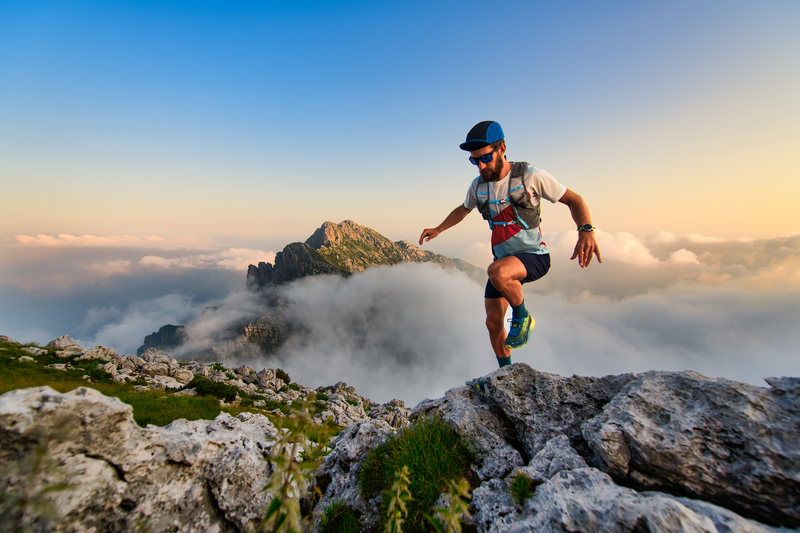
94% of researchers rate our articles as excellent or good
Learn more about the work of our research integrity team to safeguard the quality of each article we publish.
Find out more
ORIGINAL RESEARCH article
Front. Med. , 18 December 2024
Sec. Rheumatology
Volume 11 - 2024 | https://doi.org/10.3389/fmed.2024.1431642
This article is part of the Research Topic Understanding Scleroderma: Symptoms, Causes, Treatment Options, and Advanced Diagnostic Techniques View all 8 articles
The risk of lung cancer is significantly increased in patients with systemic sclerosis (SSc), yet the specific genes underlying this association remain unexplored. Our study aims to identify genes shared by SSc and lung cancer. We identified differentially expressed genes (DEGs) from SSc and lung adenocarcinoma (LUAD) datasets (SSc: GSE95065, LUAD: GSE136043) in the GEO database. We found shared genes by intersecting top genes in protein–protein interaction networks by the STRING database. The area under the ROC curve (AUC) was calculated for each shared gene in validation datasets (SSc: GSE231692; LUAD: GSE43458), identifying PRKG2 as the core shared gene. We used the UALCAN platform to assess PRKG2 expression in LUAD patients at various stages and lymph node metastasis states, and compared disease-free survival (DFS) between low and high PRKG2 expression LUAD groups. PRKG2 was overexpressed in A549 cells to study its impact on lung cancer cell proliferation and invasion in vitro. We identified seven shared genes (SCN7A, AGTR1, WIF1, PRKG2, LTF, AQP4, COL10A1), with the AUC for PRKG2 exceeding 0.93 in both diseases (SSc AUC = 0.973; LUAD AUC = 0.939). The PRKG2 expression levels of LUAD patients with different clinical stages and lymph node metastasis states were consistently lower than those observed in normal individuals. The DFS of LUAD patients in the high PRKG2 expression group was higher than that in the low expression group (p = 0.028). In vitro experiments confirmed elevated PRKG2 expression inhibits the proliferation and invasion of lung cancer cells. PRKG2 is one of the genes shared by SSc and lung cancer, affecting the proliferation and invasion of lung cancer cells.
Systemic sclerosis (SSc) is a complex disease characterized by small vessel lesions, autoantibody production, and fibrosis affecting the skin and visceral organs (1). The incidence rate of SSc is 1.4 cases per 100,000 person-years (2). This disease significantly diminishes patients’ quality of life and life expectancy (3–5). Interstitial lung disease, pulmonary arterial hypertension, infections, and tumors are common causes of mortality in SSc patients (5).
Compared to the general population, SSc patients exhibit a significantly increased risk of tumorigenesis (6). The incidence rate of tumors in SSc patients varies from 3 to 10% across different populations (6–12). Among SSc patients, lung cancer is the most prevalent tumor, followed by breast cancer (6, 8, 9, 13). Risk factors for the occurrence of lung cancer in SSc patients include long-term lung involvement, smoking, prolonged SSc duration, low age at SSc diagnosis, anti-topoisomerase I antibodies, a history of renal crisis, and male gender (14). Several studies have investigated potential common mechanisms between the two diseases, such as telomere shortening, glycolysis, oxidative stress, microbiome involvement, miRNAs, and LncRNAs (14). However, the relationship between SSc and neoplasms is complex, and there is no consensus among researchers regarding their common mechanisms. Further clarification of the shared mechanisms between these two diseases is necessary.
The occurrence of cancer in SSc patients complicates their therapeutic management. These patients require long-term, sustained immunosuppressive therapy (1), which contrasts with the reliance of anti-tumor therapy on an active immune response. Therefore, investigating potential targets for the prevention and treatment of SSc complicated by lung cancer is crucial. Genes are recognized as key elements in the pathogenesis of diseases. Identifying susceptibility genes could aid in the early prevention and intervention of SSc with concurrent lung cancer. Nevertheless, knowledge about core genes that are common to both SSc and lung cancer is currently limited, highlighting the need for further research.
The rapid advancement of bioinformatics and the availability of public databases have greatly facilitated the identification of pathogenic genes (15). To date, no bioinformatics-based research has explored the core shared genes between SSc and lung cancer. In this study, we employed bioinformatics methods to analyze the core shared genes between SSc and lung cancer, with the aim of identifying potential pathogenic genes and elucidating shared mechanisms.
