- Sensorimotor Control Lab/Vinjamuri Lab, Department of Computer Science and Electrical Engineering, University of Maryland Baltimore County, Baltimore, MD, United States
Stress has been recognized as a pivotal indicator which can lead to severe mental disorders. Persistent exposure to stress will increase the risk for various physical and mental health problems. Early and reliable detection of stress-related status is critical for promoting wellbeing and developing effective interventions. This study attempted multi-type and multi-level stress detection by fusing features extracted from multiple physiological signals including electroencephalography (EEG) and peripheral physiological signals. Eleven healthy individuals participated in validated stress-inducing protocols designed to induce social and mental stress and discriminant multi-level and multi-type stress. A range of machine learning methods were applied and evaluated on physiological signals of various durations. An average accuracy of 98.1% and 97.8% was achieved in identifying stress type and stress level respectively, using 4-s neurophysiological signals. These findings have promising implications for enhancing the precision and practicality of real-time stress monitoring applications.
1 Introduction
Stress, characterized by anxiety and psychological disruption, triggers the “fight-or-flight” response as a primal survival mechanism, impacting mental and physical wellbeing (Gedam and Paul, 2021). It can significantly impact both mental and physical wellbeing, leading to changes in behavior and physiology. Its effects vary based on individual perspectives and circumstances and if stress persists for a long time, then it will lead to serious diseases. During stressful episodes, the body activates its protective resources, triggering increased heart rate, muscle tension, blood pressure, and heightened senses [2]. All these physiological responses stem from the autonomic nervous system (ANS) and get integrated into the cerebral cortex to be regulated. The brain not only processes inputs from the external environment but also controls adjustments of the body engendered by behavioral states. As reviewed further, these stress-related processes are mediated by and feedback to the brain, impacting its abilities to regulate peripheral physiology, engage in adaptive social and health behaviors, experience and control emotions, and support cognitive functioning. Robust evidence suggests that chronic stress plays a significant role in the onset of severe and impairing psychiatric conditions (Davis et al., 2017), including major depressive disorder, bipolar disorder, and posttraumatic stress disorder, substance use disorder. Studies suggested that addressing stress-related vulnerabilities is crucial for effective treatment (McHugh et al., 2020). Perceived stress levels are also associated with sustained depression, amplifying mood symptoms in individuals with substance use disorders. Additionally, chronic stress in people with disabilities or impairments notably increases the risk of developing substance use disorders (Mills, 2023).
Addressing stress-related vulnerabilities is crucial for effective treatment (McHugh et al., 2020), with perceived stress levels associated with sustained depression and amplified mood symptoms in individuals with substance use disorders. Chronic stress notably heightens the risk of developing substance use disorders in people with disabilities or impairments. The onset of substance use disorder is strongly linked to stress, with the rise in healthcare costs and job losses during the pandemic resulting in drug-related expenditure exceeding $1 trillion in the United States in 2022, as reported by the National Institute on Drug Abuse (NIDA) (Taylor, 2022).
Traditional stress measurement heavily depends on psychological observation and self-assessment, introducing reliability challenges due to patients’ tendencies to exaggerate or downplay symptoms (Gedam and Paul, 2021). Consequently, there arises a potential necessity to develop a system capable of automatically and accurately capturing users’ stress using dependable physiological signals. The realization of stress detection in diverse circumstances could facilitate real-time monitoring, enabling immediate intervention and adaptability.
Physiological signals are crucial for identifying stress responses, especially when using electrocardiogram (ECG) signals (Jambhale et al., 2022). The stress-related information extracted from Electrocardiogram (ECG) signals, especially heart rate variability (HRV), is prevalent in stress detection (Teixeira Ribeiro and Silva Cunha, 2018). Stress detection is enhanced when integrating ECG with other electrophysiological signals (Jambhale et al., 2022), such as EEG, electrodermal (EDA) to address broader biological and emotional aspects of stress. It reached up to 95.0% accuracy when combined ECG with EEG signals for binary detection (Hemakom et al., 2023) and 79.54% accuracy in discriminating four-level stress (Xia et al., 2018). While peripheral physiological signals offer valuable insights, they may lack specificity regarding the cognitive or emotional basis of physiological arousal, highlighting the need for integrated approaches to ensure a comprehensive understanding of stress detection. Thus, EEG stands out as a safe and reliable method of stress detection. By implementing advanced machine learning/deep learning methodologies, the detection of stress and non-stress status can be significantly improved (Malviya and Mal, 2022; Mane and Shinde, 2023). Although EEG captures intricate neural activity patterns, individual physiological variations may affect its precision (Neeta et al., 2017).
Since stress is commonly categorized as eustress (positive) and distress (negative) (McEwen, 2017). Eustress with its potential to motivate growth and resilience, may contribute to improved performance. In contrast, distress often emerges from chronic stress, leading to a range of health issues (Giannakakis et al., 2022). Consequently, it becomes imperative to develop the capability to detect and distinguish distress from eustress and non-stress conditions for effective stress identification and prevention of prolonged exposure to chronic stress.
The objectives of this study encompass several key areas. One objective is to explore a multi-class stress discriminant system capable of distinguishing between social stress, mental stress, and non-stress conditions. Additionally, this study also aims to establish a comprehensive multi-level stress classification framework that spans individuals experiencing minimal stress to those under extreme stress by focusing on stress detection within a short period of time. Through these objectives, this study aims to:
1. Enhance the precision and practicality of stress detection using multi-modal neurophysiological signals, including brain signals (EEG) and peripheral physiological signals.
2. Contribute to the classification of different types of stress, specifically social stress and mental stress.
3. Evaluate the efficacy of stress identification across different time durations and determine the optimal ultra-short duration for all physiological signals.
4. Explore appropriate machine learning methodologies for stress detection in scenarios where stress types, levels, and time durations vary.
By enhancing the precision and practicality of stress identification and differentiation using ultra-short periods of multi-modal physiological signals, this study aims to explore the potential to be translated to real-time applications and thereby facilitate targeted interventions for stress management.
2 Methodology
2.1 Experimental protocol
In this experiment, eleven healthy subjects (three females, average age 24.7 years, range 18–29 years) participated in this study. Two protocols were conducted in this study, the Montreal Imaging Stress Task (MIST) (Dedovic et al., 2005) and the Trier Social Stress Test (TSST) (Kirschbaum et al., 1993), to stimulate mental stress and social stress respectively. After each section, a self-assessment questionnaire was filled out by the participant to evaluate their stress level scoring from 0–4 representing not stressed at all to extremely stressed. Between each protocol, 20 min of break was provided.
The MIST protocol consists of five sections of arithmetic tasks, including an Introduction section and four progressively challenging test sections. Figure 1A illustrates the MIST paradigm. Each section has a duration of 3 min. The Introduction section illustrates sample math tasks, recording the time taken for each question. An average time was computed from the Introduction section to establish a time limit for the subsequent four test sections. In the test sections, participants were presented with analogous math tasks but were subject to time constraints and received stressful auditory and visual feedback for incorrect/missing calculations. Key elements of the MIST interface, as shown in Figure 1B, include a central display of the math question, response options located below, a countdown displayed above, and a progress bar at the top of the screen indicating the accuracy percentage. The accuracy bar transitions from green to red if accuracy falls below 60%. Each question is assigned a time limit based on the participant’s performance in the Introduction section. Upon providing a correct response, participants receive a subtle auditory cue along with a visual indicator, denoting “correct” before transitioning to the subsequent question. Conversely, an incorrect response or a lack of response prompts an auditory alert coupled with the display of a conspicuous pop-up window conveying “Incorrect” and “Time is out,” respectively. No meditation or rest periods were provided between sections, to allow for the observation of the expected increase in stress levels.
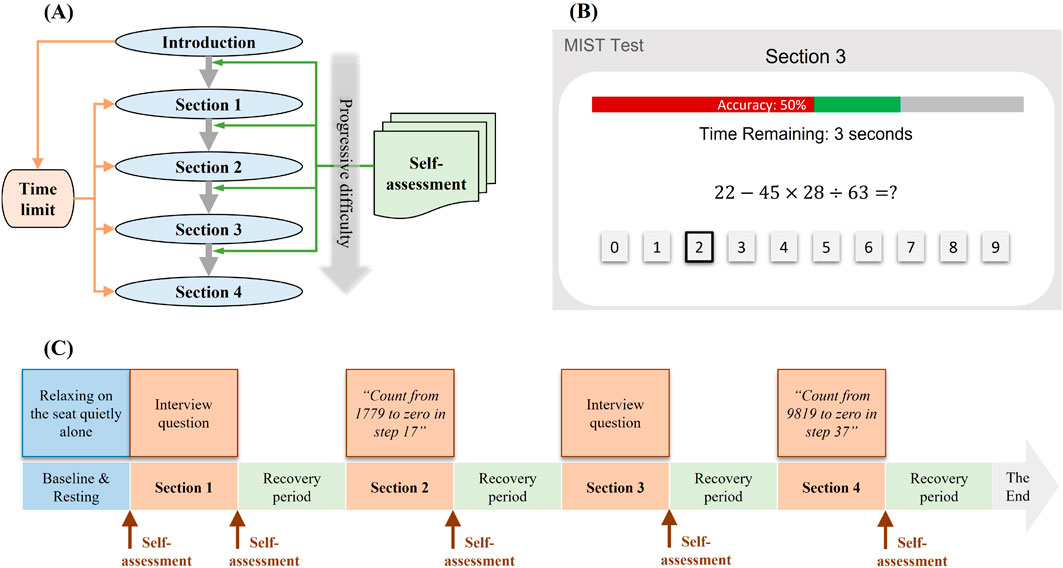
Figure 1. Experimental paradigm. (A) MIST Test procedure (B) MIST interface (C) TSST Test procedure.
