of doctors, produce more AI products, and ensure that these technologies are closely aligned with clinical needs to better serve patients. Accelerating the use of AI and clinical decision support systems (AI-CDSSs) in clinical practice is essential. However, there is a shortage of high-end composite talents in the field. According to international surveys, undergraduate medical students show a strong interest in AI and have high expectations but most have not received relevant education. They often do not understand the basic principles, limitations, and potential biases of various ML algorithms (130–133). However, large AI language models such as ChatGPT (129) can be used as virtual teaching aids to provide students with personalized and immediate medical knowledge and conduct interactive simulation learning and detection. Therefore, significant changes will gradually take place in the field of medical education. Increasingly more undergraduate medical colleges are beginning to focus on training students in AI technology. This includes providing personalized learning experiences, enhanced diagnostic training, and risk-free practical experiences (134). The School of Medicine at the University of Paris has introduced an elective course on AI-CDSS. This program allows medical students to assume the role of AI-CDSS designers and develop projects from six key aspects: determining needs, defining goals, designing educational strategies, implementation, formulating assessments, and designing project evaluations. The aim is to cultivate digital health skills and critical thinking, preparing students to adapt to the rapidly evolving digital environment (135). Dartmouth’s Geisel School of Medicine also offers elective courses on AI and its clinical applications. These courses include a preclinical hematology module, which enhances students’ understanding of advanced AI algorithms and models (136). Emory University School of Medicine offers an elective course on web-based resources and related research papers for fourth-year medical students without a background in data science or programming. An analysis of student project reports from this course concluded that even 1 month of AI and ML education during the undergraduate period can significantly improve students’ confidence in their understanding and self-reporting of AI and ML concepts (137), preparing them for current and future developments and changes in practices. Integrating AI into education fosters team cooperation with open communication between medical students, clinical medical workers, and AI experts. This approach leverages the technical skills of AI experts, the rich experience of clinical workers, and the valuable opinions and expectations of medical students, ensuring that research and development are closely aligned with clinical practice and meet clinical needs and preferences. However, this type of medical education has not been widely promoted on a global scale, primarily because of the lack of teachers knowledgeable in AI and the absence of standard guidelines for its use. To promote the effective and responsible use of AI in the future, several recommendations have been proposed: ensuring the transparency of AI system development and deployment, resolving biases in AI algorithms to ensure educational equity, verifying the output of AI educational tools, protecting the privacy of input data, obtaining informed consent, promoting multi-party cooperation, training teachers in AI ethics, conducting important ideological education, continuously maintaining the performance of AI algorithms, establishing clear accountability systems, and improving usage standards (134). In conclusion, future doctors should follow current development trends and be taught to become key users of AI as early as possible. They should understand the principles and basic scope of various algorithms and models and be able to critically analyze and evaluate the data and suggestions provided by AI. This approach will inject fresh perspectives and active force into the future optimization and standardization of clinical diagnosis and treatment via AI (74).
With the increasing integration of AI and ML algorithms into medical education, people’s understanding of high-end technology continues to deepen, and their willingness to use AI technology continues to grow. However, alongside this enthusiasm, there are also fears and concerns about human judgment being gradually reduced and replaced by AI (138). However, in fact, most studies have selectively highlighted the advantages and clinical applicability of AI products, often leading to exaggerated results to meet expectations. In addition, there are ethical challenges related to patient privacy, inherent biases in algorithms, and the interpretability-associated shortcomings of AI. In the future, it is expected that AI technology will be able to integrate medical data from any data owner worldwide without violating privacy laws, thereby crossing the technological divide and driving precision medicine for blood diseases (139).
To better use AI to promote the standardized diagnosis and treatment of blood diseases, we can comprehensively analyze and compare the current AI-assisted technology platforms or models applicable to this field. By selecting the optimal AI system, we can build a comprehensive platform for standardized, AI-assisted diagnosis and treatment of blood diseases based on the clinical pathway of the diagnosis and treatment of blood diseases. Continuous ML updates can improve diagnostic accuracy, guide clinical treatment, recommend the best and most personalized treatments, and dynamically track and adjust treatment plans as the patient’s condition changes. The platform or model should adhere to the clinical pathway, including data collection and sorting, data preprocessing and feature extraction, diagnosis, dynamic optimization of treatment regimens, prediction of disease progression, prognosis, and nursing guidance. This approach will promote the standardization of diagnosis and treatment of blood diseases. Recent research has also proposed a future involving multi-model integrated interpretation of data, where information from cell morphology samples, genetic analysis, and other datasets is combined to form a human–AI feedback loop (108). This approach aims to provide an overall semantic understanding as well as thoughtful diagnostic prediction and interpretation (140). In this way, hematologists can be assisted in real time to make the best clinical decisions. Integrated AI tools combining different ML techniques can automate repetitive tasks, including the assessment of cell morphology, FC, genetic data, and genetic mutation data, integrating all diagnostic modalities for the same blood disorder (74). However, the development and use of such multimodal DL methods should be accompanied by the establishment of regulatory oversight, legal frameworks, and monitoring systems to ensure effective clinical disclosure and safe application of AI products.
6 Conclusion
AI based on ML and DL algorithms has been continuously applied to the comprehensive diagnosis and precision treatment in hematological diseases (Table 6). Its powerful data analysis capability can improve the speed of laboratory diagnosis while maintaining or even surpassing the accuracy of human diagnosis. Notably, some AI digital recognition systems offer high degrees of visualization, presenting analysis and diagnosis results to clinicians in an intuitive and visual manner. In treating blood diseases, issues such as drug resistance, GVHD in allo-HSCT, and relapse are common. With the integration of various clinical data and ML, AI has been able to uncover potential pathogeneses, drug resistance mechanisms, and new therapeutic targets, providing more accurate guidance for drug innovation, risk stratification, and prognosis tracking. Despite the lack of AI product standards, the absence of standardized ML data, the challenges posed by the intersection of medicine and engineering, and the difficulty of interpreting algorithm models, progress has been made by establishing digital databases of blood diseases, formulating laws and regulations on AI ethics and privacy supervision, integrating AI algorithm education into medical undergraduate programs, and developing interpretable AI models. In short, AI has the potential to markedly enhance the precision of clinical diagnosis and treatment of hematological diseases. However, the ultimate goal is not to replace humans with AI but to aid the standardization of clinical diagnosis and treatment through AI products. To further improve and standardize precise diagnosis strategies and the treatment of blood diseases in the context of “AI + medicine,” more research is needed to build an integrated intelligent diagnosis and treatment platform that aligns with clinical pathways.
