- 1School of Medicine, Koç University, Istanbul, Türkiye
- 2Department of Neurosurgery, Mount Sinai Health System, New York, NY, United States
- 3College of Human Ecology, Cornell University, Ithaca, NY, United States
Introduction: Large Language Models (LLMs) are sophisticated algorithms that analyze and generate vast amounts of textual data, mimicking human communication. Notable LLMs include GPT-4o by Open AI, Claude 3.5 Sonnet by Anthropic, and Gemini by Google. This scoping review aims to synthesize the current applications and potential uses of LLMs in patient education and engagement.
Materials and methods: Following the PRISMA-ScR checklist and methodologies by Arksey, O’Malley, and Levac, we conducted a scoping review. We searched PubMed in June 2024, using keywords and MeSH terms related to LLMs and patient education. Two authors conducted the initial screening, and discrepancies were resolved by consensus. We employed thematic analysis to address our primary research question.
Results: The review identified 201 studies, predominantly from the United States (58.2%). Six themes emerged: generating patient education materials, interpreting medical information, providing lifestyle recommendations, supporting customized medication use, offering perioperative care instructions, and optimizing doctor-patient interaction. LLMs were found to provide accurate responses to patient queries, enhance existing educational materials, and translate medical information into patient-friendly language. However, challenges such as readability, accuracy, and potential biases were noted.
Discussion: LLMs demonstrate significant potential in patient education and engagement by creating accessible educational materials, interpreting complex medical information, and enhancing communication between patients and healthcare providers. Nonetheless, issues related to the accuracy and readability of LLM-generated content, as well as ethical concerns, require further research and development. Future studies should focus on improving LLMs and ensuring content reliability while addressing ethical considerations.
1 Introduction
Large Language Models (LLMs) are sophisticated algorithms that analyze and generate extensive textual data (1). These models leverage vast corpora of unlabeled text and incorporate reinforcement learning from human feedback to discern syntactical patterns and contextual nuances within languages. Consequently, LLMs can produce responses that closely mimic human communication when presented with diverse, open-ended queries (2–4). Several notable LLMs have emerged recently, including GPT-4o by Open AI (5), Claude 3.5 Sonnet by Anthropic (6), and Gemini by Google (7).
LLMs have demonstrated significant potential in medicine, with transformative applications across various domains, including clinical settings. These AI-powered systems can streamline clinical workflows, help with clinical decision-making, and ultimately improve patient outcomes. Recent studies highlight the utility of LLMs in clinical decision support, providing valuable insights that enable healthcare teams to make more informed treatment decisions (8–10). LLMs also show promise as educational tools by enhancing the quality and accessibility of materials. However, from a patient’s perspective, they present both opportunities and risks. The varying levels of medical knowledge among patients may impede their ability to critically assess the information provided by LLMs, unlike clinicians who are trained to do so.
As of July 2024, there was limited synthesis of knowledge regarding the evidence base, applications, and evaluation methods of LLMs in patient education and engagement. This scoping review aims to address this gap by mapping the available literature on potential applications of LLMs in patient education and identifying future research directions. Our primary research question is: “What are the current and potential uses of LLMs in patient education and engagement as described in the literature?” This review seeks to enhance future discussions on using LLMs for patient care, including education, engagement, workload reduction, patient-centered health customization, and communication.
2 Materials and methods
This study employed a scoping review methodology, adhering to the Preferred Reporting Items for Systematic Reviews and Meta-Analyses Extension for Scoping Reviews (PRISMA-ScR) checklist (11). The review process was based on the methodological framework developed by Arksey and O’Malley (12), with further refinements as proposed by Levac et al. (13).
2.1 Literature search
A literature search was conducted in June 2024 using the PubMed database. The search strategy, detailed in Supplementary Methods S1, combined relevant keywords and Medical Subject Headings (MeSH) terms related to LLMs and patient education.
2.2 Study selection
Citation management was facilitated by Covidence software (Veritas Health Innovation). The inclusion criteria encompassed studies addressing the use, accuracy, relevance, or effectiveness of LLMs in patient education, patient engagement, answering patient-specific questions, or generating patient education materials. Studies were excluded if they did not primarily focus on LLMs for patient education, engagement, or answering patient questions; did not assess LLMs in healthcare settings or had only indirect relations to patients; or focused solely on technical aspects or architecture of LLMs without considering their application in patient education or engagement. A detailed description of the inclusion and exclusion criteria is provided in Supplementary Methods S2.
The selection process involved two stages. In the initial screening, two authors (SA and VV) independently reviewed the titles and abstracts of retrieved articles. Studies passing the initial screening were then read in full by both authors. Studies deemed eligible by both reviewers were included in the analysis. In cases of disagreement, a third author (MK) was consulted to resolve discrepancies.
2.3 Thematic analysis
We employed thematic analysis, following the methodology proposed by Braun and Clarke (14), to address our primary research question. The process began with an author (SA) reading and coding 25 randomly selected articles, focusing on content related to the potential uses of LLMs in patient education and engagement. Subsequently, two authors (SA and MK) examined the remaining manuscripts, seeking additional themes or data that could either reinforce or challenge the established themes. This iterative process facilitated further refinement of the themes through group discussions centered on patient education and engagement.
3 Results
3.1 Literature search
The initial search strategy yielded 661 papers. After removing one duplicate, 660 papers remained for screening. Based on title and abstract screening, 365 papers (55.3%) were excluded. Full-text review was conducted for 295 papers (44.7% of the initial pool), resulting in 201 papers (30% of the initial pool) meeting the study inclusion criteria (Supplementary Figure S1). Supplementary Data S1 presents all of the included papers.
3.2 Descriptive analysis
The geographical distribution of the studies revealed a predominance from the United States, accounting for 58.2% (117/201) of the articles. Turkey and China followed, each contributing 6.4% (13/201) of the articles (Figure 1A). The studies spanned 35 medical specialties, with general medicine representing the largest proportion at 12.9% (26/201), closely followed by orthopedic surgery at 12.4% (25/201), and otolaryngology at 9.4% (19/201) (Figure 1B).
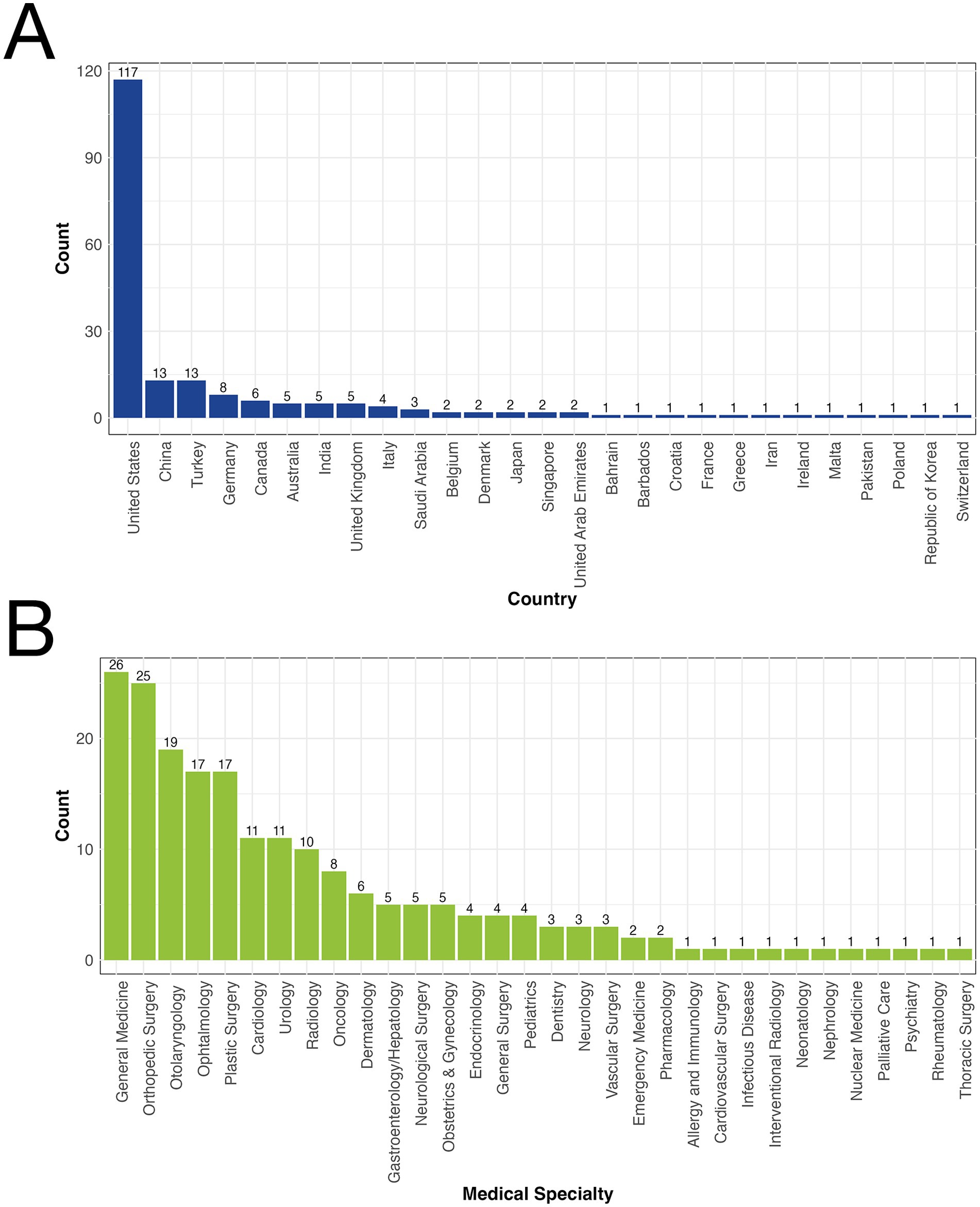
Figure 1. (A) Geographical distribution of studies on large language models (LLMs) in patient education. (B) Specialty distribution of studies on large language models (LLMs) in patient education.
3.3 Thematic analysis
Our analysis identified six main themes with associated subthemes regarding the use of LLMs in patient education and engagement:
1 Generating Patient Education Materials
a Answering Patient Questions
b Enhancing Existing Patient Education Materials
c Translation of Patient Education Materials
2 Interpreting Medical Information from a Patient Perspective
3 Providing Lifestyle Recommendations and Improving Health Literacy
4 Customized Medication Use and Self-Decision
5 Providing Pre-, Peri-, and Post-Operative Care Instructions
6 Optimizing Doctor-Patient Interaction
a Facilitating Understanding of Consent Forms
b Enhancing Communication Establishment
Table 1 presents these six themes as represented across the analyzed articles, along with illustrative quotes. Supplementary Data S2 indicates the theme to which each paper belongs.