TSST protocol incorporates two designated speaking sections with interview questions and two sections of mental arithmetic tasks, with a duration of 3 min for each section. In the speaking sections, participants were presented with questions and required to respond without any preparation time. Meanwhile, the participants were expected to maintain eye contact with the instructor and sustain their speech until the allocated time concluded. In the arithmetic sections, the participants were requested to count from a four-digit number to zero in increments of a two-digit prime number. Participants were required to restart the counting process upon making any mistakes. Subsequent to each section, participants were afforded a 10-min rest period to recover. The procedure is illustrated in Figure 1C.
2.2 Data collection
The experiment conduction and data collection were under an approved protocol by the Internal Review Board (IRB) of the University of Maryland Baltimore County. Before the stress experiment, the subjects were sitting and relaxing at the experimental table with 3 min of baseline recorded (Figure 2A). During the experiment, electrophysiological signals and EEG signals were measured and recorded with a g.tec Amplifier (g.HIamp, g.tec medical engineering GmbH, Graz Austria) at a 512 Hz sampling rate, low-pass filtered at 30 Hz. The pulse plethysmogram (Pleth), SpO2 and heart rate (HR) were measured using a pulse oximeter sensor (EnviteC) clipped on the index fingertip. The galvanic skin response (GSR) or electro-dermal activity (EDA) records the electrical conductivity using the 2 g.GSRsensors placed on the middle and ring fingertips. The temperature was recorded by g.TEMPsensor within a range of 20°C–45°C placed on the palm. In order to allow the subjects to do the tasks with their dominant hand, all electrophysiological sensors were placed on their non-dominant hands. For EEG acquisition, a high-density EEG cap based on 10/20 system position was used to collect neural signals from 33 active EEG electrodes located at Fp1, Fpz, Fp2, AF7, AF3, AF4, AF8, F7, F5, F3, Fz, F2, F4, F6, F8, FC5, FC3, FC4, FC6, C5, C3, C4, C6, CP5, CP3, CPZ, CP4, CP6, P5, P3, P4, P6, Oz (Figure 2B). During the EEG signal recording, the subjects will be instructed to minimize head movement during the 3-min collection.
2.3 Data analysis
Each 3-min data section was initially segmented into shorter lengths for analysis. To explore the potential for real-time stress detection with minimal delay, we examined four signal durations: 60 s, 30 s, 10 s, and 4 s. To effectively preserve information while using shorter segments, each duration was extracted from the original recordings with a 50% overlap. The physiological features were extracted from each segment and a detailed description of these features is given below.
2.3.1 Peripheral physiological features
2.3.1.1 HR, SpO2 and temperature
Mean and standard deviation (std) were calculated within each segment.
GSR/EDA is one of the body responses to stress and is widely used for quantifying the autonomic nervous system (ANS) activity. As a part of the response, the sweat from the body surface leads to changes of skin conductivity, indicating the changes in the skin’s electrical characteristics due to perspiration, reflecting stress variations. When a person becomes more or less stressed, the GSR increases or decreases respectively. The time series of GSR comprises two fundamental components. One is the skin conductance level (SCL) which relates to the slower-acting components and background characteristics of the signal reflecting general changes in autonomic arousal. The other is the skin conductance response (SCR) which refers to the rapid short-lasting changes in the GSR caused mainly due to neuronal activity (Ghosh et al., 2015). First, the GSR signals were decomposed into phasic components (SCR) and tonic components (SCL) using nonnegative deconvolution by solving a convex optimization approach problem using the MATLAB function cvxEDA (Greco et al., 2016). The mean, std, maximum and minimum values were calculated as features from these two components respectively. The SCR higher than threshold 0.1 was considered as effective response, and the corresponding number of responses, maximum and minimum distance between two responses were extracted as features.
PPG/Pleth displays the volumetric change of blood in the vessels according to the respiratory cycle. A standard time domain heart rate variability (HRV) and pulse rate variability (PRV) can be reliably derived from ultra-short term (60 s) PPG recording (Mejía-Mejía and Kyriacou, 2023). HRV, the temporal fluctuation of consecutive heartbeats, is discerned through the pulse wave signal generated by a PPG sensor. The pulse cycle intervals (NN intervals) extracted from this signal present a compelling alternative to ECG for HRV analysis, often referred to as (PRV) analysis (Correia et al., 2020).
Time domain features were computed including
• Beats Per Minute (BPM): An essential metric used to measure pulse rate.
• Mean of PRV Time Elapsed Between Successive Normal PPG Onset Intervals (meanNN) represents the average time between two successive normal PPG onset intervals, also considered as normal R-waves in HRV. Measured in milliseconds, this metric contributes to understanding the temporal dynamics of PRV.
• Standard Deviation of NN Intervals (SDNN) quantifies the standard deviation of NN intervals. An SDNN value greater than 50 ms suggests normal stress, providing valuable information about the variability in consecutive heartbeats.
• Percentage of Successive Beat-to-Beat Intervals >50 ms (pNN50) reflects the percentage of successive beat-to-beat intervals that differ by more than 50 ms. A decrease in pNN50 percentage suggests lower parasympathetic activity, offering insights into the autonomic nervous system’s modulation.
• Root Mean Square of Successive NN Interval Differences (RMSNN) is an important metric for estimating high-frequency variations in pulse rate, particularly pertinent for short-term RR recordings. RMSNN contributes to understanding the intricate dynamics of consecutive heartbeats.
Frequency domain measures of short-term HRV estimated the distribution of power into low-frequency band (LF, 0.04–0.15 Hz) and high-frequency band (HF, 0.15–0.4 Hz) and total frequency band (0–5 Hz). LF bands which are modulated by the sympathetic and parasympathetic nervous systems. In this study, the computed frequency domain features include HF power, LF power, LF/HF (LF power to HF power ratio), LF power to total power ratio and HF power to total power ratio.
Furthermore, Poincare parameters (Brennan et al., 2001) provide a geometrical method for analyzing HRV and represent the correlation between successive inter-beats intervals. SD1 and SD2 are used to qualify Poincare plot geometry, where SD1 is the standard deviation of short-term variability and SD2 is the standard deviation of long-term variability, as illustrated below.
where
2.3.2 Feature extraction in EEG
During the 3-min data collection, EEG signals contain a lot of noise and artifacts. First, the polynomial trends were removed from the raw EEG by subtracting the evaluated polynomial curve. To eliminate the eye movement artifacts, an algorithm based on discrete stationary wavelet transform (DSWT) combined with independent component analysis (ICA) was designed to identify and remove ocular artifacts from EEG signals. The artifact removal based on the ICA-DWT technique (Gonzalez-Carabarin et al., 2021) has been demonstrated as an effective technique in EEG data cleaning. The EEG signals were first decomposed into approximation and detail coefficients under 5-level decomposition using DSWT. After the ICA was applied to remove ocular artifacts from the approximation coefficients, the cleaned EEG signals were reconstructed then using inversive DSWT. This procedure is illustrated in Figure 3.
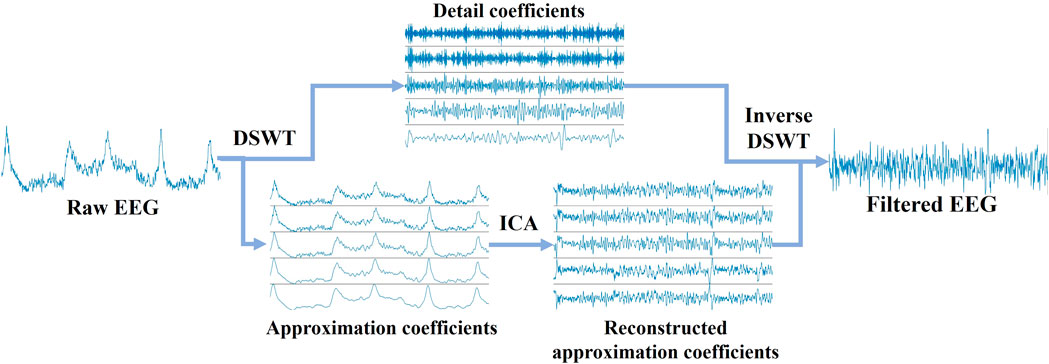
Figure 3. Diagrammatic representations of artifact removal from raw EEG signals. EEG signals were decomposed into approximation and detail coefficients using 5-level DSWT. After ocular artifacts were removed from the approximation coefficients via ICA, the signals were reconstructed with inverse DSWT.