Author contributions
S-XW: Writing – original draft, Visualization. Z-FH: Writing – review & editing. JL: Writing – review & editing. YW: Writing – review & editing. JD: Writing – review & editing. TL: Writing – review & editing.
Funding
The author(s) declare that financial support was received for the research, authorship, and/or publication of this article. This research was supported by the Guiding Science and Technology Program of Ganzhou City (20222ZDX7576) and Family Planning Commission of Jiangxi Province (202210918) and the Science and Technology Department of Jiangxi Province (20223BCG74003).
Acknowledgments
Figures 1–3 were drawn by Figdraw.
Conflict of interest
The authors declare that the research was conducted in the absence of any commercial or financial relationships that could be construed as a potential conflict of interest.
Publisher’s note
All claims expressed in this article are solely those of the authors and do not necessarily represent those of their affiliated organizations, or those of the publisher, the editors and the reviewers. Any product that may be evaluated in this article, or claim that may be made by its manufacturer, is not guaranteed or endorsed by the publisher.
References
1. Gedefaw, L, Liu, CF, Ip, RKL, Tse, HF, Yeung, MHY, Yip, SP, et al. Artificial intelligence-assisted diagnostic cytology and genomic testing for hematologic disorders. Cells. (2023) 12:1755. doi: 10.3390/cells12131755
2. Handelman, GS, Kok, HK, Chandra, RV, Razavi, AH, Huang, S, Brooks, M, et al. Peering into the black box of artificial intelligence: evaluation metrics of machine learning methods. AJR Am J Roentgenol. (2019) 212:38–43. doi: 10.2214/ajr.18.20224
3. Lin, E, Fuda, F, Luu, HS, Cox, AM, Fang, F, Feng, J, et al. Digital pathology and artificial intelligence as the next chapter in diagnostic hematopathology. Semin Diagn Pathol. (2023) 40:88–94. doi: 10.1053/j.semdp.2023.02.001
4. Katz, BZ, Feldman, MD, Tessema, M, Benisty, D, Toles, GS, Andre, A, et al. Evaluation of Scopio Labs X100 full field PBS: the first high-resolution full field viewing of peripheral blood specimens combined with artificial intelligence-based morphological analysis. Int J Lab Hematol. (2021) 43:1408–16. doi: 10.1111/ijlh.13681
5. Makhija, K, Lincz, LF, Attalla, K, Scorgie, FE, Enjeti, AK, and Prasad, R. White blood cell evaluation in haematological malignancies using a web-based digital microscopy platform. Int J Lab Hematol. (2021) 43:1379–87. doi: 10.1111/ijlh.13657
6. Fu, X, Fu, M, Li, Q, Peng, X, Lu, J, Fang, F, et al. Morphogo: an automatic bone marrow cell classification system on digital images analyzed by artificial intelligence. Acta Cytol. (2020) 64:588–96. doi: 10.1159/000509524
7. Ko, BS, Wang, YF, Li, JL, Li, CC, Weng, PF, Hsu, SC, et al. Clinically validated machine learning algorithm for detecting residual diseases with multicolor flow cytometry analysis in acute myeloid leukemia and myelodysplastic syndrome. EBioMedicine. (2018) 37:91–100. doi: 10.1016/j.ebiom.2018.10.042
8. Ni, W, Tong, X, Qian, W, Jin, J, and Zhao, H. Discrimination of malignant neutrophils of chronic myelogenous leukemia from normal neutrophils by support vector machine. Comput Biol Med. (2013) 43:1192–5. doi: 10.1016/j.compbiomed.2013.06.004
9. Zhong, P, Hong, M, He, H, Zhang, J, Chen, Y, Wang, Z, et al. Diagnosis of acute leukemia by multiparameter flow cytometry with the assistance of artificial intelligence. Diagnostics. (2022) 12:827. doi: 10.3390/diagnostics12040827
10. Qin, Y, Wen, J, Zheng, H, Huang, X, Yang, J, Song, N, et al. Varifocal-net: a chromosome classification approach using deep convolutional networks. IEEE Trans Med Imaging. (2019) 38:2569–81. doi: 10.1109/tmi.2019.2905841
11. Xia, C, Wang, J, Qin, Y, Wen, J, Liu, Z, Song, N, et al. Karyonet: chromosome recognition with end-to-end combinatorial optimization network. IEEE Trans Med Imaging. (2023) 42:2899–911. doi: 10.1109/tmi.2023.3268889
12. Liu, X, Fu, L, Chun-Wei Lin, J, and Liu, S. SRAS-net: low-resolution chromosome image classification based on deep learning. IET Syst Biol. (2022) 16:85–97. doi: 10.1049/syb2.12042
13. Zhang, J, Hu, W, Li, S, Wen, Y, Bao, Y, Huang, H, et al. Chromosome classification and straightening based on an interleaved and multi-task network. IEEE J Biomed Health Inform. (2021) 25:3240–51. doi: 10.1109/jbhi.2021.3062234
14. Li, J, Zheng, S, Shui, Z, Zhang, S, Yang, L, Sun, Y, et al. Masked conditional variational autoencoders for chromosome straightening. IEEE Trans Med Imaging. (2024) 43:216–28. doi: 10.1109/tmi.2023.3293854
15. Cao, F, Zhang, Y, Cai, Y, Animesh, S, Zhang, Y, Akincilar, SC, et al. Chromatin interaction neural network (ChINN): a machine learning-based method for predicting chromatin interactions from DNA sequences. Genome Biol. (2021) 22:226. doi: 10.1186/s13059-021-02453-5
16. Bhinder, B, Gilvary, C, Madhukar, NS, and Elemento, O. Artificial intelligence in cancer research and precision medicine. Cancer Discov. (2021) 11:900–15. doi: 10.1158/2159-8290.Cd-21-0090
17. Chen, ZH, Lin, L, Wu, CF, Li, CF, Xu, RH, and Sun, Y. Artificial intelligence for assisting cancer diagnosis and treatment in the era of precision medicine. Cancer Commun. (2021) 41:1100–15. doi: 10.1002/cac2.12215
18. Schork, NJ . Artificial intelligence and personalized medicine. Cancer Treat Res. (2019) 178:265–83. doi: 10.1007/978-3-030-16391-4_11