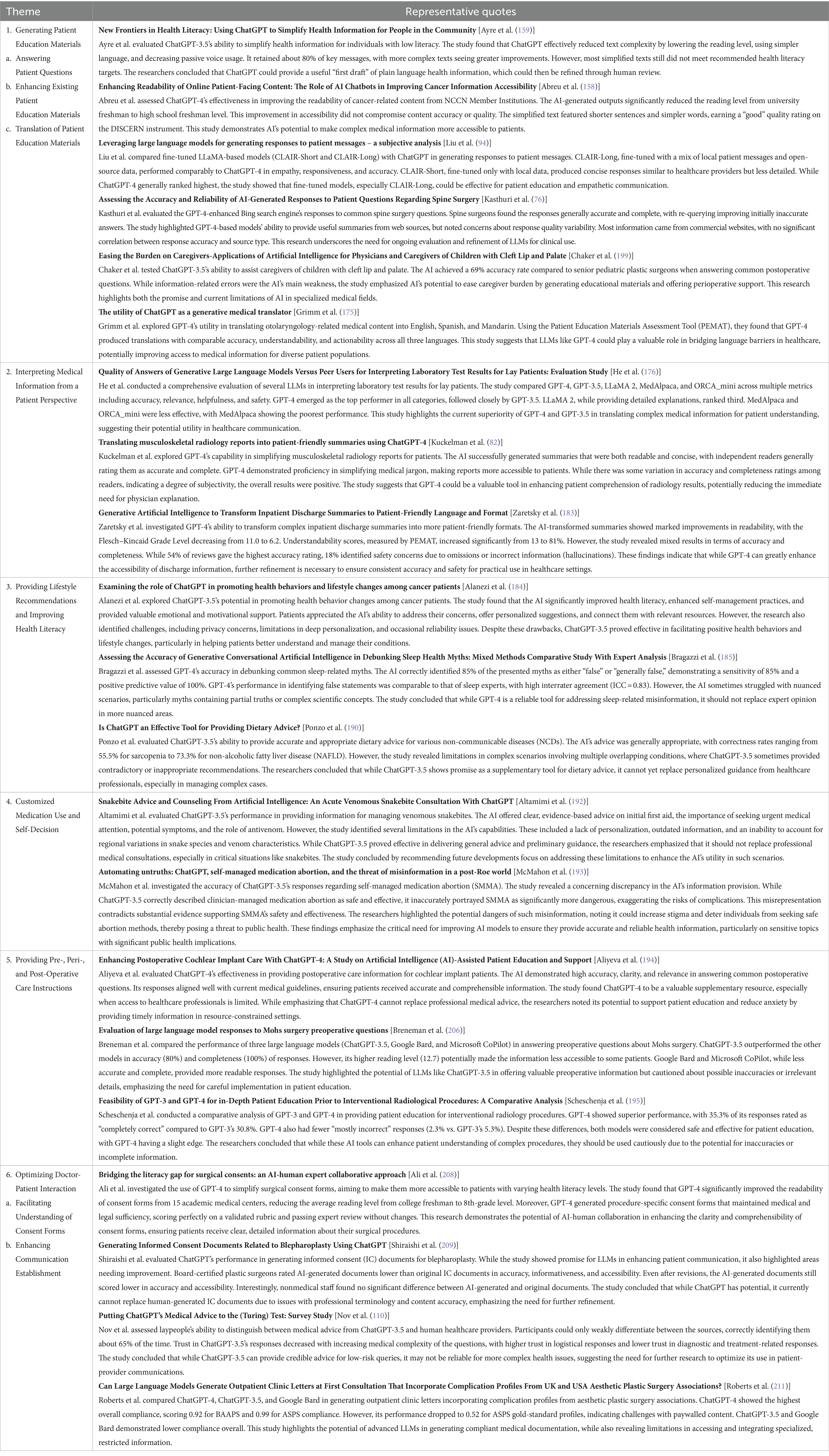
Table 1. Representative quotes illustrating key themes identified in studies on the use of large language models (LLMs) in patient education.
The theme “Generating Patient Education Materials” was predominant, encompassing 80.5% (162/201) of the articles across its three subthemes. Within this theme, “Answering Patient Questions” was the most prevalent subtheme, representing 71.6% (144/201) of all articles. The remaining themes were distributed as follows: “Interpreting Medical Information from a Patient Perspective” and “Providing Lifestyle Recommendations and Improving Health Literacy” each accounted for 4.5% (9/201) of the articles. “Providing Pre-, Peri-, and Post-Operative Care Instructions” was represented in 6.9% (14/201) of the articles, while “Optimizing Doctor-Patient Interaction” appeared in 2.5% (5/201) of the articles. The least represented theme was “Customized Medication Use and Self-Decision,” accounting for 1% (2/201) of the articles.
3.3.1 Theme 1: generating patient education materials
The generation of patient education materials emerged as a prominent theme, with three key subthemes: answering patient questions, enhancing existing materials, and translating medical content. Answering patient questions was the most significant subtheme, representing 71.6% of the articles (8, 15–157). In these studies, LLMs created educational content by responding to common questions, direct patient inquiries, and expert-formulated queries, demonstrating their potential to address diverse patient information needs.
Most studies found LLMs provided accurate responses to patient queries. Almagazzachi et al. reported 92.5% accuracy for ChatGPT’s answers to hypertension questions (18). However, accuracy varied by specialty. In a study on pediatric in-toeing, Amaral et al. found 46% of responses were excellent, and 44% were satisfactory with minimal clarification needed (19). These findings suggest LLMs’ potential in patient education, while highlighting performance differences across medical fields.
The readability of LLM-generated content varied considerably across studies. ChatGPT’s responses often required a higher reading level, potentially limiting accessibility for some patients. Campbell et al. demonstrated that ChatGPT’s unprompted answers on obstructive sleep apnea had a mean Flesch–Kincaid grade level of 14.15, which decreased to 12.45 when prompted (32). This indicates that even with specific instructions, the content remained at a college reading level. In contrast, other LLMs showed better readability in some cases. Chervonski et al. reported that Google BARD produced more accessible content, with responses on vascular surgery diseases achieving a mean Flesch Reading Ease score of 58.9, indicating improved readability (40). When compared to traditional search engines, LLMs revealed a trade-off between comprehensiveness and readability. Cohen et al. found that while ChatGPT provided more detailed and higher-quality responses to cataract surgery FAQs compared to Google, these responses were at a higher reading level (42). These findings suggest that while LLMs may offer more comprehensive information, they do not always improve accessibility for the average patient.
LLMs show promise in transforming existing materials into more readable, patient-centered formats (158–174). Numerous studies demonstrate their ability to enhance readability across various medical education materials (158–161, 163–165, 168, 170–172, 174). Fanning et al. found comparable performance between ChatGPT-3.5 and ChatGPT-4 in improving plastic surgery material readability (166). Moons et al. reported Google BARD surpassed GPT in readability improvement but tended to omit information (169). Some studies, however, found no improvement or decreased readability (162, 167), indicating variability in LLM effectiveness. Interestingly, Sudharshan et al. noted LLMs were more accurate in creating readable Spanish materials (173), suggesting potential for addressing language-specific challenges.
Research on LLMs for translating patient education materials remains limited. However, a significant study by Grimm et al. showed ChatGPT-4’s ability to produce accurate, understandable, and actionable translations of otorhinolaryngology content in English, Spanish, and Mandarin (175). This finding suggests LLMs’ potential in overcoming language barriers in patient education.
3.3.2 Theme 2: interpreting medical information from a patient perspective
Nine studies investigated LLMs’ capacity to interpret complex medical information, evaluating their feasibility, accuracy, readability, and effectiveness in translating medical jargon. He et al. found ChatGPT-4 outperformed other LLMs and human responses from Q&A websites in accuracy, helpfulness, relevance, and safety when answering laboratory test result questions (176). However, Meyer et al. reported that ChatGPT, Gemini, and Le Chat were less accurate and more generalized than certified physicians in interpreting laboratory results (177), highlighting the variability in LLM performance across different contexts.
LLMs demonstrate potential in improving radiological information interpretation and communication. Kuckelman et al. found ChatGPT-4 produced generally accurate summaries of musculoskeletal radiology reports, noting some variability in human interpretation (82). Lyu et al. showed ChatGPT-4 enhanced translated radiology report quality and accessibility, despite occasional oversimplifications (178). Sarangi et al. reported ChatGPT-3.5 effectively simplified radiological reports while maintaining essential diagnostic information, though performance varied across conditions and imaging modalities (179). Several other studies support these findings, suggesting LLMs’ promising role in radiology communication (180–182).
Zaretsky et al. evaluated ChatGPT-4’s ability to convert discharge summaries into patient-friendly formats. The transformed summaries showed significant improvements in readability and understandability. However, the study raised concerns about accuracy and completeness, noting instances of omissions and hallucinations (183).
3.3.3 Theme 3: providing lifestyle recommendations and improving health literacy
Nine studies explored LLMs’ potential in offering lifestyle recommendations and enhancing health literacy. Alanezi et al. found ChatGPT effective in promoting health behavior changes among cancer patients, boosting health literacy and self-management (184). Bragazzi et al. showed ChatGPT’s capability to debunk sleep-related myths and provide accessible advice (185). In a follow-up study, they found Google BARD slightly outperformed ChatGPT-4 in identifying false statements and offering practical sleep-related advice (186). These findings suggest LLMs’ promising role in health education and lifestyle guidance.
Gray et al. demonstrated ChatGPT’s ability to generate realistic prenatal counseling dialogues (187). Minutolo et al. proposed a conversational agent to enhance health literacy by making Patient Information Leaflets queryable (188). Mondal et al. found ChatGPT provided reasonably accurate responses to lifestyle-related disease queries (189). Ponzo et al. reported ChatGPT offered general dietary guidance for NCDs but struggled with complex, multi-condition cases (190). Willms et al. explored ChatGPT’s potential in creating physical activity app content, emphasizing the need for expert review (1). Zaleski et al. found AI-generated exercise recommendations generally accurate but lacking comprehensiveness and at a college reading level (191). These studies highlight LLMs’ diverse applications in health education while noting their limitations.
3.3.4 Theme 4: customized medication use and self-decision
Two studies explored LLMs’ potential in medication guidance and self-decision support. Altamimi et al. found ChatGPT provided accurate advice on acute venomous snakebite management, while emphasizing the importance of professional care (192). In contrast, McMahon et al. observed ChatGPT accurately described clinician-managed abortion as safe but incorrectly portrayed self-managed abortion as dangerous, highlighting potential misinformation risks (193). These findings underscore both the promise and pitfalls of using LLMs for sensitive medical information.
3.3.5 Theme 5: providing pre-/peri-/post-operative care instructions
Studies investigated LLMs’ use in surgical patient education. Aliyeva et al. found ChatGPT-4 excelled in providing postoperative care instructions for cochlear implant patients, especially in remote settings (194). LLMs showed proficiency in offering postoperative guidance across various surgical specialties (180, 195–202). Dhar et al. noted ChatGPT’s accuracy in answering tonsillectomy questions, with some pain management inaccuracies (203). Patil et al. reported ChatGPT provided quality preoperative information for ophthalmic surgeries, though occasionally overlooking adverse events (204). Meyer et al. found ChatGPT reliable for postoperative gynecological surgery instructions (205). Breneman et al. and Kienzle et al. evaluated ChatGPT for preoperative counseling in Mohs surgery and knee arthroplasty, finding it potentially useful but cautioning about non-existing references (206, 207).
3.3.6 Theme 6: optimizing doctor-patient interaction
This theme explores LLMs’ potential to enhance doctor-patient communication, particularly in simplifying consent forms and improving general medical communication. Ali et al. found ChatGPT-4 successfully simplified surgical consent forms to an 8th-grade reading level while maintaining accuracy (208). Shiraishi et al. reported that revised ChatGPT-prepared informed consent documents for blepharoplasty were more desirable than originals (209).
LLMs also showed promise in broader doctor-patient communication. An et al. introduced an LLM-based education model that improved patients’ understanding of their conditions and treatments (210). Roberts et al. demonstrated LLMs could generate comprehensible outpatient clinic letters for cosmetic surgery, potentially saving clinicians’ time (211). Xue et al. found ChatGPT performed well in logical reasoning and medical knowledge education during remote orthopedic consultations (212). These studies highlight LLMs’ potential to enhance various aspects of medical communication.