The data of gene expression profile for SSc and lung cancer were obtained from the Gene Expression Omnibus (GEO) database. The GEO database, maintained by the National Center for Biotechnology Information (NCBI), is a public repository for gene expression data, encompassing both microarray and next-generation sequencing studies. We utilized the GEO database to download and analyze datasets relevant to our research objectives, thereby gaining insights into the molecular mechanisms underlying diseases. Following screening, GSE95065 dataset was selected as the SSc expression dataset, and GSE136043 dataset as the lung adenocarcinoma (LUAD) dataset. The GSE95065 dataset includes transcriptomic sequencing data of skin biopsies from 18 SSc patients and 15 healthy controls (16). The GSE136043 dataset comprises mRNA expression profiles from five primary LUAD tissues and five non-neoplastic tissues (17). Additionally, we utilized the GSE231692 and GSE43458 datasets for validation. The GSE231692 dataset comprises expression profiles from skin biopsies of 33 SSc patients and 14 healthy controls (18). The GSE43458 dataset encompasses gene expression profiles from 80 LUAD samples and 30 corresponding normal lung tissue samples (19). We also utilized the GSE40839 and GSE231693 datasets to analyze the expression levels of the core shared gene in lung tissue fibroblasts of SSc associated interstitial lung disease (SSc-ILD) and normal populations. The GSE40839 dataset includes the expression profiles of fibroblasts in lung tissue from 10 normal controls and 8 SSc-ILD patients (20). The GSE231693 dataset contains expression profile data of fibroblasts from 20 normal lung tissues and 20 SSc-ILD lung tissues (18).
GEO2R, provided by NCBI, is an online tool designed for analyzing gene expression data within the GEO database. The box plots generated by GEO2R were used to display the distribution of expression levels across all samples, which allowed for the assessment of dataset quality, such as variability between samples and the normality of the data. Therefore, we employed GEO2R to confirm the standardization of the GSE95065 and GSE136043 datasets. Differential gene expression analysis was conducted on these two datasets. We applied the “limma” package (version 3.40.6) in R to identify differentially expressed genes (DEGs) (21). Thresholds for differential gene expression were set as p < 0.001 and |fold change| > 2 for the LUAD group, and p < 0.001 and |fold change| > 1.3 for the SSc group. Subsequently, we utilized Cytoscape software for Gene Ontology (GO) and Kyoto Encyclopedia of Genes and Genomes (KEGG) enrichment analyses on the DEGs (22) and constructed protein–protein interaction (PPI) networks using the STRING database (23), in order to consolidate all available and predicted protein–protein interactions.
We imported both PPI networks into Cytoscape software and screened these networks for top genes using the “cytoHubba” plugin (24), which ranks genes based on their network topological properties. Next, we intersected the top DEGs from both diseases to identify shared genes potentially involved in the pathogenesis of both diseases.
Ferroptosis exacerbates pulmonary fibrosis in SSc and leads to cell death in lung cancer, playing a significant role in the pathogenesis and progression of both diseases. Therefore, to identify the core shared gene between the two diseases, we investigated the correlation between these shared genes and ferroptosis-marker genes. All ferroptosis marker genes were sourced from the FerrDb database, the first global repository dedicated to the study of ferroptosis regulators and ferroptosis-disease relevance (25). We employed the “corrplot” R package to evaluate the correlation between the marker genes and the shared genes across the datasets. Furthermore, to determine the discriminatory ability of these shared genes in distinguishing LUAD and SSc patients from normal individuals, we used the “pROC” R package to construct receiver operating characteristic (ROC) curves and calculate the area under the curve (AUC) for each gene within both the SSc and LUAD validation datasets (26).
We identified gene groups closely associated with PRKG2 using the GeneMANIA database (27). Subsequently, we performed GO and KEGG enrichment analyses on PRKG2 and its associated genes. This analysis aids in elucidating the potential molecular mechanisms underlying PRKG2.
UALCAN is a powerful bioinformatics platform that incorporates sequencing data from the TCGA database (28). Utilizing these data, we assessed the expression levels of PRKG2 and its correlation with cancer staging and lymph node metastasis status in LUAD patients. GEPIA2 is a comprehensive platform designed for personalized analysis, offering data from 9,736 tumor types and 8,587 normal samples within the TCGA and GTEx projects (29). We employed GEPIA2 to analyze differences in overall survival (OS) and disease-free survival (DFS) among LUAD patients stratified by low and high PRKG2 expression.
CIBERSORT, the most frequently cited tool for assessing immune cell infiltration, operates on the principle of linear support vector regression for deconvolution analysis of the expression matrix of human immune cell subtypes (30). We utilized CIBERSORT to evaluate the influence of PRKG2 on immune cell infiltration within lung cancer tissues, with parameters set to signature genes (LM22), batch correction mode (B-mode), and 1,000 permutations. To bolster the credibility of our findings, we also referenced the TIMER database (31), an alternative approach for quantifying immune cell infiltration, to assess the impact of PRKG2 expression on the infiltration levels of distinct immune cell types in LUAD.
The human-derived LUAD A549 cell line was cultured in 100-mm cell culture dishes at a concentration of 1 × 106 cells/mL for this study. We then added 10 mL of RPMI 1640 complete medium containing 1% Penicillin/Streptomycin solution and 10% fetal bovine serum. When the cell density reached approximately 70% confluence, the PRKG2 overexpression plasmid (FLAG-PRKG2) and the negative control plasmid (FLAG-NC) were transfected into A549 cells using Lipofectamine™ 3000 (L3000075, Invitrogen™, United States) and Opti-MEM (31985, Gibco, United States). After 48 h, three groups of cells (FLAG-PRKG2 overexpression, FLAG-NC control, and blank control) were collected.
We extracted total RNA from each group of A549 cells. Subsequently, cDNA was synthesized using a reverse transcription kit. The mRNA expression of PRKG2 was quantified by qPCR using a kit (11143ES50, Yeasen Biotechnology, China), with GAPDH serving as an internal reference gene. The primer sequences were as follows:
PRKG2
forward: 5′-GGTTCCGTGAAACCCAAACA-3′
reverse: 5′-CACCACATCCTGAAGCTTGTT-3′
GAPDH
forward: 5′-ATCATCAGCAATGCCTCCTG-3′
reverse: 5′-ATGGACTGTGGTCATGAGTC-3′.
We utilized the Cell Counting Kit-8 (CCK-8, C6005, NCM Biotech, China) to assess the proliferation of A549 cells in both the FLAG-PRKG2 overexpression and control groups. Cells from each group were plated into 96-well plates. After the addition of the CCK-8 reagent, all plates were incubated at 37°C for 4 h, and then the absorbance at 450 nm was measured using a microplate reader.
Twenty four-well plates were utilized to culture each group of A549 cells. Upon reaching 100% confluence, a 200-μl pipette tip was used to create a scratch line in each group. The cells were rinsed with PBS, and photographs of the scratches were taken using a microscope. Additional photographs of the two groups of cells were captured after a 24-h interval and compared with the initial images.