19. Zhang, Z, and Wei, X. Artificial intelligence-assisted selection and efficacy prediction of antineoplastic strategies for precision cancer therapy. Semin Cancer Biol. (2023) 90:57–72. doi: 10.1016/j.semcancer.2023.02.005
20. Janssen, APA, Grimm, SH, Wijdeven, RHM, Lenselink, EB, Neefjes, J, van Boeckel, CAA, et al. Drug discovery maps, a machine learning model that visualizes and predicts kinome-inhibitor interaction landscapes. J Chem Inf Model. (2019) 59:1221–9. doi: 10.1021/acs.jcim.8b00640
21. Elshoeibi, AM, Badr, A, Elsayed, B, Metwally, O, Elshoeibi, R, Elhadary, MR, et al. Integrating AI and ML in myelodysplastic syndrome diagnosis: state-of-the-art and future prospects. Cancers. (2023) 16:65. doi: 10.3390/cancers16010065
22. Chandradevan, R, Aljudi, AA, Drumheller, BR, Kunananthaseelan, N, Amgad, M, Gutman, DA, et al. Machine-based detection and classification for bone marrow aspirate differential counts: initial development focusing on nonneoplastic cells. Lab Investig. (2020) 100:98–109. doi: 10.1038/s41374-019-0325-7
23. Matek, C, Krappe, S, Münzenmayer, C, Haferlach, T, and Marr, C. Highly accurate differentiation of bone marrow cell morphologies using deep neural networks on a large image data set. Blood. (2021) 138:1917–27. doi: 10.1182/blood.2020010568
24. Elsayed, B, Elhadary, M, Elshoeibi, RM, Elshoeibi, AM, Badr, A, Metwally, O, et al. Deep learning enhances acute lymphoblastic leukemia diagnosis and classification using bone marrow images. Front Oncol. (2023) 13:1330977. doi: 10.3389/fonc.2023.1330977
25. Liu, J, Yuan, R, Li, Y, Zhou, L, Zhang, Z, Yang, J, et al. A deep learning method and device for bone marrow imaging cell detection. Ann Transl Med. (2022) 10:208. doi: 10.21037/atm-22-486
26. Ouyang, N, Wang, W, Ma, L, Wang, Y, Chen, Q, Yang, S, et al. Diagnosing acute promyelocytic leukemia by using convolutional neural network. Clin Chim Acta. (2021) 512:1–6. doi: 10.1016/j.cca.2020.10.039
27. Eckardt, JN, Schmittmann, T, Riechert, S, Kramer, M, Sulaiman, AS, Sockel, K, et al. Deep learning identifies acute promyelocytic leukemia in bone marrow smears. BMC Cancer. (2022) 22:201. doi: 10.1186/s12885-022-09307-8
28. Wang, M, Dong, C, Gao, Y, Li, J, Han, M, and Wang, L. A deep learning model for the automatic recognition of aplastic anemia, myelodysplastic syndromes, and acute myeloid leukemia based on bone marrow smear. Front Oncol. (2022) 12:844978. doi: 10.3389/fonc.2022.844978
29. Wu, YY, Huang, TC, Ye, RH, Fang, WH, Lai, SW, Chang, PY, et al. A hematologist-level deep learning algorithm (BMSNet) for assessing the morphologies of single nuclear balls in bone marrow smears: algorithm development. JMIR Med Inform. (2020) 8:e15963. doi: 10.2196/15963
30. Wang, W, Luo, M, Guo, P, Wei, Y, Tan, Y, and Shi, H. Artificial intelligence-assisted diagnosis of hematologic diseases based on bone marrow smears using deep neural networks. Comput Methods Prog Biomed. (2023) 231:107343. doi: 10.1016/j.cmpb.2023.107343
31. Bermejo-Peláez, D, Rueda Charro, S, García Roa, M, Trelles-Martínez, R, Bobes-Fernández, A, Hidalgo Soto, M, et al. Digital microscopy augmented by artificial intelligence to interpret bone marrow samples for hematological diseases. Microsc Microanal. (2024) 30:151–9. doi: 10.1093/micmic/ozad143
32. Ahmed, N, Yigit, A, Isik, Z, and Alpkocak, A. Identification of leukemia subtypes from microscopic images using convolutional neural network. Diagnostics. (2019) 9:104. doi: 10.3390/diagnostics9030104
33. Abunadi, I, and Senan, EM. Multi-method diagnosis of blood microscopic sample for early detection of acute lymphoblastic leukemia based on deep learning and hybrid techniques. Sensors. (2022) 22:1629. doi: 10.3390/s22041629
34. Eckardt, JN, Middeke, JM, Riechert, S, Schmittmann, T, Sulaiman, AS, Kramer, M, et al. Deep learning detects acute myeloid leukemia and predicts NPM1 mutation status from bone marrow smears. Leukemia. (2022) 36:111–8. doi: 10.1038/s41375-021-01408-w
35. Dehkharghanian, T, Mu, Y, Tizhoosh, HR, and Campbell, CJV. Applied machine learning in hematopathology. Int J Lab Hematol. (2023) 45:87–94. doi: 10.1111/ijlh.14110
36. Katz, BZ, Benisty, D, Sayegh, Y, Lamm, I, and Avivi, I. Remote digital microscopy improves hematology laboratory workflow by reducing peripheral blood smear analysis turnaround time. Appl Clin Inform. (2022) 13:1108–15. doi: 10.1055/a-1957-6219
37. Lincz, LF, Makhija, K, Attalla, K, Scorgie, FE, Enjeti, AK, and Prasad, R. A comparative evaluation of three consecutive artificial intelligence algorithms released by Techcyte for identification of blasts and white blood cells in abnormal peripheral blood films. Int J Lab Hematol. (2024) 46:92–8. doi: 10.1111/ijlh.14180
38. Sirinukunwattana, K, Aberdeen, A, Theissen, H, Sousos, N, Psaila, B, Mead, AJ, et al. Artificial intelligence-based morphological fingerprinting of megakaryocytes: a new tool for assessing disease in MPN patients. Blood Adv. (2020) 4:3284–94. doi: 10.1182/bloodadvances.2020002230
39. Porwit, A, Béné, MC, Duetz, C, Matarraz, S, Oelschlaegel, U, Westers, TM, et al. Multiparameter flow cytometry in the evaluation of myelodysplasia: analytical issues: recommendations from the European LeukemiaNet/International Myelodysplastic Syndrome Flow Cytometry Working Group. Cytometry B. (2023) 104:27–50. doi: 10.1002/cyto.b.22108