Since the physiological and EEG signals were pre-filtered to 0–30 Hz during the data collection, the EEG signals were divided into five rhythms including delta (δ, 1–4 Hz), theta (θ, 4–8 Hz), alpha (α, 8–13 Hz), beta I (β1, 13–22 Hz), beta II (β2, 22–30 Hz), and broad range (1–30 Hz). The spectral powers of EEG signals from each rhythm were estimated within the whole time period. In addition to the spectral powers from each frequency band, the power ratios across the different rhythms were considered as main features of each channel of EEG signals. The full lists of all features are given in Table 2.
2.3.3 Stress discrimination
In order to investigate the detectability of stress levels and the discernment of stress types based on physiological and EEG signals, it was imperative to address the individual variability in physiological responses to stressors. Thus, stress states were discerned within each subject, accounting for the idiosyncratic changes in physiological features during stress-inducing events.
Preceding the application of classifiers, a meticulous data preprocessing step was executed. This involved the removal of trials containing inf or NaN values from the dataset. Subsequently, an 80%–20% split was performed for each class, designating 80% of the trials as the training set and the remaining 20% as the testing data. To assess the efficacy of the employed classifiers, a robust 10-fold cross-validation methodology was employed, ensuring the selection of distinct batches of training and testing data in each fold.
In the field of stress studies, a variety of classifiers have been explored for the assessment of mental stress levels. This study specifically opted for the inclusion of well-established and widely recognized classifiers, including Support Vector Machines (SVM), K-Nearest Neighbors (KNN), Naïve Bayes (NB), Linear Discriminant Analysis (LDA), and Decision Tree (DT). These classifiers were strategically chosen to facilitate multi-level stress detection and the nuanced identification of various stress types.
3 Results
In exploring the efficacy of stress detection utilizing both physiological and EEG signals for discerning stress levels and types, individual analyses of physiological and EEG signals were conducted to assess whether the combined approach enhances the discriminatory performance in stress detection.
Table 3 presents a comprehensive comparison of classification accuracy across various approaches for discriminating non-stress, social stress, and mental stress using different classifiers. In stress detection exclusively utilizing peripheral physiological signals, the Decision Tree (DT) emerged as the predominant classifier, surpassing all others and achieving remarkable average accuracy across all studied time lengths. Remarkably, the classification performance indicated that a shorter period of time leads to superior stress detection, presenting an inverse relationship between signal analysis duration and the effectiveness of stress detection using peripheral physiological signals. Conversely, concerning the utilization of EEG signals for stress detection, it showed better stress discrimination in general when compared with peripheral physiological signals. The performance using EEG consistently diminishes as the time period involved in the analysis decreases. Among all classification methods, LDA proved to be the most effective classifier for identifying stress types using EEG signals, particularly when the time periods involved in the analysis exceeded 10 s, demonstrating an average of 99.8%, 99.7% and 98.5% accuracy among all subjects for 60-s, 30-s and 10-s period of EEG respectively. A significant drop in performance occurred when only 4 s of EEG signals were analyzed, whereas it achieved an accuracy of 96.5% with KNN and DT. This implies that more extended durations enhance the discriminatory capability for various types of stress within EEG signals. Conversely, when the time periods involved in data analysis are shorter, the DT classifier excelled in effectively discriminating stress levels among all classifiers.
Table 4 shows the performance of multi-level stress detection using different classifiers. Similar to stress type detection, when stress detection solely utilizes peripheral physiological signals, the DT classifier emerged as the most effective among all classifiers considered, achieving an impressive average accuracy of 96.9% across the diverse subjects involved in the study. Contrastingly, when focusing on stress detection through EEG signals, the DT classifier did not demonstrate the same robust performance. Instead, the accuracy achieved by LDA was notable for both 60-s and 30-s signal periods; however, a significant drop from 99% to 77% occurred when the signal analysis duration was reduced from 60 s to 4 s for stress detection. This divergence indicated the sensitivity of EEG signal-based stress detection when relying on EEG signals, especially on the development of the potential of real-time stress detection.
In general, the combined detection utilizing both peripheral physiological and EEG features showed apparent improvement compared to relying on individual physiological or EEG features alone. Furthermore, it enhanced the efficiency and accuracy of multi-level stress detection, even within a short analysis period. LDA consistently outperformed all classifiers when using 60-s, 30-s, and 10-s signal durations, while the DT classifier excelled in effectively discriminating stress when the analysis time dropped to 4 s. This dynamic interplay highlights the adaptability and potential of combined features for more robust stress detection methodologies. It is perspective that the integration of physiological and EEG signals offers a comprehensive and promising approach for stress detection across various time scales, with extended periods enhancing classification accuracy.
4 Discussion
4.1 EEG rhythms in stress assessment
The investigation of brain activity in individuals experiencing stress has been the focus of recent research. In a separate study, EEG was utilized to classify positive, neutral, and negative emotions (Rothkrantz et al., 2008). Previous literature consistently demonstrated the correlation between EEG rhythms and stress (Lee and Jung-Tae, 2010). Coherence analysis of EEG signals reveals increased coherence under stress, suggesting enhanced inter-regional communication in the brain to cope with the cognitive demands of stressors (Katmah et al., 2021). Additionally, stimulation triggers influence EEG responses, as evidenced by heightened activity in specific brain regions under stress (Vanhollebeke et al., 2023). Our study analyzed brain activity by calculating different power ratios from EEG data, contributing to understanding the brain’s electrical activity patterns in response to stress, as shown in Figure 4. Key findings include an increase in delta and theta wave power in the frontal lobe during periods of stress, in which a prominent alpha suppression occurred during stress. Furthermore, a notable increase in beta power in the temporal and parietal lobes under stress suggests enhanced activity in response to stressful stimuli. These observations are particularly significant as they contribute valuable insights to the understanding of the brain’s electrical activity patterns in response to stress.
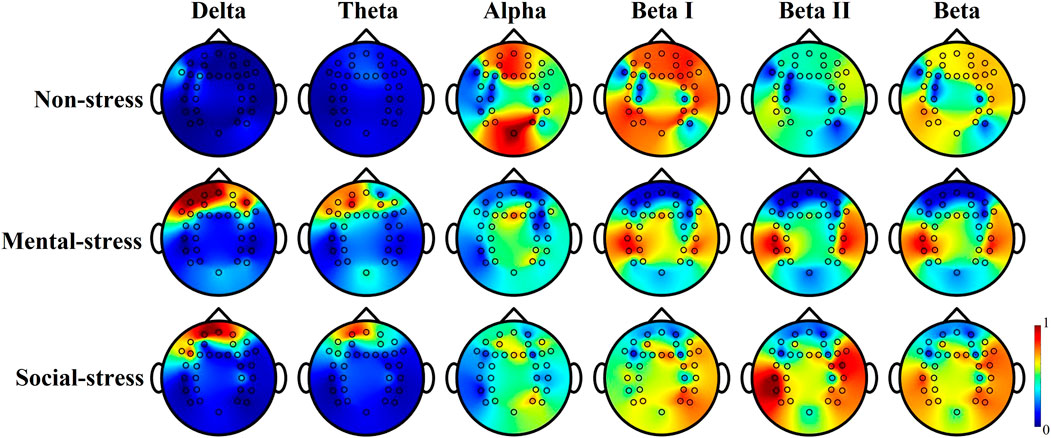
Figure 4. Tomography of relative power activation from different EEG rhythms from subject 2, 60 s timeframe. The relative power was estimated from the absolute power to total power ratio.
Research has shown that stress affects various EEG rhythms differently. Delta rhythms were identified as being correlated with the inclusion of difficult situations (Ladakis and Chouvarda, 2021), and the majority of the delta detected corresponded to the stressful condition (Choi et al., 2015) indicating the brain is healing from stress (Attar, 2022). Research has shown that the frontal theta power is closely linked to task difficulty, where the theta rhythm decreases as the task becomes more challenging (Lopez-Duran et al., 2012). Bairagi and Kulkarni (2019) concluded that the theta band (frequency ranges from 4 Hz to 7 Hz) associated with the frontal lobe is helpful in determining stress levels. Increased power (observed from Figure 4) in delta and theta waves indicates stress and challenging situations, and the activation spreads around the frontal cortex, especially the dorsolateral prefrontal cortex.
