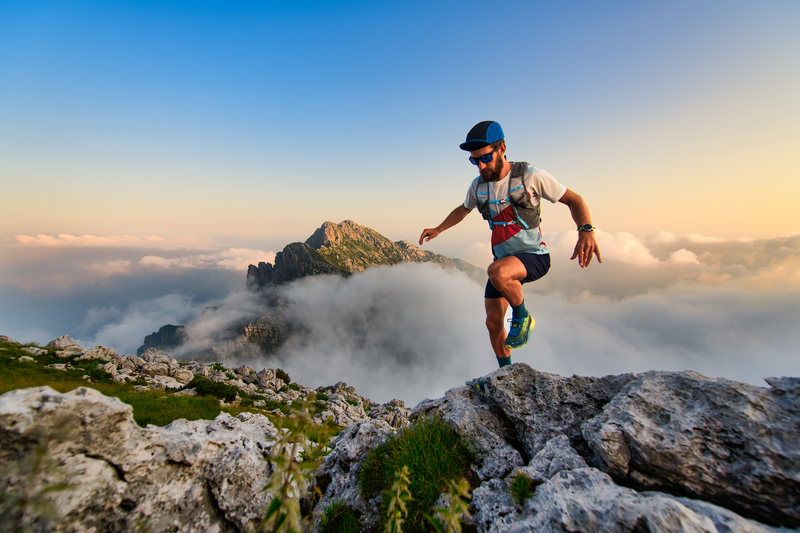
94% of researchers rate our articles as excellent or good
Learn more about the work of our research integrity team to safeguard the quality of each article we publish.
Find out more
ORIGINAL RESEARCH article
Front. Med. , 20 September 2024
Sec. Gastroenterology
Volume 11 - 2024 | https://doi.org/10.3389/fmed.2024.1428973
This article is part of the Research Topic From Bench to Bedside in Gastric Cancer: Diagnosis, Prognosis, and Treatment - Volume II View all 8 articles
Background: Coronavirus disease 2019 (COVID-19), an infectious disease caused by severe acute respiratory syndrome coronavirus-2 (SARS-CoV-2), has caused a global pandemic. Gastric cancer (GC) poses a great threat to people’s health, which is a high-risk factor for COVID-19. Previous studies have found some associations between GC and COVID-19, whereas the underlying molecular mechanisms are not well understood.
Methods: We employed bioinformatics and systems biology to explore these links between GC and COVID-19. Gene expression profiles of COVID-19 (GSE196822) and GC (GSE179252) were obtained from the Gene Expression Omnibus (GEO) database. After identifying the shared differentially expressed genes (DEGs) for GC and COVID-19, functional annotation, protein-protein interaction (PPI) network, hub genes, transcriptional regulatory networks and candidate drugs were analyzed.
Results: We identified 209 shared DEGs between COVID-19 and GC. Functional analyses highlighted immune-related pathways as key players in both diseases. Ten hub genes (CDK1, KIF20A, TPX2, UBE2C, HJURP, CENPA, PLK1, MKI67, IFI6, IFIT2) were identified. The transcription factor/gene and miRNA/gene interaction networks identified 38 transcription factors (TFs) and 234 miRNAs. More importantly, we identified ten potential therapeutic agents, including ciclopirox, resveratrol, etoposide, methotrexate, trifluridine, enterolactone, troglitazone, calcitriol, dasatinib and deferoxamine, some of which have been reported to improve and treat GC and COVID-19.
Conclusion: This research offer valuable insights into the molecular interplay between COVID-19 and GC, potentially guiding future therapeutic strategies.
Coronavirus disease 2019 (COVID-19) has caused a global pandemic, posing a significant health challenge worldwide and resulting in the deaths of over 6 million people (1). As the pandemic progresses, new variants of the severe acute respiratory syndrome coronavirus 2 (SARS-CoV-2) have emerged, with the World Health Organization (WHO) identifying variants of concern, including Alpha, Beta, Gamma, Delta, and Omicron (2, 3). SARS-CoV-2 enters the body by interacting with the angiotensin-converting enzyme 2 (ACE2) receptor and replicates within the epithelium, subsequently infecting surrounding cells (4). ACE2, a critical component of the renin-angiotensin system, has been identified as a membrane receptor for SARS-CoV-2 (5). Additionally, SARS-CoV-2 also enters host cells with the primary or auxiliary help of host proteases transmembrane protease serine 2 (TMPRSS2), FURIN (6), glucose-regulating protein 78 (GRP78) receptor (7), dipeptidyl peptidase 4 (DPP4) (8), cluster of differentiation 147 (CD147) transmembrane protein (9), tyrosine-protein kinase receptor UFO (AXL) (10), phosphatidylinositol 3-phosphate 5-kinase (PIKfyve) (11), two pore channel subtype 2 (TPC2) (12) and cathepsin L (13). Infection by SARS-CoV-2 alters alveolar vascular permeability, leading to lung injuries like pulmonary edema and pulmonary ischemia (14). Beyond the lungs, SARS-CoV-2 can spread to the brain, heart, gastrointestinal tract, and other organs through the bloodstream, causing severe complications (15–19). Due to the frequent genome reorganization of SARS-CoV-2, COVID-19 is likely to evolve and become seasonal epidemics (20). Clinical and epidemiological data suggest that underlying conditions, such as cancer, hypertension, cardiovascular disease, and diabetes, increase susceptibility to SARS-CoV-2 infection and can lead to more severe outcomes, including lung damage and death (21). Therefore, understanding how to treat COVID-19 in individuals with underlying diseases, including cancer, is of great research and clinical significance.
Gastric cancer (GC) is a major global health concern and the fourth leading cause of cancer-related deaths worldwide (22). GC incidence shows a strong geographic pattern, with the highest rates in East Asia, some South American and Eastern European countries, and the lowest in Africa and North America. More than 70% of GC cases occur in developing countries. GC patients typically have a poor prognosis and low long-term survival rates (23). Evidence suggests that GC patients are more vulnerable to SARS-CoV-2 infection, with immunotherapy and radiotherapy within three months of a COVID-19 diagnosis being risk factors for death (24). The expression profile of the SARS-CoV-2 host receptor ACE2 protein in the human gastrointestinal tract revealed that ACE2 was detectable in the gastric pits, fundic glands, gastric chief cells (pepsinogen-secreting cells), parietal cells (gastric acid-secreting cells), and pyloric glands, suggesting that gastric tissue may be susceptible to SARS-CoV-2 infection (25). Moreover, the intensity of ACE2 staining is significantly higher in gastric tumor tissues compared to adjacent non-tumor tissues, indicating that GC patients may be at increased risk of SARS-CoV-2 infection (25). A Mendelian randomization study also suggests a causal relationship between SARS-CoV-2 infection and an increased risk of gastric cancer (26). These findings underscore the importance of understanding the molecular mechanisms underlying the interaction between COVID-19 and gastric cancer.
In this study, we hypothesize that there are shared molecular mechanisms between COVID-19 and GC that could inform new therapeutic strategies. Specifically, we address the following research questions: 1. What are the common differentially expressed genes (DEGs) between COVID-19 and gastric cancer? 2. How do these shared DEGs contribute to the onset and progression of both diseases? 3. Can hub genes within the protein-protein interaction (PPI) network reveal key molecular players that may serve as potential therapeutic targets? 4. What are the potential drugs that could target these shared molecular mechanisms, and how might they contribute to the treatment of both COVID-19 and gastric cancer? To answer these questions, transcriptome profiles were obtained from the National Center for Biological Information (NCBI)-Gene Expression Omnibus (GEO) database. The datasets of COVID-19 and GC were studied to find DEGs for both diseases. These sets of DEGs were then compared to gain mutual DEGs. Moreover, the biological function of the common DEGs was analyzed to gain insights into its impact on disease onset and progression. A protein-protein interaction network was used to identify hub genes with the most obvious interactions and narrow down potential biomolecules. Next, the hub genes were used to establish the gene-regulatory network, predict potential drugs, and complete the gene-disease association network. This study identified the relationship between gastric cancer and COVID-19, potentially providing new ideas to assist in the treatment of GC and COVID-19.