40. Lewis, JE, Cooper, LAD, Jaye, DL, and Pozdnyakova, O. Automated deep learning-based diagnosis and molecular characterization of acute myeloid leukemia using flow cytometry. Mod Pathol. (2024) 37:100373. doi: 10.1016/j.modpat.2023.100373
41. Wang, Y-F, Li, G-H, Hanson, JR, Tario, JD Jr, Monaghan, SA, Ko, B-S, et al. Machine learning-facilitated cross-panel automatic classification at both sample and cell level in acute myeloid leukemia. Blood. (2023) 142:5019. doi: 10.1182/blood-2023-186683
42. Lu, Z, Morita, M, Yeager, TS, Lyu, Y, Wang, SY, Wang, Z, et al. Validation of artificial intelligence (AI)-assisted flow cytometry analysis for immunological disorders. Diagnostics. (2024) 14:420. doi: 10.3390/diagnostics14040420
43. Chong, Y, Lee, JY, Kim, Y, Choi, J, Yu, H, Park, G, et al. A machine-learning expert-supporting system for diagnosis prediction of lymphoid neoplasms using a probabilistic decision-tree algorithm and immunohistochemistry profile database. J Pathol Transl Med. (2020) 54:462–70. doi: 10.4132/jptm.2020.07.11
44. Abdul-Ghafar, J, Seo, KJ, Jung, HR, Park, G, Lee, SS, and Chong, Y. Validation of a machine learning expert supporting system, ImmunoGenius, using immunohistochemistry results of 3000 patients with lymphoid neoplasms. Diagnostic. (2023) 13:1308. doi: 10.3390/diagnostics13071308
45. Chong, Y, Thakur, N, Lee, JY, Hwang, G, Choi, M, Kim, Y, et al. Diagnosis prediction of tumours of unknown origin using immunogenius, a machine learning-based expert system for immunohistochemistry profile interpretation. Diagn Pathol. (2021) 16:19. doi: 10.1186/s13000-021-01081-8
46. Walter, W, Haferlach, C, Nadarajah, N, Schmidts, I, Kühn, C, Kern, W, et al. How artificial intelligence might disrupt diagnostics in hematology in the near future. Oncogene. (2021) 40:4271–80. doi: 10.1038/s41388-021-01861-y
47. Nimitha, N, Ezhumalai, P, and Chokkalingam, A. An improved deep convolutional neural network architecture for chromosome abnormality detection using hybrid optimization model. Microsc Res Tech. (2022) 85:3115–29. doi: 10.1002/jemt.24170
48. Vajen, B, Hänselmann, S, Lutterloh, F, Käfer, S, Espenkötter, J, Beening, A, et al. Classification of fluorescent R-band metaphase chromosomes using a convolutional neural network is precise and fast in generating karyograms of hematologic neoplastic cells. Cancer Genet. (2022) 260-261:23–9. doi: 10.1016/j.cancergen.2021.11.005
49. Bokhari, Y, Alhareeri, A, Aljouie, A, Alkhaldi, A, Rashid, M, Alawad, M, et al. Chromoenhancer: An artificial-intelligence-based tool to enhance neoplastic karyograms as an aid for effective analysis. Cells. (2022) 11:2244. doi: 10.3390/cells11142244
50. Warnat-Herresthal, S, Perrakis, K, Taschler, B, Becker, M, Baßler, K, Beyer, M, et al. Scalable prediction of acute myeloid leukemia using high-dimensional machine learning and blood transcriptomics. iScience. (2020) 23:100780. doi: 10.1016/j.isci.2019.100780
51. Vasighizaker, A, Sharma, A, and Dehzangi, A. A novel one-class classification approach to accurately predict disease-gene association in acute myeloid leukemia cancer. PLoS One. (2019) 14:e0226115. doi: 10.1371/journal.pone.0226115
52. Maleknia, M, Ahmadirad, N, Golab, F, Katebi, Y, and Ketabforoush, AHME. DNA methylation in cancer: epigenetic view of dietary and lifestyle factors. Epigenet Insights. (2023) 16:25168657231199893. doi: 10.1177/25168657231199893
53. Reilly, B, Tanaka, TN, Diep, D, Yeerna, H, Tamayo, P, Zhang, K, et al. DNA methylation identifies genetically and prognostically distinct subtypes of myelodysplastic syndromes. Blood Adv. (2019) 3:2845–58. doi: 10.1182/bloodadvances.2019000192
54. Koldobskiy, MA, Jenkinson, G, Abante, J, Rodriguez DiBlasi, VA, Zhou, W, Pujadas, E, et al. Converging genetic and epigenetic drivers of paediatric acute lymphoblastic leukaemia identified by an information-theoretic analysis. Nat Biomed Eng. (2021) 5:360–76. doi: 10.1038/s41551-021-00703-2
55. Levy, JJ, Chen, Y, Azizgolshani, N, Petersen, CL, Titus, AJ, Moen, EL, et al. MethylSPWNet and MethylCapsNet: biologically motivated organization of DNAm neural network, inspired by capsule NetworksMethylSPWNet and MethylCapsNet: biologically motivated organization of DNAm neural network, inspired by capsule networks. npj Syst Biol Appl. (2021) 7:33. doi: 10.1038/s41540-021-00193-7
56. Teschendorff, AE, Zhu, T, Breeze, CE, and Beck, S. Episcore: cell type deconvolution of bulk tissue DNA methylomes from single-cell RNA-seq data. Genome Biol. (2020) 21:221. doi: 10.1186/s13059-020-02126-9
57. Zhu, T, and Teschendorff, AE. Cell-type deconvolution of bulk DNA methylation data with EpiSCORE. Methods Mol Biol. (2023) 2629:23–42. doi: 10.1007/978-1-0716-2986-4_3
58. Levy, JJ, Titus, AJ, Petersen, CL, Chen, Y, Salas, LA, and Christensen, BC. MethylNet: an automated and modular deep learning approach for DNA methylation analysis. BMC Bioinformatics. (2020) 21:108. doi: 10.1186/s12859-020-3443-8
59. Xu, C, and Jackson, SA. Machine learning and complex biological data. Genome Biol. (2019) 20:76. doi: 10.1186/s13059-019-1689-0
60. Tojo, A . Clinical sequencing in leukemia with the assistance of artificial intelligence. Rinsho Ketsueki. (2017) 58:1913–7. doi: 10.11406/rinketsu.58.1913
61. Yokoyama, K . Artificial intelligence-guided precision medicine in hematological disorders. Rinsho Ketsueki. (2020) 61:554–63. doi: 10.11406/rinketsu.61.554
