- 1School of Artificial Intelligence, Henan Institute of Science and Technology, Xinxiang, China
- 2National and Local Joint Engineering Laboratory of Internet Application Technology on Mine, China University of Mining and Technology, Xuzhou, China
- 3School of Information Science and Engineering, Shenyang University of Technology, Shenyang, China
Introduction: The advancement of Underwater Human-Robot Interaction technology has significantly driven marine exploration, conservation, and resource utilization. However, challenges persist due to the limitations of underwater robots equipped with basic cameras, which struggle to handle complex underwater environments. This leads to blurry images, severely hindering the performance of automated systems.
Methods: We propose MUFFNet, an underwater image enhancement network leveraging multi-scale frequency analysis to address the challenge. The network introduces a frequency-domain-based convolutional attention mechanism to extract spatial information effectively. A Multi-Scale Enhancement Prior algorithm enhances high-frequency and low-frequency features while the Information Flow Interaction module mitigates information stratification and blockage. A Multi-Scale Joint Loss framework facilitates dynamic network optimization.
Results: Experimental results demonstrate that MUFFNet outperforms existing state-of-the-art models while consuming fewer computational resources and aligning enhanced images more closely with human visual perception.
Discussion: The enhanced images generated by MUFFNet exhibit better alignment with human visual perception, making it a promising solution for improving underwater robotic vision systems.
1 Introduction
Underwater Human-Robot Interaction(U-HRI) is an emerging technology that explores and optimizes interactions between humans, computer systems, and intelligent devices in underwater environments. With the increasing underwater activities, such as marine resource exploitation, environmental monitoring, and scientific research, the demand for efficient and intuitive underwater interaction technologies has grown significantly (Birk, 2022). The development of U-HRI advances ocean exploration, offering innovative solutions for marine resource research. However, image blurring is a critical factor limiting U-HRI performance and efficiency during underwater tasks. The uniquely underwater environment renders capturing sharp images with autonomous underwater vehicles (AUVs) extremely challenging. Underwater light absorption and scattering intensify with depth, significantly reducing image contrast and brightness. Light at different wavelengths decays at varying rates underwater, with red light decaying the fastest and blue light the slowest, resulting in predominantly blue or green-hued images. The presence of suspended particles and microorganisms further exacerbates image degradation. Furthermore, the underwater environment is highly dynamic, with uneven illumination and turbulence-induced relative motion between the camera and target frequently causing blurred images. Considering the above factors, research on underwater image enhancement (UIE) technologies is crucial for accurately understanding the underwater world and enhancing the efficiency and safety of U-HRI.
UIE methods are categorized into hardware-based, physical model-based, and Artificial IntelligenceDriven (AID)-based approaches. Hardware-based UIE primarily relies on specialized auxiliary imaging equipment, such as polarization filters, color correction lenses, and multi-spectral sensors, to enhance underwater image quality (Lu et al., 2017). Multi-dimensional environmental information is obtained by integrating professional sensing equipment, improving the underwater image quality. However, expensive hardware devices significantly increase system costs. Additionally, factors such as energy endurance, renewal cycles, and system maintenance further restrict the broader application of these methods.
Physical model-based UIE primarily utilizes the absorption and scattering theory of light to model the propagation dynamics of the underwater medium. It inverts the physical model by simulating the underwater propagation environment parameters to restore image quality (Bi et al., 2024; Zhuang et al., 2022). The processing of this method involves (a) building the degradation model, (b) calculating the model parameters, and (c) tackling the inverse problem. Although this technique has a solid theoretical foundation, greater flexibility, and automation, it also has limitations. Firstly, the method is computationally complex and depends on accurate environmental models and prior knowledge, which becomes particularly ineffective in extreme underwater environments. Additionally, selecting and optimizing the model for various underwater conditions poses challenges to the real-time processing capabilities and robustness of UIE.
Recently, AID-based methods have performed well in UIE tasks by utilizing Deep Neural Networks (DNN) to automatically extract underwater image features and perform complex nonlinear transformations, resulting in enhanced image quality (Cheng et al., 2023). However, the cost of data collection and the substantial computational resource requirements restrict the model’s real-time inference and generalization ability, tending to performance bottlenecks in UIE tasks. Moreover, model performance commonly underperforms when confronted with highly dynamic underwater environments.
To address the above challenges, we propose a novel network framework with AID-based, namely MUFFNet, which strikes a trade-off between effect and efficiency. MUFFNet, using an asymmetric Encoder-Decoder architecture, combines Multi-scale Enhancement Prior (MEP) and Multi-scale Joint Loss (MJ-loss) to increase the feature extraction ability and accelerate network convergence. Additionally, we incorporate a frequency-domain-based convolutional attention mechanism (FFMS) to extract frequency features. Finally, Integrating the Information Flow Interaction (IFI) module into the Decoder accelerates the circulation and fusion of feature information. Notably, the asymmetric structure has significant advantages over traditional symmetric networks in underwater image enhancement. It can specialize in high-frequency and low-frequency features, improving efficiency and reducing redundant computation through targeted module design. The flexible multi-scale feature fusion mechanism helps to better combine the information of different frequencies and is adaptable to the diversity of complex degradation of underwater images, performing differentiated processing for different degradation factors. MUFFNet considers the trade-off between resource limitations and enhanced effects, outperforming vanilla CNNs in UIE tasks. Figure 1 illustrates a performance comparison between MUFFNet and vanilla CNN architecture. The main contributions of this article are summarized as follows:
1. We propose an asymmetric network, MUFFNet, which integrates Multi-scale Enhancement Prior (MEP) and Multi-scale Joint Loss (MJ-loss) to improve the network’s feature extraction and convergence capabilities. Moreover, the design of an Information Flow Interaction (IFI) module facilitates the flow and fusion of frequency-domain information.
2. Designing frequency-domain-based convolution attention extracts high- and low-frequency information in features. Notably, the Multi-scale Enhancement Prior (MEP) can preprocess images, enriching the frequency domain information of the image to enhance the network’s feature extraction effects.
3. Comprehensive experiments demonstrate that MUFFNet surpasses baseline models in UIE tasks, achieving superior image enhancement and alignment with human visual perception while maintaining lower resource consumption.
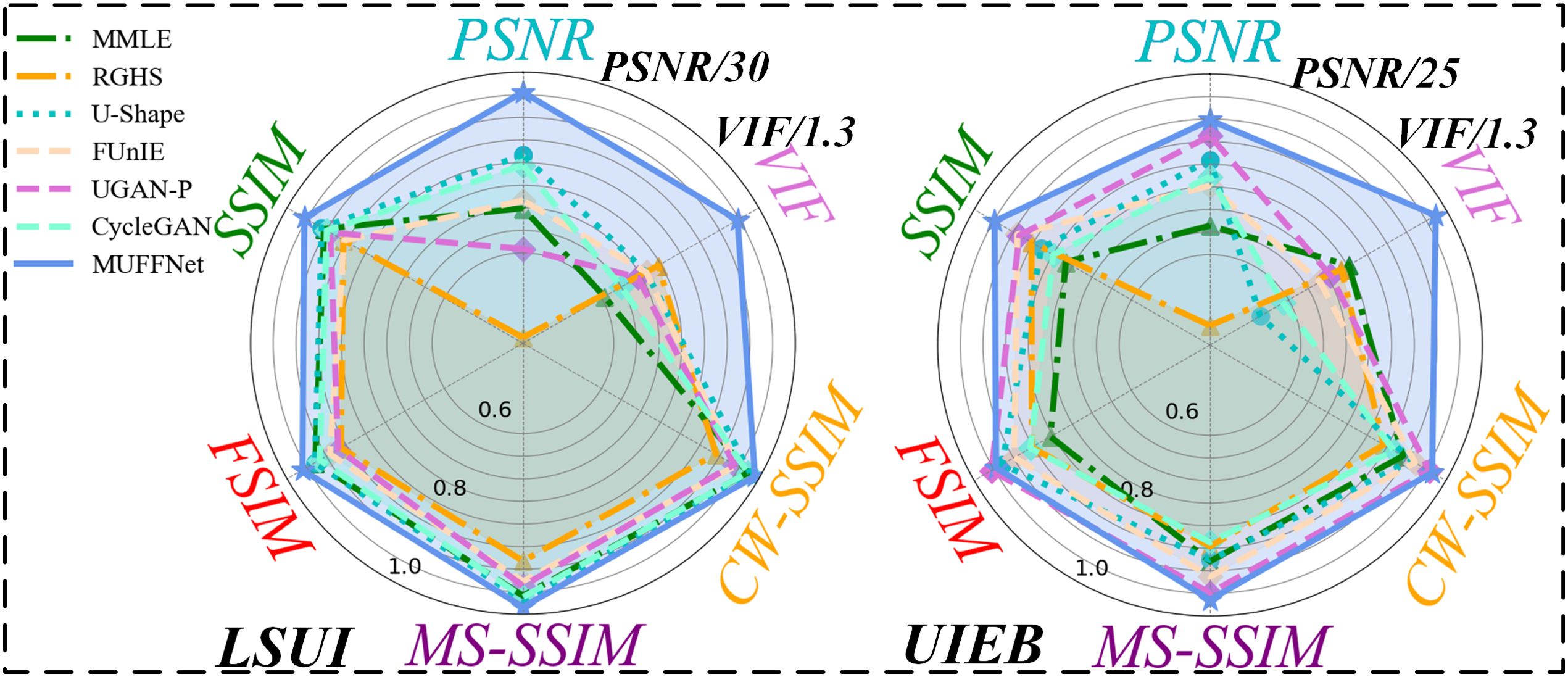
Figure 1. Performance comparison of networks on the LSUI and UIEB datasets. The PSNR and VIF values were divided by 30 and 1.3 on the LSUI dataset and 25 and 1.3 on the UIEB dataset.
2 Related works
2.1 U-HRI
The U-HRI has gained swift development, improving the efficient and secure communication between divers and autonomous underwater vehicles (AUVs).
In (Hong et al., 2024), a diver identification framework deployed on an AUV was proposed, which extracted robust features from diver pose estimates and utilized an embedding network to mitigate the risk of diver identification errors caused by similar-looking scuba gear. Similarly, (Fulton et al., 2023) introduced the SIREN framework, which employs sound generated by underwater robot vibrations for underwater communication. To improve the control of subsea remotely operated vehicles (ROVs) during subsea operations, (Xia et al., 2023a) presented a control algorithm integrating Virtual Reality (VR) and haptic simulators. This approach enhanced operators’ perception of proximity conditions and predictive capabilities, improving operational performance and accuracy. Additionally, (Xia et al., 2023b) proposed a control method based on VR for human body motion and hand gestures, significantly improving navigation and stabilization control precision in Remotely Operated Vehicles (ROVs), thereby advancing subsea engineering exploration. Research into underwater equipment encompasses diverse aspects (Praczyk, 2023; Wang et al., 2024b), greatly accelerating the progress of underwater research.
Image processing is an essential component when deploying AUVs for underwater tasks. However, the dangerous degradation of underwater image quality adversely impacts AUV decision-making, increasing the risk of underwater accidents. Upgrading underwater equipment is commonly challenging due to the expensive advanced devices, which restricts the applicability and reliability of systems. Therefore, a cost-effective, efficient, and widely applicable method is required to address these issues.
2.2 Physical model-based UIE
The physical model-based UIE algorithm can restore the natural color and clarity of underwater images by simulating the transmission characteristic of underwater light, which has obtained in-depth study due to its wide adaptability and solid theoretical foundation.