4 Discussion
This scoping review synthesizes current applications and potential uses of LLMs in patient education and engagement, offering insights into their transformative potential and integration challenges in healthcare settings. LLMs demonstrate significant promise in creating patient education materials, with studies reporting that health-related questions were accurately answered over 90% of the time by systems like ChatGPT, covering a broad range of topics from hypertension to pediatric conditions (18, 31). The depth of these responses potentially offers substantial value to patients seeking detailed understanding of their ailments. However, readability remains a notable concern, potentially limiting accessibility for some patient populations.
LLMs have demonstrated competence in interpreting complex medical information from laboratory reports, radiology results, and discharge summaries. ChatGPT-4, for instance, generated informative summaries of radiology reports, making them more accessible to non-medical professionals (82, 178). However, concerns about the quality and comprehensiveness of LLM-generated information persist. Issues such as hallucinations, omissions, or plausible but incorrect information have been noted. Zaretsky et al. observed that while ChatGPT-4 could transform discharge summaries into more patient-friendly formats, occasional inaccuracies, and omissions could potentially mislead patients (183). These findings underscore the necessity for professional oversight in deploying LLMs in healthcare settings to ensure the reliability and accuracy of AI-generated content.
LLMs show promise as lifestyle recommendations and health literacy tools, effectively encouraging healthy behaviors and dispelling health myths. Alanezi et al. found that ChatGPT provided significant support in developing health literacy among cancer patients, motivating self-management through emotional, informational, and motivational assistance (184). Bragazzi and Garbarino demonstrated ChatGPT’s effectiveness in debunking sleep-related misconceptions, accurately distinguishing between false and genuine health information (185). However, personalization and accuracy remain challenging. While AI can offer useful preliminary advice, it requires further development to provide relevant, situation-specific suggestions tailored to individual patients. This customization is crucial for ensuring that patients can trust and adhere to the recommendations provided.
LLMs play a significant role in providing information on self-medication and personalized drug utilization, offering detailed insights on drug interactions, correct usage, and potential side effects. Altamimi et al. found ChatGPT’s information helpful and accurate in guiding acute venomous snakebite management, though it appropriately emphasized the need for professional medical care (192). LLMs also show potential in patient triage, quickly analyzing symptoms and medical history to prioritize cases based on severity (10). However, the quality of LLM-provided information varies considerably. McMahon et al. reported that ChatGPT gave inaccurate and misleading information about self-managed medication abortion, incorrectly portraying it as dangerous despite evidence of its safety and efficacy (193). This inconsistency highlights the risks of relying on AI without professional oversight and underscores the need for LLMs to provide accurate, up-to-date, and context-sensitive information to support safe self-medication practices.
4.1 Implications and future research
The integration of LLMs into patient education and engagement shows significant potential for improving health literacy and healthcare delivery efficiency. However, this review highlights the need for continued improvement in the accuracy and personalization of AI-generated content. Future research should focus on developing more accurate LLM algorithms to enhance reliability as medical information sources, exploring multimodal LLMs, and establishing robust validation frameworks for their ethical use. Ensuring AI-based information aligns with the latest medical guidelines and is tailored for diverse patient populations is crucial. Conducting longitudinal studies to assess the long-term effects of LLMs on patient outcomes and satisfaction will provide valuable insights. Additionally, addressing ethical concerns, including data privacy and potential biases in LLM-generated content, is essential. These research directions are crucial for the responsible and effective integration of LLMs in healthcare settings. Finally, LLMs may carry biases from their training data, potentially propagating misinformation or reinforcing healthcare disparities. Future research should address these limitations by ensuring LLM tools are accurate, reliable, and equitable across diverse patient populations, while also exploring their long-term effects and ethical implications.
4.2 Limitations
This scoping review has several limitations. The quality of included studies varied, with some using small sample sizes or subjective assessments, potentially limiting result generalizability. Most studies were conducted in high-income countries, raising questions about their relevance to low-and middle-income settings with different healthcare needs and infrastructure. The evaluation of various LLMs and versions complicates drawing overarching conclusions. Inconsistent evaluation metrics across studies hindered result comparison and synthesis.
5 Conclusion
LLMs demonstrate transformative potential in patient education and engagement across various levels of medical care. Their ability to provide accurate, detailed, and timely information can significantly enhance patients’ understanding of their healthcare and promote active involvement. However, current limitations in accuracy and readability highlight the need for further refinement to ensure reliable integration with healthcare systems. Extensive research and development of AI tools are necessary to fully harness their potential for improving patient outcomes and healthcare efficiency. A critical priority for medical applications is to ensure the ethical and responsible use of these tools, necessitating robust supervision and validation processes.
Author contributions
SA: Conceptualization, Investigation, Methodology, Visualization, Writing – original draft, Writing – review & editing, Data curation. MK: Conceptualization, Investigation, Methodology, Visualization, Writing – original draft, Writing – review & editing, Project administration, Supervision. VV: Conceptualization, Data curation, Writing – original draft, Writing – review & editing. KM: Conceptualization, Investigation, Methodology, Project administration, Supervision, Validation, Writing – original draft, Writing – review & editing.
Funding
The author(s) declare that no financial support was received for the research, authorship, and/or publication of this article.
Conflict of interest
The authors declare that the research was conducted in the absence of any commercial or financial relationships that could be construed as a potential conflict of interest.
Publisher’s note
All claims expressed in this article are solely those of the authors and do not necessarily represent those of their affiliated organizations, or those of the publisher, the editors and the reviewers. Any product that may be evaluated in this article, or claim that may be made by its manufacturer, is not guaranteed or endorsed by the publisher.
Supplementary material
The Supplementary material for this article can be found online at: https://www.frontiersin.org/articles/10.3389/fmed.2024.1477898/full#supplementary-material
References
1. Willms, A, and Liu, S. Exploring the feasibility of using Chatgpt to create just-in-time adaptive physical activity mHealth intervention content: case study. JMIR Med Educ. (2024) 10:e51426. doi: 10.2196/51426
2. Park, YJ, Pillai, A, Deng, J, Guo, E, Gupta, M, Paget, M, et al. Assessing the research landscape and clinical utility of large language models: a scoping review. BMC Med Inform Decis Mak. (2024) 24:72. doi: 10.1186/s12911-024-02459-6
3. Meng, X, Yan, X, Zhang, K, Liu, D, Cui, X, Yang, Y, et al. The application of large language models in medicine: a scoping review. iScience. (2024) 27:109713. doi: 10.1016/j.isci.2024.109713
4. Minssen, T, Vayena, E, and Cohen, IG. The challenges for regulating medical use of Chatgpt and other large language models. JAMA. (2023) 330:315–6. doi: 10.1001/jama.2023.9651
5. Open AI. (2024). Available at: https://openai.com/index/hello-gpt-4o (accessed 2024).
6. Anthropic. (2024). Available at: https://www.anthropic.com/news/claude-3-5-sonnet (accessed 2024).
7. Google. (2023). Available at: https://gemini.google.com/ (accessed 2024).
8. Peng, W, Feng, Y, Yao, C, Zhang, S, Zhuo, H, Qiu, T, et al. Evaluating Ai in medicine: a comparative analysis of expert and Chatgpt responses to colorectal Cancer questions. Sci Rep. (2024) 14:2840. doi: 10.1038/s41598-024-52853-3
9. Sallam, M. Healthcare Chatgpt utility in healthcare education, research, and practice: systematic review on the promising perspectives and valid concerns. Healthcare (Basel). (2023) 11:887. doi: 10.3390/healthcare11060887
10. Preiksaitis, C, Ashenburg, N, Bunney, G, Chu, A, Kabeer, R, Riley, F, et al. The Role of large language models in transforming emergency medicine: scoping review. JMIR Med Inform. (2024) 12:e53787:e53787. doi: 10.2196/53787
11. Tricco, AC, Lillie, E, Zarin, W, O'Brien, KK, Colquhoun, H, Levac, D, et al. Prisma extension for scoping reviews (Prisma-Scr): checklist and explanation. Ann Intern Med. (2018) 169:467–73. doi: 10.7326/M18-0850
12. Arksey, H, and O'Malley, L. Scoping studies: towards a methodological framework. Int J Soc Res Methodol. (2005) 8:19–32. doi: 10.1080/1364557032000119616
13. Levac, D, Colquhoun, H, and O'Brien, KK. Scoping studies: advancing the methodology. Implement Sci. (2010) 5:1–9. doi: 10.1186/1748-5908-5-69/TABLES/3
14. Braun, V, and Clarke, V. Using thematic analysis in psychology. Qual Res Psychol. (2006) 3:77–101. doi: 10.1191/1478088706qp063oa