Figure 1 shows the successive workflow of this study. To determine the mutual genetic interrelationship between SARS-CoV-2 infection and gastric cancer, we used the GEO database1 of NCBI to obtain RNA-seq datasets. For SARS-CoV-2 patients, we used GSE196822 dataset (27), which includes whole-blood transcriptome profiling of 34 COVID-19 patients and 9 healthy controls. The data came from high-throughput sequencing using the Illumina HiSeq 4000 (Homo sapiens). Gastric cancer (GSE179252) (28) consists of 38 gastric tumors and paired normal 38 gastric tissues which was based on Illumina HiSeq 4000 (Homo sapiens).
The key target of the analysis is to find the DEGs for the datasets GSE196822 and GSE179252. The DEseq2 package (29) of R software (version 4.2.1) was used to identify the DEGs with false-discovery rate (FDR) < 0.05 and | log2 Fold Change| > 1. To extract the shared DEGs between COVID-19 and gastric cancer, an online VENN visual tool called Jvenn2 was used (30).
The use of gene ontology (GO) enrichment methods is widespread for demonstrating the relationship between genes and GO terms, and the GO database is a comprehensive resource on gene and gene product functions (31). GO annotated sources for biological process (BP), molecular function (MF), and cellular component (CC) were retrieved from the GO database (32). To identify pathways shared by GC and COVID-19, we considered the following three repositories as the origin of pathway classification: WikiPathways, Reactome, and the Kyoto Encyclopedia of Genes and Genomes (KEGG). We used Enrichr (33)3 for gene ontology and pathway enrichment investigations. To quantify the top pathways and functional items, a standardized index with a P-value < 0.05 was utilized.
STRING (version 11.5) database,4 an online protein-protein association networks platform, has been used to construct the PPI network with a filter condition (combined score > 0.5) (34). All the common DEGs between COVID-19 and GC were used to build the PPI network. Then, the PPI network was consumed into Cytoscape (v.3.9.1) for visual representation and hub genes’ recognition (35). We used Maximal Clique Centrality (MCC) method of Cytohubba (a plugin of Cytoscape)5 to identify the top 10 hub genes from the PPI network (36). At the same time, Cytohubba’s proximity ranking characteristics helped us to identify the shortest reachable pathways linking hub genes.
Transcription factors (TFs) are proteins attached to specific genes that control the genetic information’s transcription rate; as such, they are essential for molecular insight (37). Our approach involved utilizing the NetworkAnalyst platform (version 3.0) (38) to identify topologically feasible TFs from the JASPAR database that could be potentially integrated with hub genes. JASPAR is a publicly accessible database that compiles information on TFs across six taxonomic groups for various species (39). miRNAs targeting gene interactions are also included in studies to identify miRNAs that tend to bind to gene transcripts and thus negatively impact protein production. We used Network-Analyst to analyze miRNAs-gene interactions from Tarbase (version 8.0) (40) databases.
Another emphasis of this study was to use the hub genes of COVID-19 and GC to predict protein-drug interactions (PDIs) or drug molecule recognition. Using Enrichr’s disease/drug functions, based on hub genes, drug molecules were predicted from the Drug Signatures Database (DSigDB)6 (41), which contains 17,389 unique chemicals that span 19,531 genes and has 22,527 gene sets.
DisGeNET7 (42) is a platform that integrates and standardizes data on genes associated with diseases from diverse sources. Currently, DisGeNET has information on about 24,000 illnesses and features, 17,000 genes, and 117,000 genetic variations. To identify the relationship between relevant diseases and common DEGs, we use DisGeNET, Network-Analyst and Cytoscape to investigate the relationship between genes and diseases.
To investigate the correlation and influence between GC and COVID-19, we analyzed human RNA-seq datasets from GEO and identified shared DEGs that may trigger both COVID-19 and GC. In this study, 1,668 genes were found to be differentially expressed in COVID-19, including 839 up regulated DEGs and 829 down regulated DEGs (Supplementary Table 1). Similarly, 3,447 DEGs were identified in the GC data, including 1045 up regulated DEGs and 2402 down regulated DEGs (Supplementary Table 2). The information about the two datasets has been integrated in Table 1. To find shared DEGs between GC and COVID-19, we performed a cross-comparative evaluation using Jvenn and identified 209 common DEGs in both datasets (Figure 2 and Supplementary Table 3). There are multiple genes in common between GC and COVID-19, suggesting some similarity between the two diseases.
Table 1. Overview of datasets with their geo-features and their quantitative measurements in this analysis.
To investigate the enrichment pathways and biological significance of common DEGs between COVID-19 and GC, we used Enrichr for gene functional annotation. Figure 3 and Table 2 summarized the top 10 enriched GO categories in the biological process, molecular function and cellular component categories. Notably, common DEGs were significantly enriched in immune-related pathways, which include neutrophil degranulation (GO:0043312), neutrophil activation involved in immune response (GO:0002283), neutrophil mediated immunity (GO:0002446), innate immune response (GO:0045087), defense response to virus (GO:0051607), receptor ligand activity (GO:0048018), and cytokine activity (GO:0005125).
Figure 3. The bar graphs depicting the gene ontology enrichment analysis of shared DEGs between COVID-19 and gastric cancer. (A) biological process. (B) molecular function. (C) cellular component. The lighter the color, the more significant it is.
Pathway analysis can highlight how underlying molecular and biological processes interact (43). Figure 4 and Table 3 show the main pathways of common DEGs enrichment in WikiPathways, Reactome, and KEGG. Pathway enrichment analysis showed that common DEGs are mainly involved in the regulation of immune-related pathways, including TGF-beta Receptor Signaling WP560, Neutrophil Degranulation R-HSA-6798695, Immune System R-HSA-168256, Innate Immune System R-HSA-168249, Immunoregulatory Interactions Between A Lymphoid And A non-Lymphoid Cell R-HSA-198933, Transcriptional Regulation Of Granulopoiesis R-HSA-9616222, Interferon Alpha/Beta Signaling R-HSA-909733 and B cell receptor signaling pathway. These results provide strong evidence that these common DEGs play a role in the onset and development of COVID-19 and GC through immune-related pathways.
Figure 4. The bar graphs of pathway enrichment analysis of mutual DEGs between COVID-19 and GC. (A) WikiPathway. (B) Reactome pathway. (C) KEGG. The lighter the color, the more significant it is.
PPI networks can visualize the interrelationships between different proteins and can help us understand the underlying mechanisms by which proteins interact (44). Using STRING and Cytoscape, we built and visualized a PPI network of shared DEGs between COVID-19 and GC, which encompasses 46 nodes and 82 edges, as depicted in Figure 5. The most entangled nodes among them are hub genes. These hub genes have the potential to serve as biomarkers and may offer novel insights into therapeutic approaches. The top 10 hub genes with the highest MCC scores were identified using the cytoHubba plugin of Cytoscape, namely CDK1, KIF20A, TPX2, UBE2C, HJURP, CENPA, PLK1, MKI67, IFI6, and IFIT2 (Figure 6 and Supplementary Table 4).