(Zhang et al., 2022a) introduced a Retinex-inspired color correction and detail-preserving fusion method to address color cast and blurring issues in underwater images. To resolve underwater image color deviations and low visibility, (Zhang et al., 2022b) proposed the MLLE algorithm, inspired by the phenomena of light absorption and scattering, which significantly enhances image quality. Building on frequency-domain analysis, (Zhang et al., 2023c) developed a weighted wavelet visual perception fusion strategy that generates high-quality images by fusing multi-scale frequency information. Additionally, (Zhang et al., 2023b) utilized a piecewise color correction and dual prior optimized contrast algorithm to solve severe underwater image degradation. Meanwhile, (An et al., 2024) proposed a hybrid fusion algorithm to mitigate the visual challenges of underwater images, effectively resolving various quality issues in underwater scenes. Numerous other studies have also contributed to spurring physical model-based UIE methods from diverse perspectives (Qi et al., 2021; Hou et al., 2023; Rao et al., 2023; Kang et al., 2022; Wang et al., 2023).
Despite their advantages, physical model-based UIE methods face certain limitations. Firstly, these algorithms are sensitive to environmental parameters and tend to underperform in varying underwater scenarios. Secondly, simulating the light propagation and scattering process is widely computationally intensive, prolonging the processing time. Furthermore, reliance on specialized equipment and the complexity of underwater environments restricts the generalization and practical application (González-Sabbagh and Robles-Kelly, 2023). Therefore, developing a novel UIE method with improved generalization capabilities and superior imaging quality is essential.
2.3 AID-based UIE
The development of artificial intelligence (AI) has pioneered new research avenues for UIE, demonstrating exceptional performance in underwater image processing (Xu et al., 2023; Liu et al., 2024). To improve underwater image quality, (Peng et al., 2023) introduced a U-shape Transformer network, marking the first introduction of transformer models. This approach achieved remarkable results on public datasets and the LSUI dataset built. As a representative of the pioneering application of large foundation model technology, (Wang et al., 2025) presents a discriminative underwater image enhancement method leveraging large foundation models, which utilizes the Segment Anything Model for foreground-background segmentation, followed by adaptive color compensation and high-frequency edge fusion. The algorithm alleviates the underwater color difference issue, improving the underwater image enhancement effect. Additionally, (Zhang et al., 2024) proposed the CNMS framework, which integrates triple attention and a multi-scale cascade mechanism to capture spatial details and contextual information across images of varying scales. Compared with traditional CNNs, (Wang et al., 2024a) introduces a reinforcement learning framework for underwater image enhancement that transparently selects and configures enhancement methods in a self-organized manner, incorporating human visual perception and underwater color priors. The emergence of transformer (Vaswani et al., 2017) has further enhanced network performance in AID-based UIE tasks, yielding favorable results in underwater image enhancement (Jin et al., 2024; Zhou et al., 2023; Li et al., 2024; Cai et al., 2023).
For extreme underwater environments, (Zhang et al., 2023a) introduced Rex-Net, a model that leverages information from underwater images and reflectance to improve performance across diverse underwater scenes. Similarly, (Cong et al., 2023) proposed a physical model-guided approach based on Generative Adversarial Networks (GANs). This hybrid model combines the strengths of GANs with physical model-based techniques to address challenges in downstream underwater understanding tasks. GANs have demonstrated outstanding performance in various image processing applications, including image inpainting, super-resolution, and style transfer, making them widely studied in the UIE domain (Jiang et al., 2023; Liu et al., 2023; Wang et al., 2021; Ummar et al., 2023; Hu et al., 2023).
The continuous advancement of AID-based UIE methods has significantly improved the efficiency and performance of underwater image processing, facilitating progress in underwater tasks. However, some challenges remain unresolved. The requirements of large-scale datasets and intensive computational resources affect the adaptability and availability of these models, which may present data inconsistencies in highly dynamic underwater scenes (Cong and Zhou, 2023). Therefore, developing a UIE network with strong robustness and high-speed inference capabilities is essential to ensure efficient and reliable task execution.
3 Methodology
Focusing on AID-based UIE, we propose MUFFNet, a dynamic underwater image enhancement network based on multi-scale frequency, as illustrated in Figure 2. Utilizing the FFMS and IFI extract the image frequency information and increase the datastream follow speed, respectively. In addition, the introduction of the Multi-scale Enhancement Prior highlights critical image information, providing prior knowledge for each subnetwork. Finally, designing the Multi-scale Joint Loss accounts for diverse optimization paths, accelerating the network convergence.
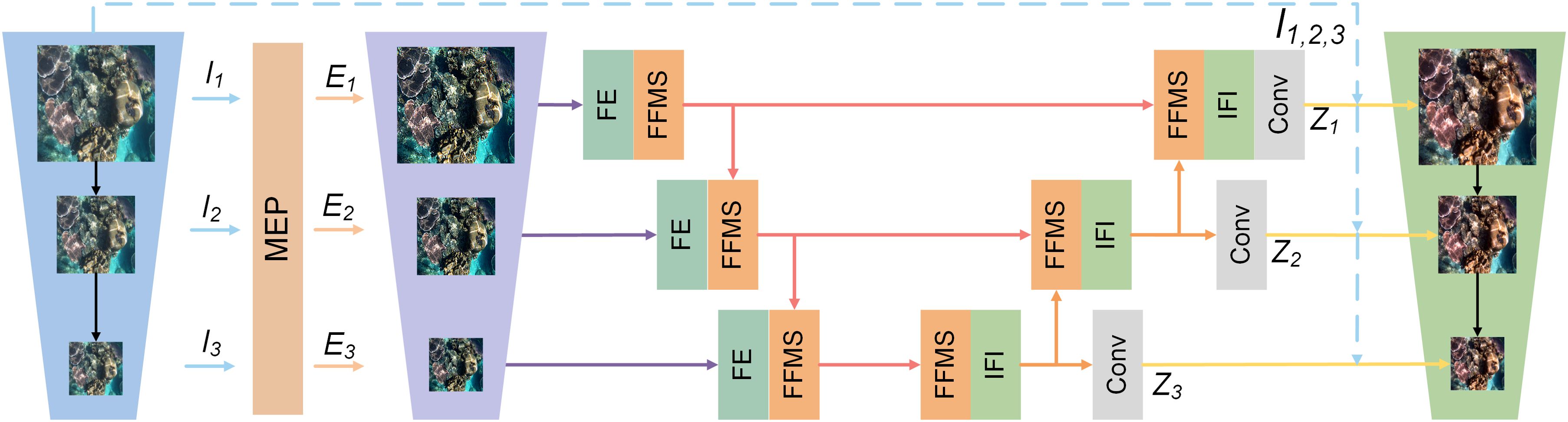
Figure 2. MUFFNet structure. The image is scaled to produce I1, I2, and I3. Then enhanced images E1, E2, and E3 are generated by the MEP, which is input into the sub-network for feature extraction, generating the final enhanced images Z1, Z2, and Z3.
3.1 FFMS module
The image frequency domain contains abundant high-frequency and low-frequency information, which aids in extracting and analyzing edge textures while reducing distractions from irrelevant features. Traditional attention mechanisms and convolutional networks typically deal with local and global information of images in the spatial domain and lack optimization specifically for frequency components, which fails to cope well with degradation in the frequency domain. Therefore, some studies have integrated neural networks with the frequency domain to enhance perceptual information from features Wu et al. (2023); Yang et al. (2023); He et al. (2023); Zhang et al. (2023d).
In the article, we propose the FFMS module, as illustrated in Figure 3, which utilizes Convolution Attention (CA) to extract in-depth features from image frequency space. Firstly, CA replaces the linear network in traditional attention mechanisms with convolution operations without cutting the image into patches. This approach reduces model parameters and accelerates inference speed. By combining CNNs with the attention mechanism, the FFMS captures both global structures and local details, demonstrating superior performance in processing complex underwater images. Secondly, the crucial high-frequency information of underwater images is identified by extracting frequency features, improving the image edge definition. The CA can highlight the helpful high-frequency information, which allocates more attention, by analyzing different frequency components while decreasing its weight for low-frequency information with interference to restrict. Remarkably, convolution operations are converted to point multiplication when transforming features into the frequency domain using the Fourier transform (FFT), significantly reducing computational resource requirements. The FFMS is defined as follows.
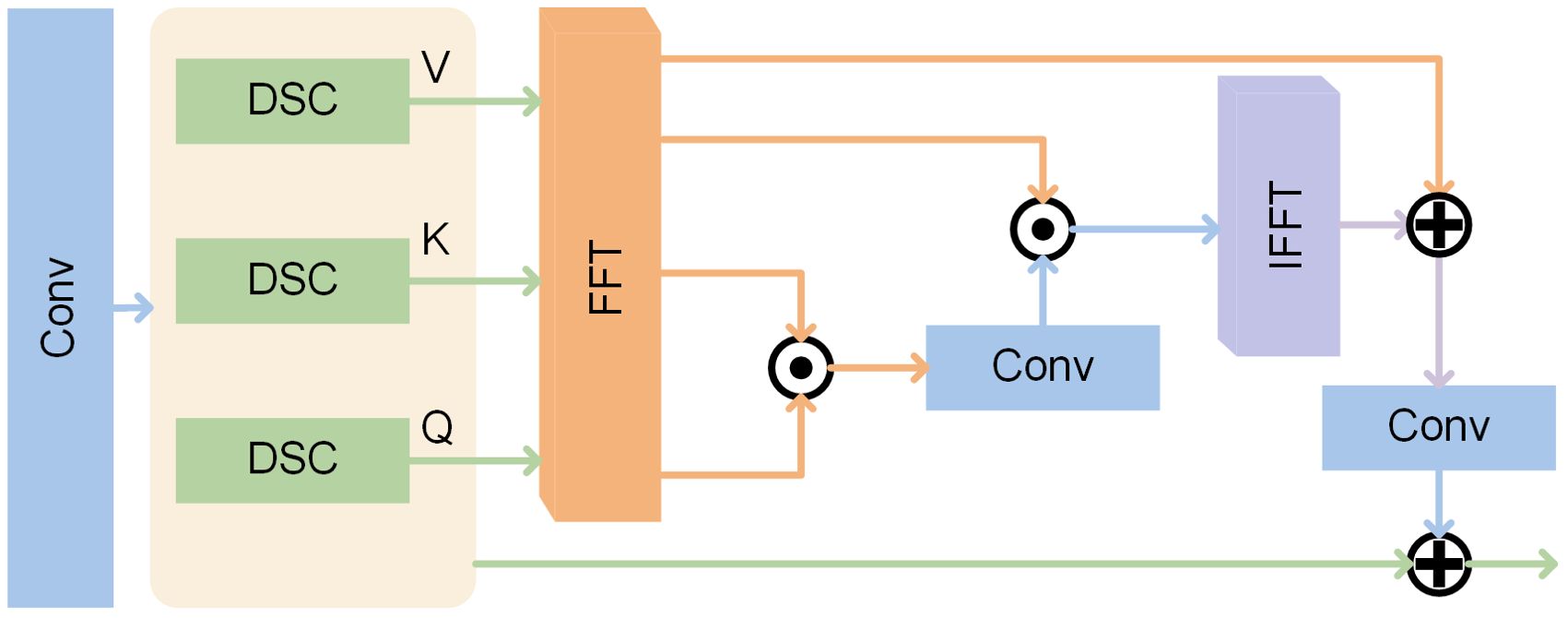
Figure 3. FFMS structure. The features pass through the DSC to obtain Q, K, and V, followed by Fourier variation for frequency domain feature extraction. Finally, the residual block obtains the feature frequency domain information.