62. Haferlach, T, and Walter, W. Challenging gold standard hematology diagnostics through the introduction of whole genome sequencing and artificial intelligence. Int J Lab Hematol. (2023) 45:156–62. doi: 10.1111/ijlh.14033
63. Rawoof, A, Swaminathan, G, Tiwari, S, Nair, RA, and Dinesh, KL. LeukmiR: a database for miRNAs and their targets in acute lymphoblastic leukemia. Database. (2020) 2020:baz151. doi: 10.1093/database/baz151
64. Hong, M, Tao, S, Zhang, L, Diao, LT, Huang, X, Huang, S, et al. RNA sequencing: new technologies and applications in cancer research. J Hematol Oncol. (2020) 13:166. doi: 10.1186/s13045-020-01005-x
65. Fan, J, Slowikowski, K, and Zhang, F. Single-cell transcriptomics in cancer: computational challenges and opportunities. Exp Mol Med. (2020) 52:1452–65. doi: 10.1038/s12276-020-0422-0
66. Lei, Y, Tang, R, Xu, J, Wang, W, Zhang, B, Liu, J, et al. Applications of single-cell sequencing in cancer research: progress and perspectives. J Hematol Oncol. (2021) 14:91. doi: 10.1186/s13045-021-01105-2
67. Schmidt, F, Ranjan, B, Lin, QXX, Krishnan, V, Joanito, I, Honardoost, MA, et al. RCA2: a scalable supervised clustering algorithm that reduces batch effects in scRNA-seq data. Nucleic Acids Res. (2021) 49:8505–19. doi: 10.1093/nar/gkab632
68. Wang, T, Johnson, TS, Shao, W, Lu, Z, Helm, BR, Zhang, J, et al. Bermuda: a novel deep transfer learning method for single-cell RNA sequencing batch correction reveals hidden high-resolution cellular subtypes. Genome Biol. (2019) 20:165. doi: 10.1186/s13059-019-1764-6
69. Ho, M, Bianchi, G, and Anderson, KC. Proteomics-inspired precision medicine for treating and understanding multiple myeloma. Expert Rev Precis Med Drug Dev. (2020) 5:67–85. doi: 10.1080/23808993.2020.1732205
70. Sampson, DL, Parker, TJ, Upton, Z, and Hurst, CP. A comparison of methods for classifying clinical samples based on proteomics data: a case study for statistical and machine learning approaches. PLoS One. (2011) 6:e24973. doi: 10.1371/journal.pone.0024973
71. Vizza, P, Aracri, F, Guzzi, PH, Gaspari, M, Veltri, P, and Tradigo, G. Machine learning pipeline to analyze clinical and proteomics data: experiences on a prostate cancer case. BMC Med Inform Decis Mak. (2024) 24:93. doi: 10.1186/s12911-024-02491-6
72. Villiers, W, Kelly, A, He, X, Kaufman-Cook, J, Elbasir, A, Bensmail, H, et al. Multi-omics and machine learning reveal context-specific gene regulatory activities of PML::RARA in acute promyelocytic leukemia. Nat Commun. (2023) 14:724. doi: 10.1038/s41467-023-36262-0
73. Liang, CA, Chen, L, Wahed, A, and Nguyen, AND. Proteomics analysis of FLT3-ITD mutation in acute myeloid leukemia using deep learning neural network. Ann Clin Lab Sci. (2019) 49:119–26.
74. Eckardt, JN, Bornhäuser, M, Wendt, K, and Middeke, JM. Application of machine learning in the management of acute myeloid leukemia: current practice and future prospects. Blood Adv. (2020) 4:6077–85. doi: 10.1182/bloodadvances.2020002997
75. Venezian Povoa, L, Ribeiro, CHC, and Silva, ITD. Machine learning predicts treatment sensitivity in multiple myeloma based on molecular and clinical information coupled with drug response. PLoS One. (2021) 16:e0254596. doi: 10.1371/journal.pone.0254596
76. Ghobadi, MZ, Emamzadeh, R, and Afsaneh, E. Exploration of mRNAs and miRNA classifiers for various ATLL cancer subtypes using machine learning. BMC Cancer. (2022) 22:433. doi: 10.1186/s12885-022-09540-1
77. Guerrero, C, Puig, N, Cedena, MT, Goicoechea, I, Perez, C, Garcés, JJ, et al. A machine learning model based on tumor and immune biomarkers to predict undetectable MRD and survival outcomes in multiple myeloma. Clin Cancer Res. (2022) 28:2598–609. doi: 10.1158/1078-0432.Ccr-21-3430
78. Kolluri, S, Lin, J, Liu, R, Zhang, Y, and Zhang, W. Machine learning and artificial intelligence in pharmaceutical research and development: a review. AAPS J. (2022) 24:19. doi: 10.1208/s12248-021-00644-3
79. Li, J, Lu, L, Zhang, YH, Xu, Y, Liu, M, Feng, K, et al. Identification of leukemia stem cell expression signatures through Monte Carlo feature selection strategy and support vector machine. Cancer Gene Ther. (2020) 27:56–69. doi: 10.1038/s41417-019-0105-y
80. Zhang, Y, Liu, D, Li, F, Zhao, Z, Liu, X, Gao, D, et al. Identification of biomarkers for acute leukemia via machine learning-based stemness index. Gene. (2021) 804:145903. doi: 10.1016/j.gene.2021.145903
81. Vamathevan, J, Clark, D, Czodrowski, P, Dunham, I, Ferran, E, Lee, G, et al. Applications of machine learning in drug discovery and development. Nat Rev Drug Discov. (2019) 18:463–77. doi: 10.1038/s41573-019-0024-5
82. Gimenez, N, Tripathi, R, Giró, A, Rosich, L, López-Guerra, M, López-Oreja, I, et al. Systems biology drug screening identifies statins as enhancers of current therapies in chronic lymphocytic leukemia. Sci Rep. (2020) 10:22153. doi: 10.1038/s41598-020-78315-0
83. Lozano, ML, Segú-Vergés, C, Coma, M, Álvarez-Roman, MT, González-Porras, JR, Gutiérrez, L, et al. Elucidating the mechanism of action of the attributed immunomodulatory role of eltrombopag in primary immune thrombocytopenia: an in silico approach. Int J Mol Sci. (2021) 22:6907. doi: 10.3390/ijms22136907
84. Singh, S, Kumar, R, Payra, S, and Singh, SK. Artificial intelligence and machine learning in pharmacological research: bridging the gap between data and drug discovery. Cureus. (2023) 15:e44359. doi: 10.7759/cureus.44359