Matrigel was applied to the upper surface of Transwell chambers (catalog number 3470, Corning, United States) for the cell invasion assay. Serum-free medium, used to culture each group of A549 cells, filled the upper chamber, whereas the lower chamber contained medium with 20% fetal bovine serum. After a 36-h incubation in a cell incubator, the upper chamber was removed, and the non-invading cells on the upper surface were wiped clean. The cells that invaded through the Matrigel were stained with methylene blue and then visualized and counted under a microscope. Except for the Matrigel application, the remaining steps of the cell migration experiment closely resembled those of the invasion assay. The detailed procedures for cell invasion and migration assays can be referred to the study by Wen et al. (32).
We utilized R software (version 4.0.5) for generating all figures. Data from the two groups were compared using an unpaired t-test. The criterion for statistical significance was a p-value of less than 0.05. Each assay was repeated a minimum of three times.
The GEO2R analysis results indicate that the GSE95065 and GSE136043 datasets have been standardized (Figures 1B,F). DEGs are depicted on volcano plots, where upregulated genes are marked with red dots, and downregulated genes with blue (Figures 1A,E). In comparison to normal individuals, the SSc group exhibited upregulation of 47 genes and downregulation of 216 genes. Likewise, the lung cancer group showed upregulation of 274 genes and downregulation of 182 genes. Subsequently, we conducted GO/KEGG functional enrichment analysis for these DEGs. The DEGs from the SSc dataset are associated with various signaling pathways, including blood coagulation, positive regulation of interleukin-10 production, epithelial cell apoptosis, neural impulse transmission, and regulation of CoA-transferase activity. Similarly, The DEGs from the lung cancer dataset are involved in pathways such as heparin binding, membrane depolarization during action potentials, negative regulation of chemotaxis, and morphogenesis of branching structures (Figures 1C,D,G,H).
Figure 1. Identification and enrichment analysis of DEGs in SSc and lung cancer datasets. (A) Volcano plot of DEGs in the GSE95065 dataset; (B) Standardization of the GSE95065 dataset; (C) GO functional enrichment analysis of DEGs in the GSE95065 dataset; (D) KEGG pathway enrichment analysis of DEGs in the GSE95065 dataset; (E) Volcano plot of DEGs in the GSE136043 dataset; (F) Standardization of the GSE136043 dataset; (G) GO functional enrichment analysis of DEGs in the GSE136043 dataset; (H) KEGG pathway enrichment analysis of DEGs in the GSE136043 dataset.
We constructed PPI networks for DEGs using the STRING database (Figures 2A,C). Subsequently, we identified the top 100 DEGs from the LUAD dataset and the top 120 DEGs from the SSc dataset. Utilizing the cytoHubba plugin, we identified several DEGs in central positions, including SERPINE1, PRKG2, AGTR1, IL-6, CCL2, and ACTB in the SSc dataset, and PROM1, FGF2, VEGFA, SPP1, PRKG2, and BDNF in the LUAD dataset (Figures 2B,D). By intersecting these top DEGs, we recognized seven genes potentially involved in the pathogenesis of both SSc and lung cancer: SCN7A, AGTR1, WIF1, PRKG2, LTF, AQP4, and COL10A1 (Figure 2E). By analyzing the correlation of these shared DEGs with ferroptosis marker genes, we found that in the SSc group, PRKG2 was significantly positively correlated with GPX4 and negatively with PTGS2, NFE2L2, and FTH1 (Figure 2F). In the lung cancer group, PRKG2 exhibited significant positive correlation with NFE2L2, SLC40A1, TFRC, and significant negative correlation with CHAC1 and HSPB1 (Figure 2G). Additionally, using independent validation datasets (LUAD: GSE43458; SSc: GSE231692), we evaluated the capacity of these shared DEGs to distinguish between the two diseases and the normal population. The results showed that PRKG2 had an AUC greater than 0.93 in both diseases (SSc AUC = 0.973; LUAD AUC = 0.939) (Figures 2H,I). In conclusion, we propose PRKG2 as a core shared gene between SSc and lung cancer.
Figure 2. Core shared DEGs from SSc and LUAD datasets. (A) PPI network of DEGs from the SSc dataset; (B) Heatmap of connections for the top 120 DEGs within the SSc dataset; (C) PPI network of DEGs from the LUAD dataset; (D) Heatmap of connections for the top 100 DEGs within the LUAD dataset; (E) Venn diagram showing the intersection of core DEGs between the SSc and LUAD datasets; (F) Correlation heatmap for the seven shared genes and ferroptosis marker genes in SSc; The numbers enclosed in circles refer to the results of the correlation analysis between two genes. (G) Correlation heatmap for the seven shared genes and ferroptosis marker genes in LUAD; The numbers enclosed in circles refer to the results of the correlation analysis between two genes. (H) ROC curve and AUC for the seven shared genes within the SSc validation dataset; (I) ROC curve and AUC for the seven shared genes within the LUAD validation dataset.
The PRKG2 expression levels in tissues from SSc and lung cancer patients were significantly lower than those of normal individuals, as evidenced by the GSE95065 and GSE136043 datasets (Figures 3A,B). Analysis of the TCGA database indicated that primary LUAD patients had lower PRKG2 expression levels than normal individuals (Figure 3C). The expression levels of PRKG2 in pulmonary fibroblasts from lung tissues of SSc-ILD patients were significantly lower than those in fibroblasts from normal lung tissues in the GSE231693 dataset. Additionally, the PRKG2 expression levels in pulmonary fibroblasts from SSc-ILD patients were also lower than in the control group in the GSE40839 dataset, yet this difference was not statistically significant (Figure 4A).
Figure 3. PRKG2 expression levels in tissues and the biological processes associated with PRKG2. (A) Expression levels of PRKG2 in the SSc dataset; (B) Expression levels of PRKG2 in the LUAD dataset; (C) Expression of PRKG2 in LUAD samples as per the TCGA database; (D) Expression of PRKG2 in LUAD samples across different pathological stages; (E) Expression of PRKG2 in LUAD samples categorized by nodal metastasis status; (F) Kaplan–Meier curve for overall survival for low and high PRKG2 expression groups in LUAD; (G) Kaplan–Meier curve for disease-free survival of low and high PRKG2 expression groups in LUAD; (H) PRKG2 and its associated genes; (I) GO/KEGG enrichment analysis for PRKG2 and its associated genes. *** indicates p < 0.001; ** indicates p < 0.01.