Additionally, literature revealed the relationship between stress and alpha rhythm of EEG from the frontal lobe (Goodman et al., 2013). A notable increase of alpha power follows with stimulus application (Katmah et al., 2021). It is commonly observed that the alpha rhythm tends to increase during periods of relaxation, while it decreases under stressful conditions, which is often observed in the prefrontal cortex (Roy et al., 2022). This observation is apparently shown in Figure 4 that both frontal and parietal lobes decreased during mental and social stress, and the suppression of the alpha powers is more obvious during the mental stress condition. The coherence analysis has shown that there is an increase in coherence during times of stress (Katmah et al., 2021). This increased coherence is interpreted as a mechanism that enhances communication between different regions of the brain for processing stressors. It is interesting that the alpha suppression during social stress is not as obvious as observed from that of mental stress, which may be due to the less sensitivity of frontal power affected by only psychosocial stress on the sensor level (Vanhollebeke et al., 2023).
Furthermore, beta power increases in the temporal lobe, indicating a positive correlation with stress (Choi et al., 2015). This study also indicates a positive correlation between stress and EEG beta rhythms, emphasizing higher beta power during stressful periods (Katmah et al., 2021; Attar, 2022), which could be particularly observed in 22–30 Hz rhythms in Figure 4 (beta II). Increased activations around bilateral superior and middle temporal cortices were observed from beta I (13–22 Hz) rhythms. Conversely, beta II (22–30 Hz) rhythm of EEG power reflects and prominent difference between stress and non-stress. This aligns with the observations from the previous study (Jena, 2015), showing average EEG activity during stress sits between 22 Hz and 25 Hz. From Figure 4, the beta rhythm activation from the left hemisphere was greater than the right hemisphere during both mental and social situations. Nevertheless, compared to beta II, beta I activation yields under social stressors. The asymmetric changes in the brain activity revealed the previously demonstrated phenomenon that stress-related EEG activation primarily occurs in the brain’s left anterior region (Gedam and Paul, 2021; Giannakakis et al., 2022), which impacts stress level detection and evaluation. While gamma rhythms exhibit decreased activity in both relaxed and stressful situations (Katmah et al., 2021) which was not considered in this study.
Figure 5 provides an initial understanding of the spatial distribution of essential features extracted from EEG across the brain. The unique spatial distributions identified from stress types versus stress levels could help emphasize the importance of considering optimal features and approaches in future research endeavors. The determination of the significance of features from each EEG channel plays a crucial role in understanding stress types and levels. This is achieved through the estimation of mutual information between these features and the corresponding stress types/levels. Mutual information is used to assess the dependence between EEG features, and it helps identify the most representative features from EEG signals based on entropy concepts. By quantifying the statistical dependency between features, the topographical concatenation and similarity of joint probability distribution functions of these features can be estimated, providing insights into the connectivity and interdependence of brain regions during stress (as shown in Figure 5).
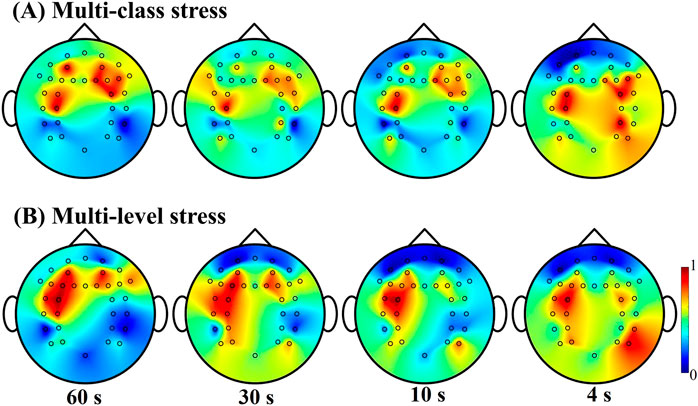
Figure 5. EEG feature importance distribution for multi-class stress detection in (A) and multi-level stress detection in (B). The heatmap represents normalized mutual information score, reflecting the significance of each EEG channel contributing to stress detection.
An interesting observation when considering an extended period of signals for stress evaluation is the concentrated presence of the contribution area around the prefrontal cortex. This suggests that the prefrontal cortex plays a key role in processing and responding to stress. However, when shorter time durations are considered, the contribution area expands to include the frontal lobe, parietal lobe, and temporal lobe. This spatial distribution indicates that different regions of the brain are involved in stress processing at different time scales. Considering the findings presented in Tables 3, 4, it becomes evident that with a longer duration of signals, EEG spectral powers could provide a more condensed and reliable capability for the contribution to a more stable evaluation of stress. This is further supported by the concentrated and prominent feature contributions observed within a 60-s timeframe, as opposed to the more dispersed tomography observed within a 4-s timeframe (as illustrated in Figure 5). Furthermore, it is evident that there is an asymmetry distribution of features observed from the frontal and temporal lobes in both stress type identification and stress level assessment. In the case of multi-level stress discrimination, the left hemisphere exhibits more significant features compared to other regions.
4.2 Peripheral physiological signals in stress assessment
In addition to examining brain cognition, peripheral signals also play a crucial role in identifying stress. These signals provide an initial response to stress before it is even consciously recognized. Multiple peripheral physiological signals contribute to classifying and identifying stress responses, including heart rate, respiration, blood pressure, muscle tension, EMG, GSR, and oxygen level, etc. By analyzing these signals, we can gain valuable insights into the presence and intensity of stress.
There are five peripheral physiological signals were involved in the study, including temperature, oxygen saturation, heart rate, PPG and GSR. Among these signals, PPG and GSR are time series signals that contain numerous temporal features crucial for identifying and understanding stress. Under a stressful situation, a cascade of hormonal changes and physiological responses lead to an elevation in heart rate, as well as a decrease in body temperature and blood oxygen saturation levels. However, the informative features which can be extracted are limited. While these three parameters are influenced by stress, they still present significant variability that can be affected by other factors such as physical activities, environmental temperatures, etc. On the other hand, when combined with PPG and GSR, which are time-series signals involving dynamic changes, more reliable monitoring and detection of stress can be achieved. This can provide a more comprehensive understanding of an individual’s stress levels.
This study included the investigation of both different types and levels of stress. One of the objectives was to explore how physiological features contribute to the identification and differentiation of stress across different time durations. Based on the classification results presented in Tables 3, 4, it is evident that the decision tree method achieves superior performance compared to other methods when utilizing peripheral physiology features for stress classification. To gain further insights into the contribution of each feature to the classification process, feature importance was assessed for the decision tree algorithm. This was done by measuring the reduction in entropy values, which represents the change in the information provided by each feature in identifying the target value. The estimation of feature importance was rescaled to a range of 0–1, as visualized in Figure 6. The mean and standard deviation extracted from temperature, SpO2 and heat rate play a significant role in stress discrimination both stress types and stress levels.
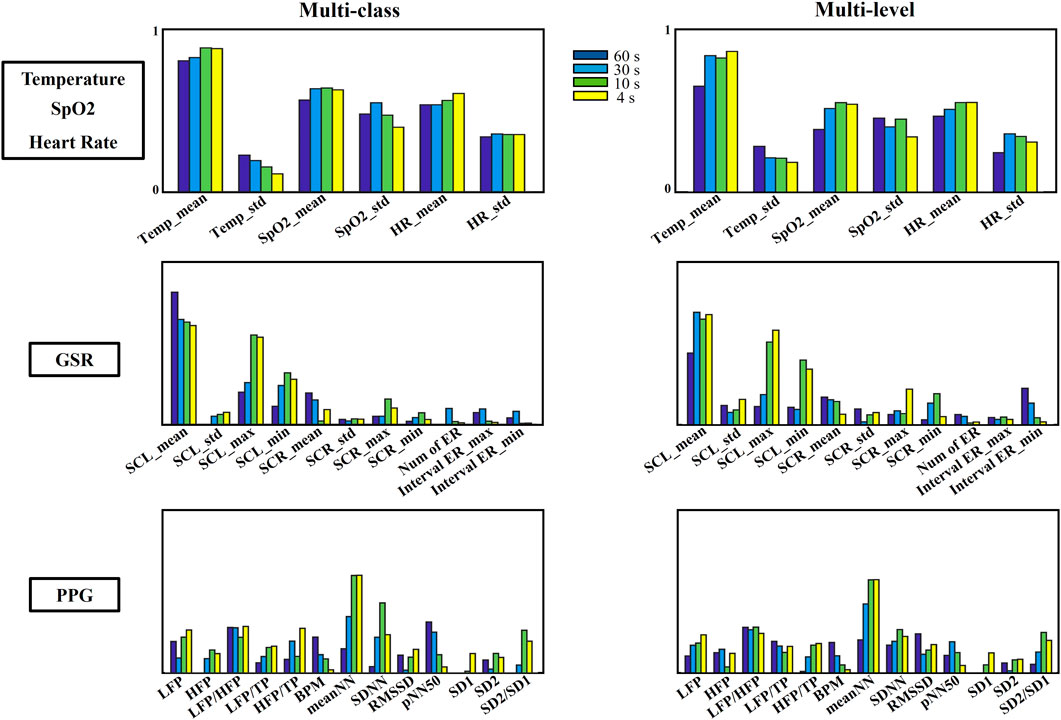
Figure 6. Feature importance of peripheral physiological features for multi-class and multi-level stress detection across different time durations.