Firstly, are obtained by the following formula.
where is Depthwise Separable Convolution (Howard et al., 2017). is Fourier transform (FFT). is inputted feature. Subsequently, the frequency-domain-based convolution attention is calculated.
where ⊙ is point-multiplication operations. The features are converted into time domain features to maintain data consistency.
where IFFT(∗) is the Inverse Fourier transform (IFFT).
Image frequency domain components are usually closely related to its global brightness, color deviation, and other characteristics. Compared to traditional methods, FFMS directly manipulates the frequency components of the image to effectively deal with the spectral distortion in underwater images due to light absorption, scattering, etc., and better adapt to the unique optical effects of the underwater environment. By selectively enhancing or suppressing different frequency components in the frequency domain, it accurately handles high-frequency details and low-frequency light and color distortion problems, improving the detail recovery and color correction capabilities of the image.
3.2 MEP module
Relying solely on FFMS to extract frequency information from the original features is potentially suboptimal due to the smooth gradients in the original images. Basic image enhancement methods, such as white balance, gamma transformation, and high-frequency wavelet techniques, can enhance image quality and prominent information, although dissatisfying complex UIE tasks.
To provide helpful feature information for FFMS, We propose a Multi-scale Enhancement Prior (MEP) to self-adaption enhance images, supplying the network with rich prior knowledge. Enhancing multi-scale images obtained down-sampled the inputted image fed into the Encoder subnetwork. Images are enhanced from various perspectives, including brightness, contrast, saturation, gamma, and sharpness, to improve the saliency of critical information while minimizing interference factors. Therein, the enhancement formula is defined as follows.
where is inputted feature. , , and are the average grayscale, grayscale conversion and Laplacian high-pass filter. are correlation coefficients confined to a fixed range. The defined range of correlation coefficients is in Table 1. The restriction function is defined as follows.
where and are the maximum and minimum values of the coefficients, respectively.
Notably, the aforementioned enhancement algorithms are differentiable, allowing parameters to be optimized via gradient descent. Therefore, we design an adaptively learnable enhancement network to adjust dynamically augmentation parameters confronting different underwater environments. The network structure is shown in Figure 4. Initial image features are extracted to obtain shallow features, which are then dynamically normalized using Conditional Normalization (CN) to enhance the network’s representation capabilities. Remarkably, the CN is widely employed in generative models, which emerged with an exciting performance in generation tasks, including style transfer and super-resolution. Hence, we expect MEP with CN to modulate the relative spatial distribution based on the current image state to obtain optimal enhancement parameters.
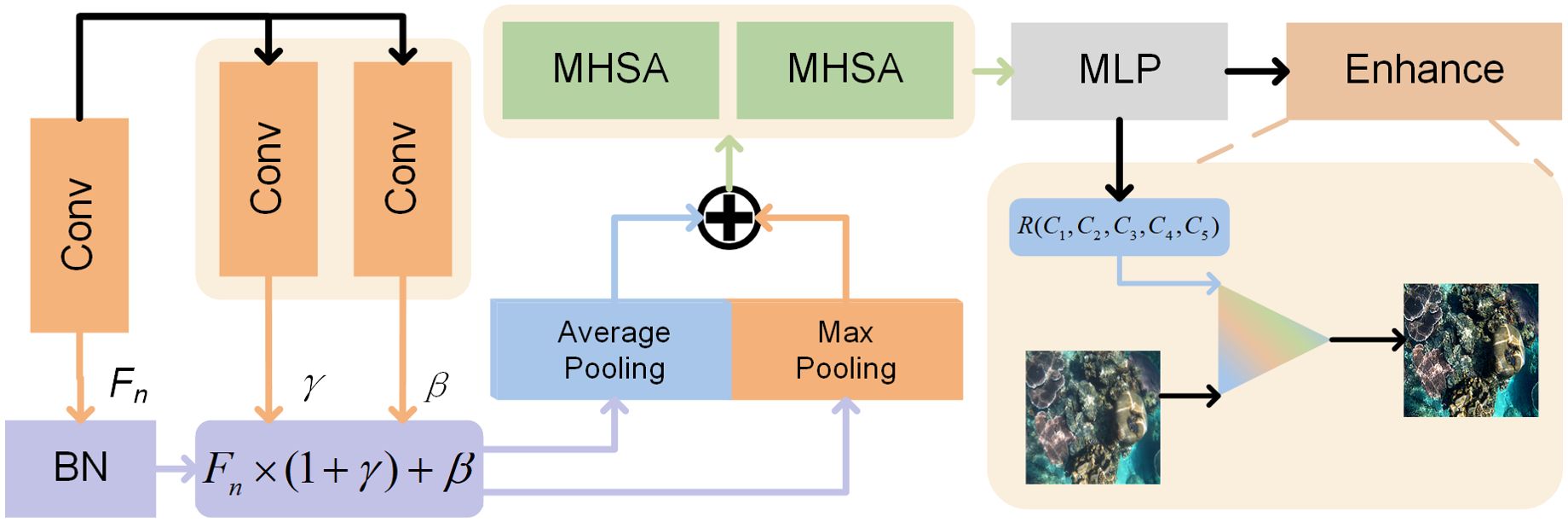
Figure 4. MEP structure. The image is flattened after Conditional Normalization, followed by feature mapping by MHSA, and adaptive generation of enhancement parameters for the initial image enhancement.
Subsequently, the features are flattened by maximum and average pooling to obtain multi-view information. Finally, Multi-Head Self Attention (MHSA) captures long-range dependencies of the flattened features, understanding complex dependencies and patterns and enhancing the network’s representation capabilities. The overall process of image enhancement is as follows.
Firstly, the two-dimensional features are processed.
where is defined as follows.
where is the Batch Normalization. and are scaling and offset factors generated by the Convolution operation, respectively.
Subsequently, the two-dimensional features are flattened and extracted.
where . and are Max Pooling and Average Pooling. is the Multi-layer Perceptron (MLP).
Rather than directly transmitting prior enhanced images to the backbone, we design a feature extraction module (FE), which integrates DSW into a ResNet-based residual block to perform initial image identification. As a transitional layer, the FE mitigatesthe excessive information gap, which enhances feature utilization and reduces the overfitting risk. The FE definition is as follows.
where is the enhanced image by MEP.
The MEP strategy performs adaptive image enhancement through a joint dynamic enhancement algorithm, dynamically selecting relevant coefficients to address different underwater scenes for image enhancement. Transmitting the enhanced features to FFMS for feature extraction facilitates frequency information identification, improving the efficiency and accuracy of information extraction.
3.3 IFI module
In Decoder, guaranteeing the complete usefulness of the extracted frequency feature is severe, potentially obtaining interference that misleads the feature restoration. Additionally, the limitations of the convolution operator, such as static arithmetic, translation invariance, and data dependency, hinder FFMS from fully utilizing the frequency features, which lack in-depth information digging.
Therefore, we design an asymmetric Encoder-Decoder architecture, integrating the Information Flow Interaction (IFI) module into the Decoder to further filter features and accelerate the circulation and fusion of feature information. The IFI is shown in Figure 5. A dual-pass modulator, similar to channel attention, adjusts the high-frequency and low-frequency feature weights, filtering unhelpful information. Merging them yields rich and refined feature information, which isolates noise, accelerating information flow. Unlike traditional integration methods, such as concatenation and addition, we perform element-wise addition of the two features via channel crossing before channel concatenation, thereby enhancing feature exploitation efficiency and gradient propagation through intensive fusion. The specific process is as follows.
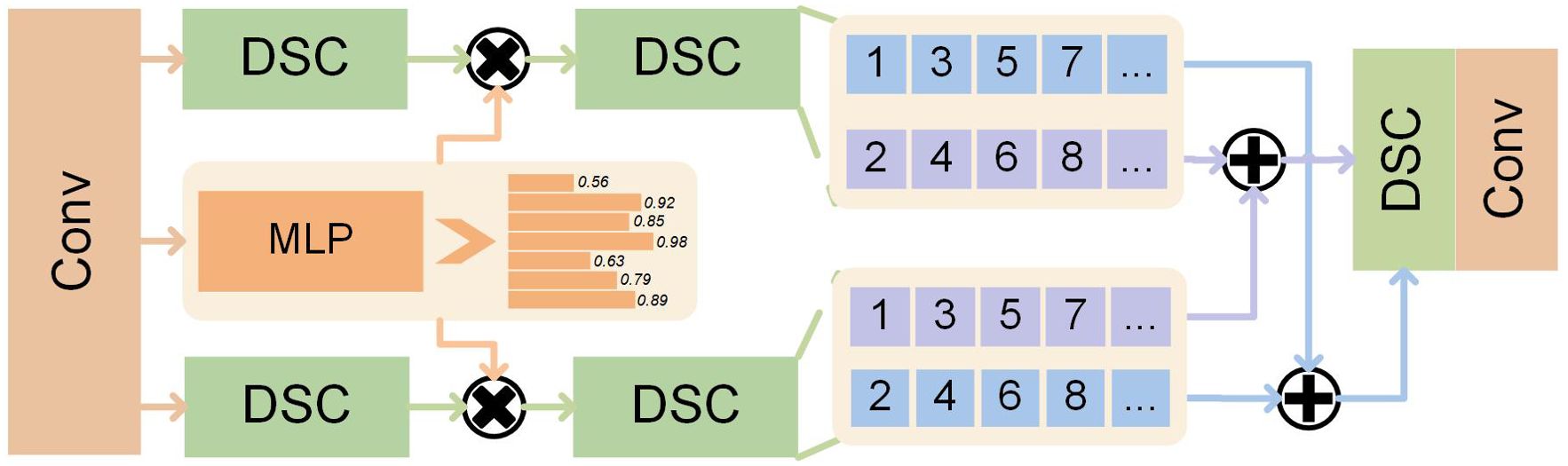
Figure 5. IFI structure. The features are passed through a multilayer perceptron to obtain the frequency domain weights, which are tuned to the low-frequency and high-frequency information respectively, and finally cross-fusion to obtain the compact frequency domain features.
where is defined as.
Subsequently, the two features are fused.
where and are defined as, respectively.
where C is the number of channels for features. denotes the feature map for obtaining the ith channel.
IFI can favorably filter out interference and accelerate information flow, ensuring immune noise in the upsampling process and improving UIE efficiency and performance.
3.4 MJ-Loss loss function
Designing the loss function is the key to improving the network’s stability and generalization. A welldesigned loss function can encourage the network to explore the optimal path from various perspectives, accelerating the network convergence rate.
Enabling the network to capture multi-scale features by Multi-scale loss is pivotal for handling objects containing various sizes, shapes, and complexity. The method has demonstrated its superiority in image tasks, including image enhancement, object detection, and image segmentation. We propose a Multi-scale Joint Loss (MJ-Loss) to guide network training. Each subnetwork generates the enhanced image, calculating and adding the loss to obtain the final loss function. The overall loss is defined as follows.
where is the loss of the subnetwork in ith layer. In , mean absolute error (MAE) as the primary loss function is defined as follows.
where N is the number of samples, and are ground truth and predicted values, respectively.
Furthermore, adding an auxiliary loss can further improve the network optimization and performance, facilitating the gradient flow and encouraging the network to learn more valuable information from various stages of the UIE. Therefore, the article primarily utilizes frequency domain feature extraction as the network core, and the addition of frequency domain loss corresponds to the network, enhancing the network’s performance and efficiency in frequency domain extraction. The frequency domain loss is defined as follows.
where is the Fourier transform (FFT). Subsequently, the overall loss is denoted by.
where α and β are correlation coefficients. , as the main loss function, improves the image spatial domain recovery. , as an auxiliary constraint, ensures the network optimization for the image frequency domain characteristics. We set α to 0.9 and β to 0.1 as the optimal loss weights after extensive experiments.