Figure 4. Inhibitory effects of PRKG2 on lung cancer cells in vitro. (A) Expression levels of PRKG2 in fibroblasts of lung tissues of SSc-ILD and normal populations within the datasets GSE40839 and GSE231693; (B) Overexpression of PRKG2 in A549 cells; (C) MTT assay for the impact of PRKG2 on A549 cell proliferation; (D) Wound-healing assay for the effect of PRKG2 on A549 cell migration; (E) Transwell assay for the influence of PRKG2 on A549 cell migration and invasion. *** indicates p < 0.001.
The expression of PRKG2 in LUAD patients with different clinical stages and lymph node metastasis status was significantly lower than that in the normal population (Figures 3D,E). We categorized all lung cancer patients from the GEPIA2 database into low and high PRKG2 expression groups based on the median expression value of PRKG2. The median DFS was significantly longer in the high PRKG2 expression group compared with the low expression group (p = 0.028), whereas the median OS was shorter (p = 0.037) (Figures 3F,G).
To investigate the signaling pathways associated with PRKG2, we identified a close association between PRKG2 and genes such as PTS, IRAG1, KCNMB1, KCNMB4, KCNMB3, SPR, GUCY1A1, and PDE5A (Figure 3H). The GO/KEGG functional enrichment analysis for PRKG2 and these related genes revealed that these genes influence various cellular components, including those involved in the nitric oxide stimulation of guanylate cyclase, cGMP metabolic processes, and calcium-activated potassium channels (Figure 3I).
After analyzing data from the CIBERSORT database, we observed a positive correlation between PRKG2 expression and the infiltration of M2 macrophages, mast cells, and dendritic cells, as well as a negative correlation with Treg cells and natural killer cells (Figures 5A,B). The data from TIMER database also demonstrated significant positive correlations between PRKG2 expression and the infiltration of CD8+ T cells, macrophages, dendritic cells, and neutrophils (Figure 5C).
Figure 5. Associations between PRKG2 expression and immune cell infiltration in lung cancer. (A) Heatmap depicting the associations between PRKG2 and immune cells; (B) Relative percentages of associations between PRKG2 and immune cells; (C) Correlations between PRKG2 expression and the infiltration of immune cells.
To elucidate the effects of PRKG2 on proliferation, migration, and invasion of A549 cells, we overexpressed PRKG2 in A549 cells in vitro (Figure 4B). The MTT proliferation assay indicated that PRKG2 significantly inhibited the growth of A549 cells (Figure 4C). Additionally, another assay showed that elevated PRKG2 expression slowed the wound healing of A549 cells (Figures 4D,E). The Transwell assay revealed that high PRKG2 expression inhibits the migration and invasion of A549 cells (Figure 4E).
Systemic sclerosis (SSc) is an autoimmune disease with one of the highest mortalities (33, 34), leading to substantial suffering and a heavy burden on the lives of affected patients (1). As early as the 1990s, a seminal population-based study by Persson et al. identified a significant increase in cancer incidence among SSc patients (35). Subsequent research from various countries has consistently highlighted an elevated cancer risk in SSc, with a particular emphasis on lung cancer (10, 36–39). Currently, lung cancer is the leading cause of cancer-related death in China, characterized by early metastasis and high mortality (40). Deaths attributable to lung cancer also account for a significant proportion of all-cause mortality in SSc patients (14).
With advances in gene sequencing technology, numerous public databases have been established. Researchers have since developed a range of bioinformatics tools, yielding meaningful findings that have significantly indicated potential research directions. However, variations in sequencing methodologies and standards result in the incomparability of sequencing datasets, posing challenges for analysis. Consequently, drawing on prior research, we applied a novel approach to address these challenges in our study, a method also employed by other investigators (41, 42). The GSE95065 and GSE136043 datasets are standardized transcriptomic profiles from the GEO database, facilitating our analysis of gene expression at the mRNA level. By analyzing differential gene expression of tissues between patients and normal individuals within datasets, we eliminated discrepancies arising from different detection methods and data processing protocols. This approach ensures the comparability of the DEGs from the two datasets.
After ensuring the comparability of results between datasets, we conducted a further search for potential core genes associated with both diseases. We selected varying numbers of DEGs from the datasets with the aim of identifying additional core genes specific to SSc. The linkage analysis of the top DEGs revealed the presence of multiple genes at the core locus for both diseases, with PRKG2 being particularly notable. The intersection of top DEGs also suggested that PRKG2 expression is aberrant in both conditions. Ferroptosis, an iron-dependent programmed cell death, is characterized by the accumulation of lipid peroxides and the peroxidation of unsaturated fatty acids in the cell membrane, resulting in membrane rupture and cell demise (43). Cao et al. demonstrated that ferroptosis in macrophages exacerbates fibrosis in the SSc mouse model (44). Zhang et al. identified ferroptosis and its pro-inflammatory drivers in SSc-ILD at the single-cell transcriptome level (45). Wu et al. observed upregulated expression of ferroptosis-related genes in SSc patients, which are involved in regulating cellular proliferation, differentiation, and migration (46). Also, ferroptosis triggers lung cancer cell death, modulates the tumor microenvironment, impacts metastasis, and alters cell sensitivity to chemotherapeutic agents (47). Considering the impact of ferroptosis on both diseases, we analyzed the correlation between seven shared genes and ferroptosis marker genes, revealing that PRKG2 exhibits the strongest association. Furthermore, analysis of independent validation datasets confirmed that PRKG2 is the most effective gene for distinguishing SSc and LUAD from the normal population, suggesting that PRKG2 is a core gene shared between SSc and lung cancer.