Figure 5. PPI network of shared DEGs between COVID-19 and GC. The DEGs in the figure are represented by circles and the edges mean the connections between the nodes. The PPI network consists of 46 nodes and 82 edges.
Figure 6. The hub genes were obtained from the PPI network. The nodes in orange represent the top 10 prominent hub genes and the interactions among them and other molecules. This network contains 26 nodes and 60 edges.
To figure out how hub genes modulate COVID-19 and GC at the transcriptional level, this study also investigated the interaction between TFs and genes, as well as miRNAs. In this study, a network-based approach was used to decode regulatory transcription factors and miRNAs as a way to gain insight into hub genes and find substantial changes that occur at the transcriptional level. As shown in Figure 7, the figure exhibits the interaction network of 38 transcription factors, such as ESR2, REL, SRY, SPIB, NR2F1, BRCA1, FOS, CREB1, FOXC1 and GATA2 (Supplementary Table 5). Similarly, Figure 8 represents the interaction network of miRNAs regulators and hub genes, containing 234 miRNAs, such as hsa-mir-16-5p, hsa-mir-192-5p, hsa-mir-215-5p, hsa-mir-92a-3p, hsa-mir-193b-3p, hsa-let-7e-5p, hsa-mir-1283, hsa-mir-218-5p, hsa-mir-1-3p and hsa-mir-671-5p (Supplementary Table 6).
Figure 7. The regulatory network of TFs-gene. The circles represent hub gene, and transcription factor was represented by quadrilateral shape. The network contains 38 nodes and 75 edges.
Figure 8. The regulatory network of miRNAs-gene. The pink circle represents the hub gene and the green triangle represents the miRNA. The network contains 243 nodes and 256 edges.
To search for potential drugs to treat COVID-19 and GC, possible drug molecules were predicted based on the transcriptional characteristics from the DSigDB database (45). The top 8 compounds were identified according to their P-values (Table 4). The potential drug compounds were ciclopirox, resveratrol, etoposide, methotrexate, trifluridine, enterolactone, troglitazone, calcitriol, dasatinib and deferoxamine. These drugs have the possibility to be used as treatment for GC and COVID-19.
Different diseases can generally be considered to be associated with each other if they have one or more similar genes. With the DisGeNET database, Network-Analyst was used to analyze gene-disease associations (Figure 9). Network-Analyst further revealed the stomach neoplasms, colonic neoplasms, autosomal recessive predisposition, liver cirrhosis experimental, mammary neoplasms, neoplasm metastasis, and prostatic neoplasms to be most associated with the identified COVID-19/GC-related common DEGs.
Figure 9. The Gene-disease association network. The square nodes represent diseases and the round nodes represent DEGs.
A strong correlation between COVID-19 and GC has been reported (24–26), with GC patients being more susceptible to viral infection post-surgery, chemotherapy, or radiotherapy. Once infected, the disease progression in GC patients is often more rapid and severe, leading to higher mortality rates. This study aims to explore the molecular mechanisms underlying this correlation to potentially inform new therapeutic strategies.
Here, we identified 209 common DEGs between COVID-19 and GC and explored the biological function of shared DEGs in the pathogenesis of COVID-19 and GC. Notably, these common DEGs are significantly enriched in many immune-related pathways. Alterations in neutrophil number and function have been identified as one of the immunopathological markers associated with severe COVID-19 (46). Studies have shown that neutrophils in healthy individuals can become dysfunctional in degranulation due to factors secreted by epithelial cells which were infected by SARS-CoV-2 (47). Also, neutrophils play an important role in tumor progression and metastasis (48). Cytokine activity was affected by SARS-CoV-2 infection. Impaired acquired immune responses and uncontrolled inflammatory innate responses to SARS-CoV-2 may lead to cytokine storms (49). The occurrence and development of tumors are actually closely related to the immune system, and cancer patients generally have immune dysfunction and low resistance. At the same time, in the process of tumor treatment, including surgery, chemotherapy and radiotherapy, it has a great impact on the body, which will have a certain impact on the patient’s immune system. Targeting immune-related pathways offers a promising avenue for the development of therapeutic strategies against both COVID-19 and gastric cancer.
The common DEGs are utilized to construct the PPI network, in which the hub gene is the most significant regulator in the common pathogenetic processes of GC and COVID-19. CDK1 is an important regulator of cell cycle at G1/S and G2/M checkpoints (50). It has been reported that CDK1 is highly expressed in gastric cancer. Phosphorylation of islet-1 serine 269 by CDK1 can increase its transcriptional activity and promote the proliferation of gastric cancer cells (51), and inhibition of CDK1 can inhibit the proliferation, migration and invasion of GC cells (52). CDK1 is highly expressed in PBMCs of COVID-19 patients and is involved in the apoptosis process; CDK1 may also be associated with a worsening of the course of COVID-19, which is characterized by an extreme decrease in immune cells (53). KIF20A (also known as mitotic kinesin-like protein 2, MKlp2) transports chromosomes during mitosis and plays a key role in cell division. KIF20A is highly expressed in almost all cancers, including gastric cancer (54), melanoma (55), hepatocellular carcinoma (56), and breast cancer (57). Several studies have also shown that KIF20A is a hub gene involved in SARS-CoV-2 infection (58, 59). TPX2 is a microtubule-associated protein that activates the cell cycle kinase protein Aurora-A, which then plays an vital role in spindle formation in mitosis (60), and high TPX2 expression is associated with tumor progression and low survival in gastric cancer (61). TPX2 may be a novel COVID-19 intervention target and biomarker (62). Overexpression of UBE2C is associated with poor prognosis of patients with gastric cancer, and it is also a potential biomarker for intestinal-type gastric cancer (63). A study of peripheral blood transcriptome sequencing in patients with pneumonia found that the expression of UBE2C in patients with severe pneumonia was higher than that in patients with mild pneumonia (64). HJURP (65), CENPA (66), PLK1 (67) and IFI6 (68) were found to significantly increase in gastric cancer tissues compared with normal tissues. In addition, HJURP (69), PLK1 (58), MKI67 (70) and IFI6 (71) have been identified as potential therapeutic target for COVID-19 patients. IFIT2 have been proved to have important roles in regulating apoptosis. Chen et al. (72) showed that decreased expression of IFIT2 promotes gastric cancer progression and predicts poor patient prognosis. Similarly, the significant downregulation of IFIT2 has been observed in patients with severe COVID-19 (73). These findings suggest that targeting these hub genes could be a promising therapeutic strategy in managing both diseases.