In UIE tasks, the MJ-Loss can accelerate the network convergence, accurately locating the prominent features of the underwater image. Moreover, with the model characteristics, the loss function encourages the network to optimize issues from multiple perspectives, reaching the optimal gradient direction.
4 Experiments
4.1 Experiment settings
4.1.1 Datasets
To comprehensively evaluate the effectiveness of MUFFNet in UIE, we value its effectiveness on various datasets, including the renowned UIEB and the refined LUSI. LUSI contains 4,279 images selected elaborately from diverse public underwater datasets, which exhibit high quality and generalization Peng et al. (2023). MUFFNet is trained mainly using LSUI, divided into 2,996 images for training, 856 for testing, and 430 for validation. UIEB, divided into 624 images for training and 179 for validation, evaluates the robustness and expression capabilities of MUFFNet, which possesses more noise and low-quality images. In addition, 60 challenging no-reference images and the RUIE dataset were further compared with SOTA methods, validating the MUFFNet performance in different underwater scenes.
4.1.2 Evaluation metrics
For reference data testing, we utilize various metrics to certify the model performance from different perspectives. PSNR and SSIM series, including SSIM, F-SIM, MS-SSIM, and CW-SSIM, served as the primary metrics to evaluate the image enhancement effect, reflecting the gap between the enhanced image and the ground truth. A higher PSNR denotes closer image contents, while a higher SSIM series indicates closer structural and textural similarity. Remarkably, the SSIM series metrics from multiple perspectives assess the gap between images. SSIM focuses on brightness, contrast, and structural similarity. F-SIM emphasizes texture features and is used to evaluate image texture richness. MS-SSIM better captures image structural information at different resolutions through multi-scale computation and is used to evaluate high-resolution images. CW-SSIM enhances the processing of high-frequency textures and details through wavelet transform and is used to evaluate images with complex details. In addition, Learned Perceptual Image Patch Similarity (SPIPS) and Visual Information Fidelity (VIF), as extra metrics, are used to measure the perceptual similarity and fidelity between images, providing criteria based on human visual perception.
For non-reference data testing, we employ PI, UIQA, Entropy, CEF, UCIQE, UICM, and URanker (Guo et al., 2023). Higher Entropy and UIQA donate more information and higher actual quality of underwater images. UCIQE, UICM, and URanker serve as metrics for evaluating underwater images. Higher values generally indicate better image quality, aligning more closely with human perception of high-quality underwater images. PI combines various evaluation methods, integrating low-level visual features and high-level perceptual features to gain full-quality assessment, in which lower PI denotes a smaller negative impact factor of the image. Moreover, CEF evaluates the image contrast, with better values indicating stronger pixel contrast and color gap. However, the above no-reference image metrics cannot completely represent the image quality, as models with high values in metrics tend to produce worse image quality in subsequent experiments.
4.1.3 Implementation details
The experiment environments for training and testing include Window-10, 256G RAM, Inter Xeon Gold 5318Y CPU (2.10GHz), and NVIDIA A10 GPU. The compilation environment consists of Python 3.9.19 and Pytorch 2.1.1+cu121. During training the network, the training images are resized to a fixed size of 256×256 and normalized to [0,1], followed by augmentation through random flipping and rotation. Using the Cosine Annealing strategy for learning rate decay, the learning rate was initially 0.0025. Using the AdmaW gradient optimization strategy to update the gradient, the trained epochs are 500, including 20 epochs for warm-up training.
4.2 Performance comparison
4.2.1 Performance comparison on the LSUI dataset
Using the LSUI dataset trains MUFFNet, comparing SOTA networks in identical experimental environments to verify the MUFFNet superiority. The comparison of performance metrics for the networks is presented in Table 2, where MUFFNet demonstrates superior performance across all indicators, reducing resource consumption while achieving outstanding image quality. Although GAN-based networks exhibit acceptable inference speeds, they incur higher resource consumption and show no substantial improvements in image quality. This is because GANs are constrained by the need for sufficient and high-quality training data, which hinders stable training, leading to insufficient generalization ability and the potential generation of fake information when encountering unfamiliar underwater scenes. Due to the dynamically complex characteristics of underwater scenes, physical model-based UIE methods fail to achieve an optimal trade-off between image quality and inference speed in various underwater environments. Although the EncoderDecoder network U-Shape achieves PSNR and SSIM scores of 24.514 and 0.912, respectively, falling short of MUFFNet by 4.177 and 0.044, it consumes excessive resources, making it less suitable for optimal deployment in UIE. The enhanced effect on LUSI is shown in Figure 6A. The underwater images generated by MUFFNet are the closest to real-world scenes, effectively eliminating the color gap and aligning with human perception. Physical model-based methods, lacking universality, suffer from severe color deviations and unrealistic hues when confronted with challenging underwater scenes. It causes severe interference with downstream tasks such as object detection, trajectory planning, and operator observation. In comparison, images enhanced by GANs are closer to real-world scenes and achieve higher PSNR. However, the image details contain numerous artifacts, as shown in Figure 6B, which cause significant interference with downstream tasks. For example, the network performance may be affected by potentially extracted noise information in underwater object detection.
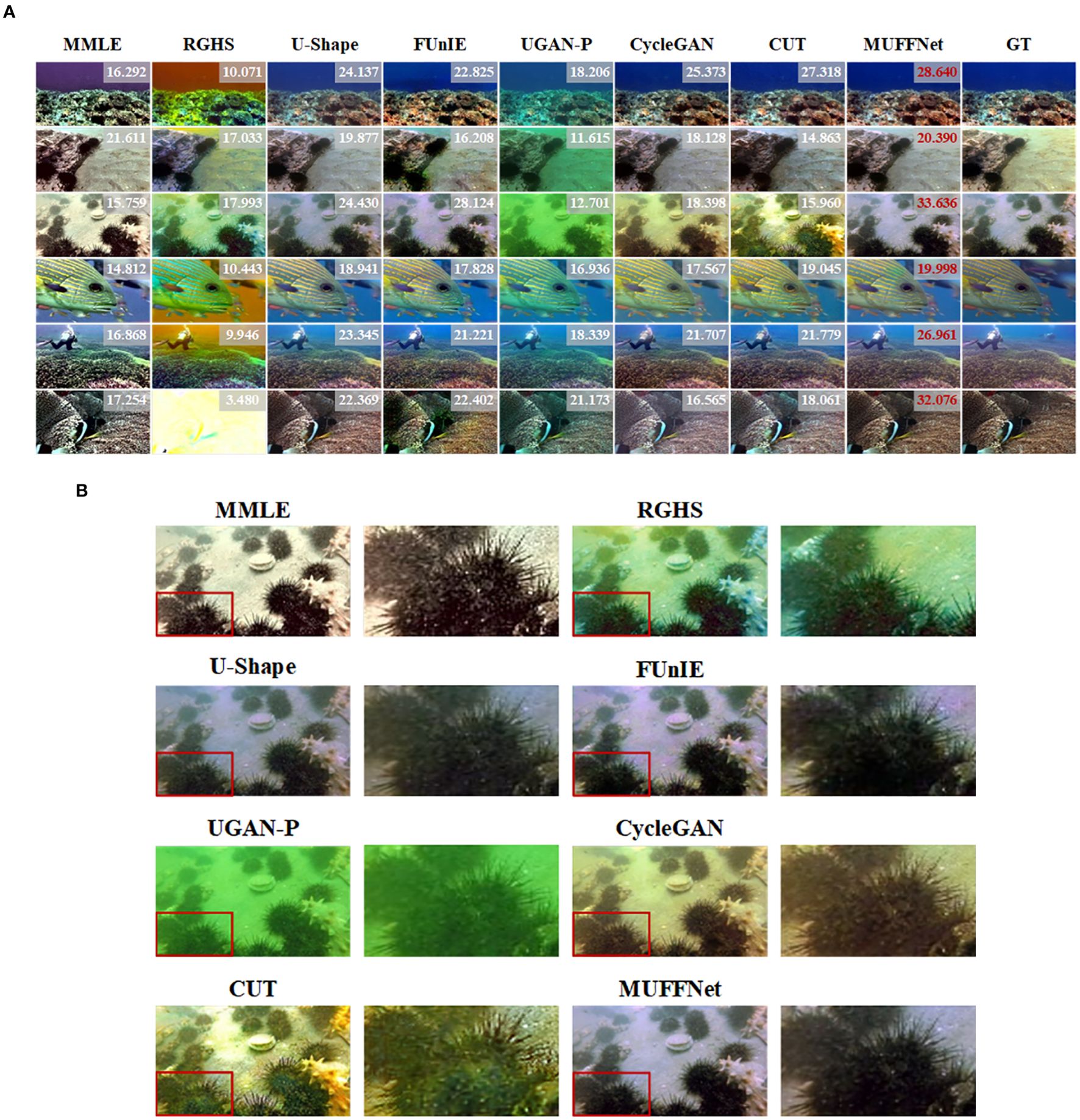
Figure 6. Comparison results on the LSUI dataset. (A) Comparison effects on the LSUI dataset. The value in the upper right corner of the image is PSNR. (B) Detailed comparison on the LSUI dataset.
Experimental results on LSUI show that MUFFNet can generate images that closely resemble realworld underwater scenes, effectively suppressing color deviation. By leveraging the frequency domain information from the image, MUFFNet separates and weights different frequency components, which avoids noise interference in the spatial domain, effectively handling optical degradation and the impact of underwater impurities on the image. Remarkably, MUFFNet, the prerequirement of downstream tasks, provides high-quality images that reduce the influence of image noise and enhance the performance of downstream tasks.
4.2.2 Performance comparison on the UIEB dataset
We conduct training and comparison on the UIEB dataset to further verify the model robustness, in which the dataset includes underwater images with different depths, quality, and illumination, which present challenges for model performance. As shown in Table 2, although the PSNR dwarfs LSUI, MUFFNet achieves superior PSNR, SSIM, and PI scores compared to other algorithms, demonstrating its robustness in complex underwater environments. The enhancement effect is compared in Figure 7A, where MUFFNet produces images that align with real-world perception when handling underwater scenes involving multimotion and noise. Conversely, GAN-based models generate noticeable artifacts, and physics-based models exhibit significant color casts and particles, as detailed in Figure 7B. Due to the small dataset size and the diverse underwater environments in UIEB, regular artifacts from GANs negatively affect downstream task performance. In contrast, physics-based methods show varying degrees of sharpening and color shifts, creating noticeable gaps between the enhanced and real-world images. MUFFNet strikes an optimal trade-off in overall image quality, suppressing noise and enhancing edge detail quality through targeted inspection and screening of frequency domain information.