85. Gu, M, Kovilakam, SC, Dunn, WG, Marando, L, Barcena, C, Mohorianu, I, et al. Multiparameter prediction of myeloid neoplasia risk. Nat Genet. (2023) 55:1523–30. doi: 10.1038/s41588-023-01472-1
86. Mahmood, N, Shahid, S, Bakhshi, T, Riaz, S, Ghufran, H, and Yaqoob, M. Identification of significant risks in pediatric acute lymphoblastic leukemia (ALL) through machine learning (ML) approach. Med Biol Eng Comput. (2020) 58:2631–40. doi: 10.1007/s11517-020-02245-2
87. Nazha, A, Komrokji, R, Meggendorfer, M, Jia, X, Radakovich, N, Shreve, J, et al. Personalized prediction model to risk stratify patients with myelodysplastic syndromes. J Clin Oncol. (2021) 39:3737–46. doi: 10.1200/jco.20.02810
88. Pourhomayoun, M, and Shakibi, M. Predicting mortality risk in patients with COVID-19 using machine learning to help medical decision-making. Smart Health. (2021) 20:100178. doi: 10.1016/j.smhl.2020.100178
89. Yagin, FH, Cicek, İB, Alkhateeb, A, Yagin, B, Colak, C, Azzeh, M, et al. Explainable artificial intelligence model for identifying COVID-19 gene biomarkers. Comput Biol Med. (2023) 154:106619. doi: 10.1016/j.compbiomed.2023.106619
90. Rahimi, S, Chu, C, Grad, R, Karanofsky, M, Arsenault, M, Ronquillo, C, et al. Explainable machine learning model to predict COVID-19 severity among older adults in the province of Quebec. Ann Fam Med. (2023) 21:3619. doi: 10.1370/afm.21.s1.3619
91. Rodríguez-Belenguer, P, Piñana, JL, Sánchez-Montañés, M, Soria-Olivas, E, Martínez-Sober, M, and Serrano-López, AJ. A machine learning approach to identify groups of patients with hematological malignant disorders. Comput Methods Prog Biomed. (2024) 246:108011. doi: 10.1016/j.cmpb.2024.108011
92. Parekh, AE, Shaikh, OA, Simran, MS, and Hasibuzzaman, MA. Artificial intelligence (AI) in personalized medicine: AI-generated personalized therapy regimens based on genetic and medical history: short communication. Ann Med Surg. (2023) 85:5831–3. doi: 10.1097/ms9.0000000000001320
93. Omenn, GS, Magis, AT, Price, ND, and Hood, L. Personal dense dynamic data clouds connect systems biomedicine to scientific wellness. Methods Mol Biol. (2022) 2486:315–34. doi: 10.1007/978-1-0716-2265-0_15
94. Niu, P, Yao, B, Wei, L, Zhu, H, Fang, C, and Zhao, Y. Construction of prognostic risk prediction model based on high-throughput sequencing expression profile data in childhood acute myeloid leukemia. Blood Cells Mol Dis. (2019) 77:43–50. doi: 10.1016/j.bcmd.2019.03.008
95. Eckardt, JN, Röllig, C, Metzeler, K, Kramer, M, Stasik, S, Georgi, JA, et al. Prediction of complete remission and survival in acute myeloid leukemia using supervised machine learning. Haematologica. (2023) 108:690–704. doi: 10.3324/haematol.2021.280027
96. Wagner, S, Vadakekolathu, J, Tasian, SK, Altmann, H, Bornhäuser, M, Pockley, AG, et al. A parsimonious 3-gene signature predicts clinical outcomes in an acute myeloid leukemia multicohort study. Blood Adv. (2019) 3:1330–46. doi: 10.1182/bloodadvances.2018030726
97. Kashef, A, Khatibi, T, and Mehrvar, A. Prediction of cranial radiotherapy treatment in pediatric acute lymphoblastic leukemia patients using machine learning: a case study at Mahak hospital. Asian Pac J Cancer Prev. (2020) 21:3211–9. doi: 10.31557/apjcp.2020.21.11.3211
98. Xu, ZL, and Huang, XJ. Optimizing outcomes for haploidentical hematopoietic stem cell transplantation in severe aplastic anemia with intensive GVHD prophylaxis: a review of current findings. Expert Rev Hematol. (2021) 14:449–55. doi: 10.1080/17474086.2021.1923475
99. Wu, P, Huo, W, Zhao, H, Lv, J, Lv, S, and An, Y. Risk factors and predictive model for mortality in patients undergoing allogeneic hematopoietic stem cell transplantation admitted to the intensive care unit. Exp Ther Med. (2024) 27:168. doi: 10.3892/etm.2024.12457
100. Fuse, K, Uemura, S, Tamura, S, Suwabe, T, Katagiri, T, Tanaka, T, et al. Patient-based prediction algorithm of relapse after allo-HSCT for acute leukemia and its usefulness in the decision-making process using a machine learning approach. Cancer Med. (2019) 8:5058–67. doi: 10.1002/cam4.2401
101. Gandelman, JS, Byrne, MT, Mistry, AM, Polikowsky, HG, Diggins, KE, Chen, H, et al. Machine learning reveals chronic graft-versus-host disease phenotypes and stratifies survival after stem cell transplant for hematologic malignancies. Haematologica. (2019) 104:189–96. doi: 10.3324/haematol.2018.193441
102. Duminuco, A, Mosquera-Orgueira, A, Nardo, A, Di Raimondo, F, and Palumbo, GA. AIPSS-MF machine learning prognostic score validation in a cohort of myelofibrosis patients treated with ruxolitinib. Cancer Rep. (2023) 6:e 1881. doi: 10.1002/cnr2.1881
103. Maffioli, M, Mora, B, Ball, S, Iurlo, A, Elli, EM, Finazzi, MC, et al. A prognostic model to predict survival after 6 months of ruxolitinib in patients with myelofibrosis. Blood Adv. (2022) 6:1855–64. doi: 10.1182/bloodadvances.2021006889
104. Zhou, X, Xu, R, Wu, Y, Zhou, L, and Xiang, T. The role of proteasomes in tumorigenesis. Genes Dis. (2024) 11:101070. doi: 10.1016/j.gendis.2023.06.037
105. Ren, L, Xu, B, Xu, J, Li, J, Jiang, J, Ren, Y, et al. A machine learning model to predict survival and therapeutic responses in multiple myeloma. Int J Mol Sci. (2023) 24:6683. doi: 10.3390/ijms24076683