In this study, we identified a variety of compounds and medications that may treat COVID-19 and GC, including ciclopirox, resveratrol, etoposide, methotrexate, trifluridine, enterolactone, troglitazone, calcitriol, dasatinib and deferoxamine. Ciclopirox is an antifungal drug that was recently identified as a promising cancer treatment (74). Ciclopirox regulates the growth and autophagic cell death of GC cells by regulating the phosphorylation of STAT3 at Tyr705 and Ser727 residues, and suggests that ciclopirox may be a potential treatment for GC (75). Consistent with this study, Zhang et al. (76) identified ciclopirox as a potential therapeutic agent for the treatment of patients with SARS-CoV-2 infection through drug prediction and simulated docking patterns. Resveratrol is considered an anti-inflammatory and antiviral agent. Resveratrol inhibits the progression of gastric cancer by anti-inflammatory, antioxidant (77), antibacterial (78), inducing cell cycle arrest (79), promoting apoptosis (80), and inhibiting proliferation (81). Besides, resveratrol downregulates neutrophil extracellular traps (NETs) generation by neutrophils in patients with severe COVID-19 (82). Similarly, network pharmacology has shown that resveratrol can alleviate COVID-19-related hyperinflammation (83). Etoposide is a class of anticancer drugs (84). Etoposide induces cell death through the mitochondria-dependent effects of p53 (85). The interaction of etoposide with pertuzumab or trastuzumab induces programmed cell death in gastric cancer cells through exogenous and endogenous apoptotic pathways (86). Besides, etoposide may be a very effective treatment to protect critically ill patients from death caused by a storm of COVID-19-specific cytokines (87). Methotrexate is a tightly bound dihydrofolate reductase (DHFR) inhibitor that is used both as an antineoplastic agent and as an immunosuppressant (88). Clinical and experimental data suggest that methotrexate has a protective effect on SARS-CoV-2 infection by downregulating ACE2 (89). Trifluridine/tipiracil could be a new treatment option for patients with heavily pretreated advanced gastric cancer after progression on, or intolerance to, two or more previous lines of chemotherapy, including a fluoropyrimidine, a platinum agent, a taxane or irinotecan (or both), and an anti-HER2 therapy (in patients with HER2-positive disease) (90). Similarly, trifluridine is considered to have good anti-SARS-CoV-2 capability by virtual screening, ADME/T, and binding free energy analysis (91, 92). Enterolactone is a bioactive phenolic metabolite known as mammalian lignans derived from dietary lignans (93). Enterolactone has potent anti-cancer and/or protective properties against different cancers, including gastric (94), breast (95), colorectal (96), lung (97), ovarian, endometrial (98), and hepatocellular carcinoma (99). In another bioinformatics and systems biology analysis, enterolactone was similarly identified as a potential treatment for COVID-19 (70). Troglitazone induces apoptosis in gastric cancer cells through the NAG-1 pathway (100). In addition, troglitazone has been identified as a potential inhibitor of SARS-CoV-2 replicase (101). Calcitriol alleviates COVID-19 complications by modulating pro-inflammatory cytokines, antiviral proteins, and autophagy (102). Similarly, there was an improvement in peripheral arterial oxygen saturation and inspired oxygen fraction in hospitalized patients with COVID-19 treated with calcitriol (103). Dasatinib promotes TRAIL-mediated apoptosis by upregulating CHOP-dependent death receptor 5 in gastric cancer (104). Dasatinib can reduce SARS-CoV-2-related mortality, delay its onset, and reduce the number of other clinical symptoms (105). Deferoxamine is a widely used iron chelator used to treat iron overload. Deferoxamine targets mitochondria and impair mitochondrial respiration and [Fe-S] cluster/heme biogenesis in cancer cells, thereby inhibiting tumor proliferation and migration and inducing cell death (106). Deferoxamine has iron chelation, antiviral, and immunomodulatory effects to help control SARS-CoV-2 (107).
This study explores the relationship between COVID-19 and GC using bioinformatics and systems biology approaches; however, several limitations should be acknowledged. First, our analysis relies on data retrieved from specific public databases, which may introduce biases related to sample selection, data collection methods, and population differences. Second, the gene expression data used in this study may be subject to methodological biases, including batch effects and variations in experimental conditions across datasets. Although we identified several hub genes and pathways potentially linking COVID-19 and GC, our study is based on computational predictions. Experimental validation through in vitro and in vivo studies is necessary to confirm the biological significance and therapeutic relevance of the identified targets. Additionally, translating these findings into clinical practice presents significant challenges, requiring further research to ensure these insights can be effectively applied in therapeutic settings.
In this study, transcriptome analysis was applied to summarize the relationship between gastric cancer and COVID-19. DEGs for GC and COVID-19 were obtained in the GEO dataset, 209 shared DEGs were identified, and associations between gastric cancer and COVID-19 were found. To clarify what role these DEGs play at the transcriptional level, enrichment analysis was conducted. We also used these common DEGs to obtain a PPI network and defined the 10 most important hub genes: CDK1, KIF20A, TPX2, UBE2C, HJURP, CENPA, PLK1, MKI67, IFI6, and IFIT2. Besides, we established a TF-gene and miRNA-gene interaction network for hub genes and identified key TFs and miRNAs. More importantly, we identified a variety of compounds and drugs that may treat COVID-19 and GC, such as ciclopirox, resveratrol, etoposide, methotrexate, trifluridine, enterolactone, troglitazone, calcitriol, dasatinib and deferoxamine. This study shows new possibilities for the treatment of COVID-19 and GC.
The original contributions presented in this study are included in this article/Supplementary material, further inquiries can be directed to the corresponding authors.
XM: Formal analysis, Resources, Writing – original draft. TH: Project administration, Validation, Writing – original draft, Writing – review & editing. XL: Methodology, Software, Writing – original draft. XZ: Data curation, Methodology, Supervision, Writing – review & editing. HP: Data curation, Formal analysis, Methodology, Visualization, Writing – original draft. AD: Investigation, Writing – review & editing. YZ: Funding acquisition, Visualization, Writing – review & editing. KY: Funding acquisition, Project administration, Writing – review & editing. ZW: Funding acquisition, Resources, Writing – review & editing.
The authors declare that financial support was received for the research, authorship, and/or publication of this article. This work was supported by grants from the Natural Science Foundation of China (82472700, 82472745, 82472365, 82373400, 82372660, 82272685, 82202260 and 82173248), the Science and Technology Major Program of Sichuan Province (2022ZDZX0019), the Sichuan Science and Technology Program (2024NSFSC0645, 2024NSFSC1894, 2023YFS0128, 2023NSFSC1874), the Project funded by China Postdoctoral Science Foundation (2022TQ0221), 1.3.5 project for disciplines of excellence, West China Hospital, Sichuan University (ZYGD22006), the Sichuan University postdoctoral interdisciplinary Innovation Fund (10822041A2103).
We are most grateful for GEO databases providing their platform and thanking contributors who have uploaded their valuable datasets. Additionally, we appreciated Core Facility of West China Hospital for their technique support. Also, the graphic abstract of the overall general workflow for this study was created with BioRender.com.
The authors declare that the research was conducted in the absence of any commercial or financial relationships that could be construed as a potential conflict of interest.
All claims expressed in this article are solely those of the authors and do not necessarily represent those of their affiliated organizations, or those of the publisher, the editors and the reviewers. Any product that may be evaluated in this article, or claim that may be made by its manufacturer, is not guaranteed or endorsed by the publisher.
The Supplementary Material for this article can be found online at: https://www.frontiersin.org/articles/10.3389/fmed.2024.1428973/full#supplementary-material
Supplementary Table 1 | The differentially expressed genes of COVID-19.
Supplementary Table 2 | The differentially expressed genes of GC.
Supplementary Table 3 | Common DEGs between COVID-19 and GC.
Supplementary Table 4 | Hub genes in network string_interactions ranked by MCC method.
Supplementary Table 5 | The TFs and their interactions with hub genes.
Supplementary Table 6 | The microRNAs and their interactions with hub genes.