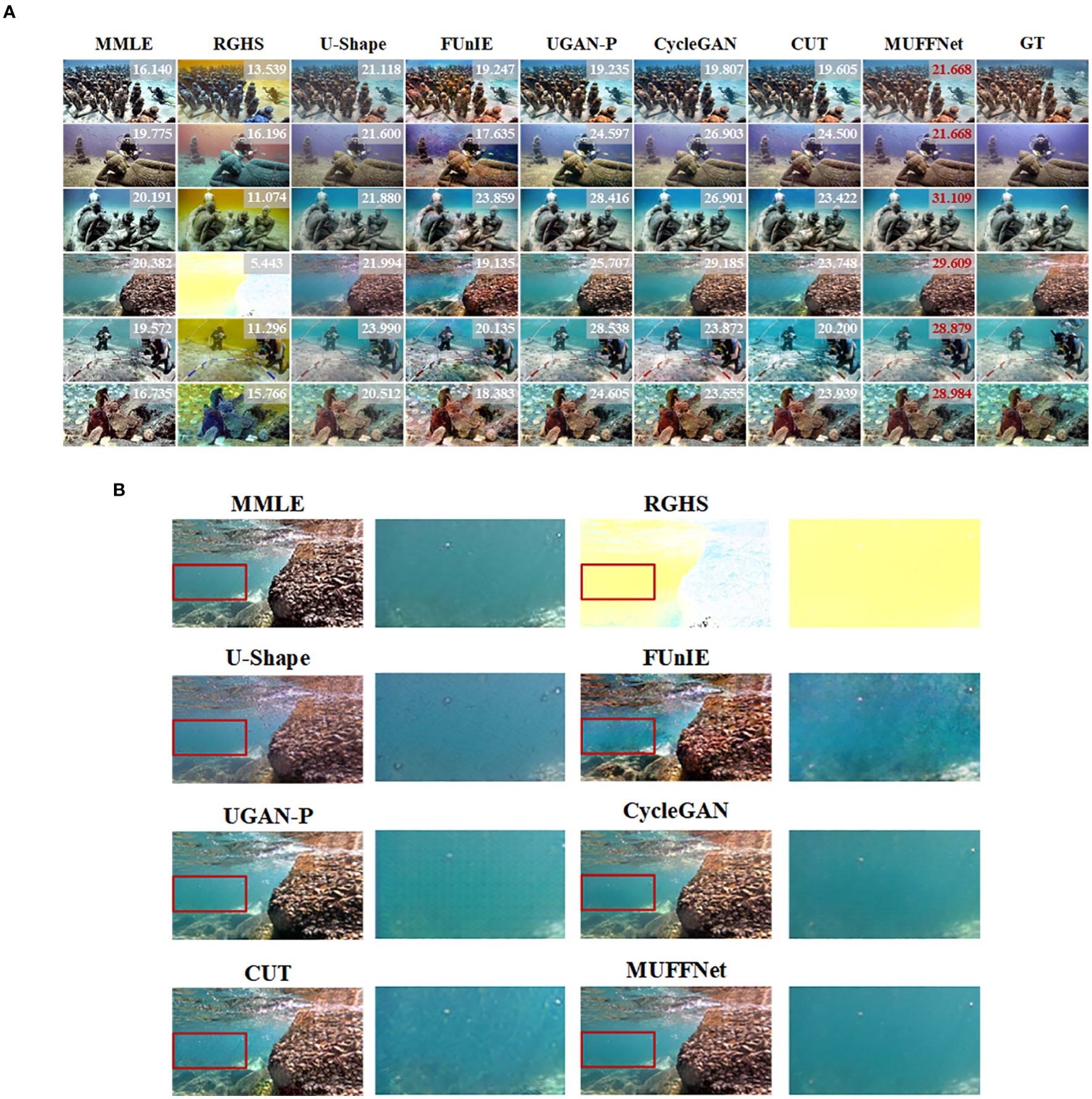
Figure 7. Comparison results on the UIEB dataset. (A) Comparison effects on the UIEB dataset. The value in the upper right corner of the image is PSNR. (B) Detailed comparison on the UIEB dataset.
For more severely degraded images, MUFFNet demonstrates superior enhancement capabilities. The initial enhancement of underwater images effectively mitigates degradation issues and provides the network with rich feature information. By integrating frequency domain feature extraction, the model improves the digging of potentially valuable information within the image, thereby improving the robustness of the network.
4.2.3 Performance comparison on the Challenage-60 dataset
The above experiments are in reference images, which cannot fully reflect the effectiveness in real-world applications. Therefore, we evaluate MUFFNet using non-reference images from the UIEB dataset to obtain a more objective and credible assessment, which presents challenges for model performance in practical applications. The non-reference metric comparison, shown in Table 3, reveals that MUFFNet performs comparably to baseline methods and even surpasses them on certain metrics. Notably, non-reference metrics focus narrowly on specific aspects of images, such as contrast, color, and brightness, while neglecting overall quality. The single non-reference metric possesses a large gap with human eye perception, which cannot objectively reflect real-world enhanced image quality.
As illustrated in Figure 8A, although some methods outperform MUFFNet in quantitative metrics, they produce inferior results in actual image rendering quality. Figure 8B demonstrates that MMLE and RGHS, which are physical model-based algorithms, achieve decent PI values but result in real-world image effects that are significantly worse, with severe color deviations and noise. Similarly, GANs and U-Shape also exhibit artifacts and blurring, even when their PI metrics are higher than those of MUFFNet. While MUFFNet performs less effectively on CEF, its enhanced images display sharper details and more regular structures, better aligning with human visual perception.
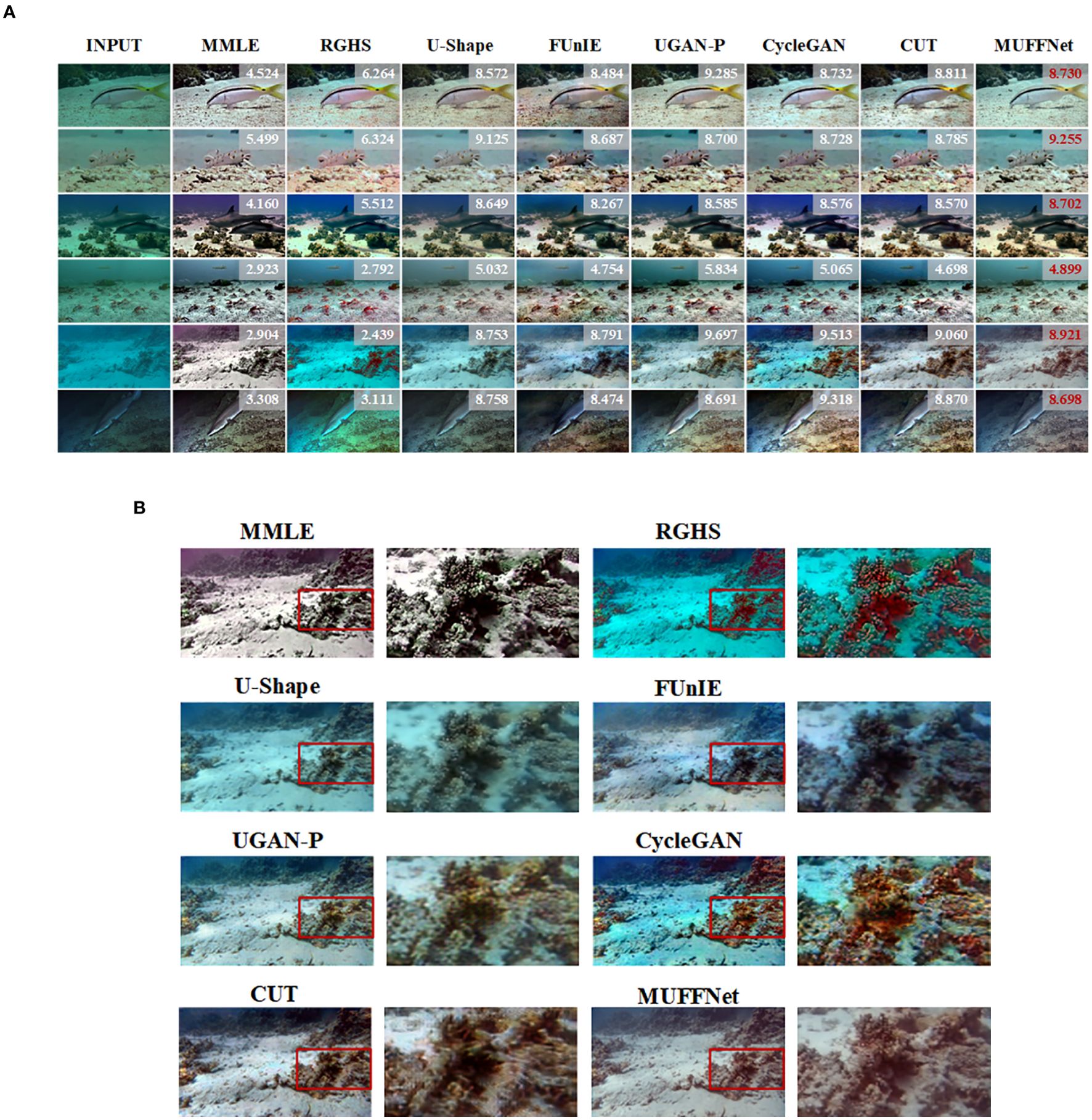
Figure 8. Comparison results on the challenage-60. (A) Comparison effects on the challenage-60. The value in the upper right corner of the image is PI. (B) Detailed comparison on the challenge-60.
The experimental results demonstrate that MUFFNet has robust performance and strong generalization capabilities. By extracting features from the underwater frequency domain, MUFFNet enhances edge details while effectively suppressing noise, producing sharper and more realistic underwater images. Leveraging frequency-domain-based convolution attention and Multi-level Joint Loss, MUFFNet simultaneously focuses on local details and the global context, striking an optimal trade-off between structural integrity and detailed enhancement.
4.2.4 Performance analysis
As shown in Figure 8, while most enhanced underwater images exhibit improved detail clarity and overall visualization, challenges remain in extreme environments with high water turbidity or poor lighting. In such scenarios, the high-frequency noise in the background water body may be overly extracted, obscuring key details within the image. This over-extraction can introduce slight noise and blur in the background, reducing image clarity and negatively affecting human visual perception.
Additionally, in underwater scenes with extreme color bias, the network may struggle to exploit the potential information within the image fully. As a result, the enhanced images may exhibit slight color distortions. Although contrast and detail are improved, the overall color tone may deviate from the real-world scene, leading to an unnatural visual effect. This issue primarily arises from the model’s limitations in managing global color balance during the frequency domain information extraction process, making it less effective in handling extreme underwater color biases.
In the future, we plan to integrate additional environmental features, such as depth information and prior knowledge of water bodies, to enable targeted optimizations. Furthermore, we will explore the combined processing of multi-domain information to enhance the network’s ability to deliver superior enhancement effects, especially in extreme underwater environments.
4.3 Ablation experiments
4.3.1 Generalization
Using UIQS and UCCS subsets of the RUIE dataset further validates the network robustness in complex underwater scenes. The UIQS subset is divided into five groups of data [A,B,C,D,E], in which the underwater complexity and depth level increase progressively from group A to D. Based on underwater color deviation, the UCCS subset is divided into three groups, blue, green, and blue-green, to evaluate the model’s performance under varying color deviations. The enhancement results are shown in Figure 9, where MUFFNet demonstrates superior performance in diverse and complex underwater environments. As underwater conditions become increasingly challenging, baseline networks produce augmented images that gradually exhibit blurring and artifacts. Moreover, baseline networks struggle to achieve a balanced state, resulting in a commonplace performance across different tones. Conversely, MUFFNet effectively mitigates these issues, delivering a superior enhancement effect across varying tones.