GSR, which represents the electrical properties of skin conductivity, has been demonstrated a high correlation to emotional cognition including stress. The Skin Conductance Level (SCL) derived from GSR directly reflects the general automatic arousal and it has been demonstrated as a reliable indicator of stress (Sánchez-Reolid et al., 2020). This indicator remains important in stress identification even when the measurement duration is significantly reduced from 60 s to 4 s, as illustrated in Figure 6. The significance of this indicator in stress identification persists, maintaining its effectiveness within very short periods. Notably, SCL emerges as the most influential feature for discriminating stress across varying time lengths derived from GSR and all SCL features demonstrate an increased contribution to stress discrimination, with this effect particularly pronounced in multi-level stress detection. Especially, the maximum value of SCL demonstrates enhanced accuracy with decreasing time durations, both in cases of stress type and stress level. This indicates its potential for detecting stress changes within shorter time periods. Conversely, the sensitivity of SCR appears to diminish with decreasing timeframes, especially in terms of the average value of SCR. The effective responses of SCR contribute minimally to stress identification compared to other features. Fewer effective responses were effectively recognized and captured within a short period time. This may be attributed to the fact that SCR responses are particularly pronounced during emotional arousal and are closely linked to stimuli associated with stressors (Lutin et al., 2021). Literature shows the phasic components of GSR have established strong direct correlations with theta waves (<4 Hz) and high inverse correlation with delta waves (4–8 Hz) (Posada-Quintero and Chon, 2019).
Heart rate variability (HRV) is another crucial factor that could indicate stress conditions, lots of studies have demonstrated its efficacy in the detection of stress (Salahuddin et al., 2007a; Lu et al., 2009; Correia et al., 2020). HRV is a reliable primary metric for assessing ANS activity in stress identification, with its fluctuations reflecting an individual’s capacity to adapt to changes in the intervals between successive peaks. Decreases in short-term HRV are indicative of acute stress, suggesting a negative impact during stressful situations (He et al., 2019a). Pulse rate variability (PRV), recorded from the PPG sensor, is an alternative method for assessing ANS activity, which reflects changes in arterial blood volume during each cardiac cycle. Previous studies have demonstrated the correlations between PPG features and stress (Maaoui et al., 2016). Some disagreements are also found in previous studies which impose caution when comparing metrics from different sensors (Correia et al., 2020) and short-term versus chronic stress cases (Schubert et al., 2009).
In this study, time, frequency, and geometric domain features were extracted and analyzed from PPG signals to evaluate both the levels and types of induced stress. In general, the features extracted from the time domain show greater importance compared to other feature domains (Figure 6). Particularly, the feature measuring the time between two successive normal PPG onset intervals (NN) outstand among other features, and it shows a general upward trend as the duration of the PPG signal decreases. In contrast, a downward trend in contribution was observed for features extracted from beats per minute (BPM) and the percentage of successive beat-to-beat intervals (pNN50) over shorter time periods. This decline is attributed to the challenges in capturing a complete cycle of the beat pattern for accurate estimation. When considering multi-level classification, a slightly higher importance of the geometrical features (SD1, SD2, and SD2/SD1) can be observed. Furthermore, an increased contribution can be observed from meanNN, SDNN, and SD2/SD1 as the time duration decreases from 60 s to 4 s. This indicates that these features become more influential in capturing stress-related patterns within shorter timeframes. Moreover, it is evident that certain features in the time domain are sensitive to the time duration of stress processing. For instance, the average pulse cycle interval (meanNN) becomes more precise with shorter durations both in multi-class and multi-level cases. The frequency domain features demonstrate stability across different time periods, with no significant changes in their contribution observed across various time durations. Conversely, accurate BPM and pNN50 are best captured over longer timeframes, as ultra-short time windows may lead to inaccurate counts due to missed cycles, especially when the heartbeats remain consistent for a prolonged period. In such cases, shorter time durations fail to successfully capture stress-related features.
4.3 Multimodal stress discrimination using machine learning methods
This study introduces multiple machine learning approaches to assess stress across different stress types and various stress levels, by integrating multiple physiological signals. All these machine-learning approaches demonstrated their efficacy in stress detection using single or multimodal physiological signals in previous studies (Gedam and Paul, 2021). The performance of stress classification in this study varies depending on the physiological signals and their corresponding time lengths utilized.
While the performance using EEG alone is significantly superior to that of peripheral physiological signals, and extended periods contribute to an enhanced classification accuracy in the identification of stress. Previous studies have demonstrated the capability and reliability of stress recognition from EEG with up to 94.6% accuracy for 2-level of stress and around 83% accuracy using SVM and NB for multiple levels of stress (Subhani et al., 2017). KNN has shown its superior capacity in multi-level stress detection (Khosrowabadi, 2018; Plechawska-Wójcik et al., 2019), which also achieved the best performance when using 4-s EEG signals. Three levels of stress can be detected with an average of 75% performance (Jun and Smitha, 2016). Optimized SVM accomplished accurate binary stress detection using ultra-short duration of EEG signals (2, 4, 8, 10 s) (Sharma et al., 2022). Similar results can be observed from Tables 3, 4, for both multi-class and multi-level stress discrimination, The SVM achieved an accuracy of over 98% when the time durations were 30 s and 10 s, which was only slightly lower than the performance achieved using LDA. The LDA has demonstrated its effectiveness in brain cognition detection from EEG with a very low computational burden (Arpaia et al., 2020), making it efficient in real-time multi-level stress detection within a few seconds (Minguillon et al., 2018).
For stress detection using peripheral physiological signals alone, SVM is widely used in emotion and stress recognition systems (Rizwan et al., 2019). The performance of SVM is usually higher than other classifier algorithms in distinguishing multi-levels of stress (Rizwan et al., 2019; Sharma and Chopra, 2020). GSR signals were effectively utilized to identify multi-level relaxation responses through DT classification, achieving over 99% accuracy (Martinez et al., 2019). Additionally, single PPG sensors were employed to classify stress levels, attaining accuracies of 86% with SVM and 82% with DT (Awasthi et al., 2020). In this study, the DT outperforms other methods but is less suitable for EEG signals. The DT selects variables at each decision node by calculating the entropy for each variable and its potential splits, with the change in entropy across all nodes representing the information gain provided by a feature for the target variable. The entropy plays a crucial role in node splitting and division among multiple classes. The classification performance is affected by the independence of variables within each class. The possible reason for the better performance of the DT on physiological signals may be due to higher feature independence, whereas EEG features derived from EEG signals tend to be more dependent, hindering perfect splits using the DT method.
The combination of peripheral physiological signals and brain activity demonstrated superior performance compared to the use of individual signals alone in this study. The results indicate significant improvements in stress detection when utilizing both modalities, as illustrated in Tables 3, 4. Previous study also suggested that there is a significant increase accuracy of stress detection has been evidenced from multiple physiological signals when compared to individual contributions (Shi et al., 2010; Wijsman et al., 2011; Kurniawan et al., 2013; Zhang et al., 2017; Aigrain et al., 2018; Jambhale et al., 2022). Machine learning models have been effectively utilized to leverage the combined power of ECG and EEG signals for stress detection which achieved 79% discrimination of four levels of stress using SVM (Xia et al., 2018). SVM and KNN yielded the highest average classification accuracy and the accuracy increased up to 20% when combining ECG with EEG (Hemakom et al., 2023). Therefore, to enhance the reliability of the stress detection strategy, the integration of multi-modality physiological signals has garnered significant attention in the field. This approach emphasizes achievement and improvement and thereby demonstrates the importance of obtaining a comprehensive evaluation of stress using diverse physiological signals (Aigrain et al., 2018; Panicker and Gayathri, 2019). The inclusion of additional signal types enables effective feature selection from a broader range of choices, further enhancing the accuracy and efficacy of stress detection outcomes (Wijsman et al., 2011). More common cases are the combination of peripheral physiological signals and EEG signals, which have demonstrated high utility and reliability in stress assessment (Salai et al., 2016; Rizwan et al., 2019; Gedam and Paul, 2021; Katmah et al., 2021; Malviya and Mal, 2022). These observations emphasize the significance of incorporating both types of signals in stress detection systems. Fortunately, the improved performance using peripheral physiological signals compensates for the decrease in accuracy from EEG when the time length is reduced after combining peripheral physiological and EEG signals. The overall conclusion is that the integration of peripheral physiological and EEG signals offers a comprehensive and promising approach to stress detection, with extended periods enhancing classification accuracy. These findings have implications for developing real-time, efficient stress detection systems with minimum delay.
4.4 The short duration of stress identification for future real-time applications
In analyzing individual EEG or peripheral physiological signals for stress classification, our study revealed a clear pattern in accuracy relative to the duration of the signal recording. Longer durations enhanced the ability to discriminate between various types and levels of stress within EEG signals, while shorter durations performed better using peripheral physiological signals. However, combining EEG and peripheral physiological data proved highly effective for stress classification, with longer durations enhancing accuracy across various time scales.