15. Al-Sharif, EM, Penteado, RC, Dib El Jalbout, N, Topilow, NJ, Shoji, MK, Kikkawa, DO, et al. Evaluating the accuracy of Chatgpt and Google Bard in fielding oculoplastic patient queries: a comparative study on artificial versus human intelligence. Ophthalmic. Plast Reconstr Surg. (2024) 40:303–11. doi: 10.1097/IOP.0000000000002567
16. Alapati, R, Campbell, D, Molin, N, Creighton, E, Wei, Z, Boon, M, et al. Evaluating insomnia queries from an artificial intelligence Chatbot for patient education. J Clin Sleep Med. (2024) 20:583–94. doi: 10.5664/jcsm.10948
17. Alessandri-Bonetti, M, Liu, HY, Palmesano, M, Nguyen, VT, and Egro, FM. Online patient education in body contouring: a comparison between Google and Chatgpt. J Plast Reconstr Aesthet Surg. (2023) 87:390–402. doi: 10.1016/j.bjps.2023.10.091
18. Almagazzachi, A, Mustafa, A, Eighaei Sedeh, A, Vazquez Gonzalez, AE, Polianovskaia, A, Abood, M, et al. Generative artificial intelligence in patient education: Chatgpt takes on hypertension questions. Cureus. (2024) 16:e53441. doi: 10.7759/cureus.53441
19. Amaral, JZ, Schultz, RJ, Martin, BM, Taylor, T, Touban, B, McGraw-Heinrich, J, et al. Evaluating chat generative pre-trained transformer responses to common pediatric in-toeing questions. J Pediatr Orthop. (2024) 44:e592–7. doi: 10.1097/BPO.0000000000002695
20. Amin, KS, Mayes, LC, Khosla, P, and Doshi, RH. Assessing the efficacy of large language models in health literacy: a comprehensive cross-sectional study. Yale J Biol Med. (2024) 97:17–27. doi: 10.59249/ZTOZ1966
21. Anastasio, AT, FBT, M, Karavan, MPJr, and Adams, SBJr. Evaluating the quality and usability of artificial intelligence-generated responses to common patient questions in foot and ankle surgery. Foot Ankle Orthop. (2023) 8:24730114231209919. doi: 10.1177/24730114231209919
22. Atarere, J, Naqvi, H, Haas, C, Adewunmi, C, Bandaru, S, Allamneni, R, et al. Applicability of online chat-based artificial intelligence models to colorectal Cancer screening. Dig Dis Sci. (2024) 69:791–7. doi: 10.1007/s10620-024-08274-3
23. Athavale, A, Baier, J, Ross, E, and Fukaya, E. The potential of Chatbots in chronic venous disease patient management. JVS Vasc Insights. (2023) 1:1. doi: 10.1016/j.jvsvi.2023.100019
24. Ayers, JW, Poliak, A, Dredze, M, Leas, EC, Zhu, Z, Kelley, JB, et al. Comparing physician and artificial intelligence Chatbot responses to patient questions posted to a public social media forum. JAMA Intern Med. (2023) 183:589–96. doi: 10.1001/jamainternmed.2023.1838
25. Ayoub, NF, Lee, YJ, Grimm, D, and Balakrishnan, K. Comparison between Chatgpt and Google search as sources of postoperative patient instructions. JAMA Otolaryngol Head Neck Surg. (2023) 149:556–8. doi: 10.1001/jamaoto.2023.0704
26. Ayoub, NF, Lee, YJ, Grimm, D, and Divi, V. Head-to-head comparison of Chatgpt versus Google search for medical knowledge acquisition. Otolaryngol Head Neck Surg. (2024) 170:1484–91. doi: 10.1002/ohn.465
27. Balel, Y. Can Chatgpt be used in Oral and maxillofacial surgery? J Stomatol Oral Maxillofac Surg. (2023) 124:101471. doi: 10.1016/j.jormas.2023.101471
28. Bellinger, JR, De La Chapa, JS, Kwak, MW, Ramos, GA, Morrison, D, and Kesser, BW. Bppv information on Google versus ai (Chatgpt). Otolaryngol Head Neck Surg. (2024) 170:1504–11. doi: 10.1002/ohn.506
29. Bernstein, IA, Zhang, YV, Govil, D, Majid, I, Chang, RT, Sun, Y, et al. Comparison of ophthalmologist and large language model Chatbot responses to online patient eye care questions. JAMA Netw Open. (2023) 6:e2330320. doi: 10.1001/jamanetworkopen.2023.30320
30. Brozović, J, Mikulić, B, Tomas, M, Juzbašić, M, and Blašković, M. Assessing the performance of Bing chat artificial intelligence: dental exams, clinical guidelines, and Patients' frequent questions. J Dent. (2024) 144:104927. doi: 10.1016/j.jdent.2024.104927
31. Caglar, U, Yildiz, O, Meric, A, Ayranci, A, Gelmis, M, Sarilar, O, et al. Evaluating the performance of Chatgpt in answering questions related to pediatric urology. J Pediatr Urol. (2024) 20:26.e1–5. doi: 10.1016/j.jpurol.2023.08.003
32. Campbell, DJ, Estephan, LE, Mastrolonardo, EV, Amin, DR, Huntley, CT, and Boon, MS. Evaluating Chatgpt responses on obstructive sleep apnea for patient education. J Clin Sleep Med. (2023) 19:1989–95. doi: 10.5664/jcsm.10728
33. Campbell, DJ, Estephan, LE, Sina, EM, Mastrolonardo, EV, Alapati, R, Amin, DR, et al. Evaluating Chatgpt responses on thyroid nodules for patient education. Thyroid. (2024) 34:371–7. doi: 10.1089/thy.2023.0491
34. Cappellani, F, Card, KR, Shields, CL, Pulido, JS, and Haller, JA. Reliability and accuracy of artificial intelligence Chatgpt in providing information on ophthalmic diseases and management to patients. Eye (Lond). (2024) 38:1368–73. doi: 10.1038/s41433-023-02906-0
35. Carnino, JM, Pellegrini, WR, Willis, M, Cohen, MB, Paz-Lansberg, M, Davis, EM, et al. Assessing Chatgpt's responses to otolaryngology patient questions. Ann Otol Rhinol Laryngol. (2024) 133:658–64. doi: 10.1177/00034894241249621
36. Chen, D, Parsa, R, Hope, A, Hannon, B, Mak, E, Eng, L, et al. Physician and artificial intelligence Chatbot responses to Cancer questions from social media. JAMA Oncol. (2024) 10:956–60. doi: 10.1001/jamaoncol.2024.0836
37. Chen, X, Zhang, W, Zhao, Z, Xu, P, Zheng, Y, Shi, D, et al. Icga-Gpt: report generation and question answering for Indocyanine green angiography images. Br J Ophthalmol. (2024) 108:1450–6. doi: 10.1136/bjo-2023-324446
38. Cheong, KX, Zhang, C, Tan, TE, Fenner, BJ, Wong, WM, Teo, KY, et al. Comparing generative and retrieval-based Chatbots in answering patient questions regarding age-related macular degeneration and diabetic retinopathy. Br J Ophthalmol. (2024) 108:1443–9. doi: 10.1136/bjo-2023-324533
39. Cheong, RCT, Unadkat, S, McNeillis, V, Williamson, A, Joseph, J, Randhawa, P, et al. Artificial intelligence Chatbots as sources of patient education material for obstructive sleep Apnoea: Chatgpt versus Google Bard. Eur Arch Otorrinolaringol. (2024) 281:985–93. doi: 10.1007/s00405-023-08319-9
40. Chervonski, E, Harish, KB, Rockman, CB, Sadek, M, Teter, KA, Jacobowitz, GR, et al. Generative artificial intelligence Chatbots may provide appropriate informational responses to common vascular surgery questions by patients. Vascular. (2024):17085381241240550. doi: 10.1177/17085381241240550
41. Christy, M, Morris, MT, Goldfarb, CA, and Dy, CJ. Appropriateness and reliability of an online artificial intelligence Platform's responses to common questions regarding distal radius fractures. J Hand Surg Am. (2024) 49:91–8. doi: 10.1016/j.jhsa.2023.10.019
42. Cohen, SA, Brant, A, Fisher, AC, Pershing, S, Do, D, and Pan, C. Dr. Google vs. Dr. Chatgpt: exploring the use of artificial intelligence in ophthalmology by comparing the accuracy, safety, and readability of responses to frequently asked patient questions regarding cataracts and cataract surgery. Semin Ophthalmol. (2024) 39:472–9. doi: 10.1080/08820538.2024.2326058
43. Connors, C, Gupta, K, Khusid, JA, Khargi, R, Yaghoubian, AJ, Levy, M, et al. Evaluation of the current status of artificial intelligence for Endourology patient education: a blind comparison of Chatgpt and Google Bard against traditional information resources. J Endourol. (2024) 38:843–51. doi: 10.1089/end.2023.0696
44. Cornelison, BR, Erstad, BL, and Edwards, C. Accuracy of a Chatbot in answering questions that patients should ask before taking a new medication. J Am Pharm Assoc. (2003) 64:102110. doi: 10.1016/j.japh.2024.102110
45. Croen, BJ, Abdullah, MS, Berns, E, Rapaport, S, Hahn, AK, Barrett, CC, et al. Evaluation of patient education materials from large-language artificial intelligence models on carpal tunnel release. Hand. (2024) N Y:15589447241247332. doi: 10.1177/15589447241247332
46. Crook, BS, Park, CN, Hurley, ET, Richard, MJ, and Pidgeon, TS. Evaluation of online artificial intelligence-generated information on common hand procedures. J Hand Surg Am. (2023) 48:1122–7. doi: 10.1016/j.jhsa.2023.08.003
47. Cung, M, Sosa, B, Yang, HS, McDonald, MM, Matthews, BG, Vlug, AG, et al. The performance of artificial intelligence Chatbot large language models to address skeletal biology and bone health queries. J Bone Miner Res. (2024) 39:106–15. doi: 10.1093/jbmr/zjad007
48. Davis, R, Eppler, M, Ayo-Ajibola, O, Loh-Doyle, JC, Nabhani, J, Samplaski, M, et al. Evaluating the effectiveness of artificial intelligence-powered large language models application in disseminating appropriate and readable health information in urology. J Urol. (2023) 210:688–94. doi: 10.1097/JU.0000000000003615
49. Dimitriadis, F, Alkagiet, S, Tsigkriki, L, Kleitsioti, P, Sidiropoulos, G, Efstratiou, D, et al. Chatgpt and patients with heart failure. Angiology. (2024):33197241238403. doi: 10.1177/00033197241238403
50. Doğan, L, Özçakmakcı, GB, and Yılmaz, ĬE. The performance of Chatbots and the Aapos website as a tool for amblyopia education. J Pediatr Ophthalmol Strabismus. (2024) 61:325–31. doi: 10.3928/01913913-20240409-01
51. Dubin, JA, Bains, SS, DeRogatis, MJ, Moore, MC, Hameed, D, Mont, MA, et al. Appropriateness of frequently asked patient questions following Total hip arthroplasty from Chatgpt compared to arthroplasty-trained nurses. J Arthroplast. (2024) 39:S306–11. doi: 10.1016/j.arth.2024.04.020
52. Durairaj, KK, Baker, O, Bertossi, D, Dayan, S, Karimi, K, Kim, R, et al. Artificial intelligence versus expert plastic surgeon: comparative study shows Chatgpt "wins" Rhinoplasty consultations: should we be worried? Facial Plast Surg Aesthet Med. (2023) 26:270–5. doi: 10.1089/fpsam.2023.0224
53. Fahy, S, Niemann, M, Böhm, P, Winkler, T, and Oehme, S. Assessment of the quality and readability of information provided by Chatgpt in relation to the use of platelet-rich plasma therapy for osteoarthritis. J Pers Med. (2024) 14:495. doi: 10.3390/jpm14050495
54. Fahy, S, Oehme, S, Milinkovic, D, Jung, T, and Bartek, B. Assessment of quality and readability of information provided by Chatgpt in relation to anterior cruciate ligament injury. J Pers Med. (2024) 14:104. doi: 10.3390/jpm14010104