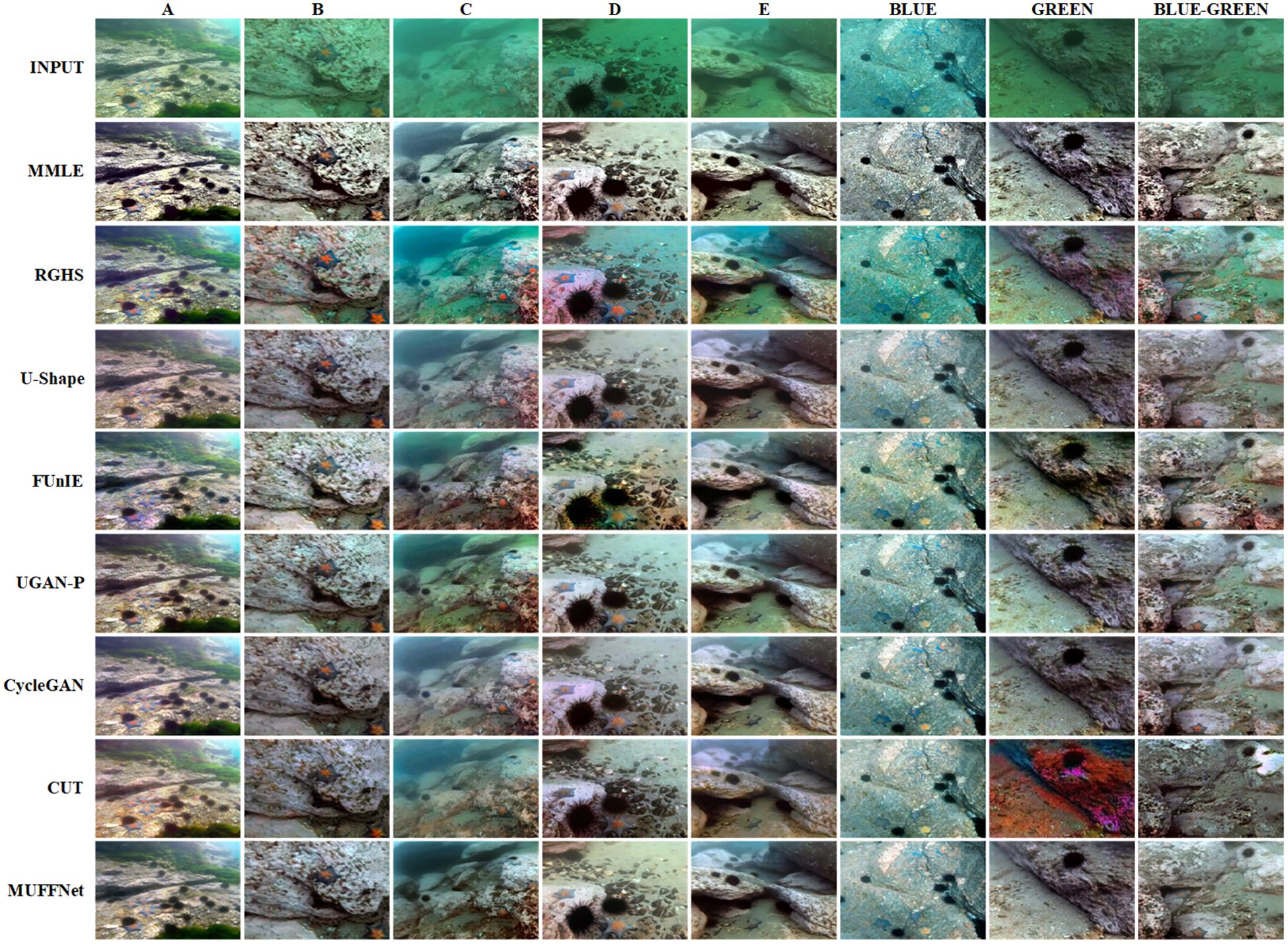
Figure 9. Comparison effects on the UIQS and UCCS subsets of the RUIE dataset. The UIQS subset is divided into five groups of data (A-E), in which the underwater complexity and depth level increase progressively from group A to D. The UCCS subset is divided into three groups, blue, green, and blue-green.
4.3.2 Multi-scale Enhancement Prior
To validate the beneficial effect of Multi-scale Enhancement Prior in MUFFNet, gradually removing MEP submodules was trained and compared in a unified environment. The comparison results, presented in Table 4, reveal no significant fluctuations in evaluation metrics. However, the visual effect comparison in Figure 10A underscores the critical role of MEP in image refinement. Without the Enhancement Prior, MUFFNet lacks abundant representation information and detail fidelity, resulting in insufficient insight into crucial location information and generating incongruous image edges. Similarly, removing the Multi-scale Prior causes the network to lose multi-scale information, accelerating information forgetting and narrowing the receptive field. Consequently, enhanced images exhibit severe detail deviation and imbalances in overall tone. The MEP-equipped MUFFNet, on the other hand, receives prior knowledge containing prominent information in advance, enabling it to extract multi-scale highlights and prolong information retention. Figure 10B demonstrates the impact of MEP, which enhances image edge details and global smoothness, effectively highlighting pivotal features essential for image restoration.
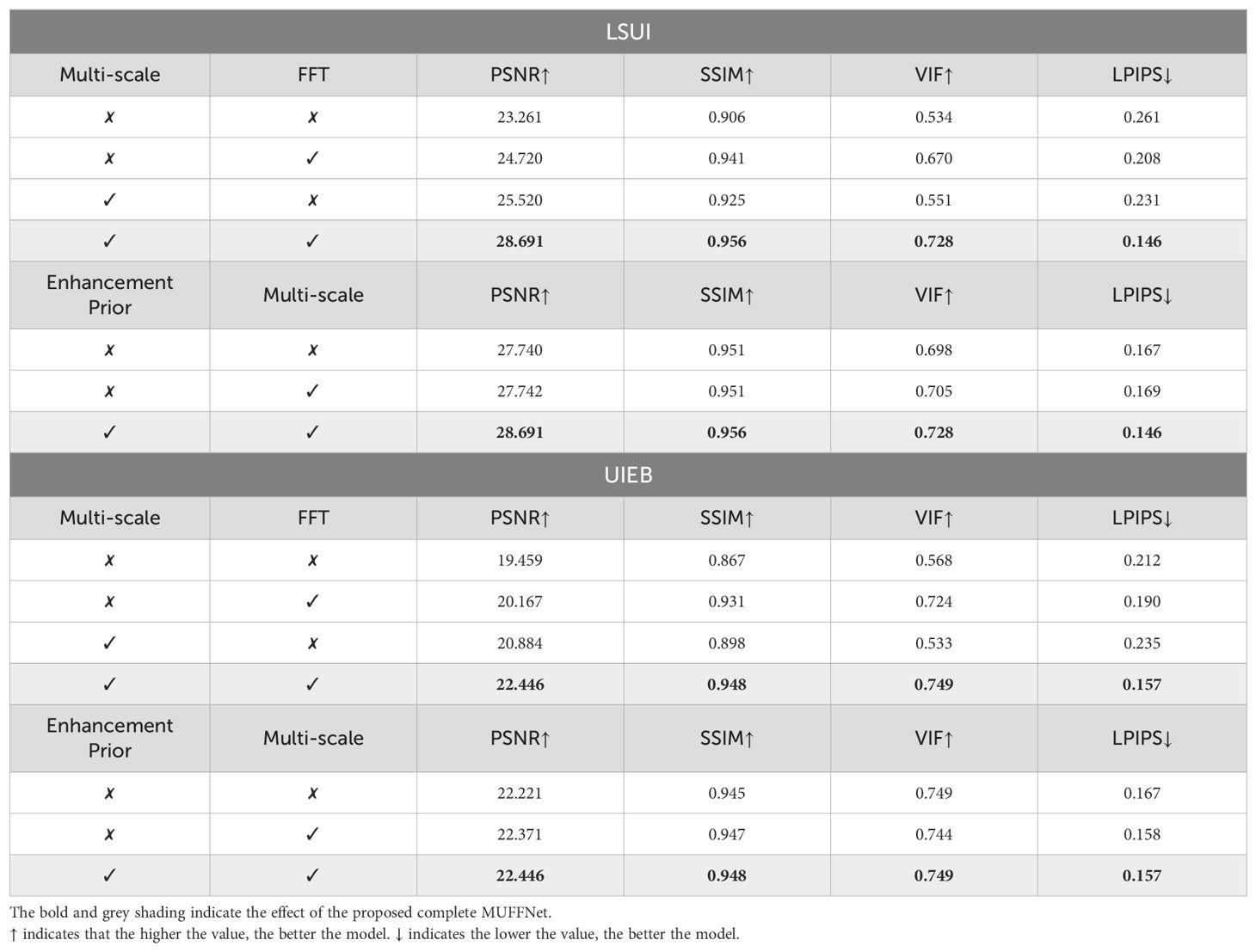
Table 4. Metrics comparison of model effects using different modules (Enhancement Prior and Multi-scale) and loss (L1, FFT and Multi-scale).
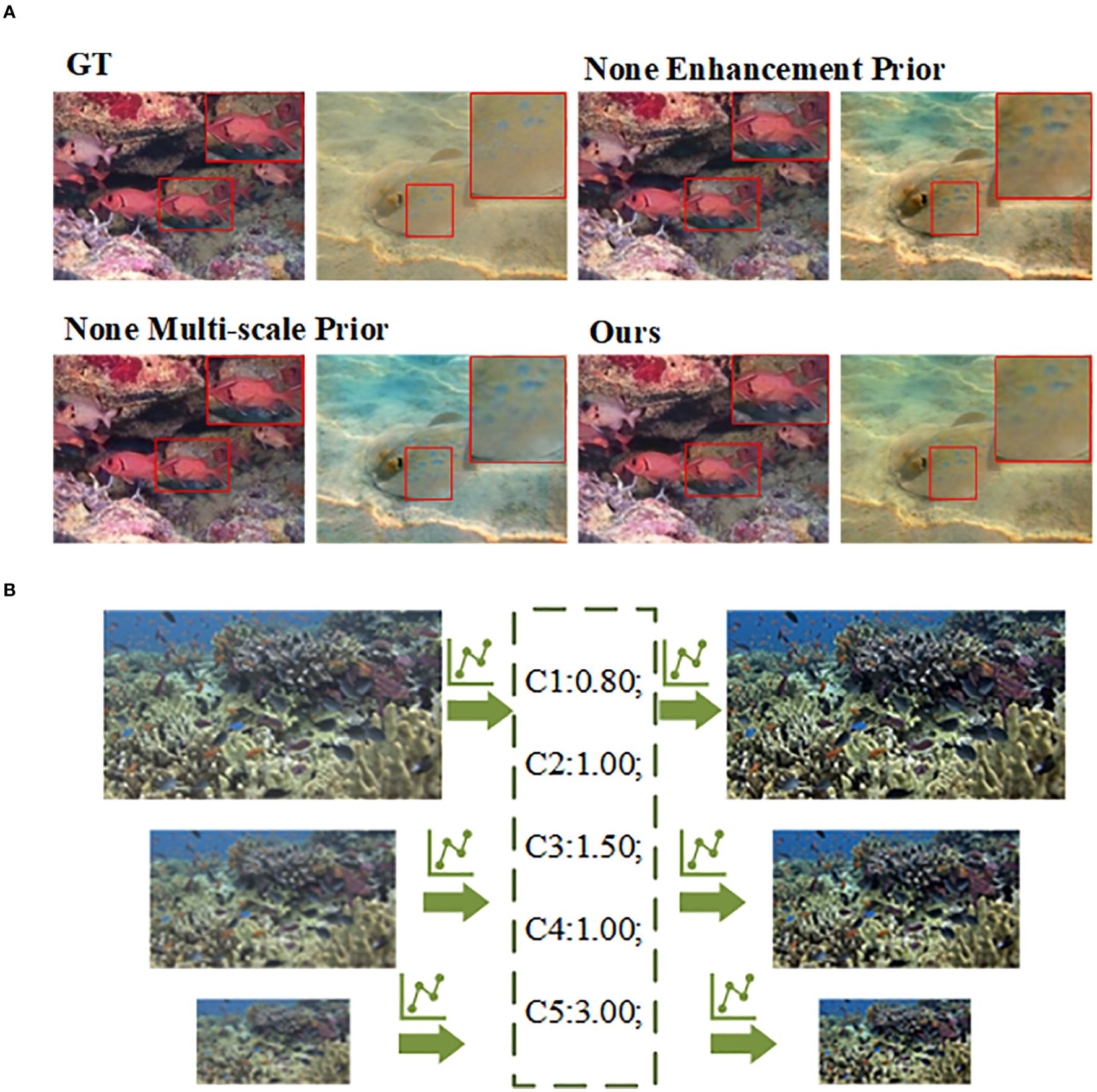
Figure 10. Results of ablation experiments with Multi-scale Enhancement Prior. (A) Detailed comparison using different modules (Enhancement Prior and Multi-scale). (B) MEP enhancement process.