In the GSE95065 and GSE136043 datasets, PRKG2 expressions of SSc and LUAD patients were significantly lower than those of normal individuals, a finding also corroborated by data from the TCGA database. Subsequently, we observed that PRKG2 expressions of LUAD patients across various stages and lymph node metastasis statuses were significantly lower than that of the normal population. LUAD patients with low PRKG2 expression showed significantly shorter median DFS (p = 0.028), yet longer median OS (p = 0.037). This discrepancy may arise because OS is more susceptible to the influence of various confounding factors, including comorbidities. This suggests that PRKG2 may exert a sustained inhibitory effect on the onset and progression of lung cancer. Building on this hypothesis, we overexpressed PRKG2 in lung cancer cell lines in vitro and found that it significantly inhibited cell growth, invasion, and migration, aligning with previous research. Browning et al.’s study suggests that PRKG2 may suppress the proliferation of colon epithelial cells by downregulating Sox9 (48). Additionally, Chen et al. demonstrated that PRKG2 can inhibit the proliferation of various cancer cells, including lung, ovarian, and breast cancer cells, by blocking the MAPK/ERK, PI3K/Akt, Raf/MEK signaling pathways, and EGF/EGFR-related signaling cascades (49–52). Consequently, low expression of PRKG2 facilitates the growth and migration of lung cancer.
The PRKG2 gene, approximately 125 kb in length and comprising 19 exons, is located on chromosome 4 (53). It primarily encodes type II cGMP-dependent protein kinase (cGK II) (54). Reports indicate that robust cGK II signaling is detectable in the lungs of normal mice (55, 56), and PRKG2 is highly expressed in fibroblasts within normal lung tissue (57). As previously mentioned, long-term lung involvement is a notable risk factor for lung cancer development in SSc patients (14). Many researchers also found a correlation between pulmonary fibrosis and the site of lung cancer occurrence, implying that sustained fibrosis may underlie lung cancer development in SSc patients (7, 8, 14). Additionally, some studies propose a link between the carcinogenic mechanism of SSc and pro-fibrotic factors, such as miR-21, miR-199a-3p, miR-199a-5p, and miR-214 (58–61). These molecules, on one hand, enhance the expression of TGF-β to promote fibrosis (59), and on the other hand, they may also contribute to tumorigenesis (60). Although the PRKG2 expression levels in pulmonary fibroblasts from SSc-ILD patients compared to those from the normal population in the GSE40839 dataset showed no statistically significant difference, PRKG2 expression levels in pulmonary fibroblasts from SSc-ILD lungs were lower than those in normal lung tissues in both datasets (GSE231693 and GSE40839), with this difference being statistically significant in the GSE231693 dataset. This finding implies that low level of PRKG2 in SSc patients may promote pulmonary fibrosis, leading to the onset and progression of lung cancer.
Immune cell infiltration is recognized to directly modulate the onset and progression of lung cancer, a notion that is widely accepted within the scientific community (62). In our study, the expression level of PRKG2 demonstrated a significant positive correlation with macrophages and dendritic cells within the immune microenvironment of lung cancer. Conversely, it exhibited a negative correlation with Treg cells. These correlations suggest that PRKG2 may play a role in inhibiting lung cancer development through the modulation of these immune cells. This aligns with the findings reported by Kanoh, who concluded that PRKG2 modulates NF-κB activation through PP2A in human cells, thereby influencing innate immunity and inflammation (63). This could elucidate the strong correlation we observed in this study between PRKG2 and innate immune cells. Nevertheless, the current understanding of how PRKG2 mediates immune regulation is limited, necessitating further investigation in future studies.
Our study also has several limitations. Firstly, we analyzed the core shared gene between SSc and LUAD using two datasets and validated our findings with two additional independent datasets, yet potential selection bias may still be present. Secondly, while we examined the effects of PRKG2 on lung cancer cells and the expression of PRKG2 in fibroblasts from lung tissues of SSc-ILD patients, we did not evaluate the effects of PRKG2 in SSc animal models, an aspect we plan to address in future research. Thirdly, although there is a suggested correlation between PRKG2 expression levels and the immune microenvironment of lung cancer, further investigation using immunohistochemical scoring is required due to limited data. Fourthly, due to the absence of demographic and clinical characteristic data, such as SSc subtypes and autoantibodies, from these datasets, we were unable to conduct subgroup analyses to elucidate the impact of these clinical variables on the occurrence of lung cancer in SSc patients. Patients with different SSc subtypes or those carrying various autoantibodies may exhibit distinct gene expression profiles, potentially influencing their development risk of lung cancer. Consequently, the lack of these data may hinder a comprehensive understanding of our study’s findings.
In summary, this study represents the first to investigate the shared genetic underpinnings between SSc and lung cancer. As a core gene common to both diseases, PRKG2 exerts a significant influence on the proliferation and migration of lung cancer.
The original contributions presented in the study are included in the article/Supplementary material, further inquiries can be directed to the corresponding author.
Ethical approval was not required for the studies on humans in accordance with the local legislation and institutional requirements because only commercially available established cell lines were used.
PP: Data curation, Visualization, Writing – original draft. XL: Data curation, Formal analysis, Investigation, Writing – review & editing. YW: Data curation, Investigation, Writing – review & editing. HW: Formal analysis, Investigation, Writing – review & editing. CX: Supervision, Writing – review & editing. JL: Data curation, Supervision, Writing – review & editing.
The author(s) declare that no financial support was received for the research, authorship, and/or publication of this article.
The authors declare that the research was conducted in the absence of any commercial or financial relationships that could be construed as a potential conflict of interest.
All claims expressed in this article are solely those of the authors and do not necessarily represent those of their affiliated organizations, or those of the publisher, the editors and the reviewers. Any product that may be evaluated in this article, or claim that may be made by its manufacturer, is not guaranteed or endorsed by the publisher.