55. Gabriel, J, Shafik, L, Alanbuki, A, and Larner, T. The utility of the Chatgpt artificial intelligence tool for patient education and enquiry in robotic radical prostatectomy. Int Urol Nephrol. (2023) 55:2717–32. doi: 10.1007/s11255-023-03729-4
56. Gajjar, AA, Kumar, RP, Paliwoda, ED, Kuo, CC, Adida, S, Legarreta, AD, et al. Usefulness and accuracy of artificial intelligence Chatbot responses to patient questions for neurosurgical procedures. Neurosurgery. (2024) 95:171–178. doi: 10.1227/neu.0000000000002856
57. Garcia Valencia, OA, Thongprayoon, C, Miao, J, Suppadungsuk, S, Krisanapan, P, Craici, IM, et al. Empowering inclusivity: improving readability of living kidney donation information with Chatgpt. Front Digit Health. (2024) 6:1366967. doi: 10.3389/fdgth.2024.1366967
58. Ghanem, D, Shu, H, Bergstein, V, Marrache, M, Love, A, Hughes, A, et al. Educating patients on osteoporosis and bone health: can "Chatgpt" provide high-quality content? Eur J Orthop Surg Traumatol. (2024) 34:2757–65. doi: 10.1007/s00590-024-03990-y
59. Ghanem, YK, Rouhi, AD, Al-Houssan, A, Saleh, Z, Moccia, MC, Joshi, H, et al. Dr. Google to Dr. Chatgpt: assessing the content and quality of artificial intelligence-generated medical information on appendicitis. Surg Endosc. (2024) 38:2887–93. doi: 10.1007/s00464-024-10739-5
60. Gordon, EB, Towbin, AJ, Wingrove, P, Shafique, U, Haas, B, Kitts, AB, et al. Enhancing patient communication with chat-Gpt in radiology: evaluating the efficacy and readability of answers to common imaging-related questions. J Am Coll Radiol. (2024) 21:353–9. doi: 10.1016/j.jacr.2023.09.011
61. Gül, Ş, Erdemir, İ, Hanci, V, Aydoğmuş, E, and Erkoç, YS. How artificial intelligence can provide information about subdural hematoma: assessment of readability, reliability, and quality of Chatgpt, Bard, and perplexity responses. Medicine (Baltimore). (2024) 103:e38009. doi: 10.1097/MD.0000000000038009
62. Günay, S, Yiğit, Y, Halhalli, HC, Tulgar, S, Alkahlout, BH, and Azad, AM. Ai in patient education: assessing the impact of Chatgpt-4 on conveying comprehensive information about chest pain. Am J Emerg Med. (2024) 77:220–1. doi: 10.1016/j.ajem.2023.12.047
63. Haidar, O, Jaques, A, McCaughran, PW, and Metcalfe, MJ. Ai-generated information for vascular patients: assessing the standard of procedure-specific information provided by the Chatgpt Ai-language model. Cureus. (2023) 15:e49764. doi: 10.7759/cureus.49764
64. Halawani, A, Mitchell, A, Saffarzadeh, M, Wong, V, Chew, BH, and Forbes, CM. Accuracy and readability of kidney stone patient information materials generated by a large language model compared to official urologic organizations. Urology. (2024) 186:107–13. doi: 10.1016/j.urology.2023.11.042
65. Hernandez, CA, Vazquez Gonzalez, AE, Polianovskaia, A, Amoro Sanchez, R, Muyolema Arce, V, Mustafa, A, et al. The future of patient education: Ai-driven guide for type 2 diabetes. Cureus. (2023) 15:e48919. doi: 10.7759/cureus.48919
66. Hershenhouse, JS, Mokhtar, D, Eppler, MB, Rodler, S, Storino Ramacciotti, L, Ganjavi, C, et al. Accuracy, readability, and understandability of large language models for prostate Cancer information to the public. Prostate Cancer Prostatic Dis. (2024). doi: 10.1038/s41391-024-00826-y
67. Hillmann, HAK, Angelini, E, Karfoul, N, Feickert, S, Mueller-Leisse, J, and Duncker, D. Accuracy and comprehensibility of chat-based artificial intelligence for patient information on atrial fibrillation and cardiac implantable electronic devices. Europace. (2023) 26:euad369. doi: 10.1093/europace/euad369
68. Hirpara, MM, Amin, L, Aloyan, T, Shilleh, N, and Lewis, P. Does the internet provide quality information on metoidioplasty? Using the modified ensuring quality information for patients tool to evaluate artificial intelligence-generated and online information on metoidioplasty. Ann Plast Surg. (2024) 92:S361–5. doi: 10.1097/SAP.0000000000003797
69. Høj, S, Thomsen, SF, Meteran, H, and Sigsgaard, T. Artificial intelligence and allergic rhinitis: does Chatgpt increase or impair the knowledge? J Public Health (Oxf). (2024) 46:123–6. doi: 10.1093/pubmed/fdad219
70. Hristidis, V, Ruggiano, N, Brown, EL, Ganta, SRR, and Stewart, S. Chatgpt vs Google for queries related to dementia and other cognitive decline: comparison of results. J Med Internet Res. (2023) 25:e48966. doi: 10.2196/48966
71. Ibrahim, MT, Khaskheli, SA, Shahzad, H, and Noordin, S. Language-adaptive artificial intelligence: assessing Chatgpt's answer to frequently asked questions on Total hip arthroplasty questions. J Pak Med Assoc. (2024) 74:S161–4. doi: 10.47391/JPMA.AKU-9S-25
72. Jazi, AHD, Mahjoubi, M, Shahabi, S, Alqahtani, AR, Haddad, A, Pazouki, A, et al. Bariatric evaluation through Ai: a survey of expert opinions versus Chatgpt-4 (Beta-Seov). Obes Surg. (2023) 33:3971–80. doi: 10.1007/s11695-023-06903-w
73. Johns, WL, Kellish, A, Farronato, D, Ciccotti, MG, and Hammoud, S. Chatgpt can offer satisfactory responses to common patient questions regarding elbow ulnar collateral ligament reconstruction. Arthrosc Sports Med Rehabil. (2024) 6:100893. doi: 10.1016/j.asmr.2024.100893
74. Johnson, CM, Bradley, CS, Kenne, KA, Rabice, S, Takacs, E, Vollstedt, A, et al. Evaluation of Chatgpt for pelvic floor surgery counseling. Urogynecology (Phila). (2024) 30:245–50. doi: 10.1097/SPV.0000000000001459
75. Juhi, A, Pipil, N, Santra, S, Mondal, S, Behera, JK, and Mondal, H. The capability of Chatgpt in predicting and explaining common drug-drug interactions. Cureus. (2023) 15:e36272. doi: 10.7759/cureus.36272
76. Kasthuri, VS, Glueck, J, Pham, H, Daher, M, Balmaceno-Criss, M, McDonald, CL, et al. Assessing the accuracy and reliability of Ai-generated responses to patient questions regarding spine surgery. J Bone Joint Surg Am. (2024) 106:1136–42. doi: 10.2106/JBJS.23.00914
77. Kim, MJ, Admane, S, Chang, YK, Shih, KK, Reddy, A, Tang, M, et al. Chatbot performance in defining and differentiating palliative care, supportive care, hospice care. J Pain Symptom Manage. (2024) 67:e381–91. doi: 10.1016/j.jpainsymman.2024.01.008
78. King, RC, Samaan, JS, Yeo, YH, Mody, B, Lombardo, DM, and Ghashghaei, R. Appropriateness of Chatgpt in answering heart failure related questions. Heart Lung Circ. (2024) 33:1314–8. doi: 10.1016/j.hlc.2024.03.005
79. King, RC, Samaan, JS, Yeo, YH, Peng, Y, Kunkel, DC, Habib, AA, et al. A multidisciplinary assessment of Chatgpt's knowledge of amyloidosis: observational study. JMIR Cardio. (2024) 8:e53421. doi: 10.2196/53421
80. Köroğlu, EY, Fakı, S, Beştepe, N, Tam, AA, Çuhacı Seyrek, N, Topaloglu, O, et al. A novel approach: evaluating Chatgpt's utility for the management of thyroid nodules. Cureus. (2023) 15:e47576. doi: 10.7759/cureus.47576
81. Kozaily, E, Geagea, M, Akdogan, ER, Atkins, J, Elshazly, MB, Guglin, M, et al. Accuracy and consistency of online large language model-based artificial intelligence chat platforms in answering Patients' questions about heart failure. Int J Cardiol. (2024) 408:132115. doi: 10.1016/j.ijcard.2024.132115
82. Kuckelman, IJ, Wetley, K, Yi, PH, and Ross, AB. Translating musculoskeletal radiology reports into patient-friendly summaries using Chatgpt-4. Skeletal Radiol. (2024) 53:1621–4. doi: 10.1007/s00256-024-04599-2
83. Kuckelman, IJ, Yi, PH, Bui, M, Onuh, I, Anderson, JA, and Ross, AB. Assessing Ai-powered patient education: a case study in radiology. Acad Radiol. (2024) 31:338–42. doi: 10.1016/j.acra.2023.08.020
84. Kuşcu, O, Pamuk, AE, Sütay Süslü, N, and Hosal, S. Is Chatgpt accurate and reliable in answering questions regarding head and neck Cancer? Front Oncol. (2023) 13:1256459. doi: 10.3389/fonc.2023.1256459
85. Lambert, R, Choo, ZY, Gradwohl, K, Schroedl, L, and Ruiz De Luzuriaga, A. Assessing the application of large language models in generating dermatologic patient education materials according to Reading level: qualitative study. JMIR Dermatol. (2024) 7:e55898. doi: 10.2196/55898
86. Lang, S, Vitale, J, Fekete, TF, Haschtmann, D, Reitmeir, R, Ropelato, M, et al. Are large language models valid tools for patient information on lumbar disc herniation? The spine surgeons' perspective. Brain Spine. (2024) 4:102804. doi: 10.1016/j.bas.2024.102804
87. Lechien, JR, Carroll, TL, Huston, MN, and Naunheim, MR. Chatgpt-4 accuracy for patient education in laryngopharyngeal reflux. Eur Arch Otorrinolaringol. (2024) 281:2547–52. doi: 10.1007/s00405-024-08560-w
88. Lee, TJ, Campbell, DJ, Patel, S, Hossain, A, Radfar, N, Siddiqui, E, et al. Unlocking health literacy: the ultimate guide to hypertension education from Chatgpt versus Google Gemini. Cureus. (2024) 16:e59898. doi: 10.7759/cureus.59898
89. Lee, TJ, Rao, AK, Campbell, DJ, Radfar, N, Dayal, M, and Khrais, A. Evaluating Chatgpt-3.5 and Chatgpt-4.0 responses on hyperlipidemia for patient education. Cureus. (2024) 16:e61067. doi: 10.7759/cureus.61067
90. Li, L, Li, P, Wang, K, Zhang, L, Zhao, H, and Ji, H. Benchmarking state-of-the-art large language models for migraine patient education: a comparison of performances on the responses to common queries. J Med Internet Res. (2024) 26:e55927. doi: 10.2196/55927
91. Li, W, Chen, J, Chen, F, Liang, J, and Yu, H. Exploring the potential of Chatgpt-4 in responding to common questions about Abdominoplasty: An Ai-based case study of a plastic surgery consultation. Aesth Plast Surg. (2024) 48:1571–83. doi: 10.1007/s00266-023-03660-0
92. Lim, B, Seth, I, Kah, S, Sofiadellis, F, Ross, RJ, Rozen, WM, et al. Using generative artificial intelligence tools in cosmetic surgery: a study on Rhinoplasty, facelifts, and blepharoplasty procedures. J Clin Med. (2023) 12:6524. doi: 10.3390/jcm12206524
93. Liu, HY, Alessandri Bonetti, M, De Lorenzi, F, Gimbel, ML, Nguyen, VT, and Egro, FM. Consulting the digital doctor: Google versus Chatgpt as sources of information on breast implant-associated anaplastic large cell lymphoma and breast implant illness. Aesth Plast Surg. (2024) 48:590–607. doi: 10.1007/s00266-023-03713-4