1. Huang T, He J, Zhou X, Pan H, He F, Du A, et al. Discovering common pathogenetic processes between covid-19 and tuberculosis by bioinformatics and system biology approach. Front Cell Infect Microbiol. (2023) 13:1280223. doi: 10.3389/fcimb.2023.1280223
2. Lino A, Cardoso M, Martins-Lopes P, Gonçalves H. Omicron – the new SARS-Cov-2 challenge? Rev Med Virol. (2022) 32:e2358. doi: 10.1002/rmv.2358
3. Mittal A, Khattri A, Verma V. Structural and antigenic variations in the spike protein of emerging SARS-Cov-2 variants. PLoS Pathog. (2022) 18:e1010260. doi: 10.1371/journal.ppat.1010260
4. Mason R. Pathogenesis of covid-19 from a cell biology perspective. Eur Respir J. (2020) 55:2000607. doi: 10.1183/13993003.00607-2020
5. Hoffmann M, Kleine-Weber H, Schroeder S, Krüger N, Herrler T, Erichsen S, et al. SARS-Cov-2 cell entry depends on ace2 and tmprss2 and is blocked by a clinically proven protease inhibitor. Cell. (2020) 181:271–80. doi: 10.1016/j.cell.2020.02.052
6. Li D, Liu X, Zhang L, He J, Chen X, Liu S, et al. Covid-19 disease and malignant cancers: The impact for the furin gene expression in susceptibility to SARS-Cov-2. Int J Biol Sci. (2021) 17:3954–67. doi: 10.7150/ijbs.63072
7. Allam L, Ghrifi F, Mohammed H, El H, El J, El H, et al. Targeting the grp78-dependant SARS-cov-2 cell entry by peptides and small molecules. Bioinform Biol Insights. (2020) 14:1214668159. doi: 10.1177/1177932220965505
8. Strollo R, Pozzilli P. Dpp4 inhibition: Preventing SARS-Cov-2 infection and/or progression of covid-19? Diabetes Metab Res Rev. (2020) 36:e3330. doi: 10.1002/dmrr.3330
9. Behl T, Kaur I, Aleya L, Sehgal A, Singh S, Sharma N, et al. Cd147-spike protein interaction in covid-19: Get the ball rolling with a novel receptor and therapeutic target. Sci Total Environ. (2022) 808:152072. doi: 10.1016/j.scitotenv.2021.152072
10. Wang S, Qiu Z, Hou Y, Deng X, Xu W, Zheng T, et al. Axl is a candidate receptor for SARS-cov-2 that promotes infection of pulmonary and bronchial epithelial cells. Cell Res. (2021) 31:126–40. doi: 10.1038/s41422-020-00460-y
11. Ou X, Liu Y, Lei X, Li P, Mi D, Ren L, et al. Characterization of spike glycoprotein of SARS-cov-2 on virus entry and its immune cross-reactivity with SARS-cov. Nat Commun. (2020) 11:1620. doi: 10.1038/s41467-020-15562-9
12. Cao X. Covid-19: Immunopathology and its implications for therapy. Nat Rev Immunol. (2020) 20:269–70. doi: 10.1038/s41577-020-0308-3
13. Yang L, Liu S, Liu J, Zhang Z, Wan X, Huang B, et al. Covid-19: Immunopathogenesis and immunotherapeutics. Signal Transduct Target Ther. (2020) 5:128. doi: 10.1038/s41392-020-00243-2
14. Teuwen L, Geldhof V, Pasut A, Carmeliet P. Covid-19: The vasculature unleashed. Nat Rev Immunol. (2020) 20:389–91. doi: 10.1038/s41577-020-0343-0
15. Zaim S, Chong J, Sankaranarayanan V, Harky A. Covid-19 and multiorgan response. Curr Probl Cardiol. (2020) 45:100618. doi: 10.1016/j.cpcardiol.2020.100618
16. Song Y, Huang T, Pan H, Du A, Wu T, Lan J, et al. The influence of covid-19 on colorectal cancer was investigated using bioinformatics and systems biology techniques. Front Med (Lausanne). (2023) 10:1169562. doi: 10.3389/fmed.2023.1169562
17. Huang T, Yu B, Zhou X, Pan H, Du A, Bai J, et al. Exploration of the link between covid-19 and alcoholic hepatitis from the perspective of bioinformatics and systems biology. Medcomm Future Med. (2023) 2:e42. doi: 10.1002/mef2.42
18. Huang T, Zheng D, Song Y, Pan H, Qiu G, Xiang Y, et al. Demonstration of the impact of covid-19 on metabolic associated fatty liver disease by bioinformatics and system biology approach. Medicine (Baltimore). (2023) 102:e34570. doi: 10.1097/MD.0000000000034570
19. Huang T, Jiang N, Song Y, Pan H, Du A, Yu B, et al. Bioinformatics and system biology approach to identify the influences of SARS-cov-2 on metabolic unhealthy obese patients. Front Mol Biosci. (2023) 10:1274463. doi: 10.3389/fmolb.2023.1274463
20. Zhu N, Zhang D, Wang W, Li X, Yang B, Song J, et al. A novel coronavirus from patients with pneumonia in China, 2019. N Engl J Med. (2020) 382:727–33. doi: 10.1056/NEJMoa2001017
21. Zhou F, Yu T, Du R, Fan G, Liu Y, Liu Z, et al. Clinical course and risk factors for mortality of adult inpatients with covid-19 in Wuhan, China: A retrospective cohort study. Lancet. (2020) 395:1054–62. doi: 10.1016/S0140-6736(20)30566-3
22. Sung H, Ferlay J, Siegel R, Laversanne M, Soerjomataram I, Jemal A, et al. Global cancer statistics 2020: Globocan estimates of incidence and mortality worldwide for 36 cancers in 185 countries. Ca Cancer J Clin. (2021) 71:209–49. doi: 10.3322/caac.21660
23. Jemal A, Bray F, Center M, Ferlay J, Ward E, Forman D. Global cancer statistics. Ca Cancer J Clin. (2011) 61:69–90. doi: 10.3322/caac.20107
24. Martin S, Kaeuffer C, Leyendecker P, Tuzin N, Tazi Y, Schaff-Wendling F, et al. Covid-19 in patients with cancer: A retrospective study of 212 cases from a French SARS-cov-2 cluster during the first wave of the covid-19 pandemic. Oncologist. (2021) 26:e1656–9. doi: 10.1002/onco.13831
25. An X, Lin W, Liu H, Zhong W, Zhang X, Zhu Y, et al. SARS-Cov-2 host receptor ace2 protein expression atlas in human gastrointestinal tract. Front Cell Dev Biol. (2021) 9:659809. doi: 10.3389/fcell.2021.659809
26. Li J, Bai H, Qiao H, Du C, Yao P, Zhang Y, et al. Causal effects of covid-19 on cancer risk: A Mendelian randomization study. J Med Virol. (2023) 95:e28722. doi: 10.1002/jmv.28722
27. Banerjee U, Chunchanur S, Balaji KN, Singh A, Chakravortty D, et al. Systems-level profiling of early peripheral host-response landscape variations across covid-19 severity states in an Indian cohort. Genes Immun. (2023) 24:183–93. doi: 10.1038/s41435-023-00210-1
28. Lou S, Wang Y, Zhang J, Yin X, Zhang Y, Wang Y, et al. Patient-level DNA damage repair pathway profiles and anti-tumor immunity for gastric cancer. Front Immunol. (2021) 12:806324. doi: 10.3389/fimmu.2021.806324
29. Love M, Huber W, Anders S. Moderated estimation of fold change and dispersion for rna-seq data with deseq2. Genome Biol. (2014) 15:550. doi: 10.1186/s13059-014-0550-8
30. Bardou P, Mariette J, Escudié F, Djemiel C, Klopp C. Jvenn: An interactive venn diagram viewer. BMC Bioinformatics. (2014) 15:293. doi: 10.1186/1471-2105-15-293