To the current time, many attempts have been made to explore the repeatability and reliability of ultra-short periods of physiological signals for a clear guideline for a stable real-time stress detection application. Researchers have achieved real-time stress detection using multi-modal physiological signals (Bin et al., 2015), using wearable sensors (Prashant Bhanushali et al., 2020), portable systems (Minguillon et al., 2018) or smart devices (Ciabattoni et al., 2017), This study attempted to investigate the efficiency of stress detection from various time-duration biomedical signals utilizing the correlation between physiological signals and stress.
Previous studies attempt to evaluate the performance of stress detection using different lengths of time-series physiological signals. The window length adopted in stress identification is usually in minutes which is not possible for effective and efficient real-time detection. Usually, at least 2 min of the HRV/PRV signals are required for reliable and accurate feature extraction. It has been demonstrated real-time stress detection from ECG signals using the on-chip reservoir computer (Chandrasekaran et al., 2020). Three-minute overlapping segments of ECG were employed for both offline training and real-time simulation. Previous literature revealed the efficiency and reliability of stress detection using multiple physiological sensors and achieved over 98% accuracy for over 100 s of interval. The longer time included the better the performance (Ghaderi et al., 2015). It was found that PRV recordings are more stable and convenient than ECG recordings and PRV indices can be reliably estimated from signals as short as 90 s (Mejía-Mejía and Kyriacou, 2023) and PRV indices can be extracted from ultra-short PPG signals (60s) (Zubair and Yoon, 2020). Furthermore, it validated that it was possible to preserve stress-related features for acute stress monitoring within 50 s (Salahuddin et al., 2007b). With the utilization of physiological signals, many previous studies have realized real-time stress detection using different biosignals. A time duration of 10 s of ECG signals enabled real-time cognitive stress detection using CNN and achieved over 80% accuracy (He et al., 2019b). A short period time of 5-s segment of PPG signals achieved 99% accuracy in discriminating multi-level stress (Mukherjee et al., 2022). The window length considered in this study included 5 s–20 s in a 5 s step and the detection results revealed an increased accuracy with time duration decreased.
Notable findings have shown the achievement on investigation on the impact of different time durations on stress detection using EEG. Remarkably, even shorter durations of EEG signals have demonstrated the capacity to achieve high levels of identification accuracy. EEG-based stress detection showed its reliability, with an average accuracy exceeding 89% in real-time scenarios, as evidenced in [60], achieving the capability and effectiveness of EEG-based approaches in real-time stress detection applications. Moreover, it suggested that the average accuracy of binary classification significantly improves from 70% to 96% when the time window decreases from 10 s to 2 s as reported in (Jun and Smitha, 2016). Similarly, Sharma et al. (2022) demonstrated a decline in detection performance when the signal length is reduced from 10 s to an ultra-short duration of 2 s in binary stress detection from EEG. The real-time applications worker’s stress monitoring enabled the stress assessment updated every 2 s and reached more than 90% accuracy. When time latency increases to 4 s, the stress discriminating accuracy reaches 100% (Arpaia et al., 2020).
The results of this study provide promising outcomes in stress identification, as well as discrimination of stress types and stress levels, using multiple physiological signals within an ultra-short period. The remarkable improvement in classification accuracy achieved with reduced time windows demonstrates the feasibility of efficient and accurate stress detection in shorter durations. These findings have significant implications for the development of real-time stress monitoring systems and provide a foundation for further research in this field. However, it is important to note that when processing data within ultra-short time durations (less than 2 s), the complete periodicity of signals, particularly in the case of PPG signals and phasic responses from GSR, may not be captured effectively. Additionally, utilizing ultra-short time durations can lead to the omission of certain feature extractions during PRV signal processing. On average, there is an approximate data loss of 1.4% and 9.3% when the time duration is reduced to 10 s and 4 s, respectively. The primary cause of this data loss stems from the inability to capture the average time between two successive pulse onsets (meanNN), resulting in a sequence of missing values for related features such as SDNN, RMSSD, pNN50, SD1, SD2, and SD2/SD1. In this scenario, instances with data loss were removed entirely from the analysis. In the context of multi-modality stress detection, which combines brain signals with peripheral physiology signals, a crucial consideration is the decision regarding time duration. Balancing the preservation of informative features and minimizing time latency becomes essential for enabling potential real-time applications. To achieve an effective compromise, it is necessary to carefully select a time duration that captures sufficient information from all physiological signals. This ensures that the stress detection system maintains a high level of accuracy while minimizing any potential delays in real-time applications.
5 Conclusion
This study explored the capability of stress discrimination for multi-class and multi-level stress detection using various lengths of physiological signals and the results demonstrated the adaptability and potential of combined multimodal features for more robust stress detection. This study also explored the efficacy of precise stress detection with a short duration of signal, offering promising prospects for real-time applications in wearable devices. In future research. Different discrimination methods were discussed, and it revealed the selection of the optimal approach aligned with the specific stress type and duration of the physiological signals employed for stress detection. The Decision Tree outperformed other classifiers for peripheral signals, especially for ultra-short durations (4 s), achieving an average accuracy of 98.3%. For EEG signals, Linear Discriminant Analysis proved most effective with longer signal durations (over 10 s), reaching 99.8% accuracy for 60-s EEG windows in differentiating stress types. By integrating peripheral and EEG signals, the performance estimated from ultra-short timeframes improve to 98%. This emphasizes the balance between maximizing feature extraction and minimizing latency for real-time applications. Continued research is essential to further advance real-time stress detection technologies. The current research is primarily conducted in controlled laboratory environments, relying on physical tests and questionnaires that may be subject to user-entered data biases and inaccuracies. The measurement of physiological parameters in real-time and real-world conditions presents challenges. Variabilities in recorded signals caused by factors such as temperature, physical activity, motion artifacts, and mood fluctuations need to be considered. Additionally, more subjects will be included in this research, and future studies will also take into account parameters that influence stress responses, such as gender and age. Addressing these considerations will enhance the accuracy and applicability of real-time stress detection systems in practical settings. Continued research efforts in this direction will contribute to the development of comprehensive and reliable stress detection solutions that can positively impact various domains, including healthcare, wellbeing, and performance optimization.
Data availability statement
The raw data supporting the conclusions of this article will be made available by the authors, without undue reservation.
Ethics statement
The studies involving humans were approved by the present study was conducted under IRB Approved Protocol at the University of Maryland Baltimore County (Protocol #408–Selection of Optimal Control Signals for Human Machine Interfaces). The studies were conducted in accordance with the local legislation and institutional requirements. The participants provided their written informed consent to participate in this study.
Author contributions
DP: Data curation, Formal Analysis, Validation, Visualization, Writing–original draft, Writing–review and editing. ST: Data curation, Methodology, Software, Writing–review and editing. KT: Methodology, Software, Writing–review and editing. AA: Writing–review and editing. RV: Conceptualization, Funding acquisition, Project administration, Resources, Supervision, Writing–review and editing.
Funding
The author(s) declare that financial support was received for the research, authorship, and/or publication of this article. This research was funded by National Science Foundation (NSF) CAREER Award, grant number HCC-2053498 and NSF IUCRC BRAIN CNS-2333292.
Conflict of interest
The authors declare that the research was conducted in the absence of any commercial or financial relationships that could be construed as a potential conflict of interest.
Publisher’s note
All claims expressed in this article are solely those of the authors and do not necessarily represent those of their affiliated organizations, or those of the publisher, the editors and the reviewers. Any product that may be evaluated in this article, or claim that may be made by its manufacturer, is not guaranteed or endorsed by the publisher.
References
Aigrain, J., Spodenkiewicz, M., Dubuiss, S., Detyniecki, M., Cohen, D., and Chetouani, M. (2018). Multimodal stress detection from multiple assessments. IEEE Trans. Affect Comput. 9, 491–506. doi:10.1109/TAFFC.2016.2631594
Arpaia, P., Moccaldi, N., Prevete, R., Sannino, I., and Tedesco, A. (2020). A wearable EEG instrument for real-time frontal asymmetry monitoring in worker stress analysis. IEEE Trans. Instrum. Meas. 69, 8335–8343. doi:10.1109/TIM.2020.2988744
Attar, E. T. (2022). Review of electroencephalography signals approaches for mental stress assessment. Neurosciences 27, 209–215. doi:10.17712/nsj.2022.4.20220025
Awasthi, K., Nanda, P., and Suma, K. V. (2020). “Performance analysis of Machine Learning techniques for classification of stress levels using PPG signals,” in Proceedings of CONECCT 2020 - 6th IEEE International Conference on Electronics, Computing and Communication Technologies, 1–6. doi:10.1109/CONECCT50063.2020.9198481
Bairagi, V., and Kulkarni, S. (2019). “A novel method for stress measuring using EEG signals,” in Advances in intelligent systems and computing, 887.