The Supplementary material for this article can be found online at: https://www.frontiersin.org/articles/10.3389/fmed.2024.1431642/full#supplementary-material
1. Volkmann, ER, Andréasson, K, and Smith, V. Systemic sclerosis. Lancet. (2023) 401:304–18. doi: 10.1016/S0140-6736(22)01692-0
2. Bairkdar, M, Rossides, M, Westerlind, H, Hesselstrand, R, Arkema, EV, and Holmqvist, M. Incidence and prevalence of systemic sclerosis globally: a comprehensive systematic review and meta-analysis. Rheumatology (Oxford). (2021) 60:3121–33. doi: 10.1093/rheumatology/keab190
3. Ioannidis, JP, Vlachoyiannopoulos, PG, Haidich, AB, Medsger, TA Jr, Lucas, M, Michet, CJ, et al. Mortality in systemic sclerosis: an international meta-analysis of individual patient data. Am J Med. (2005) 118:2–10. doi: 10.1016/j.amjmed.2004.04.031
4. Jacobsen, S, Halberg, P, and Ullman, S. Mortality and causes of death of 344 Danish patients with systemic sclerosis (scleroderma). Br J Rheumatol. (1998) 37:750–5. doi: 10.1093/rheumatology/37.7.750
5. Tyndall, AJ, Bannert, B, Vonk, M, Airò, P, Cozzi, F, Carreira, PE, et al. Causes and risk factors for death in systemic sclerosis: a study from the EULAR scleroderma trials and research (EUSTAR) database. Ann Rheum Dis. (2010) 69:1809–15. doi: 10.1136/ard.2009.114264
6. Bonifazi, M, Tramacere, I, Pomponio, G, Gabrielli, B, Avvedimento, EV, La Vecchia, C, et al. Systemic sclerosis (scleroderma) and cancer risk: systematic review and meta-analysis of observational studies. Rheumatology (Oxford). (2013) 52:143–54. doi: 10.1093/rheumatology/kes303
7. Rosenthal, AK, McLaughlin, JK, Gridley, G, and Nyrén, O. Incidence of cancer among patients with systemic sclerosis. Cancer. (1995) 76:910–4. doi: 10.1002/1097-0142(19950901)76:5<910::AID-CNCR2820760528>3.0.CO;2-T
8. Abu-Shakra, M, Guillemin, F, and Lee, P. Cancer in systemic sclerosis. Arthritis Rheum. (1993) 36:460–4. doi: 10.1002/art.1780360405
9. Roumm, AD, and Medsger, TA Jr. Cancer and systemic sclerosis. An Epidemiologic study. Arthritis Rheum. (1985) 28:1336–40. doi: 10.1002/art.1780281204
10. Hill, CL, Nguyen, AM, Roder, D, and Roberts-Thomson, P. Risk of cancer in patients with scleroderma: a population based cohort study. Ann Rheum Dis. (2003) 62:728–31. doi: 10.1136/ard.62.8.728
11. Derk, CT, Rasheed, M, Artlett, CM, and Jimenez, SA. A cohort study of cancer incidence in systemic sclerosis. J Rheumatol. (2006) 33:1113–6.
12. Chatterjee, S, Dombi, GW, Severson, RK, and Mayes, MD. Risk of malignancy in scleroderma: a population-based cohort study. Arthritis Rheum. (2005) 52:2415–24. doi: 10.1002/art.21225
13. Olesen, AB, Svaerke, C, Farkas, DK, and Sørensen, HT. Systemic sclerosis and the risk of cancer: a nationwide population-based cohort study. Br J Dermatol. (2010) 163:800–6. doi: 10.1111/j.1365-2133.2010.09861.x
14. Lepri, G, Catalano, M, Bellando-Randone, S, Pillozzi, S, Giommoni, E, Giorgione, R, et al. Systemic sclerosis association with malignancy. Clin Rev Allergy Immunol. (2022) 63:398–416. doi: 10.1007/s12016-022-08930-4
15. Borrego-Yaniz, G, Terrón-Camero, LC, Kerick, M, Andrés-León, E, and Martin, J. A holistic approach to understanding immune-mediated inflammatory diseases: bioinformatic tools to integrate omics data. Comput Struct Biotechnol J. (2024) 23:96–105. doi: 10.1016/j.csbj.2023.11.045
16. Lenna, S, Assassi, S, Farina, GA, Mantero, JC, Scorza, R, Lafyatis, R, et al. The HLA-B*35 allele modulates ER stress, inflammation and proliferation in PBMCs from limited cutaneous systemic sclerosis patients. Arthritis Res Ther. (2015) 17:363. doi: 10.1186/s13075-015-0881-1
17. Jiang, N, Zou, C, Zhu, Y, Luo, Y, Chen, L, Lei, Y, et al. HIF-1ɑ-regulated mi R-1275 maintains stem cell-like phenotypes and promotes the progression of LUAD by simultaneously activating Wnt/β-catenin and notch signaling. Theranostics. (2020) 10:2553–70. doi: 10.7150/thno.41120
18. Jia, G, Ramalingam, TR, Heiden, JV, Gao, X, DePianto, D, Morshead, KB, et al. An interleukin 6 responsive plasma cell signature is associated with disease progression in systemic sclerosis interstitial lung disease. iScience. (2023) 26:108133. doi: 10.1016/j.isci.2023.108133
19. Kabbout, M, Garcia, MM, Fujimoto, J, Liu, DD, Woods, D, Chow, CW, et al. ETS2 mediated tumor suppressive function and MET oncogene inhibition in human non-small cell lung cancer. Clin Cancer Res. (2013) 19:3383–95. doi: 10.1158/1078-0432.CCR-13-0341
20. Lindahl, GE, Stock, CJ, Shi-Wen, X, Leoni, P, Sestini, P, Howat, SL, et al. Microarray profiling reveals suppressed interferon stimulated gene program in fibroblasts from scleroderma-associated interstitial lung disease. Respir Res. (2013) 14:80. doi: 10.1186/1465-9921-14-80
21. Ritchie, ME, Phipson, B, Wu, D, Hu, Y, Law, CW, Shi, W, et al. Limma powers differential expression analyses for RNA-sequencing and microarray studies. Nucleic Acids Res. (2015) 43:e47. doi: 10.1093/nar/gkv007
22. Yu, G, Wang, LG, Han, Y, and He, QY. Cluster profiler: an R package for comparing biological themes among gene clusters. Omics. (2012) 16:284–7. doi: 10.1089/omi.2011.0118
23. Szklarczyk, D, Gable, AL, Nastou, KC, Lyon, D, Kirsch, R, Pyysalo, S, et al. The STRING database in 2021: customizable protein-protein networks, and functional characterization of user-uploaded gene/measurement sets. Nucleic Acids Res. (2021) 49:D605–12. doi: 10.1093/nar/gkaa1074