31. Thomas P, Hill D, Mi H, Osumi-Sutherland D, Van Auken K, Carbon S, et al. Gene ontology causal activity modeling (go-cam) moves beyond go annotations to structured descriptions of biological functions and systems. Nat Genet. (2019) 51:1429–33. doi: 10.1038/s41588-019-0500-1
32. The Gene Ontology Consortium. Expansion of the gene ontology knowledgebase and resources. Nucleic Acids Res. (2017) 45:D331–8. doi: 10.1093/nar/gkw1108
33. Chen E, Tan C, Kou Y, Duan Q, Wang Z, Meirelles G, et al. Enrichr: Interactive and collaborative html5 gene list enrichment analysis tool. BMC Bioinform. (2013) 14:128. doi: 10.1186/1471-2105-14-128
34. Szklarczyk D, Gable A, Lyon D, Junge A, Wyder S, Huerta-Cepas J, et al. String v11: Protein-protein association networks with increased coverage, supporting functional discovery in genome-wide experimental datasets. Nucleic Acids Res. (2019) 47:D607–13. doi: 10.1093/nar/gky1131
35. Shannon P, Markiel A, Ozier O, Baliga N, Wang J, Ramage D, et al. Cytoscape: A software environment for integrated models of biomolecular interaction networks. Genome Res. (2003) 13:2498–504. doi: 10.1101/gr.1239303
36. Chin C, Chen S, Wu H, Ho C, Ko M, Lin C. Cytohubba: Identifying hub objects and sub-networks from complex interactome. BMC Syst Biol. (2014) 8:S11. doi: 10.1186/1752-0509-8-S4-S11
37. Lambert S, Jolma A, Campitelli L, Das P, Yin Y, Albu M, et al. The human transcription factors. Cell. (2018) 172:650–65. doi: 10.1016/j.cell.2018.01.029
38. Zhou G, Soufan O, Ewald J, Hancock R, Basu N, Xia J. Networkanalyst 3.0: A visual analytics platform for comprehensive gene expression profiling and meta-analysis. Nucleic Acids Res. (2019) 47:W234–41. doi: 10.1093/nar/gkz240
39. Castro-Mondragon J, Riudavets-Puig R, Rauluseviciute I, Lemma R, Turchi L, Blanc-Mathieu R, et al. Jaspar 2022: The 9th release of the open-access database of transcription factor binding profiles. Nucleic Acids Res. (2022) 50:D165–73. doi: 10.1093/nar/gkab1113
40. Karagkouni D, Paraskevopoulou M, Chatzopoulos S, Vlachos I, Tastsoglou S, Kanellos I, et al. Diana-tarbase v8: A decade-long collection of experimentally supported miRNA-gene interactions. Nucleic Acids Res. (2018) 46:D239–45. doi: 10.1093/nar/gkx1141
41. Yoo M, Shin J, Kim J, Ryall K, Lee K, Lee S, et al. Dsigdb: Drug signatures database for gene set analysis. Bioinformatics. (2015) 31:3069–71. doi: 10.1093/bioinformatics/btv313
42. Piñero J, Ramírez-Anguita J, Saüch-Pitarch J, Ronzano F, Centeno E, Sanz F, et al. The disgenet knowledge platform for disease genomics: 2019 update. Nucleic Acids Res. (2020) 48:D845–55. doi: 10.1093/nar/gkz1021
43. Wittig U, De Beuckelaer A. Analysis and comparison of metabolic pathway databases. Brief Bioinform. (2001) 2:126–42. doi: 10.1093/bib/2.2.126
44. Chen B, Fan W, Liu J, Wu F. Identifying protein complexes and functional modules–from static PPI networks to dynamic PPI networks. Brief Bioinform. (2014) 15:177–94. doi: 10.1093/bib/bbt039
45. Mahmud S, Chen W, Meng H, Jahan H, Liu Y, Hasan S. Prediction of drug-target interaction based on protein features using undersampling and feature selection techniques with boosting. Anal Biochem. (2020) 589:113507. doi: 10.1016/j.ab.2019.113507
46. Reusch N, De Domenico E, Bonaguro L, Schulte-Schrepping J, Baßler K, Schultze J, et al. Neutrophils in covid-19. Front Immunol. (2021) 12:652470. doi: 10.3389/fimmu.2021.652470
47. Muralidharan A, Wyatt T, Reid SP. SARS-Cov-2 dysregulates neutrophil degranulation and reduces lymphocyte counts. Biomedicines. (2022) 10:382. doi: 10.3390/biomedicines10020382
48. Lee W, Ko S, Mohamed M, Kenny H, Lengyel E, Naora H. Neutrophils facilitate ovarian cancer premetastatic niche formation in the Omentum. J Exp Med. (2019) 216:176–94. doi: 10.1084/jem.20181170
49. Hu B, Huang S, Yin L. The cytokine storm and covid-19. J Med Virol. (2021) 93:250–6. doi: 10.1002/jmv.26232
50. Syn N, Lim P, Kong L, Wang L, Wong A, Lim C, et al. Pan-cdk inhibition augments cisplatin lethality in nasopharyngeal carcinoma cell lines and xenograft models. Signal Transduct Target Ther. (2018) 3:9. doi: 10.1038/s41392-018-0010-0
51. Shi Q, Ni X, Lei M, Xia Q, Dong Y, Zhang Q, et al. Phosphorylation of islet-1 serine 269 by cdk1 increases its transcriptional activity and promotes cell proliferation in gastric cancer. Mol Med. (2021) 27:47. doi: 10.1186/s10020-021-00302-6
52. Huang Z, Zhang S, Du J, Zhang X, Zhang W, Huang Z, et al. Cyclin-dependent kinase 1 (cdk1) is co-expressed with cdca5: Their functions in gastric cancer cell line mgc-803. Med Sci Monit. (2020) 26:e923664. doi: 10.12659/MSM.923664
53. Xiong Y, Liu Y, Cao L, Wang D, Guo M, Jiang A, et al. Transcriptomic characteristics of bronchoalveolar lavage fluid and peripheral blood mononuclear cells in covid-19 patients. Emerg Microbes Infect. (2020) 9:761–70. doi: 10.1080/22221751.2020.1747363
54. Sheng Y, Wang W, Hong B, Jiang X, Sun R, Yan Q, et al. Upregulation of kif20a correlates with poor prognosis in gastric cancer. Cancer Manag Res. (2018) 10:6205–16. doi: 10.2147/CMAR.S176147
55. Jiang J, Liu C, Xu G, Liang T, Yu C, Liao S, et al. Identification of hub genes associated with melanoma development by comprehensive bioinformatics analysis. Front Oncol. (2021) 11:621430. doi: 10.3389/fonc.2021.621430
56. Shi C, Huang D, Lu N, Chen D, Zhang M, Yan Y, et al. Aberrantly activated gli2-kif20a axis is crucial for growth of hepatocellular carcinoma and predicts poor prognosis. Oncotarget. (2016) 7:26206–19. doi: 10.18632/oncotarget.8441
57. Nakamura M, Takano A, Thang P, Tsevegjav B, Zhu M, Yokose T, et al. Characterization of kif20a as a prognostic biomarker and therapeutic target for different subtypes of breast cancer. Int J Oncol. (2020) 57:277–88. doi: 10.3892/ijo.2020.5060
58. Chen J, Xie T, Lin Z, Li Y, Xie Y, Li Z, et al. Identification of key pathways and genes in SARS-Cov-2 infecting human intestines by bioinformatics analysis. Biochem Genet. (2022) 60:1076–94. doi: 10.1007/s10528-021-10144-w
59. Zhang F, Yu C, Xu W, Li X, Feng J, Shi H, et al. Identification of critical genes and molecular pathways in covid-19 myocarditis and constructing gene regulatory networks by bioinformatic analysis. PLoS One. (2022) 17:e0269386. doi: 10.1371/journal.pone.0269386
60. Ozlü N, Srayko M, Kinoshita K, Habermann B, O’Toole E, Müller-Reichert T, et al. An essential function of the c. Elegans ortholog of tpx2 is to localize activated aurora a kinase to mitotic spindles. Dev Cell. (2005) 9:237–48. doi: 10.1016/j.devcel.2005.07.002