4.3.3 Multi-scale Joint Loss
We employed various loss functions to train the network and conducted comparisons to validate the superiority of the Multi-scale Joint Loss. Table 4 illustrates model performance significantly decreased when removing the FFT loss and multi-scale loss functions. Incorporating FFT loss into the training process improves the model’s performance, demonstrating its beneficial impact. Building on this, adding the multi-scale loss strategy further enhances the network, delivering notably improved results. The image enhancement comparisons in Figure 11 highlight the advantages of our proposed optimization strategy. Networks trained without these components fail to dynamically capture critical features, making them unable to identify an optimal mapping space. It is prone to falling into local optima along a fixed path in complex environments in complex environments, resulting in suboptimal enhancement effects. In contrast, our proposed MJ-Loss accounts for both frequency and spatial domains, enabling the model to determine the optimal scheme and achieve superior imaging results.
4.4 Downstream task evaluation
To evaluate the effectiveness of MUFFNet on downstream tasks, we utilized the Scale-Invariant Feature Transform (SIFT) algorithm to detect feature points on enhanced images. We assessed the feature-matching accuracy to quantify the network’s capability to preserve image details. Additionally, the Canny edge detection operator was employed to identify edges in the images processed by MUFFNet. The edge pixel percentage and the edge continuity scores are analyzed to validate the enhancement performance of MUFFNet in edge clarity and consistency.
As shown in Figure 12 and Table 5, MUFFNet generates informative images that facilitate feature point extraction and edge detection while effectively reducing artifacts and noise in the original images. This improvement enhances the performance of downstream tasks. Although MMLE achieves the highest number of extracted feature points, its feature-matching accuracy is unsatisfactory. It is because MMLE amplifies the irrelevant interference within the image, leading to significant noise in the extracted features. Similarly, images processed by MMLE exhibit substantial interference after edge detection, which diminishes the clarity and continuity of edge details. For GAN series networks, missing feature points and blurred edges are observed. These limitations arise from their inability to sufficiently enhance the original image, resulting in a loss of critical feature information. The U-Shape network potentially amplifies some noise in the image, which is suboptimal in both feature point and edge detection. In contrast, MUFFNet demonstrates superior performance by extracting abundant features while achieving higher feature-matching accuracy. It indicates that MUFFNet effectively suppresses most of the noise and focuses on extracting more meaningful information from the images.
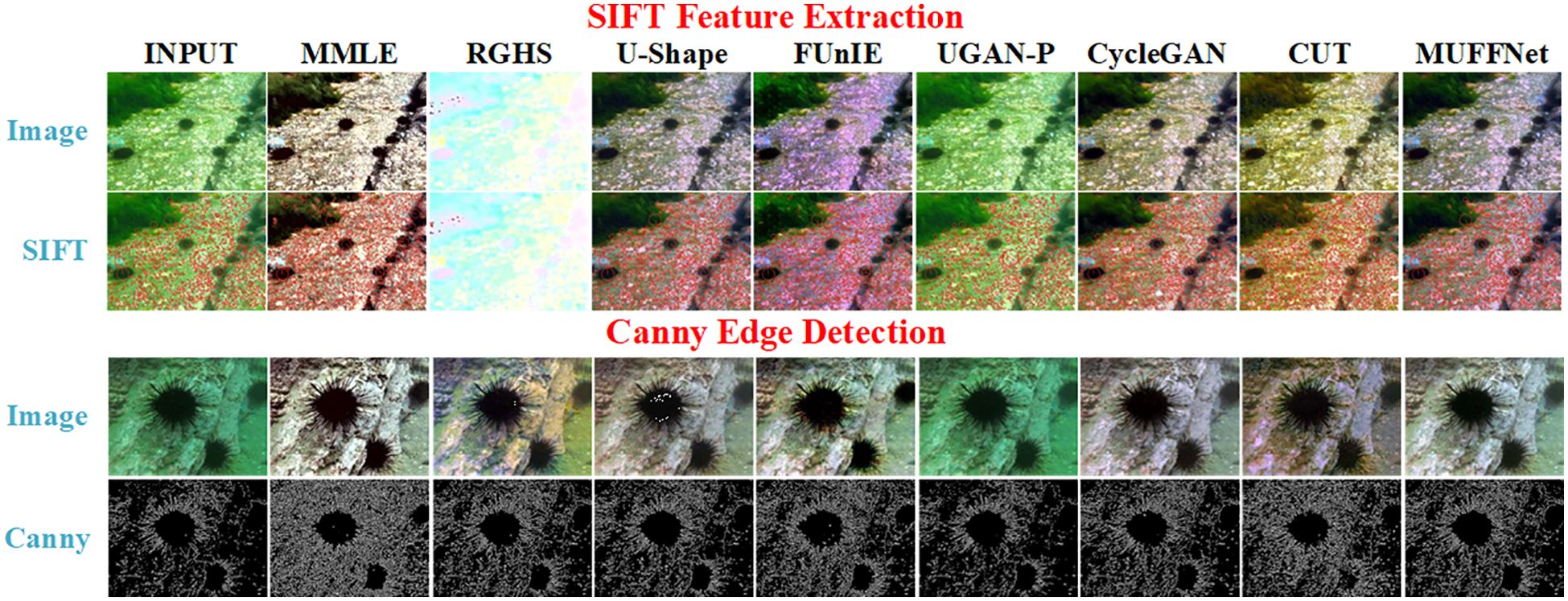
Figure 12. Downstream task effect comparison, including SIFT feature extraction and Canny edge detection.
Overall, MUFFNet produces high-quality images that enhance the performance of downstream tasks, thereby demonstrating the practical benefits of its enhancement capabilities. Images enhanced by MUFFNet align with the naturalness of human visual perception and optimize the quality of input data for critical tasks such as underwater object detection and recognition, improving the accuracy and robustness of the model.
5 Conclusion
This article proposes a dynamic underwater enhancement network based on multi-scale frequency, MUFFNet, to offer high-resolution underwater images for U-HRI. Recognizing the critical role of high-frequency and low-frequency information in UIE, a frequency-domain-based convolution attention mechanism is proposed to extract deep frequency domain features. Introducing a Multi-scale Enhancement Prior algorithm boosts frequency domain information extraction, generating unique enhancement parameters tailored to different underwater scenes and enriching the network with abundant frequency domain information via a multi-scale approach. An Information Flow Interaction module and Multiscale Joint Loss are employed to accelerate information flow and fusion while optimizing the network’s convergence trajectory. Experiments demonstrated that MUFFNet outperforms SOTA models in various underwater scenarios while consuming fewer computational resources. The enhanced images generated by MUFFNet align more closely with human visual perception, providing downstream tasks with clear and reliable images and significantly reducing the risk of misinterpretation. In the future, we aim to integrate MUFFNet with downstream tasks, such as underwater object detection, further advancing research in underwater tasks.
Data availability statement
The original contributions presented in the study are included in the article/supplementary material. Further inquiries can be directed to the corresponding author.
Author contributions
DK: Writing – review & editing, Writing – original draft, Conceptualization. YZ: Writing – original draft, Writing – review & editing, Methodology. XZ: Supervision, Writing – review & editing. YW: Writing – review & editing. LC: Writing – review & editing.
Funding
The author(s) declare financial support was received for the research, authorship, and/or publication of this article. This study was supported in part by the Science and Technology Project of Henan Province under Grant nos.232102211070, 242102211025, the Key Scientific Research Projects of Colleges and Universities of Henan Province under Grant no. 23B520009, and the Henan Provincial focus on research and development Project under Grant no. 231111220700.
Conflict of interest
The authors declare that the research was conducted in the absence of any commercial or financial relationships that could be construed as a potential conflict of interest.
Generative AI statement
The author(s) declare that no Generative AI was used in the creation of this manuscript.
Publisher’s note
All claims expressed in this article are solely those of the authors and do not necessarily represent those of their affiliated organizations, or those of the publisher, the editors and the reviewers. Any product that may be evaluated in this article, or claim that may be made by its manufacturer, is not guaranteed or endorsed by the publisher.
References
An S., Xu L., Senior Member I., Deng Z., Zhang H. (2024). Hfm: A hybrid fusion method for underwater image enhancement. Eng. Appl. Artif. Intell. 127, 107219. doi: 10.1016/j.engappai.2023.107219
Bi X., Wang P., Guo W., Zha F., Sun L. (2024). Rgb/event signal fusion framework for multidegraded underwater image enhancement. Front. Mar. Sci. 11, 1366815. doi: 10.3389/fmars.2024.1366815
Birk A. (2022). A survey of underwater human-robot interaction (u-hri). Curr. Robotics Rep. 3, 199–211. doi: 10.1007/s43154-022-00092-7
Cai X., Jiang N., Chen W., Hu J., Zhao T. (2023). Cure-net: a cascaded deep network for underwater image enhancement. IEEE J. oceanic Eng. 49, 226–236. doi: 10.1109/JOE.2023.3245760
Cheng J., Wu Z., Wang S., Demonceaux C., Jiang Q. (2023). Bidirectional collaborative mentoring network for marine organism detection and beyond. IEEE Trans. Circuits Syst. Video Technol. 33, 6595–6608. doi: 10.1109/TCSVT.2023.3264442
Cong R., Yang W., Zhang W., Li C., Guo C.-L., Huang Q., et al. (2023). Pugan: Physical model-guided underwater image enhancement using gan with dual-discriminators. IEEE Trans. Image Process. 32, 4472–4485. doi: 10.1109/TIP.2023.3286263
Cong S., Zhou Y. (2023). A review of convolutional neural network architectures and their optimizations. Artif. Intell. Rev. 56, 1905–1969. doi: 10.1007/s10462-022-10213-5
Fabbri C., Islam M. J., Sattar J. (2018). “Enhancing underwater imagery using generative adversarial networks,” in 2018 IEEE international conference on robotics and automation (ICRA). (Brisbane, QLD, AustraliaIEEE International Conference on Robotics and Automation (ICRA)). 7159–7165, doi: 10.1109/ICRA.2018.8460552
Fulton M., Sattar J., Absar R. (2023). Siren: Underwater robot-to-human communication using audio. IEEE Robotics Automation Lett. 8, 6139–6146 doi: 10.1109/LRA.2023.3303719
González-Sabbagh S. P., Robles-Kelly A. (2023). A survey on underwater computer vision. ACM Computing Surveys 55, 1–39. doi: 10.1145/3578516
Guo C., Wu R., Jin X., Han L., Zhang W., Chai Z., et al. (2023). “Underwater ranker: Learn which is better and how to be better,” in Proceedings of the AAAI conference on artificial intelligence, Vol. 37. 702–709. doi: 10.1609/aaai.v37i1.25147
He Z., Ran W., Liu S., Li K., Lu J., Xie C., et al. (2023). Low-light image enhancement with multi-scale attention and frequency-domain optimization. IEEE Trans. Circuits Syst. Video Technol. 34 (4), 2861–2875. doi: 10.1109/TCSVT.2023.3313348
Hong J., Enan S. S., Sattar J. (2024). Diver identification using anthropometric data ratios for underwater multi-human-robot collaboration. IEEE Robotics Automation Lett. 9 (4), 3514–3521. doi: 10.1109/LRA.2024.3366026
Hou G., Li N., Zhuang P., Li K., Sun H., Li C. (2023). Non-uniform illumination underwater image restoration via illumination channel sparsity prior. IEEE Trans. Circuits Syst. Video Technol. 34 (2), 799–814. doi: 10.1109/TCSVT.2023.3290363
Howard A. G., Zhu M., Chen B., Kalenichenko D., Wang W., Weyand T., et al. (2017). Mobilenets: Efficient convolutional neural networks for mobile vision applications. arXiv preprint arXiv:1704.04861.