106. Katsenou, A, O’Farrell, R, Dowling, P, Heckman, CA, O'Gorman, P, and Bazou, D. Using proteomics data to identify personalized treatments in multiple myeloma: a machine learning approach. Int J Mol Sci. (2023) 24:15570. doi: 10.3390/ijms242115570
107. Hill, HA, Jain, P, Ok, CY, Sasaki, K, Chen, H, Wang, ML, et al. Integrative prognostic machine learning models in mantle cell lymphoma. Cancer Res Commun. (2023) 3:1435–46. doi: 10.1158/2767-9764.Crc-23-0083
108. Kratz, A, Lee, SH, Zini, G, Riedl, JA, Hur, M, and Machin, S. Digital morphology analyzers in hematology: ICSH review and recommendations. Int J Lab Hematol. (2019) 41:437–47. doi: 10.1111/ijlh.13042
109. Moser, T, Kühberger, S, Lazzeri, I, Vlachos, G, and Heitzer, E. Bridging biological cfDNA features and machine learning approaches. Trends Genet. (2023) 39:285–307. doi: 10.1016/j.tig.2023.01.004
110. Fan, BE, Yong, BSJ, Li, R, Wang, SSY, Aw, MYN, Chia, MF, et al. From microscope to micropixels: a rapid review of artificial intelligence for the peripheral blood film. Blood Rev. (2024) 64:101144. doi: 10.1016/j.blre.2023.101144
111. Khalid, N, Qayyum, A, Bilal, M, Al-Fuqaha, A, and Qadir, J. Privacy-preserving artificial intelligence in healthcare: techniques and applications. Comput Biol Med. (2023) 158:106848. doi: 10.1016/j.compbiomed.2023.106848
112. Riley, P . Three pitfalls to avoid in machine learning. Nature. (2019) 572:27–9. doi: 10.1038/d41586-019-02307-y
113. Sarkar, A, Praetorius, JP, and Figge, MT. Deep learning-based characterization of neutrophil activation phenotypes in ex vivo human Candida blood infections. Comput Struct Biotechnol J. (2024) 23:1260–73. doi: 10.1016/j.csbj.2024.03.006
114. Liu, X, Sangers, TE, Nijsten, T, Kayser, M, Pardo, LM, Wolvius, EB, et al. Predicting skin cancer risk from facial images with an explainable artificial intelligence (XAI) based approach: a proof-of-concept study. EClinicalMedicine. (2024) 71:102550. doi: 10.1016/j.eclinm.2024.102550
115. van der Velden, BHM, Kuijf, HJ, Gilhuijs, KGA, and Viergever, MA. Explainable artificial intelligence (XAI) in deep learning-based medical image analysis. Med Image Anal. (2022) 79:102470. doi: 10.1016/j.media.2022.102470
116. Zhang, Y, Weng, Y, and Lund, J. Applications of explainable artificial intelligence in diagnosis and surgery. Diagnostics. (2022) 12:237. doi: 10.3390/diagnostics12020237
117. Kırboğa, KK, Abbasi, S, and Küçüksille, EU. Explainability and white box in drug discovery. Chem Biol Drug Des. (2023) 102:217–33. doi: 10.1111/cbdd.14262
118. Ali, S, Akhlaq, F, Imran, AS, Kastrati, Z, Daudpota, SM, and Moosa, M. The enlightening role of explainable artificial intelligence in medical & healthcare domains: a systematic literature review. Comput Biol Med. (2023) 166:107555. doi: 10.1016/j.compbiomed.2023.107555
119. Çubukçu, HC, Topcu, D, and Yenice, S. Machine learning-based clinical decision support using laboratory data. Clin Chem Lab Med. (2024) 62:793–823. doi: 10.1515/cclm-2023-1037
120. Lin, C, Chen, H, Huang, J, Peng, J, Guo, L, Yang, Z, et al. Chromosomenet: a massive dataset enabling benchmarking and building based lines of clinical chromosome classification. Comput Biol Chem. (2022) 100:107731. doi: 10.1016/j.compbiolchem.2022.107731
121. Ibrahim, H, Liu, X, and Denniston, AK. Reporting guidelines for artificial intelligence in healthcare research. Clin Experiment Ophthalmol. (2021) 49:470–6. doi: 10.1111/ceo.13943
122. Ibrahim, H, Liu, X, Rivera, SC, Moher, D, Chan, AW, Sydes, MR, et al. Reporting guidelines for clinical trials of artificial intelligence interventions: the SPIRIT-AI and CONSORT-AI guidelines. Trials. (2021) 22:11. doi: 10.1186/s13063-020-04951-6
123. Cruz Rivera, S, Liu, X, Chan, AW, Denniston, AK, and Calvert, MJSPIRIT-AI and CONSORT-AI Working Group; SPIRIT-AI and CONSORT-AI Steering Group; SPIRIT-AI and CONSORT-AI Consensus Group. Guidelines for clinical trial protocols for interventions involving artificial intelligence: the SPIRIT-AI extension. Nat Med. (2020) 26:1351–63. doi: 10.1038/s41591-020-1037-7
124. Rivera, SC, Liu, X, Chan, AW, Denniston, AK, and Calvert, MJSPIRIT-AI and CONSORT-AI Working Group. Guidelines for clinical trial protocols for interventions involving artificial intelligence: the SPIRIT-AI extension. BMJ. (2020) 370:m3210. doi: 10.1136/bmj.m3210
125. Liu, X, Rivera, SC, Moher, D, Calvert, MJ, and Denniston, AKSPIRIT-AI and CONSORT-AI Working Group. Reporting guidelines for clinical trial reports for interventions involving artificial intelligence: the CONSORT-AI extension. BMJ. (2020) 370:m3164. doi: 10.1136/bmj.m3164
126. Liu, X, Cruz Rivera, S, Moher, D, Calvert, MJ, and Denniston, AKSPIRIT-AI and CONSORT-AI Working Group. Reporting guidelines for clinical trial reports for interventions involving artificial intelligence: the CONSORT-AI extension. Lancet Digit Health. (2020) 2:e537–48. doi: 10.1016/s2589-7500(20)30218-1
127. Liu, X, Cruz Rivera, S, Moher, D, Calvert, MJ, and Denniston, AK. Reporting guidelines for clinical trial reports for interventions involving artificial intelligence: the CONSORT-AI extensionDiretrizes para relatórios de ensaios clínicos com intervenções que utilizam inteligência artificial: a extensão CONSORT-AI. Rev Panam Salud Publica. (2024) 48:e13. doi: 10.26633/rpsp.2024.13