61. Tomii C, Inokuchi M, Takagi Y, Ishikawa T, Otsuki S, Uetake H, et al. Tpx2 expression is associated with poor survival in gastric cancer. World J Surg Oncol. (2017) 15:14. doi: 10.1186/s12957-016-1095-y
62. Li J, Ren J, Liao H, Guo W, Feng K, Huang T, et al. Identification of dynamic gene expression profiles during sequential vaccination with chadox1/bnt162b2 using machine learning methods. Front Microbiol. (2023) 14:1138674. doi: 10.3389/fmicb.2023.1138674
63. Zhang J, Liu X, Yu G, Liu L, Wang J, Chen X, et al. Ube2c is a potential biomarker of intestinal-type gastric cancer with chromosomal instability. Front Pharmacol. (2018) 9:847. doi: 10.3389/fphar.2018.00847
64. Huang S, Feng C, Chen L, Huang Z, Zhou X, Li B, et al. Molecular mechanisms of mild and severe pneumonia: Insights from RNA sequencing. Med Sci Monit. (2017) 23:1662–73. doi: 10.12659/msm.900782
65. Wang J, Liu X, Chu H, Li N, Huang L, Chen J. Centromere protein i (cenp-i) is upregulated in gastric cancer, predicts poor prognosis, and promotes tumor cell proliferation and migration. Technol Cancer Res Treat. (2021) 20:2091179898. doi: 10.1177/15330338211045510
66. Xu Y, Liang C, Cai X, Zhang M, Yu W, Shao Q. High centromere protein-a (cenp-a) expression correlates with progression and prognosis in gastric cancer. Onco Targets Ther. (2020) 13:13237–46. doi: 10.2147/OTT.S263512
67. Huang J, Wen F, Huang W, Bai Y, Lu X, Shu P. Identification of hub genes and discovery of promising compounds in gastric cancer based on bioinformatics analysis. Biomark Med. (2020) 14:1069–84. doi: 10.2217/bmm-2019-0608
68. Liu Z, Gu S, Lu T, Wu K, Li L, Dong C, et al. Ifi6 depletion inhibits esophageal squamous cell carcinoma progression through reactive oxygen species accumulation via mitochondrial dysfunction and endoplasmic reticulum stress. J Exp Clin Cancer Res. (2020) 39:144. doi: 10.1186/s13046-020-01646-3
69. Hasan M, Rahman M, Islam M, Islam M, Hossain M, Moni M. Systems biology and bioinformatics approach to identify blood based signatures molecules and drug targets of patient with covid-19. Inform Med Unlocked. (2022) 28:100840. doi: 10.1016/j.imu.2021.100840
70. Yan C, Niu Y, Wang X. Blood transcriptome analysis revealed the crosstalk between covid-19 and HIV. Front Immunol. (2022) 13:1008653. doi: 10.3389/fimmu.2022.1008653
71. Villamayor L, Rivero V, López-García D, Topham D, Martínez-Sobrido L, Nogales A, et al. Interferon alpha inducible protein 6 is a negative regulator of innate immune responses by modulating rig-i activation. Front Immunol. (2023) 14:1105309. doi: 10.3389/fimmu.2023.1105309
72. Chen L, Zhai W, Zheng X, Xie Q, Zhou Q, Tao M, et al. Decreased ifit2 expression promotes gastric cancer progression and predicts poor prognosis of the patients. Cell Physiol Biochem. (2018) 45:15–25. doi: 10.1159/000486219
73. Franco J, Chattopadhyay S, Pan Z. How different pathologies are affected by Ifit expression. Viruses. (2023) 15:342. doi: 10.3390/v15020342
74. Fan H, He Y, Xiang J, Zhou J, Wan X, You J, et al. Ros generation attenuates the anti-cancer effect of cpx on cervical cancer cells by inducing autophagy and inhibiting glycophagy. Redox Biol. (2022) 53:102339. doi: 10.1016/j.redox.2022.102339
75. Chen L, Chen D, Li J, He L, Chen T, Song D, et al. Ciclopirox drives growth arrest and autophagic cell death through stat3 in gastric cancer cells. Cell Death Dis. (2022) 13:1007. doi: 10.1038/s41419-022-05456-7
76. Zhang Y, Duan Z, Guan Y, Xu T, Fu Y, Li G. Identification of 3 key genes as novel diagnostic and therapeutic targets for OA and covid-19. Front Immunol. (2023) 14:1167639. doi: 10.3389/fimmu.2023.1167639
77. Zaidi S, Ahmed K, Yamamoto T, Kondo T, Usmanghani K, Kadowaki M, et al. Effect of resveratrol on Helicobacter pylori-induced interleukin-8 secretion, reactive oxygen species generation and morphological changes in human gastric epithelial cells. Biol Pharm Bull. (2009) 32:1931–5. doi: 10.1248/bpb.32.1931
78. Martini S, Bonechi C, Rossi C, Figura N. Increased susceptibility to resveratrol of Helicobacter pylori strains isolated from patients with gastric carcinoma. J Nat Prod. (2011) 74:2257–60. doi: 10.1021/np100761u
79. Atten M, Godoy-Romero E, Attar B, Milson T, Zopel M, Holian O. Resveratrol regulates cellular PKC alpha and delta to inhibit growth and induce apoptosis in gastric cancer cells. Invest New Drugs. (2005) 23:111–9. doi: 10.1007/s10637-005-5855-8
80. Riles W, Erickson J, Nayyar S, Atten M, Attar B, Holian O. Resveratrol engages selective apoptotic signals in gastric adenocarcinoma cells. World J Gastroenterol. (2006) 12:5628–34. doi: 10.3748/wjg.v12.i35.5628
81. Aquilano K, Baldelli S, Rotilio G, Ciriolo M. Trans-resveratrol inhibits h2o2-induced adenocarcinoma gastric cells proliferation via inactivation of mek1/2-erk1/2-c-jun signalling axis. Biochem Pharmacol. (2009) 77:337–47. doi: 10.1016/j.bcp.2008.10.034
82. de Souza A, Leal V, Fernandes I, Gozzi-Silva S, Beserra D, Oliveira E, et al. Resveratrol downmodulates neutrophil extracellular trap (net) generation by neutrophils in patients with severe covid-19. Antioxidants (Basel). (2022) 11:1690. doi: 10.3390/antiox11091690
83. Xiao Z, Ye Q, Duan X, Xiang T. Network pharmacology reveals that resveratrol can alleviate covid-19-related hyperinflammation. Dis Markers. (2021) 2021:4129993. doi: 10.1155/2021/4129993
84. Burden D, Kingma P, Froelich-Ammon S, Bjornsti M, Patchan M, Thompson R, et al. Topoisomerase ii. etoposide interactions direct the formation of drug-induced enzyme-DNA cleavage complexes. J Biol Chem. (1996) 271:29238–44. doi: 10.1074/jbc.271.46.29238
85. Jamil S, Lam I, Majd M, Tsai S, Duronio V. Etoposide induces cell death via mitochondrial-dependent actions of p53. Cancer Cell Int. (2015) 15:79. doi: 10.1186/s12935-015-0231-z
86. Gornowicz A, Szymanowski W, Czarnomysy R, Bielawski K, Bielawska A. Anti-her2 monoclonal antibodies intensify the susceptibility of human gastric cancer cells to etoposide by promoting apoptosis, but not autophagy. PLoS One. (2021) 16:e0255585. doi: 10.1371/journal.pone.0255585
87. Lovetrue B. The ai-discovered aetiology of covid-19 and rationale of the irinotecan+ etoposide combination therapy for critically ill covid-19 patients. Med Hypotheses. (2020) 144:110180. doi: 10.1016/j.mehy.2020.110180