Bin, M. S., Khalifa, O. O., and Saeed, R. A. (2015). “Real-time personalized stress detection from physiological signals,” in Proceedings - 2015 International Conference on Computing, Control, Networking, Electronics and Embedded Systems Engineering (Khartoum, Sudan: ICCNEEE), 352–356. doi:10.1109/ICCNEEE.2015.7381390
Brennan, M., Palaniswami, M., and Kamen, P. (2001). Do existing measures of Poincare plot geometry reflect nonlinear features of heart rate variability? IEEE Trans. Biomed. Eng. 48, 1342–1347. doi:10.1109/10.959330
Chandrasekaran, S. T., Bhanushali, S. P., Banerjee, I., and Sanyal, A. (2020). A bio-inspired reservoir-computer for real-time stress detection from ECG signal. IEEE Solid State Circuits Lett. 3, 290–293. doi:10.1109/LSSC.2020.3016924
Choi, Y., Kim, M., and Chun, C. (2015). Measurement of occupants’ stress based on electroencephalograms (EEG) in twelve combined environments. Build. Environ. 88, 65–72. doi:10.1016/j.buildenv.2014.10.003
Ciabattoni, L., Ferracuti, F., Longhi, S., Pepa, L., Romeo, L., and Verdini, F. (2017). “Real-time mental stress detection based on smartwatch,” in 2017 IEEE International Conference on Consumer Electronics, ICCE 2017, 110–111. doi:10.1109/ICCE.2017.7889247
Correia, B., Dias, N., Costa, P., and Pêgo, J. M. (2020). Validation of a wireless bluetooth photoplethysmography sensor used on the earlobe for monitoring heart rate variability features during a stress-inducing mental task in healthy individuals. Sensors 20, 3905–3919. doi:10.3390/s20143905
Davis, M. T., Holmes, S. E., Pietrzak, R. H., and Esterlis, I. (2017). Neurobiology of chronic stress-related psychiatric disorders: evidence from molecular imaging studies. Chronic Stress 1, 2470547017710916. doi:10.1177/2470547017710916
Dedovic, K., Renwick, R., Mahani, N. K., Engert, V., Lupien, S. J., and Pruessner, J. C. (2005). The Montreal Imaging Stress Task: using functional imaging to investigate the effects of perceiving and processing psychosocial stress in the human brain. J. psychiatry & Neurosci. JPN 30, 319–325.
Gedam, S., and Paul, S. (2021). A review on mental stress detection using wearable sensors and machine learning techniques. IEEE Access 9, 84045–84066. doi:10.1109/ACCESS.2021.3085502
Ghaderi, A., Frounchi, J., and Farnam, A. (2015). “Machine learning-based signal processing using physiological signals for stress detection,” in 2015 22nd Iranian Conference on Biomedical Engineering (Tehran, Iran: ICBME), 93–98. doi:10.1109/ICBME.2015.7404123
Ghosh, A., Danieli, M., and Riccardi, G. (2015). “Annotation and prediction of stress and workload from physiological and inertial signals,” in Proceedings of the Annual International Conference of the IEEE Engineering in Medicine and Biology Society, EMBS 2015-Novem, 1621–1624. doi:10.1109/EMBC.2015.7318685
Giannakakis, G., Grigoriadis, D., Giannakaki, K., Simantiraki, O., Roniotis, A., and Tsiknakis, M. (2022). Review on psychological stress detection using biosignals. IEEE Trans. Affect. Comput. 13, 440–460. doi:10.1109/TAFFC.2019.2927337
Gonzalez-Carabarin, L., Castellanos-Alvarado, E. A., Castro-Garcia, P., and Garcia-Ramirez, M. A. (2021). Machine Learning for personalised stress detection: inter-individual variability of EEG-ECG markers for acute-stress response. Comput. Methods Programs Biomed. 209, 106314. doi:10.1016/j.cmpb.2021.106314
Goodman, R. N., Rietschel, J. C., Lo, L.-C., Costanzo, M. E., and Hatfield, B. D. (2013). Stress, emotion regulation and cognitive performance: the predictive contributions of trait and state relative frontal EEG alpha asymmetry. Int. J. Psychophysiol. 87, 115–123. doi:10.1016/j.ijpsycho.2012.09.008
Greco, A., Valenza, G., Lanata, A., Scilingo, E. P., and Citi, L. (2016). CvxEDA: a convex optimization approach to electrodermal activity processing. IEEE Trans. Biomed. Eng. 63, 797–804. doi:10.1109/TBME.2015.2474131
He, J., Li, K., Liao, X., Zhang, P., and Jiang, N. (2019a). Real-time detection of acute cognitive stress using a convolutional neural network from electrocardiographic signal. IEEE Access 7, 42710–42717. doi:10.1109/ACCESS.2019.2907076
He, J., Li, K., Liao, X., Zhang, P., and Jiang, N. (2019b). Real-time detection of acute cognitive stress using a convolutional neural network from electrocardiographic signal. IEEE Access 7, 42710–42717. doi:10.1109/ACCESS.2019.2907076
Hemakom, A., Atiwiwat, D., and Israsena, P. (2023). ECG and EEG based detection and multilevel classification of stress using machine learning for specified genders: a preliminary study. PLoS One 18, 02910700–e291124. doi:10.1371/journal.pone.0291070
Jambhale, K., Mahajan, S., Rieland, B., Banerjee, N., Dutt, A., Kadiyala, S. P., et al. (2022). Identifying biomarkers for accurate detection of stress. Sensors 22, 8703. doi:10.3390/s22228703
Jena, S. (2015). Examination stress and its effect on EEG. Int. J. Med. Sci. Public Health 4, 1493. doi:10.5455/ijmsph.2015.23042015308
Jun, G., and Smitha, K. G. (2016). “EEG based stress level identification,” in 2016 IEEE International Conference on Systems, Man, and Cybernetics, SMC 2016 - Conference Proceedings, 3270–3274. doi:10.1109/SMC.2016.7844738
Katmah, R., Al-Shargie, F., Tariq, U., Babiloni, F., Al-Mughairbi, F., and Al-Nashash, H. (2021). A review on mental stress assessment methods using eeg signals. Sensors 21, 5043. doi:10.3390/s21155043
Khosrowabadi, R. (2018). Stress and perception of emotional stimuli: long-term stress rewiring the brain. Basic Clin. Neurosci. J. 9, 107–120. doi:10.29252/nirp.bcn.9.2.107
Kirschbaum, C., Pirke, K. M., and Hellhammer, D. H. (1993). The “Trier social stress test” - a tool for investigating psychobiological stress responses in a laboratory setting. Neuropsychobiology 28, 76–81. doi:10.1159/000119004
Kurniawan, H., Maslov, A. V., and Pechenizkiy, M. (2013). “Stress detection from speech and galvanic skin response signals,” in Proceedings of CBMS 2013 - 26th IEEE International Symposium on Computer-Based Medical Systems (IEEE Computer Society), 209–214. doi:10.1109/CBMS.2013.6627790
Ladakis, I., and Chouvarda, I. (2021). Overview of biosignal analysis methods for the assessment of stress. Emerg. Sci. J. 5, 233–244. doi:10.28991/esj-2021-01267
Lee, S.-H. S., and Jung-Tae, (2010). “Stress and EEG,” in Convergence and hybrid information technologies.