94. Liu, S, McCoy, AB, Wright, AP, Carew, B, Genkins, JZ, Huang, SS, et al. Leveraging large language models for generating responses to patient messages-a subjective analysis. J Am Med Inform Assoc. (2024) 31:1367–79. doi: 10.1093/jamia/ocae052
95. Lv, X, Zhang, X, Li, Y, Ding, X, Lai, H, and Shi, J. Leveraging large language models for improved patient access and self-management: Assessor-blinded comparison between expert-and Ai-generated content. J Med Internet Res. (2024) 26:e55847. doi: 10.2196/55847
96. Mashatian, S, Armstrong, DG, Ritter, A, Robbins, J, Aziz, S, Alenabi, I, et al. Building trustworthy generative artificial intelligence for diabetes care and limb preservation: a medical knowledge extraction case. J Diabetes Sci Technol. (2024):19322968241253568. doi: 10.1177/19322968241253568
97. Mastrokostas, PG, Mastrokostas, LE, Emara, AK, Wellington, IJ, Ginalis, E, Houten, JK, et al. Gpt-4 as a source of patient information for anterior cervical discectomy and fusion: a comparative analysis against Google web search. Global. Spine J. (2024):21925682241241241. doi: 10.1177/21925682241241241
98. McCarthy, CJ, Berkowitz, S, Ramalingam, V, and Ahmed, M. Evaluation of an artificial intelligence Chatbot for delivery of Ir patient education material: a comparison with societal website content. J Vasc Interv Radiol. (2023) 34:1760–8.e32. doi: 10.1016/j.jvir.2023.05.037
99. Mika, AP, Martin, JR, Engstrom, SM, Polkowski, GG, and Wilson, JM. Assessing Chatgpt responses to common patient questions regarding Total hip arthroplasty. J Bone Joint Surg Am. (2023) 105:1519–26. doi: 10.2106/JBJS.23.00209
100. Mika, AP, Mulvey, HE, Engstrom, SM, Polkowski, GG, Martin, JR, and Wilson, JM. Can Chatgpt answer patient questions regarding Total knee arthroplasty? J Knee Surg. (2024) 37:664–73. doi: 10.1055/s-0044-1782233
101. Mishra, V, Sarraju, A, Kalwani, NM, and Dexter, JP. Evaluation of prompts to simplify cardiovascular disease information generated using a large language model: cross-sectional study. J Med Internet Res. (2024) 26:e55388. doi: 10.2196/55388
102. Moazzam, Z, Lima, HA, Endo, Y, Noria, S, Needleman, B, and Pawlik, TM. A paradigm shift: online artificial intelligence platforms as an informational resource in bariatric surgery. Obes Surg. (2023) 33:2611–4. doi: 10.1007/s11695-023-06675-3
103. Moise, A, Centomo-Bozzo, A, Orishchak, O, Alnoury, MK, and Daniel, SJ. Can Chatgpt guide parents on Tympanostomy tube insertion? Children (Basel). (2023) 10:1634. doi: 10.3390/children10101634
104. Mondal, H, Mondal, S, and Podder, I. Using Chatgpt for writing articles for Patients' education for dermatological diseases: a pilot study. Indian Dermatol Online J. (2023) 14:482–6. doi: 10.4103/idoj.idoj_72_23
105. Mondal, H, Panigrahi, M, Mishra, B, Behera, JK, and Mondal, S. A pilot study on the capability of artificial intelligence in preparation of Patients' educational materials for Indian public health issues. J Family Med Prim Care. (2023) 12:1659–62. doi: 10.4103/jfmpc.jfmpc_262_23
106. Monroe, CL, Abdelhafez, YG, Atsina, K, Aman, E, Nardo, L, and Madani, MH. Evaluation of responses to cardiac imaging questions by the artificial intelligence large language model Chatgpt. Clin Imaging. (2024) 112:110193. doi: 10.1016/j.clinimag.2024.110193
107. Mootz, AA, Carvalho, B, Sultan, P, Nguyen, TP, and Reale, SC. The accuracy of Chatgpt-generated responses in answering commonly asked patient questions about labor epidurals: a survey-based study. Anesth Analg. (2024) 138:1142–4. doi: 10.1213/ANE.0000000000006801
108. Munir, MM, Endo, Y, Ejaz, A, Dillhoff, M, Cloyd, JM, and Pawlik, TM. Online artificial intelligence platforms and their applicability to gastrointestinal surgical operations. J Gastrointest Surg. (2024) 28:64–9. doi: 10.1016/j.gassur.2023.11.019
109. Musheyev, D, Pan, A, Loeb, S, and Kabarriti, AE. How well Do artificial intelligence Chatbots respond to the top search queries about urological malignancies? Eur Urol. (2024) 85:13–6. doi: 10.1016/j.eururo.2023.07.004
110. Nov, O, Singh, N, and Mann, D. Putting Chatgpt's medical advice to the (Turing) test: survey study. JMIR Med Educ. (2023) 9:e46939. doi: 10.2196/46939
111. O'Hagan, R, Kim, RH, Abittan, BJ, Caldas, S, Ungar, J, and Ungar, B. Trends in accuracy and appropriateness of alopecia Areata information obtained from a popular online large language model, Chatgpt. Dermatology. (2023) 239:952–7. doi: 10.1159/000534005
112. Pan, A, Musheyev, D, Bockelman, D, Loeb, S, and Kabarriti, AE. Assessment of artificial intelligence Chatbot responses to top searched queries about Cancer. JAMA Oncol. (2023) 9:1437–40. doi: 10.1001/jamaoncol.2023.2947
113. Parekh, AS, McCahon, JAS, Nghe, A, Pedowitz, DI, Daniel, JN, and Parekh, SG. Foot and ankle patient education materials and artificial intelligence Chatbots: a comparative analysis. Foot Ankle Spec. (2024):19386400241235834. doi: 10.1177/19386400241235834
114. Pohl, NB, Derector, E, Rivlin, M, Bachoura, A, Tosti, R, Kachooei, AR, et al. A quality and readability comparison of artificial intelligence and popular health website education materials for common hand surgery procedures. Hand Surg Rehabil. (2024) 43:101723. doi: 10.1016/j.hansur.2024.101723
115. Potapenko, I, Malmqvist, L, Subhi, Y, and Hamann, S. Artificial intelligence-based Chatgpt responses for patient questions on optic disc Drusen. Ophthalmol Ther. (2023) 12:3109–19. doi: 10.1007/s40123-023-00800-2
116. Pradhan, F, Fiedler, A, Samson, K, Olivera-Martinez, M, Manatsathit, W, and Peeraphatdit, T. Artificial intelligence compared with human-derived patient educational materials on cirrhosis. Hepatol Commun. (2024) 8:e0367. doi: 10.1097/HC9.0000000000000367
117. Rahimli Ocakoglu, S, and Coskun, B. The emerging role of Ai in patient education: a comparative analysis of Llm accuracy for pelvic organ prolapse. Med Princ Pract. (2024) 33:330–7. doi: 10.1159/000538538
118. Razdan, S, Siegal, AR, Brewer, Y, Sljivich, M, and Valenzuela, RJ. Assessing Chatgpt's ability to answer questions pertaining to erectile dysfunction: can our patients trust it? Int J Impot Res. (2023). doi: 10.1038/s41443-023-00797-z
119. Reichenpfader, D, Rösslhuemer, P, and Denecke, K. Large language model-based evaluation of medical question answering systems: algorithm development and case study. Stud Health Technol Inform. (2024) 313:22–7. doi: 10.3233/SHTI240006
120. Roster, K, Kann, RB, Farabi, B, Gronbeck, C, Brownstone, N, and Lipner, SR. Readability and health literacy scores for Chatgpt-generated dermatology public education materials: cross-sectional analysis of sunscreen and melanoma questions. JMIR Dermatol. (2024) 7:e50163. doi: 10.2196/50163
121. Samaan, JS, Yeo, YH, Rajeev, N, Hawley, L, Abel, S, Ng, WH, et al. Assessing the accuracy of responses by the language model Chatgpt to questions regarding bariatric surgery. Obes Surg. (2023) 33:1790–6. doi: 10.1007/s11695-023-06603-5
122. Şan, H, Bayrakçi, Ö, Çağdaş, B, Serdengeçti, M, and Alagöz, E. Reliability and readability analysis of Gpt-4 and Google Bard as a patient information source for the Most commonly applied radionuclide treatments in Cancer patients. Rev Esp Med Nucl Imagen Mol (Engl Ed). (2024):500021. doi: 10.1016/j.remnie.2024.500021
123. Sciberras, M, Farrugia, Y, Gordon, H, Furfaro, F, Allocca, M, Torres, J, et al. Accuracy of information given by Chatgpt for patients with inflammatory bowel disease in relation to Ecco guidelines. J Crohns Colitis. (2024) 18:1215–21. doi: 10.1093/ecco-jcc/jjae040
124. Şenoymak, MC, Erbatur, NH, Şenoymak, İ, and Fırat, SN. The role of artificial intelligence in endocrine management: assessing Chatgpt's responses to Prolactinoma queries. J Pers Med. (2024) 14:330. doi: 10.3390/jpm14040330
125. Seth, I, Cox, A, Xie, Y, Bulloch, G, Hunter-Smith, DJ, Rozen, WM, et al. Evaluating Chatbot efficacy for answering frequently asked questions in plastic surgery: a Chatgpt case study focused on breast augmentation. Aesthet Surg J. (2023) 43:1126–35. doi: 10.1093/asj/sjad140
126. Shah, YB, Ghosh, A, Hochberg, AR, Rapoport, E, Lallas, CD, Shah, MS, et al. Comparison of Chatgpt and traditional patient education materials for Men's health. Urol Pract. (2024) 11:87–94. doi: 10.1097/UPJ.0000000000000490
127. Shen, SA, Perez-Heydrich, CA, Xie, DX, and Nellis, JC. Chatgpt vs. web search for patient questions: what does Chatgpt Do better? Eur Arch Otorrinolaringol. (2024) 281:3219–25. doi: 10.1007/s00405-024-08524-0
128. Shiraishi, M, Lee, H, Kanayama, K, Moriwaki, Y, and Okazaki, M. Appropriateness of artificial intelligence Chatbots in diabetic foot ulcer management. Int J Low Extrem Wounds. (2024):15347346241236811. doi: 10.1177/15347346241236811
129. Song, H, Xia, Y, Luo, Z, Liu, H, Song, Y, Zeng, X, et al. Evaluating the performance of different large language models on health consultation and patient education in Urolithiasis. J Med Syst. (2023) 47:125. doi: 10.1007/s10916-023-02021-3
130. Spallek, S, Birrell, L, Kershaw, S, Devine, EK, and Thornton, L. Can we use Chatgpt for mental health and substance use education? Examining its quality and potential harms. JMIR Med Educ. (2023) 9:e51243. doi: 10.2196/51243
131. Srinivasan, N, Samaan, JS, Rajeev, ND, Kanu, MU, Yeo, YH, and Samakar, K. Large language models and bariatric surgery patient education: a comparative readability analysis of Gpt-3.5, Gpt-4, Bard, and online institutional resources. Surg Endosc. (2024) 38:2522–32. doi: 10.1007/s00464-024-10720-2
132. Subramanian, T, Araghi, K, Amen, TB, Kaidi, A, Sosa, B, Shahi, P, et al. Chat generative Pretraining transformer answers patient-focused questions in cervical spine surgery. Clin Spine Surg. (2024) 37:E278–81. doi: 10.1097/BSD.0000000000001600
133. Tailor, PD, Dalvin, LA, Chen, JJ, Iezzi, R, Olsen, TW, Scruggs, BA, et al. A comparative study of responses to retina questions from either experts, expert-edited large language models, or expert-edited large language models alone. Ophthalmol Sci. (2024) 4:100485. doi: 10.1016/j.xops.2024.100485
134. Tailor, PD, Xu, TT, Fortes, BH, Iezzi, R, Olsen, TW, Starr, MR, et al. Appropriateness of ophthalmology recommendations from an online chat-based artificial intelligence model. Mayo Clin Proc Digit Health. (2024) 2:119–28. doi: 10.1016/j.mcpdig.2024.01.003