88. Rana S, Dranchak P, Dahlin J, Lamy L, Li W, Oliphant E, et al. Methotrexate-based protacs as dhfr-specific chemical probes. Cell Chem Biol. (2024) 31:221–33. doi: 10.1016/j.chembiol.2023.09.014
89. Schälter F, Dürholz K, Bucci L, Burmester G, Caporali R, Figuereido C, et al. Does methotrexate influence covid-19 infection? Case series and mechanistic data. Arthritis Res Ther. (2021) 23:166. doi: 10.1186/s13075-021-02464-4
90. Shitara K, Doi T, Dvorkin M, Mansoor W, Arkenau H, Prokharau A, et al. Trifluridine/tipiracil versus placebo in patients with heavily pretreated metastatic gastric cancer (tags): A randomised, double-blind, placebo-controlled, phase 3 trial. Lancet Oncol. (2018) 19:1437–48. doi: 10.1016/S1470-2045(18)30739-3
91. Maurya S, Maurya A, Mishra N, Siddique H. Virtual screening, adme/t, and binding free energy analysis of anti-viral, anti-protease, and anti-infectious compounds against nsp10/nsp16 methyltransferase and main protease of SARS cov-2. J Recept Signal Transduct Res. (2020) 40:605–12. doi: 10.1080/10799893.2020.1772298
92. Elzupir A. Molecular docking and dynamics investigations for identifying potential inhibitors of the 3-chymotrypsin-like protease of SARS-cov-2: Repurposing of approved pyrimidonic pharmaceuticals for covid-19 treatment. Molecules. (2021) 26:7458. doi: 10.3390/molecules26247458
93. Clavel T, Henderson G, Engst W, Doré J, Blaut M. Phylogeny of human intestinal bacteria that activate the dietary lignan secoisolariciresinol diglucoside. FEMS Microbiol Ecol. (2006) 55:471–8. doi: 10.1111/j.1574-6941.2005.00057.x
94. Yang J, Cho L, Ko K, Shin A, Ma S, Choi B, et al. Genetic susceptibility on caga-interacting molecules and gene-environment interaction with phytoestrogens: A putative risk factor for gastric cancer. PLoS One. (2012) 7:e31020. doi: 10.1371/journal.pone.0031020
95. Ingram D, Sanders K, Kolybaba M, Lopez D. Case-control study of phyto-oestrogens and breast cancer. Lancet. (1997) 350:990–4. doi: 10.1016/S0140-6736(97)01339-1
96. Kuijsten A, Arts I, Hollman P, Van’T V, Kampman E. Plasma enterolignans are associated with lower colorectal adenoma risk. Cancer Epidemiol Biomark Prev. (2006) 15:1132–6. doi: 10.1158/1055-9965.EPI-05-0991
97. Schabath M, Hernandez L, Wu X, Pillow P, Spitz M. Dietary phytoestrogens and lung cancer risk. JAMA. (2005) 294:1493–504. doi: 10.1001/jama.294.12.1493
98. Aarestrup J, Kyrø C, Knudsen K, Weiderpass E, Christensen J, Kristensen M, et al. Plasma enterolactone and incidence of endometrial cancer in a case-cohort study of Danish women. Br J Nutr. (2013) 109:2269–75. doi: 10.1017/S0007114512004424
99. Miura D, Saarinen N, Miura Y, Santti R, Yagasaki K. Hydroxymatairesinol and its mammalian metabolite enterolactone reduce the growth and metastasis of subcutaneous ah109a hepatomas in rats. Nutr Cancer. (2007) 58:49–59. doi: 10.1080/01635580701308133
100. Wang C, Wang J, Bai P. Troglitazone induces apoptosis in gastric cancer cells through the nag-1 pathway. Mol Med Rep. (2011) 4:93–7. doi: 10.3892/mmr.2010.381
101. Chandra A, Gurjar V, Ahmed M, Alqahtani A, Qamar I, Singh N. Exploring potential inhibitor of SARS-cov2 replicase from FDA approved drugs using insilico drug discovery methods. J Biomol Struct Dyn. (2022) 40:5507–14. doi: 10.1080/07391102.2020.1871416
102. Gilani S, Bin-Jumah M, Nadeem M, Kazmi I. Vitamin d attenuates covid-19 complications via modulation of proinflammatory cytokines, antiviral proteins, and autophagy. Expert Rev Anti Infect Ther. (2022) 20:231–41. doi: 10.1080/14787210.2021.1941871
103. Elamir Y, Amir H, Lim S, Rana Y, Lopez C, Feliciano N, et al. A randomized pilot study using calcitriol in hospitalized covid-19 patients. Bone. (2022) 154:116175. doi: 10.1016/j.bone.2021.116175
104. Wang X, Xue Q, Wu L, Wang B, Liang H. Dasatinib promotes trail-mediated apoptosis by upregulating chop-dependent death receptor 5 in gastric cancer. FEBS Open Bio. (2018) 8:732–42. doi: 10.1002/2211-5463.12404
105. Pastor-Fernández A, Bertos A, Sierra-Ramírez A, Del M, Merino J, de Ávila A, et al. Treatment with the senolytics dasatinib/quercetin reduces SARS-Cov-2-related mortality in mice. Aging Cell. (2023) 22:e13771. doi: 10.1111/acel.13771
106. Sandoval-Acuña C, Torrealba N, Tomkova V, Jadhav S, Blazkova K, Merta L, et al. Targeting mitochondrial iron metabolism suppresses tumor growth and metastasis by inducing mitochondrial dysfunction and mitophagy. Cancer Res. (2021) 81:2289–303. doi: 10.1158/0008-5472.CAN-20-1628
Keywords: bioinformatic analysis, hub genes, gastric cancer, COVID-19, therapeutic drug
Citation: Ma X, Huang T, Li X, Zhou X, Pan H, Du A, Zeng Y, Yuan K and Wang Z (2024) Exploration of the link between COVID-19 and gastric cancer from the perspective of bioinformatics and systems biology. Front. Med. 11:1428973. doi: 10.3389/fmed.2024.1428973
Received: 07 May 2024; Accepted: 04 September 2024;
Published: 20 September 2024.
Edited by:
Alberto Bongiovanni, IRCCS Istituto Romagnolo per lo Studio dei Tumori (IRST) “Dino Amadori”, ItalyReviewed by:
Tuo Shao, Massachusetts General Hospital and Harvard Medical School, United StatesCopyright © 2024 Ma, Huang, Li, Zhou, Pan, Du, Zeng, Yuan and Wang. This is an open-access article distributed under the terms of the Creative Commons Attribution License (CC BY). The use, distribution or reproduction in other forums is permitted, provided the original author(s) and the copyright owner(s) are credited and that the original publication in this journal is cited, in accordance with accepted academic practice. No use, distribution or reproduction is permitted which does not comply with these terms.
*Correspondence: Zhen Wang, d2FuZ3poZW5Ac2N1LmVkdS5jbg==; Kefei Yuan, eWtmMTNAMTYzLmNvbQ==
†These authors have contributed equally to this work
Disclaimer: All claims expressed in this article are solely those of the authors and do not necessarily represent those of their affiliated organizations, or those of the publisher, the editors and the reviewers. Any product that may be evaluated in this article or claim that may be made by its manufacturer is not guaranteed or endorsed by the publisher.
Research integrity at Frontiers
Learn more about the work of our research integrity team to safeguard the quality of each article we publish.