Hu K., Weng C., Shen C., Wang T., Weng L., Xia M. (2023). A multi-stage underwater image aesthetic enhancement algorithm based on a generative adversarial network. Eng. Appl. Artif. Intell. 123, 106196. doi: 10.1016/j.engappai.2023.106196
Huang D., Wang Y., Song W., Sequeira J., Mavromatis S. (2018). “Shallow-water image enhancement using relative global histogram stretching based on adaptive parameter acquisition,” in MultiMedia Modeling: 24th International Conference, MMM 2018, Bangkok, Thailand, February 5-7, 2018, Proceedings, Part I 24. (Springer International Publishing) 453–465.
Islam M. J., Xia Y., Sattar J. (2020). Fast underwater image enhancement for improved visual perception. IEEE Robotics Automation Lett. 5, 3227–3234. doi: 10.1109/LSP.2016
Jiang Q., Kang Y., Wang Z., Ren W., Li C. (2023). Perception-driven deep underwater image enhancement without paired supervision. IEEE Trans. Multimedia. 26, 4884–4897. doi: 10.1109/TMM.2023.3327613
Jin J., Jiang Q., Wu Q., Xu B., Cong R. (2024). Underwater salient object detection via dualstage self-paced learning and depth emphasis. IEEE Trans. Circuits Syst. Video Technol. doi: 10.1109/TCSVT.2024.3491907
Kang Y., Jiang Q., Li C., Ren W., Liu H., Wang P. (2022). A perception-aware decomposition and fusion framework for underwater image enhancement. IEEE Trans. Circuits Syst. Video Technol. 33, 988–1002. doi: 10.1109/TCSVT.2022.3208100
Li Y., Mi Z., Wang Y., Jiang S., Fu X. (2024). Taformer: A transmission-aware transformer for underwater image enhancement. IEEE Trans. Circuits Syst. Video Technol. 35 (1), 601–616. doi: 10.1109/TCSVT.2024.3455353
Liu Q., Zhang Q., Liu W., Chen W., Liu X., Wang X. (2023). Wsds-gan: A weak-strong dual supervised learning method for underwater image enhancement. Pattern Recognition 143, 109774. doi: 10.1016/j.patcog.2023.109774
Liu T., Zhu K., Wang X., Song W., Wang H. (2024). Lightweight underwater image adaptive enhancement based on zero-reference parameter estimation network. Front. Mar. Sci. 11, 1378817. doi: 10.3389/fmars.2024.1378817
Lu H., Li Y., Zhang Y., Chen M., Serikawa S., Kim H. (2017). Underwater optical image processing: a comprehensive review. Mobile Networks Appl. 22, 1204–1211. doi: 10.1007/s11036-017-0863-4
Park T., Efros A. A., Zhang R., Zhu J.-Y. (2020). “Contrastive learning for unpaired image-to-image translation,” in Computer Vision–ECCV 2020: 16th European Conference, Glasgow, UK, August 23–28, 2020, Proceedings, Part IX 16. 319–345 (Springer International Publishing). 319–345.
Peng L., Zhu C., Bian L. (2023). U-shape transformer for underwater image enhancement. IEEE Trans. Image Process. 32, 3066–3079. doi: 10.1109/TIP.2023.3276332
Praczyk T. (2023). Neural control system for a swarm of autonomous underwater vehicles. KnowledgeBased Syst. 276, 110783. doi: 10.1016/j.knosys.2023.110783
Qi Q., Zhang Y., Tian F., Wu Q. J., Li K., Luan X., et al. (2021). Underwater image co-enhancement with correlation feature matching and joint learning. IEEE Trans. Circuits Syst. Video Technol. 32, 1133–1147. doi: 10.1109/TCSVT.2021.3074197
Rao Y., Liu W., Li K., Fan H., Wang S., Dong J. (2023). Deep color compensation for generalized underwater image enhancement. IEEE Trans. Circuits Syst. Video Technol. 34 (4), 2577–2590. doi: 10.1109/TCSVT.2023.3305777
Ummar M., Dharejo F. A., Alawode B., Mahbub T., Piran M. J., Javed S. (2023). Window-based transformer generative adversarial network for autonomous underwater image enhancement. Eng. Appl. Artif. Intell. 126, 107069. doi: 10.1016/j.engappai.2023.107069
Vaswani A., Shazeer N., Parmar N., Uszkoreit J., Jones L., Gomez A. N., et al. (2017). Attention is all you need. Adv. Neural Inf. Process. Syst. 30, 5998–6008.
Wang H., Köser K., Ren P. (2025). Large foundation model empowered discriminative underwater image enhancement. IEEE Trans. Geosci. Remote Sens. 1–1 doi: 10.1109/TGRS.2025.3525962
Wang H., Sun S., Ren P. (2023). Underwater color disparities: Cues for enhancing underwater images toward natural color consistencies. IEEE Trans. Circuits Syst. Video Technol. 34, 738–753. doi: 10.1109/TCSVT.2023.3289566
Wang Z., Xiang X., Duan Y., Yang S. (2024b). Adversarial deep reinforcement learning based robust depth tracking control for underactuated autonomous underwater vehicle. Eng. Appl. Artif. Intell. 130, 107728. doi: 10.1016/j.engappai.2023.107728
Wang H., Zhang W., Ren P. (2024a). Self-organized underwater image enhancement. ISPRS J. Photogrammetry Remote Sens. 215, 1–14. doi: 10.1016/j.isprsjprs.2024.06.019
Wang P., Zhu H., Huang H., Zhang H., Wang N. (2021). Tms-gan: A twofold multi-scale generative adversarial network for single image dehazing. IEEE Trans. Circuits Syst. Video Technol. 32, 2760–2772. doi: 10.1109/TCSVT.2021.3097713
Wu Z., Liu W., Li J., Xu C., Huang D. (2023). Sfhn: spatial-frequency domain hybrid network for image super-resolution. IEEE Trans. Circuits Syst. Video Technol. 33, 6459–6473. doi: 10.1109/TCSVT.2023.3271131
Xia P., Xu F., Song Z., Li S., Du J. (2023a). Sensory augmentation for subsea robot teleoperation. Comput. Industry 145, 103836. doi: 10.1016/j.compind.2022.103836
Xia P., You H., Ye Y., Du J. (2023b). Rov teleoperation via human body motion mapping: Design and experiment. Comput. Industry 150, 103959. doi: 10.1016/j.compind.2023.103959
Xu S., Zhang M., Song W., Mei H., He Q., Liotta A. (2023). A systematic review and analysis of deep learning-based underwater object detection. Neurocomputing 527, 204–232. doi: 10.1016/j.neucom.2023.01.056
Yang J., Wei P., Zheng N. (2023). Cross time-frequency transformer for temporal action localization. IEEE Trans. Circuits Syst. Video Technol. 34 (6), 4625–4638. doi: 10.1109/TCSVT.2023.3326692
Zhang W., Dong L., Xu W. (2022a). Retinex-inspired color correction and detail preserved fusion for underwater image enhancement. Comput. Electron. Agric. 192, 106585. doi: 10.1016/j.compag.2021.106585
Zhang W., Jin S., Zhuang P., Liang Z., Li C. (2023b). Underwater image enhancement via piecewise color correction and dual prior optimized contrast enhancement. IEEE Signal Process. Lett. 30, 229–233. doi: 10.1109/LSP.2023.3255005
Zhang Y., Li Q., Qi M., Liu D., Kong J., Wang J. (2023d). Multi-scale frequency separation network for image deblurring. IEEE Trans. Circuits Syst. Video Technol. 33, 5525–5537. doi: 10.1109/TCSVT.2023.3259393
Zhang D., Wu C., Zhou J., Zhang W., Lin Z., Polat K., et al. (2024). Robust underwater image enhancement with cascaded multi-level sub-networks and triple attention mechanism. Neural Networks 169, 685–697. doi: 10.1016/j.neunet.2023.11.008
Zhang D., Zhou J., Zhang W., Lin Z., Yao J., Polat K., et al. (2023a). Rex-net: A reflectance-guided underwater image enhancement network for extreme scenarios. Expert Syst. Appl. 231, 120842. doi: 10.1016/j.eswa.2023.120842
Zhang W., Zhou L., Zhuang P., Li G., Pan X., Zhao W., et al. (2023c). Underwater image enhancement via weighted wavelet visual perception fusion. IEEE Trans. Circuits Syst. Video Technol. 34 (4), 2469–2483 doi: 10.1109/TCSVT.2023.3299314
Zhang W., Zhuang P., Sun H.-H., Li G., Kwong S., Li C. (2022b). Underwater image enhancement via minimal color loss and locally adaptive contrast enhancement. IEEE Trans. Image Process. 31, 3997–4010. doi: 10.1109/TIP.2022.3177129
Zhou J., Li B., Zhang D., Yuan J., Zhang W., Cai Z., et al. (2023). Ugif-net: An efficient fully guided information flow network for underwater image enhancement. IEEE Trans. Geosci. Remote Sens. 61, 1–17. doi: 10.1109/TGRS.2023.3293912
Zhu J.-Y., Park T., Isola P., Efros A. A. (2017). “Unpaired image-to-image translation using cycleconsistent adversarial networks,” in Proceedings of the IEEE international conference on computer vision. p. 2223–2232.
Keywords: underwater image enhancement, underwater human-robot interaction, multi-scale knowledge, multi-frequency extraction, convolutional attention, deep learning
Citation: Kong D, Zhang Y, Zhao X, Wang Y and Cai L (2025) MUFFNet: lightweight dynamic underwater image enhancement network based on multi-scale frequency. Front. Mar. Sci. 12:1541265. doi: 10.3389/fmars.2025.1541265
Received: 07 December 2024; Accepted: 20 January 2025;
Published: 11 February 2025.
Edited by:
Zhibin Yu, Ocean University of China, ChinaReviewed by:
Peixian Zhuang, Tsinghua University, ChinaHao Wang, China University of Petroleum, China
Copyright © 2025 Kong, Zhang, Zhao, Wang and Cai. This is an open-access article distributed under the terms of the Creative Commons Attribution License (CC BY). The use, distribution or reproduction in other forums is permitted, provided the original author(s) and the copyright owner(s) are credited and that the original publication in this journal is cited, in accordance with accepted academic practice. No use, distribution or reproduction is permitted which does not comply with these terms.
*Correspondence: Xiaohu Zhao, emhhb3hpYW9odUBjdW10LmVkdS5jbg==