Lopez-Duran, N. L., Nusslock, R., George, C., and Kovacs, M. (2012). Frontal EEG asymmetry moderates the effects of stressful life events on internalizing symptoms in children at familial risk for depression. Psychophysiology 49, 510–521. doi:10.1111/j.1469-8986.2011.01332.x
Lu, G., Yang, F., Taylor, J. A., and Stein, J. F. (2009). A comparison of photoplethysmography and ECG recording to analyse heart rate variability in healthy subjects. J. Med. Eng. & Technol. 33, 634–641. doi:10.3109/03091900903150998
Lutin, E., Hashimoto, R., de Raedt, W., and van Hoof, C. (2021). “Feature extraction for stress detection in electrodermal activity,” in BIOSIGNALS 2021 - 14th International Conference on Bio-Inspired Systems and Signal Processing; Part of the 14th International Joint Conference on Biomedical Engineering Systems and Technologies, 177–185. doi:10.5220/0010244601770185
Maaoui, C., Bousefsaf, F., and Pruski, A. (2016). Automatic human stress detection based on webcam photoplethysmographic signals. J. Mech. Med. Biol. 16, 1650039–1650113. doi:10.1142/S0219519416500391
Malviya, L., and Mal, S. (2022). A novel technique for stress detection from EEG signal using hybrid deep learning model. Neural Comput. Appl. 34, 19819–19830. doi:10.1007/s00521-022-07540-7
Mane, S. A. M., and Shinde, A. (2023). StressNet: hybrid model of LSTM and CNN for stress detection from electroencephalogram signal (EEG). Results Control Optim. 11, 100231. doi:10.1016/j.rico.2023.100231
Martinez, R., Salazar-Ramirez, A., Arruti, A., Irigoyen, E., Martin, J. I., and Muguerza, J. (2019). A self-paced relaxation response detection system based on galvanic skin response analysis. IEEE Access 7, 43730–43741. doi:10.1109/ACCESS.2019.2908445
McEwen, B. S. (2017). Neurobiological and systemic effects of chronic stress. Chronic Stress 1. doi:10.1177/2470547017692328
McHugh, R. K., Sugarman, D. E., Meyer, L., Fitzmaurice, G. M., and Greenfield, S. F. (2020). The relationship between perceived stress and depression in substance use disorder treatment. Drug Alcohol Dependence 207, 107819. doi:10.1016/j.drugalcdep.2019.107819
Mejía-Mejía, E., and Kyriacou, P. A. (2023). Duration of photoplethysmographic signals for the extraction of pulse rate variability indices. Biomed. Signal Process. Control 80, 104214. doi:10.1016/j.bspc.2022.104214
Mills, M. L. (2023). Impairment, disability, and substance use disorder. Subst. Use & Misuse 58, 221–228. doi:10.1080/10826084.2022.2155475
Minguillon, J., Perez, E., Lopez-Gordo, M. A., Pelayo, F., and Sanchez-Carrion, M. J. (2018). Portable system for real-time detection of stress level. Sensors Switz. 18, 2504–2515. doi:10.3390/s18082504
Mukherjee, N., Mukhopadhyay, S., and Gupta, R. (2022). Real-time mental stress detection technique using neural networks towards a wearable health monitor. Meas. Sci. Technol. 33, 044003. doi:10.1088/1361-6501/ac3aae
Neeta, M., Patil, B., Mirajkar, R. P., Patil, M. S., and Patil, M. P. (2017). A method for detection and reduction of stress using EEG. Int. Res. J. Eng. Technol. 4, 1598–1604.
Panicker, S. S., and Gayathri, P. (2019). A survey of machine learning techniques in physiology based mental stress detection systems. Biocybern. Biomed. Eng. 39, 444–469. doi:10.1016/j.bbe.2019.01.004
Plechawska-Wójcik, M., Tokovarov, M., Kaczorowska, M., and Zapala, D. (2019). A three-class classification of cognitiveworkload based on EEG spectral data. Appl. Sci. Switz. 9, 5340. doi:10.3390/app9245340
Posada-Quintero, H. F., and Chon, K. H. (2019). “Phasic component of electrodermal activity is more correlated to brain activity than tonic component,” in 2019 IEEE EMBS International Conference on Biomedical and Health Informatics, BHI 2019 - Proceedings, 1–4. doi:10.1109/BHI.2019.8834567
Prashant Bhanushali, S., Sadasivuni, S., Banerjee, I., and Sanyal, A. (2020). “Digital machine learning circuit for real-time stress detection from wearable ECG sensor,” in Midwest Symposium on Circuits and Systems 2020-Augus (IEEE), 978–981. doi:10.1109/MWSCAS48704.2020.9184466
Rizwan, M. F., Farhad, R., Mashuk, F., Islam, F., and Imam, M. H. (2019). “Design of a biosignal based stress detection system using machine learning techniques,” in 1st International Conference on Robotics, Electrical and Signal Processing Techniques, ICREST 2019, 364–368. doi:10.1109/ICREST.2019.8644259
Rothkrantz, L. J. M., Horlings, R., and Datcu, D. (2008). “Emotion recognition using brain activity,” in Proceedings of the 9th International Conference on Computer Systems and Technologies and Workshop for PhD Students in Computing (Gabrovo, Bulgaria: CompSysTech’08), 1–6. doi:10.1145/1500879.1500888
Roy, S., Islam, M., Yusuf, M. S. U., and Jahan, N. (2022). EEG based stress analysis using rhythm specific spectral feature for video game play. Comput. Biol. Med. 148, 105849. doi:10.1016/j.compbiomed.2022.105849
Salahuddin, L., Cho, J., Jeong, M. G., and Kim, D. (2007a). “Ultra short term analysis of heart rate variability for monitoring mental stress in mobile settings,” in Annual International Conference of the IEEE Engineering in Medicine and Biology - Proceedings, 4656–4659. doi:10.1109/IEMBS.2007.4353378
Salahuddin, L., Cho, J., Jeong, M. G., and Kim, D. (2007b). “Ultra short term analysis of heart rate variability for monitoring mental stress in mobile settings,” in Annual International Conference of the IEEE Engineering in Medicine and Biology - Proceedings, 4656–4659. doi:10.1109/IEMBS.2007.4353378
Salai, M., Vassányi, I., and Kósa, I. (2016). Stress detection using low cost heart rate sensors. J. Healthc. Eng. 2016, 1–13. doi:10.1155/2016/5136705
Sánchez-Reolid, R., López, M. T., and Fernández-Caballero, A. (2020). Machine learning for stress detection from electrodermal activity: a scoping review. 1–29. doi:10.20944/preprints202011.0043.v1
Schubert, C., Lambertz, M., Nelesen, R. A., Bardwell, W., Choi, J. B., and Dimsdale, J. E. (2009). Effects of stress on heart rate complexity-A comparison between short-term and chronic stress. Biol. Psychol. 80, 325–332. doi:10.1016/j.biopsycho.2008.11.005
Sharma, L. D., Bohat, V. K., Habib, M., Al-Zoubi, A. M., Faris, H., and Aljarah, I. (2022). Evolutionary inspired approach for mental stress detection using EEG signal. Expert Syst. Appl. 197, 116634. doi:10.1016/j.eswa.2022.116634
Sharma, R., and Chopra, K. (2020). EEG signal analysis and detection of stress using classification techniques. J. Inf. Optim. Sci. 41, 229–238. doi:10.1080/02522667.2020.1714187
Shi, Y., Nguyen, M. H., Blitz, P., French, B., Fisk, S., Torre, F. D.La, et al. (2010). “Personalized stress detection from physiological measurements,” in Second international symposium on quality of life Technology.
Subhani, A. R., Mumtaz, W., Saad, M. N. B. M., Kamel, N., and Malik, A. S. (2017). Machine learning framework for the detection of mental stress at multiple levels. IEEE Access 5, 13545–13556. doi:10.1109/ACCESS.2017.2723622
Taylor, C. (2022). Drug overdoses are costing the U.S. economy $1 trillion a year. government report estimates. CNBC. Available at: https://www.cnbc.com/2022/02/08/drug-overdoses-cost-the-us-around-1-trillion-a-year-report-says.html.
Teixeira Ribeiro, R., and Silva Cunha, J. P. (2018). A regression approach based on separability maximization for modeling a continuous-valued stress index from electrocardiogram data. Biomed. Signal Process. Control 46, 33–45. doi:10.1016/j.bspc.2018.06.006
Vanhollebeke, G., Kappen, M., De Raedt, R., Baeken, C., van Mierlo, P., and Vanderhasselt, M. A. (2023). Effects of acute psychosocial stress on source level EEG power and functional connectivity measures. Sci. Rep. 13, 8807–8813. doi:10.1038/s41598-023-35808-y
Wijsman, J., Grundlehner, B., Liu, H., Hermens, H., and Penders, J. (2011). “Towards mental stress detection using wearable physiological sensors,” in Proceedings of the Annual International Conference of the IEEE Engineering in Medicine and Biology Society (Boston, MA, USA: EMBS), 1798–1801. doi:10.1109/IEMBS.2011.6090512
Xia, L., Malik, A. S., and Subhani, A. R. (2018). A physiological signal-based method for early mental-stress detection. Biomed. Signal Process. Control 46, 18–32. doi:10.1016/j.bspc.2018.06.004
Zhang, B., Morère, Y., Sieler, L., Langlet, C., Bolmont, B., and Bourhis, G. (2017). Reaction time and physiological signals for stress recognition. Biomed. Signal Process. Control 38, 100–107. doi:10.1016/j.bspc.2017.05.003
Keywords: stress detection, physiological signals, EEG, multi-level stress, multi-class stress, mental stress, social stress
Citation: Pei D, Tirumala S, Tun KT, Ajendla A and Vinjamuri R (2024) Identifying neurophysiological correlates of stress. Front. Med. Eng. 2:1434753. doi: 10.3389/fmede.2024.1434753
Received: 18 May 2024; Accepted: 14 October 2024;
Published: 24 October 2024.
Edited by:
Jagannath M., Vellore Institute of Technology (VIT), IndiaCopyright © 2024 Pei, Tirumala, Tun, Ajendla and Vinjamuri. This is an open-access article distributed under the terms of the Creative Commons Attribution License (CC BY). The use, distribution or reproduction in other forums is permitted, provided the original author(s) and the copyright owner(s) are credited and that the original publication in this journal is cited, in accordance with accepted academic practice. No use, distribution or reproduction is permitted which does not comply with these terms.
*Correspondence: Ramana Vinjamuri, cnZpbmphbTFAdW1iYy5lZHU=