128. Cruz Rivera, S, Liu, X, Chan, AW, Denniston, AK, and Calvert, MJ. Guidelines for clinical trial protocols for interventions involving artificial intelligence: the SPIRIT-AI extensionDiretrizes para protocolos de ensaios clínicos com intervenções que utilizam inteligência artificial: a extensão SPIRIT-AI. Rev Panam Salud Publica. (2023) 47:e149. doi: 10.26633/rpsp.2023.149
129. Leng, L . Challenge, integration, and change: ChatGPT and future anatomical education. Med Educ Online. (2024) 29:2304973. doi: 10.1080/10872981.2024.2304973
130. Stewart, J, Lu, J, Gahungu, N, Goudie, A, Fegan, PG, Bennamoun, M, et al. Western Australian medical students’ attitudes towards artificial intelligence in healthcare. PLoS One. (2023) 18:e0290642. doi: 10.1371/journal.pone.0290642
131. Allam, AH, Eltewacy, NK, Alabdallat, YJ, Owais, TA, Salman, S, and Ebada, MA. Knowledge, attitude, and perception of Arab medical students towards artificial intelligence in medicine and radiology: a multi-national cross-sectional study. Eur Radiol. (2023) 34:1–14. doi: 10.1007/s00330-023-10509-2
132. Sit, C, Srinivasan, R, Amlani, A, Muthuswamy, K, Azam, A, Monzon, L, et al. Attitudes and perceptions of UK medical students towards artificial intelligence and radiology: a multicentre survey. Insights Imaging. (2020) 11:14. doi: 10.1186/s13244-019-0830-7
133. Tung, AYZ, and Dong, LW. Malaysian medical students’ attitudes and readiness toward AI (artificial intelligence): a cross-sectional study. J Med Educat Curri Develop. (2023) 10:23821205231201164. doi: 10.1177/23821205231201164
134. Franco D'Souza, R, Mathew, M, Mishra, V, and Surapaneni, KM. Twelve tips for addressing ethical concerns in the implementation of artificial intelligence in medical education. Med Educ Online. (2024) 29:2330250. doi: 10.1080/10872981.2024.2330250
135. Tsopra, R, Peiffer-Smadja, N, Charlier, C, Campeotto, F, Lemogne, C, Ruszniewski, P, et al. Putting undergraduate medical students in AI-CDSS designers’ shoes: an innovative teaching method to develop digital health critical thinking. Int J Med Inform. (2023) 171:104980. doi: 10.1016/j.ijmedinf.2022.104980
136. Park, SH, Pinto-Powell, R, Thesen, T, Lindqwister, A, Levy, J, Chacko, R, et al. Preparing healthcare leaders of the digital age with an integrative artificial intelligence curriculum: a pilot study. Med Educ Online. (2024) 29:2315684. doi: 10.1080/10872981.2024.2315684
137. Abid, A, Murugan, A, Banerjee, I, Purkayastha, S, Trivedi, H, and Gichoya, J. Ai education for fourth-year medical students: two-year experience of a web-based, self-guided curriculum and mixed methods study. JMIR Med Educ. (2024) 10:e46500. doi: 10.2196/46500
138. Weber, S, Wyszynski, M, Godefroid, M, Plattfaut, R, and Niehaves, B. How do medical professionals make sense (or not) of AI? A social-media-based computational grounded theory study and an online survey. Comput Struct Biotechnol J. (2024) 24:146–59. doi: 10.1016/j.csbj.2024.02.009
139. Warnat-Herresthal, S, Schultze, H, Shastry, KL, Manamohan, S, Mukherjee, S, Garg, V, et al. Swarm learning for decentralized and confidential clinical machine learning. Nature. (2021) 594:265–70. doi: 10.1038/s41586-021-03583-3
140. Tayebi, RM, Mu, Y, Dehkharghanian, T, Ross, C, Sur, M, Foley, R, et al. Automated bone marrow cytology using deep learning to generate a histogram of cell types. Commun Med. (2022) 2:45. doi: 10.1038/s43856-022-00107-6
141. Wang, C, Wei, XL, Li, CX, Wang, YZ, Wu, Y, Niu, YX, et al. Efficient and highly accurate diagnosis of malignant hematological diseases based on whole-slide images using deep learning. Front Oncol. (2022) 12:879308. doi: 10.3389/fonc.2022.879308
142. Musleh, S, Islam, MT, Alam, MT, Househ, M, Shah, Z, and Alam, T. ALLD: acute lymphoblastic leukemia detector. Stud Health Technol Inform. (2022) 289:77–80. doi: 10.3233/shti210863
143. Chen, P, Chen, XR, Chen, N, Zhang, L, Zhang, L, Zhu, J, et al. Detection of metastatic tumor cells in the bone marrow aspirate smears by artificial intelligence (AI)-based Morphogo system. Front Oncol. (2021) 11:742395. doi: 10.3389/fonc.2021.742395
144. Duetz, C, Van Gassen, S, Westers, TM, van Spronsen, MF, Bachas, C, Saeys, Y, et al. Computational flow cytometry as a diagnostic tool in suspected-myelodysplastic syndromes. Cytometry A. (2021) 99:814–24. doi: 10.1002/cyto.a.24360
Keywords: artificial intelligence, machine learning, deep learning, precision medicine, diagnosis, treatment, Hematology
Citation: Wang S-X, Huang Z-F, Li J, Wu Y, Du J and Li T (2024) Optimization of diagnosis and treatment of hematological diseases via artificial intelligence. Front. Med. 11:1487234. doi: 10.3389/fmed.2024.1487234
Edited by:
Eleftheria Hatzimichael, University of Ioannina, GreeceReviewed by:
Ioannis Mitroulis, Democritus University of Thrace, GreecePier Paolo Piccaluga, University of Bologna, Italy
Copyright © 2024 Wang, Huang, Li, Wu, Du and Li. This is an open-access article distributed under the terms of the Creative Commons Attribution License (CC BY). The use, distribution or reproduction in other forums is permitted, provided the original author(s) and the copyright owner(s) are credited and that the original publication in this journal is cited, in accordance with accepted academic practice. No use, distribution or reproduction is permitted which does not comply with these terms.
*Correspondence: Zou-Fang Huang, nfyyjsjj@126.com; Jun Du, dujun-pathfinder@outlook.com; Ting Li, 44369218@qq.com