135. Tao, BK, Handzic, A, Hua, NJ, Vosoughi, AR, Margolin, EA, and Micieli, JA. Utility of Chatgpt for automated creation of patient education handouts: An application in neuro-ophthalmology. J Neuroophthalmol. (2024) 44:119–24. doi: 10.1097/WNO.0000000000002074
136. WLT, T, Cheng, R, Weinblatt, AI, Bergstein, V, and Long, WJ. An artificial intelligence Chatbot is an accurate and useful online patient resource prior to Total knee arthroplasty. J Arthroplast. (2024) 39:S358–62. doi: 10.1016/j.arth.2024.02.005
137. Tepe, M, and Emekli, E. Assessing the responses of large language models (Chatgpt-4, Gemini, and Microsoft Copilot) to frequently asked questions in breast imaging: a study on readability and accuracy. Cureus. (2024) 16:e59960. doi: 10.7759/cureus.59960
138. Tharakan, S, Klein, B, Bartlett, L, Atlas, A, Parada, SA, and Cohn, RM. Do Chatgpt and Google differ in answers to commonly asked patient questions regarding Total shoulder and Total elbow arthroplasty? J Shoulder Elb Surg. (2024) 33:e429–37. doi: 10.1016/j.jse.2023.11.014
139. Thia, I, and Saluja, M. Chatgpt: is this patient education tool for urological malignancies readable for the general population? Res Rep Urol. (2024) 16:31–7. doi: 10.2147/RRU.S440633
140. Van Bulck, L, and Moons, P. What if your patient switches from Dr. Google to Dr. Chatgpt? A vignette-based survey of the trustworthiness, value, and danger of Chatgpt-generated responses to health questions. Eur J Cardiovasc Nurs. (2024) 23:95–8. doi: 10.1093/eurjcn/zvad038
141. Washington, CJ, Abouyared, M, Karanth, S, Braithwaite, D, Birkeland, A, Silverman, DA, et al. The use of Chatbots in head and neck mucosal malignancy treatment recommendations. Otolaryngol Head Neck Surg. (2024) 171:1062–8. doi: 10.1002/ohn.818
142. Wei, K, Fritz, C, and Rajasekaran, K. Answering head and neck Cancer questions: An assessment of Chatgpt responses. Am J Otolaryngol. (2024) 45:104085. doi: 10.1016/j.amjoto.2023.104085
143. Wrenn, SP, Mika, AP, Ponce, RB, and Mitchell, PM. Evaluating Chatgpt's ability to answer common patient questions regarding hip fracture. J Am Acad Orthop Surg. (2024) 32:656–9. doi: 10.5435/JAAOS-D-23-00877
144. Wright, BM, Bodnar, MS, Moore, AD, Maseda, MC, Kucharik, MP, Diaz, CC, et al. Is Chatgpt a trusted source of information for Total hip and knee arthroplasty patients? Bone Jt Open. (2024) 5:139–46. doi: 10.1302/2633-1462.52.BJO-2023-0113.R1
145. Wu, G, Zhao, W, Wong, A, and Lee, DA. Patients with floaters: answers from virtual assistants and large language models. Digit Health. (2024) 10:20552076241229933. doi: 10.1177/20552076241229933
146. Wu, Y, Zhang, Z, Dong, X, Hong, S, Hu, Y, Liang, P, et al. Evaluating the performance of the language model Chatgpt in responding to common questions of people with epilepsy. Epilepsy Behav. (2024) 151:109645. doi: 10.1016/j.yebeh.2024.109645
147. Yalla, GR, Hyman, N, Hock, LE, Zhang, Q, Shukla, AG, and Kolomeyer, NN. Performance of artificial intelligence Chatbots on Glaucoma questions adapted from patient brochures. Cureus. (2024) 16:e56766. doi: 10.7759/cureus.56766
148. Yan, S, Du, D, Liu, X, Dai, Y, Kim, MK, Zhou, X, et al. Assessment of the reliability and clinical applicability of Chatgpt's responses to Patients' common queries about Rosacea. Patient Prefer Adherence. (2024) 18:249–53. doi: 10.2147/PPA.S444928
149. Yan, SY, Liu, YF, Ma, L, Xiao, LL, Hu, X, Guo, R, et al. Walking forward or on hold: could the Chatgpt be applied for seeking health information in neurosurgical settings? Ibrain. (2024) 10:111–5. doi: 10.1002/ibra.12149
150. Ye, C, Zweck, E, Ma, Z, Smith, J, and Katz, S. Doctor versus artificial intelligence: patient and physician evaluation of large language model responses to rheumatology patient questions in a cross-sectional study. Arthritis Rheumatol. (2024) 76:479–84. doi: 10.1002/art.42737
151. Yeo, YH, Samaan, JS, Ng, WH, Ting, PS, Trivedi, H, Vipani, A, et al. Assessing the performance of Chatgpt in answering questions regarding cirrhosis and hepatocellular carcinoma. Clin Mol Hepatol. (2023) 29:721–32. doi: 10.3350/cmh.2023.0089
152. Yılmaz, IBE, and Doğan, L. Talking technology: exploring Chatbots as a tool for cataract patient education. Clin Exp Optom. (2024):1–9. doi: 10.1080/08164622.2023.2298812
153. Yüce, A, Erkurt, N, Yerli, M, and Misir, A. The potential of Chatgpt for high-quality information in patient education for sports surgery. Cureus. (2024) 16:e58874. doi: 10.7759/cureus.58874
154. Yun, JY, Kim, DJ, Lee, N, and Kim, EK. A comprehensive evaluation of Chatgpt consultation quality for augmentation mammoplasty: a comparative analysis between plastic surgeons and laypersons. Int J Med Inform. (2023) 179:105219. doi: 10.1016/j.ijmedinf.2023.105219
155. Zalzal, HG, Abraham, A, Cheng, J, and Shah, RK. Can ChatGPT help patients answer their otolaryngology questions? Laryngoscope Investig Otolaryngol. (2024) 9:e1193. doi: 10.1002/lio2.1193
156. Zhang, S, Liau, ZQG, Tan, KLM, and Chua, WL. Evaluating the accuracy and relevance of Chatgpt responses to frequently asked questions regarding total knee replacement. Knee Surg Relat Res. (2024) 36:15. doi: 10.1186/s43019-024-00218-5
157. Zhang, Y, Dong, Y, Mei, Z, Hou, Y, Wei, M, Yeung, YH, et al. Performance of large language models on benign prostatic hyperplasia frequently asked questions. Prostate. (2024) 84:807–13. doi: 10.1002/pros.24699
158. Abreu, AA, Murimwa, GZ, Farah, E, Stewart, JW, Zhang, L, Rodriguez, J, et al. Enhancing readability of online patient-facing content: the role of Ai Chatbots in improving Cancer information accessibility. J Natl Compr Cancer Netw. (2024) 22:e237334. doi: 10.6004/jnccn.2023.7334
159. Ayre, J, Mac, O, McCaffery, K, McKay, BR, Liu, M, Shi, Y, et al. New Frontiers in health literacy: using Chatgpt to simplify health information for people in the community. J Gen Intern Med. (2024) 39:573–7. doi: 10.1007/s11606-023-08469-w
160. Baldwin, AJ. An artificial intelligence language model improves readability of burns first aid information. Burns. (2024) 50:1122–7. doi: 10.1016/j.burns.2024.03.005
161. Browne, R, Gull, K, Hurley, CM, Sugrue, RM, and O'Sullivan, JB. Chatgpt-4 can help hand surgeons communicate better with patients. J Hand Surg Glob Online. (2024) 6:436–8. doi: 10.1016/j.jhsg.2024.03.008
162. Covington, EW, Watts Alexander, CS, Sewell, J, Hutchison, AM, Kay, J, Tocco, L, et al. Unlocking the future of patient education: Chatgpt vs. Lexicomp® as sources of patient education materials. J Am Pharm Assoc (2003). (2024):102119. doi: 10.1016/j.japh.2024.102119
163. Dihan, Q, Chauhan, MZ, Eleiwa, TK, Hassan, AK, Sallam, AB, Khouri, AS, et al. Using large language models to generate educational materials on childhood Glaucoma. Am J Ophthalmol. (2024) 265:28–38. doi: 10.1016/j.ajo.2024.04.004
164. Eid, K, Eid, A, Wang, D, Raiker, RS, Chen, S, and Nguyen, J. Optimizing ophthalmology patient education via Chatbot-generated materials: readability analysis of Ai-generated patient education materials and the American Society of Ophthalmic Plastic and Reconstructive Surgery Patient Brochures. Ophthalmic Plast Reconstr Surg. (2024) 40:212–6. doi: 10.1097/IOP.0000000000002549
165. Eppler, MB, Ganjavi, C, Knudsen, JE, Davis, RJ, Ayo-Ajibola, O, Desai, A, et al. Bridging the gap between urological research and patient understanding: the role of large language models in automated generation of Layperson's summaries. Urol Pract. (2023) 10:436–43. doi: 10.1097/UPJ.0000000000000428
166. Fanning, JE, Escobar-Domingo, MJ, Foppiani, J, Lee, D, Miller, AS, Janis, JE, et al. Improving readability and automating content analysis of plastic surgery webpages with Chatgpt. J Surg Res. (2024) 299:103–11. doi: 10.1016/j.jss.2024.04.006
167. Hung, YC, Chaker, SC, Sigel, M, Saad, M, and Slater, ED. Comparison of patient education materials generated by chat generative pre-trained transformer versus experts: An innovative way to increase readability of patient education materials. Ann Plast Surg. (2023) 91:409–12. doi: 10.1097/SAP.0000000000003634
168. Kirchner, GJ, Kim, RY, Weddle, JB, and Bible, JE. Can artificial intelligence improve the readability of patient education materials? Clin Orthop Relat Res. (2023) 481:2260–7. doi: 10.1097/CORR.0000000000002668
169. Moons, P, and Van Bulck, L. Using Chatgpt and Google Bard to improve the readability of written patient information: a proof of concept. Eur J Cardiovasc Nurs. (2024) 23:122–6. doi: 10.1093/eurjcn/zvad087
170. Patel, EA, Fleischer, L, Filip, P, Eggerstedt, M, Hutz, M, Michaelides, E, et al. The use of artificial intelligence to improve readability of otolaryngology patient education materials. Otolaryngol Head Neck Surg. (2024) 171:603–8. doi: 10.1002/ohn.816
171. Rouhi, AD, Ghanem, YK, Yolchieva, L, Saleh, Z, Joshi, H, Moccia, MC, et al. Can artificial intelligence improve the readability of patient education materials on aortic stenosis? A pilot study. Cardiol Ther. (2024) 13:137–47. doi: 10.1007/s40119-023-00347-0
172. Sridharan, K, and Sivaramakrishnan, G. Enhancing readability of Usfda patient communications through large language models: a proof-of-concept study. Expert Rev Clin Pharmacol. (2024) 17:731–41. doi: 10.1080/17512433.2024.2363840
173. Sudharshan, R, Shen, A, Gupta, S, and Zhang-Nunes, S. Assessing the utility of Chatgpt in simplifying text complexity of patient educational materials. Cureus. (2024) 16:e55304. doi: 10.7759/cureus.55304
174. Vallurupalli, M, Shah, ND, and Vyas, RM. Validation of Chatgpt 3.5 as a tool to optimize readability of patient-facing craniofacial education materials. Plast Reconstr Surg Glob Open. (2024) 12:e5575. doi: 10.1097/GOX.0000000000005575
175. Grimm, DR, Lee, YJ, Hu, K, Liu, L, Garcia, O, Balakrishnan, K, et al. The utility of Chatgpt as a generative medical translator. Eur Arch Otorrinolaringol. (2024). doi: 10.1007/s00405-024-08708-8