24. Chin, CH, Chen, SH, Wu, HH, Ho, CW, Ko, MT, and Lin, CY. Cyto Hubba: identifying hub objects and sub-networks from complex interactome. BMC Syst Biol. (2014) 8:S11. doi: 10.1186/1752-0509-8-S4-S11
25. Zhou, N, Yuan, X, Du, Q, Zhang, Z, Shi, X, Bao, J, et al. Ferr Db V2: update of the manually curated database of ferroptosis regulators and ferroptosis-disease associations. Nucleic Acids Res. (2023) 51:D571–82. doi: 10.1093/nar/gkac935
26. Robin, X, Turck, N, Hainard, A, Tiberti, N, Lisacek, F, Sanchez, JC, et al. pROC: an open-source package for R and S+ to analyze and compare ROC curves. BMC Bioinformatics. (2011) 12:77. doi: 10.1186/1471-2105-12-77
27. Warde-Farley, D, Donaldson, SL, Comes, O, Zuberi, K, Badrawi, R, Chao, P, et al. The gene MANIA prediction server: biological network integration for gene prioritization and predicting gene function. Nucleic Acids Res. (2010) 38:W214–20. doi: 10.1093/nar/gkq537
28. Chandrashekar, DS, Karthikeyan, SK, Korla, PK, Patel, H, Shovon, AR, Athar, M, et al. UALCAN: an update to the integrated cancer data analysis platform. Neoplasia (New York, NY). (2022) 25:18–27. doi: 10.1016/j.neo.2022.01.001
29. Tang, Z, Kang, B, Li, C, Chen, T, and Zhang, Z. GEPIA2: an enhanced web server for large-scale expression profiling and interactive analysis. Nucleic Acids Res. (2019) 47:W556–60. doi: 10.1093/nar/gkz430
30. Chen, B, Khodadoust, MS, Liu, CL, Newman, AM, and Alizadeh, AA. Profiling tumor infiltrating immune cells with CIBERSORT. Methods Mol Biol. (2018) 1711:243–59. doi: 10.1007/978-1-4939-7493-1_12
31. Li, T, Fan, J, Wang, B, Traugh, N, Chen, Q, Liu, JS, et al. TIMER: a web server for comprehensive analysis of tumor-infiltrating immune cells. Cancer Res. (2017) 77:e108–10. doi: 10.1158/0008-5472.CAN-17-0307
32. Wen, M, Kwon, Y, Wang, Y, Mao, JH, and Wei, G. Elevated expression of UBE2T exhibits oncogenic properties in human prostate cancer. Oncotarget. (2015) 6:25226–39. doi: 10.18632/oncotarget.4712
33. Steen, VD, and Medsger, TA. Changes in causes of death in systemic sclerosis, 1972-2002. Ann Rheum Dis. (2007) 66:940–4. doi: 10.1136/ard.2006.066068
34. Elhai, M, Meune, C, Boubaya, M, Avouac, J, Hachulla, E, Balbir-Gurman, A, et al. Mapping and predicting mortality from systemic sclerosis. Ann Rheum Dis. (2017) 76:1897–905. doi: 10.1136/annrheumdis-2017-211448
35. Rosenthal, AK, McLaughlin, JK, Linet, MS, and Persson, I. Scleroderma and malignancy: an epidemiological study. Ann Rheum Dis. (1993) 52:531–3. doi: 10.1136/ard.52.7.531
36. Zhong, H, Zhou, J, Zhang, S, Xu, Y, Hou, Y, Li, M, et al. Risk and prognosis factors for systemic sclerosis with lung cancer: a single-Centre case-control study in China. Int J Clin Pract. (2021) 75:e13819. doi: 10.1111/ijcp.13819
37. Peng, H, Wu, X, Wen, Y, Li, C, Lin, J, Li, J, et al. Association between systemic sclerosis and risk of lung cancer: results from a pool of cohort studies and Mendelian randomization analysis. Autoimmun Rev. (2020) 19:102633. doi: 10.1016/j.autrev.2020.102633
38. Colaci, M, Giuggioli, D, Sebastiani, M, Manfredi, A, Vacchi, C, Spagnolo, P, et al. Lung cancer in scleroderma: results from an Italian rheumatologic center and review of the literature. Autoimmun Rev. (2013) 12:374–9. doi: 10.1016/j.autrev.2012.06.003
39. Kuo, CF, Luo, SF, Yu, KH, Chou, IJ, Tseng, WY, Chang, HC, et al. Cancer risk among patients with systemic sclerosis: a nationwide population study in Taiwan. Scand J Rheumatol. (2012) 41:44–9. doi: 10.3109/03009742.2011.618145
40. Chen, W, Zheng, R, Baade, PD, Zhang, S, Zeng, H, Bray, F, et al. Cancer statistics in China, 2015. CA Cancer J Clin. (2016) 66:115–32. doi: 10.3322/caac.21338
41. Li, H, Yu, L, Zhang, X, Shang, J, and Duan, X. Exploring the molecular mechanisms and shared gene signatures between rheumatoid arthritis and diffuse large B cell lymphoma. Front Immunol. (2022) 13:1036239. doi: 10.3389/fimmu.2022.1036239
42. Liu, TT, Yin, DT, Wang, N, Li, N, Dong, G, and Peng, MF. Identifying and analyzing the key genes shared by papillary thyroid carcinoma and Hashimoto’s thyroiditis using bioinformatics methods. Front Endocrinol (Lausanne). (2023) 14:1140094. doi: 10.3389/fendo.2023.1140094
43. Jiang, X, Stockwell, BR, and Conrad, M. Ferroptosis: mechanisms, biology and role in disease. Nat Rev Mol Cell Biol. (2021) 22:266–82. doi: 10.1038/s41580-020-00324-8
44. Cao, D, Zheng, J, Li, Z, Yu, Y, Chen, Z, and Wang, Q. ACSL4 inhibition prevents macrophage ferroptosis and alleviates fibrosis in bleomycin-induced systemic sclerosis model. Arthritis Res Ther. (2023) 25:212. doi: 10.1186/s13075-023-03190-9
45. Zhang, D, Li, Y, Du, C, Sang, L, Liu, L, Li, Y, et al. Evidence of pyroptosis and ferroptosis extensively involved in autoimmune diseases at the single-cell transcriptome level. J Transl Med. (2022) 20:363. doi: 10.1186/s12967-022-03566-6
46. Wu, C, Liu, J, Chen, Z, Wu, Y, and Gao, F. Comprehensive analysis of ferroptosis-related hub gene signatures as a potential pathogenesis and therapeutic target for systemic sclerosis: a bioinformatics analysis. Int J Immunopathol Pharmacol. (2023) 37:3946320231187783. doi: 10.1177/03946320231187783