176. He, Z, Bhasuran, B, Jin, Q, Tian, S, Hanna, K, Shavor, C, et al. Quality of answers of generative large language models versus peer users for interpreting laboratory test results for lay patients: evaluation study. J Med Internet Res. (2024) 26:e56655. doi: 10.2196/56655
177. Meyer, A, Soleman, A, Riese, J, and Streichert, T. Comparison of Chatgpt, Gemini, and Le chat with physician interpretations of medical laboratory questions from an online health forum. Clin Chem Lab Med. (2024) 62:2425–34. doi: 10.1515/cclm-2024-0246
178. Lyu, Q, Tan, J, Zapadka, ME, Ponnatapura, J, Niu, C, Myers, KJ, et al. Translating radiology reports into plain language using Chatgpt and Gpt-4 with prompt learning: results, limitations, and potential. Vis Comput Ind Biomed Art. (2023) 6:9. doi: 10.1186/s42492-023-00136-5
179. Sarangi, PK, Lumbani, A, Swarup, MS, Panda, S, Sahoo, SS, Hui, P, et al. Assessing Chatgpt's proficiency in simplifying radiological reports for healthcare professionals and patients. Cureus. (2023) 15:e50881. doi: 10.7759/cureus.50881
180. Rogasch, JMM, Metzger, G, Preisler, M, Galler, M, Thiele, F, Brenner, W, et al. Chatgpt: can You prepare my patients for [(18) F] Fdg pet/Ct and explain my reports? J Nucl Med. (2023) 64:1876–9. doi: 10.2967/jnumed.123.266114
181. Tepe, M, and Emekli, E. Decoding medical jargon: the use of Ai language models (Chatgpt-4, Bard, Microsoft Copilot) in radiology reports. Patient Educ Couns. (2024) 126:108307. doi: 10.1016/j.pec.2024.108307
182. Woo, KC, Simon, GW, Akindutire, O, Aphinyanaphongs, Y, Austrian, JS, Kim, JG, et al. Evaluation of Gpt-4 ability to identify and generate patient instructions for actionable incidental radiology findings. J Am Med Inform Assoc. (2024) 31:1983–93. doi: 10.1093/jamia/ocae117
183. Zaretsky, J, Kim, JM, Baskharoun, S, Zhao, Y, Austrian, J, Aphinyanaphongs, Y, et al. Generative artificial intelligence to transform inpatient discharge summaries to patient-friendly language and format. JAMA Netw Open. (2024) 7:e240357. doi: 10.1001/jamanetworkopen.2024.0357
184. Alanezi, F. Examining the role of Chatgpt in promoting health behaviors and lifestyle changes among Cancer patients. Nutr Health. (2024):2601060241244563. doi: 10.1177/02601060241244563
185. Bragazzi, NL, and Garbarino, S. Assessing the accuracy of generative conversational artificial intelligence in debunking sleep health myths: mixed methods comparative study with expert analysis. JMIR Form Res. (2024) 8:e55762. doi: 10.2196/55762
186. Garbarino, S, and Bragazzi, NL. Evaluating the effectiveness of artificial intelligence-based tools in detecting and understanding sleep health misinformation: comparative analysis using Google Bard and Openai Chatgpt-4. J Sleep Res. (2024):e14210. doi: 10.1111/jsr.14210
187. Gray, M, Baird, A, Sawyer, T, James, J, DeBroux, T, Bartlett, M, et al. Increasing realism and variety of virtual patient dialogues for prenatal counseling education through a novel application of Chatgpt: exploratory observational study. JMIR Med Educ. (2024) 10:e50705. doi: 10.2196/50705
188. Minutolo, A, Damiano, E, De Pietro, G, Fujita, H, and Esposito, M. A conversational agent for querying Italian patient information leaflets and improving health literacy. Comput Biol Med. (2022) 141:105004. doi: 10.1016/j.compbiomed.2021.105004
189. Mondal, H, Dash, I, Mondal, S, and Behera, JK. Chatgpt in answering queries related to lifestyle-related diseases and disorders. Cureus. (2023) 15:e48296. doi: 10.7759/cureus.48296
190. Ponzo, V, Goitre, I, Favaro, E, Merlo, FD, Mancino, MV, Riso, S, et al. Is Chatgpt an effective tool for providing dietary advice? Nutrients. (2024) 16:469. doi: 10.3390/nu16040469
191. Zaleski, AL, Berkowsky, R, Craig, KJT, and Pescatello, LS. Comprehensiveness, accuracy, and readability of exercise recommendations provided by an Ai-based Chatbot: mixed methods study. JMIR Med Educ. (2024) 10:e51308. doi: 10.2196/51308
192. Altamimi, I, Altamimi, A, Alhumimidi, AS, and Temsah, MH. Snakebite advice and counseling from artificial intelligence: An acute venomous snakebite consultation with Chatgpt. Cureus. (2023) 15:e40351. doi: 10.7759/cureus.40351
193. McMahon, HV, and McMahon, BD. Automating untruths: Chatgpt, self-managed medication abortion, and the threat of misinformation in a post-roe world. Front Digit Health. (2024) 6:1287186. doi: 10.3389/fdgth.2024.1287186
194. Aliyeva, A, Sari, E, Alaskarov, E, and Nasirov, R. Enhancing postoperative Cochlear implant care with Chatgpt-4: a study on artificial intelligence (Ai)-assisted patient education and support. Cureus. (2024) 16:e53897. doi: 10.7759/cureus.53897
195. Scheschenja, M, Viniol, S, Bastian, MB, Wessendorf, J, König, AM, and Mahnken, AH. Feasibility of Gpt-3 and Gpt-4 for in-depth patient education prior to interventional radiological procedures: a comparative analysis. Cardiovasc Intervent Radiol. (2024) 47:245–50. doi: 10.1007/s00270-023-03563-2
196. Bains, SS, Dubin, JA, Hameed, D, Sax, OC, Douglas, S, Mont, MA, et al. Use and application of large language models for patient questions following Total knee arthroplasty. J Arthroplast. (2024) 39:2289–94. doi: 10.1016/j.arth.2024.03.017
197. Borna, S, Gomez-Cabello, CA, Pressman, SM, Haider, SA, Sehgal, A, Leibovich, BC, et al. Comparative analysis of artificial intelligence virtual assistant and large language models in post-operative care. Eur J Investig Health Psychol Educ. (2024) 14:1413–24. doi: 10.3390/ejihpe14050093
198. Capelleras, M, Soto-Galindo, GA, Cruellas, M, and Apaydin, F. Chatgpt and Rhinoplasty recovery: An exploration of Ai's role in postoperative guidance. Facial Plast Surg. (2024) 40:628–31. doi: 10.1055/a-2219-4901
199. Chaker, SC, Hung, YC, Saad, M, Golinko, MS, and Galdyn, IA. Easing the burden on caregivers-applications of artificial intelligence for physicians and caregivers of children with cleft lip and palate. Cleft Palate Craniofac J. (2024):10556656231223596. doi: 10.1177/10556656231223596
200. Shao, CY, Li, H, Liu, XL, Li, C, Yang, LQ, Zhang, YJ, et al. Appropriateness and comprehensiveness of using Chatgpt for perioperative patient education in thoracic surgery in different language contexts: survey study. Interact J Med Res. (2023) 12:e46900. doi: 10.2196/46900
201. Lee, JC, Hamill, CS, Shnayder, Y, Buczek, E, Kakarala, K, and Bur, AM. Exploring the role of artificial intelligence Chatbots in preoperative counseling for head and neck Cancer surgery. Laryngoscope. (2024) 134:2757–61. doi: 10.1002/lary.31243
202. Nanji, K, Yu, CW, Wong, TY, Sivaprasad, S, Steel, DH, Wykoff, CC, et al. Evaluation of postoperative ophthalmology patient instructions from Chatgpt and Google search. Can J Ophthalmol. (2024) 59:e69–71. doi: 10.1016/j.jcjo.2023.10.001
203. Dhar, S, Kothari, D, Vasquez, M, Clarke, T, Maroda, A, McClain, WG, et al. The utility and accuracy of Chatgpt in providing post-operative instructions following tonsillectomy: a pilot study. Int J Pediatr Otorhinolaryngol. (2024) 179:111901. doi: 10.1016/j.ijporl.2024.111901
204. Patil, NS, Huang, R, Mihalache, A, Kisilevsky, E, Kwok, J, Popovic, MM, et al. The ability of artificial intelligence Chatbots Chatgpt and Google Bard to accurately convey preoperative information for patients undergoing ophthalmic surgeries. Retina. (2024) 44:950–3. doi: 10.1097/IAE.0000000000004044
205. Meyer, R, Hamilton, KM, Truong, MD, Wright, KN, Siedhoff, MT, Brezinov, Y, et al. Chatgpt compared with Google search and healthcare institution as sources of postoperative patient instructions after gynecological surgery. BJOG. (2024) 131:1154–6. doi: 10.1111/1471-0528.17746
206. Breneman, A, Gordon, ER, Trager, MH, Ensslin, CJ, Fisher, J, Humphreys, TR, et al. Evaluation of large language model responses to Mohs surgery preoperative questions. Arch Dermatol Res. (2024) 316:227. doi: 10.1007/s00403-024-02956-8
207. Kienzle, A, Niemann, M, Meller, S, and Gwinner, C. Chatgpt may offer an adequate substitute for informed consent to patients prior to Total knee arthroplasty-yet caution is needed. J Pers Med. (2024) 14:69. doi: 10.3390/jpm14010069
208. Ali, R, Connolly, ID, Tang, OY, Mirza, FN, Johnston, B, Abdulrazeq, HF, et al. Bridging the literacy gap for surgical consents: An Ai-human expert collaborative approach. NPJ Digit Med. (2024) 7:63. doi: 10.1038/s41746-024-01039-2
209. Shiraishi, M, Tomioka, Y, Miyakuni, A, Moriwaki, Y, Yang, R, Oba, J, et al. Generating informed consent documents related to blepharoplasty using Chatgpt. Ophthalmic Plast Reconstr Surg. (2024) 40:316–20. doi: 10.1097/IOP.0000000000002574
210. An, Y, Fang, Q, and Wang, L. Enhancing patient education in Cancer care: intelligent Cancer patient education model for effective communication. Comput Biol Med. (2024) 169:107874. doi: 10.1016/j.compbiomed.2023.107874
211. Roberts, RHR, Ali, SR, Dobbs, TD, and Whitaker, IS. Can large language models generate outpatient clinic letters at first consultation that incorporate complication profiles from Uk and USA aesthetic plastic surgery associations? Aesthet Surg J Open Forum. (2024) 6:ojad 109. doi: 10.1093/asjof/ojad109
Keywords: large language models, ChatGPT, patient education, artificial intelligence, machine learning, deep learning
Citation: Aydin S, Karabacak M, Vlachos V and Margetis K (2024) Large language models in patient education: a scoping review of applications in medicine. Front. Med. 11:1477898. doi: 10.3389/fmed.2024.1477898
Edited by:
Rafat Damseh, United Arab Emirates University, United Arab EmiratesReviewed by:
Johanna Mora, Bristol Myers Squibb, United StatesBeenish Chaudhry, University of Louisiana at Lafayette, United States
Copyright © 2024 Aydin, Karabacak, Vlachos and Margetis. This is an open-access article distributed under the terms of the Creative Commons Attribution License (CC BY). The use, distribution or reproduction in other forums is permitted, provided the original author(s) and the copyright owner(s) are credited and that the original publication in this journal is cited, in accordance with accepted academic practice. No use, distribution or reproduction is permitted which does not comply with these terms.
*Correspondence: Konstantinos Margetis, S29uc3RhbnRpbm9zLk1hcmdldGlzQG1vdW50c2luYWkub3Jn