47. Wu, S, Zhu, C, Tang, D, Dou, QP, Shen, J, and Chen, X. The role of ferroptosis in lung cancer. Biomark Res. (2021) 9:82. doi: 10.1186/s40364-021-00338-0
48. Wang, R, Kwon, IK, Thangaraju, M, Singh, N, Liu, K, Jay, P, et al. Type 2 cGMP-dependent protein kinase regulates proliferation and differentiation in the colonic mucosa. Am J Physiol Gastrointest Liver Physiol. (2012) 303:G209–19. doi: 10.1152/ajpgi.00500.2011
49. Wu, Y, Yuan, M, Su, W, Zhu, M, Yao, X, Wang, Y, et al. The constitutively active PKG II mutant effectively inhibits gastric cancer development via a blockade of EGF/EGFR-associated signalling cascades. Ther Adv Med Oncol. (2018) 10:1758834017751635. doi: 10.1177/1758834017751635
50. Wu, Y, Cai, Q, Li, W, Cai, Z, Liu, Y, Li, H, et al. Active PKG II inhibited the growth and migration of ovarian cancer cells through blocking Raf/MEK and PI3K/Akt signaling pathways. Biosci Rep. (2019) 39:BSR20190405. doi: 10.1042/BSR20190405
51. Tao, Y, Gu, YJ, Cao, ZH, Bian, XJ, Lan, T, Sang, JR, et al. Endogenous cGMP-dependent protein kinase reverses EGF-induced MAPK/ERK signal transduction through phosphorylation of VASP at Ser 239. Oncol Lett. (2012) 4:1104–8. doi: 10.3892/ol.2012.851
52. Wu, M, Chen, Y, Jiang, L, Li, Y, Lan, T, Wang, Y, et al. Type II cGMP-dependent protein kinase inhibits epidermal growth factor-induced phosphatidylinositol-3-kinase/Akt signal transduction in gastric cancer cells. Oncol Lett. (2013) 6:1723–8. doi: 10.3892/ol.2013.1630
53. Vaandrager, AB, Hogema, BM, and de Jonge, HR. Molecular properties and biological functions of cGMP-dependent protein kinase II. Front Biosci. (2005) 10:2150–64. doi: 10.2741/1687
54. Orstavik, S, Solberg, R, Taskén, K, Nordahl, M, Altherr, MR, Hansson, V, et al. Molecular cloning, cDNA structure, and chromosomal localization of the human type II cGMP-dependent protein kinase. Biochem Biophys Res Commun. (1996) 220:759–65. doi: 10.1006/bbrc.1996.0477
55. Uhler, MD. Cloning and expression of a novel cyclic GMP-dependent protein kinase from mouse brain. J Biol Chem. (1993) 268:13586–91. doi: 10.1016/S0021-9258(19)38689-2
56. Pfeifer, A, Aszódi, A, Seidler, U, Ruth, P, Hofmann, F, and Fässler, R. Intestinal secretory defects and dwarfism in mice lacking cGMP-dependent protein kinase II. Science. (1996) 274:2082–6. doi: 10.1126/science.274.5295.2082
57. Kulaksiz, H, Schmid, A, Hönscheid, M, Ramaswamy, A, and Cetin, Y. Clara cell impact in air-side activation of CFTR in small pulmonary airways. Proc Natl Acad Sci USA. (2002) 99:6796–801. doi: 10.1073/pnas.102171199
58. Zhang, L, Wu, H, Zhao, M, and Lu, Q. Meta-analysis of differentially expressed micro RNAs in systemic sclerosis. Int J Rheum Dis. (2020) 23:1297–304. doi: 10.1111/1756-185X.13924
59. Zhu, H, Luo, H, Li, Y, Zhou, Y, Jiang, Y, Chai, J, et al. Micro RNA-21 in scleroderma fibrosis and its function in TGF-β-regulated fibrosis-related genes expression. J Clin Immunol. (2013) 33:1100–9. doi: 10.1007/s10875-013-9896-z
60. Bautista-Sánchez, D, Arriaga-Canon, C, Pedroza-Torres, A, De La Rosa-Velázquez, IA, González-Barrios, R, Contreras-Espinosa, L, et al. The promising role of mi R-21 as a Cancer biomarker and its importance in RNA-based therapeutics. Mol Ther Nucleic Acids. (2020) 20:409–20. doi: 10.1016/j.omtn.2020.03.003
61. Yang, Y, Li, Z, Yuan, H, Ji, W, Wang, K, Lu, T, et al. Reciprocal regulatory mechanism between mi R-214-3p and FGFR1 in FGFR1-amplified lung cancer. Oncogenesis. (2019) 8:50. doi: 10.1038/s41389-019-0151-1
62. Binnewies, M, Roberts, EW, Kersten, K, Chan, V, Fearon, DF, Merad, M, et al. Understanding the tumor immune microenvironment (TIME) for effective therapy. Nat Med. (2018) 24:541–50. doi: 10.1038/s41591-018-0014-x
Keywords: systemic sclerosis, lung cancer, differentially expressed genes, ferroptosis, PRKG2
Citation: Pan P, Liu X, Wang Y, Wang H, Xu C and Lu J (2024) Unveiling the shared genes between systemic sclerosis and lung cancer. Front. Med. 11:1431642. doi: 10.3389/fmed.2024.1431642
Received: 12 May 2024; Accepted: 06 December 2024;
Published: 18 December 2024.
Edited by:
João Eurico Fonseca, University of Lisbon, PortugalReviewed by:
Gonçalo Boleto, Lisbon Academic Center of Medicine, PortugalCopyright © 2024 Pan, Liu, Wang, Wang, Xu and Lu. This is an open-access article distributed under the terms of the Creative Commons Attribution License (CC BY). The use, distribution or reproduction in other forums is permitted, provided the original author(s) and the copyright owner(s) are credited and that the original publication in this journal is cited, in accordance with accepted academic practice. No use, distribution or reproduction is permitted which does not comply with these terms.
*Correspondence: Junhui Lu, aGFleWxqaEAxNjMuY29t
Disclaimer: All claims expressed in this article are solely those of the authors and do not necessarily represent those of their affiliated organizations, or those of the publisher, the editors and the reviewers. Any product that may be evaluated in this article or claim that may be made by its manufacturer is not guaranteed or endorsed by the publisher.
Research integrity at Frontiers
Learn more about the work of our research integrity team to safeguard the quality of each article we publish.